Agent-Based Social Simulation of the Covid-19 Pandemic: A Systematic Review

,
and
Malmö University, Sweden
Journal of Artificial
Societies and Social Simulation 24 (3) 5
<https://www.jasss.org/24/3/5.html>
DOI: 10.18564/jasss.4601
Received: 20-Dec-2020 Accepted: 28-Apr-2021 Published: 30-Jun-2021
Abstract
When planning interventions to limit the spread of Covid-19, the current state of knowledge about the disease and specific characteristics of the population need to be considered. Simulations can facilitate policy making as they take prevailing circumstances into account. Moreover, they allow for the investigation of the potential effects of different interventions using an artificial population. Agent-based Social Simulation (ABSS) is argued to be particularly useful as it can capture the behavior of and interactions between individuals. We performed a systematic literature review and identified 126 articles that describe ABSS of Covid-19 transmission processes. Our review showed that ABSS is widely used for investigating the spread of Covid-19. Existing models are very heterogeneous with respect to their purpose, the number of simulated individuals, and the modeled geographical region as well as how they model transmission dynamics, disease states, human behavior, and interventions. To this end, a discrepancy can be identified between the needs of policy makers and what is implemented by the simulation models. This also includes how thoroughly the models consider and represent the real-world, e.g., in terms of factors that affect the transmission probability or how humans make decisions. Shortcomings were also identified in the transparency of the presented models, e.g., in terms of documentation or availability, as well as in their validation, which might limit their suitability for supporting decision-making processes. We discuss how these issues can be mitigated to further establish ABSS as powerful tool for crisis management.Introduction
Since the Covid-19 disease was first identified in December 2019, it has spread almost around the entire world and become a global pandemic causing approximately 75 000 000 cases and 1 600 000 deaths within the first 12 month (Dong et al. 2020). To contain the spread of the responsible SARS-CoV-2 virus, different strategies were adopted worldwide, which then had to be revised in accordance with new insights. Moreover, interventions that were found to be successful in some regions were less expedient in others (Chu et al. 2020; Hale et al. 2020). This can be due to, for instance, socio-demographic characteristics of the population, cultural differences in the way of life or individual behavior, and country-specific variations in infrastructure and medical care capacities (Pullano et al. 2020).
Since the outbreak of the pandemic, many of simulation studies have been conducted to investigate different aspects of the disease spread, the resulting hospital occupancy, or economic effects (Currie et al. 2020; Nicola et al. 2020). Many of these studies were based on traditional mathematical macromodels that are not capable of simulating individual behavior (Shinde et al. 2020). In contrast, the microscopic simulation of individual interactions and decisions allows for a more in-depth analysis of the effects that different interventions have on transmission processes.
Agent-based simulation is well-suited for investigating social phenomena, such as the spread of diseases among a population of autonomous individuals with heterogeneous characteristics and behavior (Davidsson 2002; Gilbert 1999). The related research area is often referred to as Agent-based Social Simulation (ABSS). Similar to other approaches for fighting Covid-19 (e.g. Chen et al. 2020 and Nguyen et al. 2020), ABSS makes use of Artificial Intelligence to imitate human-like behavior and decision-making. This, for instance, allows for assessing different potential interventions in a cost- and time-efficient way. With regard to the Covid-19 pandemic, ABSS enables the consideration of different risk or age groups, individual progress of the disease, medical conditions, and compliance with interventions, but also the definition of personal contact networks such as households or workplaces, as well as daily routines. Thereby, ABSS can contribute to a better understanding of the pandemic and identifying suitable interventions for containing the spread of the virus (Squazzoni et al. 2020).
In order to better understand how ABSS can be used in pandemics, we performed a systematic review to identify agent-based models of Covid-19 transmission, which can be used to investigate the introduction, management, or removal of different interventions. We provide an analysis of these models including a comparison of the interventions that can be investigated, inputs to the transmission model, characteristics of individuals, and modeled disease states.
The research questions that we aim to answer with this study include the following:
- For what purpose has ABSS of Covid-19 transmission been used during the pandemic?
- How is the behavior of individuals modeled and how do they make decisions?
- What population size and geographical area are simulated?
- How are transmission processes between individuals modeled?
- How are disease states and the progress of the disease modeled?
- Which interventions are investigated and how do researchers assess the feasibility of these interventions?
- How is the trustworthiness of the models and the generated results ensured, e.g. for the use by decision and policy makers?
Search Strategy and Selection Criteria
Since most research on simulating the spread of Covid-19 is not yet formally published, we decided to also search preprint repositories. We used the COVIDScholar.org search engine, which applies natural language processing to identify and to collect research papers related to Covid-19 from different relevant publishers, databases, and preprint repositories such as PubMed, arXiv, and medRxiv. The search string included the terms “simulation”, “agent”, and “transmission.”1 To complete the findings, we performed both iterative forward and backward snowballing to find additional relevant articles (Wohlin 2014). The review has been conducted according to the PRISMA guidelines (Liberati et al. 2009), and the flow diagram of the selection process is shown in Figure 1.
Articles were included in the review based on the following eligibility criteria:
- Published in English;
- Published in a journal or proceedings, or available as preprint in a recognized archive (i.e. PubMed, arXiv, medRxiv);
- Uses an ABSS model that allows for investigating the spread of Covid-19, i.e. a micro-level model where the identity and status of each individual can be tracked throughout the simulation;
- Article describes the simulation model and the transmission process;
- For each model and each team of authors, only the latest version of the preprint or, if existing, the peer-reviewed article is included;
- Uploaded or published earlier than October 1, 2020 (articles published after this date are considered if a preprint was published before).
Information regarding the presented simulation models was extracted from the articles using a list of characteristics that was developed and extended based on a first review of all identified articles (see Appendix I). The assessment was conducted based on the articles and, if available, on supplemental materials such as technical descriptions that were published with the article. As most models were not available for download, the source code was not reviewed for further insights in the models’ functionalities. Whenever the assessment of a particular feature was ambiguous, the article was discussed among all authors. The detailed results of the analysis are shown in Appendix II and a list of all reviewed articles can also be found in Appendix III.
The consideration of articles published in English language, the limitation of the study’s time period to October 2020, and the inclusion of non peer-reviewed articles may have introduced bias and affected the results. However, even though preprints do not necessarily fulfill the same requirements as a scientific article, they provide a complementary perspective on existing modeling approaches.
Classification of the Articles
To answer the research questions, 72 attributes were extracted and analyzed for the different models. These include both simple attributes, where the existence of a particular functionality is mostly assessed as either true or false, and more complex attributes where a textual description is provided on how and to what extent the model implements or corresponds to the attribute. All classifications that are presented in this study refer to both how the model has been used in the article and what the authors claim the model can be used for. In Table 1, an overview of all attributes is presented. A more comprehensive codebook can be found in Appendix I, which also includes a definition of each attribute, as well as the coding of the assessment.
Category | Attributes |
---|---|
Article | Authors, Date published, Peer-reviewed |
Purpose | NPI introduction, NPI adaptive management, NPI removal, PI introduction, PI adaptive management, PI removal |
Non-pharmaceutical interventions (NPI) | Lockdown, Curfew, Limitation of public gatherings, Limitation of private gatherings, Quarantine/Isolation, Social/physical distancing, Face masks, Closure of schools, Closure of universities, Closure of all workplaces, Closure of offices, Closure of leisure, Closure of shopping, Mobility restrictions/travel bans, Contact tracing |
Pharmaceutical interventions (PI) | Vaccination, Treatment |
Input | Census data, Mobility data, GIS data |
Output | #infections, #deaths, #hospitalized, Infection chains, Economic effects |
Transmission model | Progress of disease (State), Progress of disease (Time since infection), Age or age group, Location, Distance, Density, Contact or exposure time, Protection, Other |
Attributes of individuals | Age or age group, Gender, Workplace, Profession, Household, Location, Contacts, Contact rate, Protection, Health status, Other |
Disease status | Susceptible, Exposed, Infected, Infected without symptoms, Infected with symptoms, Severely ill, Critically ill, Dead, Recovered |
Model characteristics | Name of the model, Number of individuals, Region, Framework, Accessibility, Agent behavior, Validation, Calibration |
For each article, the names of the authors, whether or not the article has been peer-reviewed, and the date when it was first published are provided. For articles that were uploaded to open-access archives, the upload date of the latest version is provided, however, this cannot be later than December 1, 2020, when this study was conducted.
We distinguish between different purposes of the models. This can be the investigation of the spread of the virus as well as of the effects of non-pharmaceutical (NPI) or of pharmaceutical interventions (PI) over time. Moreover, we distinguish between the introduction, adaptive (dynamic) management, and removal of these interventions. There is a total of 16 different NPIs and two PIs that were simulated, e.g. lockdowns, face masks, or vaccinations.
In terms of the input data that are used for initializing the model, we distinguish between three different types of data: socio-demographic (census) data, mobility data on movement patterns, and spatial GIS data of an area. Accordingly, we also analyze the output data provided by the models, e.g. in terms of the reported performance measures such as number of infected, hospitalized, or deceased individuals, but also more comprehensive output data such as infection chains or economic effects.
The transmission model, which defines how transmissions occur between individuals, can include different attributes and factors when determining the contagion probability. In our study, we identified a total of eight common factors that might positively or negatively affect the probability of an individual being infected when meeting an infected individual, e.g. the progress of the disease, the distance between the individuals, or the exposure time. In addition, this study also investigates what personal attributes are used to describe properties of individuals. In total, there are ten different personal traits that were used in the models, e.g. age, health status, or the wearing of protective equipment such as face masks.
Compartment models are often used to model individual disease progress and disease states (Brauer 2008). Each phase of the disease describes the condition of the individual, e.g. the need of medical care or the occurrence of symptoms, but also its capability of infecting others. This study assesses nine different disease states ranging from susceptible to deceased with different infection states.
Finally, we summarize other relevant model characteristics that cannot be assigned to the previously described categories. This includes more technical properties of the models, e.g. the number of simulated individuals, the used framework, or the accessibility of the model via download, but also information on how agent behavior is modeled and how the model has been calibrated and validated.
Results
Based on the previously defined criteria, the following 126 articles were identified and further investigated: Abdollahi et al. (2020); Ahmed et al.(2020); Akay & Barbastathis(2020); Akbarpour et al.(2020); Alagoz et al.(2021); Aleta et al.(2020); Almagor & Picascia (2020); Alsing et al. (2020); Azzimonti et al. (2020); Bahl et al. (2020); Barrett et al. (2020); Bhattacharyya & Vinay (2020); Bicher et al. (2020); Bisin & Moro (2020); Biswas & Sen (2020); Block et al.(2020); Bossert et al.(2020); Bouchnita & Jebrane (2020); Braun et al. (2020); Brootherhood et al. (2020); Buhat et al. (2020); Cencetti et al. (2021); Chang et al. (2020a); Chang et al. (2020b); Chao et al. (2020); Churches & Jorm (2020); Coletti et al. (2020); Cremonini & Maghool (2020); Cuevas (2020); Davids et al. (2020); Dignum et al. (2020); D’Orazio et al. (2021); Eilersen & Sneppen (2020); Elbanna et al. (2020); Espana et al. (2020); Fang et al. (2020); Ferguson et al. (2020); Fiore et al. (2020); Firth et al. (2020); Gardner et al. (2020); Gasparek et al. (2020); Gaudou et al. (2020); German et al. (2020); Giacopelli (2020); Goldenbogen et al. (2020); Gomez et al. (2020); Gopalan & Tyagi (2020); Grauer et al. (2020); Gressman & Peck (2020); Gutin et al. (2020); Head et al. (2020); Hellewell et al. (2020); Herbrich et al. (2020); Hernández-Orallo et al. (2020); Herrmann & Schwartz (2020); Hinch et al. (2020); Hoertel et al. (2020); Huang et al. (2020); Jackson (2020); Jalayer et al. (2020); Jenness et al. (2020); Kai et al. (2020); Kano et al. (2020); Karaivanov (2020); Karatayev et al. (2020); Karin et al. (2020); Kartha & Pathan (2020); Kerr et al. (2020); Klôh et al. (2020); Kolumbus & Nisan (2020); Koo et al. (2020); Kretzschmar et al. (2020); Kucharski et al. (2020); Kwon et al. (2020); Leng et al. (2020); Li et al. (2020); Lorch et al. (2020); Mahdizadeh Gharakhanlou & Hooshangi (2020); Maheshwari & Albert (2020); Mahmood & Dabdawb (2020); Mahmood et al. (2020); Manout et al. (2020); Manzo & Rijt (2020); Marquioni & de Aguiar (2020); Martos et al. (2020); McCombs & Kadelka (2020); Michaels & Stevenson (2020); Milne & Xie (2020); Mohsen & Alarabi (2020); Müller et al. (2020); Nadini et al. (2020); Nande et al. (2020); Narassima et al.(2020); Neilan et al. (2020); Ng et al. (2020a); Ng et al. (2020b); Núñez-Corrales & Jakobsson (2020); Peak et al. (2020); Pescarmona et al. (2020); Phillips et al. (2020); Pollmann et al. (2020); Rajabi et al. (2020); Rechtin et al. (2020); Reich et al. (2020); Rockett et al. (2020); Rosenstrom et al. (2020); Scabini et al. (2020); Silva et al. (2020); Situngkir (2020); Small & Cavanagh (2020); Son & RISEWIDs Team (2020); Thompson et al. (2020); Topirceanu et al. (2020); Tuomisto et al. (2020); Wagner et al. (2020); Wallentin et al. (2020); Wang et al. (2020); Wells et al. (2020); Wells & Lurgi (2020); Wilder et al. (2020); Willem et al. (2020); Xiao et al. (2020); Xue et al. (2020); Yang et al. (2020); Zhang et al. (2020); Zhao (2020).
Out of these 126 articles, 47 articles (37.3%) have been published in peer-reviewed journals or proceedings. This includes journals from different disciplines such as medicine, biology, computer science, and multi-disciplinary journals. The remaining articles were mostly published via preprint repositories, such as arXiv and medRxiv. The earliest article included in this study was published on February 2, 2020 while the latest article is scheduled for publication on February 15, 2021 (a preprint was uploaded to arxiv.org in May 2020). Only 10 articles (7.9%) were made available as preprints or published prior to April 2020. Considering the early phase of the pandemic, the high number of non-peer-reviewed publications is unsurprising. On one hand, it was the primary goal of the researchers to provide useful simulation models on the short-term, rather than writing high-quality publications. It can be assumed that such publications will follow once the peak of the pandemic is over. On the other hand, especially in the beginning of the pandemic, available data and insights on the spread of the virus changed almost on a daily basis, thus requiring the adaption of models. Hence, due to long reviewing times of scientific publications, the publication of preprints seems more feasible as these can be updated more easily.
The general purpose of all models is to estimate the spread of Covid-19 over time. Their specific purpose can be distinguished in two ways: by the modeled interventions – i.e. no interventions, non-pharmaceutical interventions (NPI), or pharmaceutical interventions (PI) – and by their application, i.e. introduction, adaptive management, or removal of interventions. The vast majority of the models (119, 94.4%) were used for simulating the effects of one or multiple NPIs, whereas eight models (6.3%) support the simulation of PIs. Out of these, two models (1.6%) consider PIs only, whereas the other models also include NPIs. Five models (4.0%) do not explicitly simulate any interventions, just the spreading of the virus. As illustrated in Figure 2, 115 models (91.3%) of the 119 models that simulate NPIs can be used for analyzing the introduction of NPIs; eight models (6.4%) for the adaptive management, i.e. dynamic introduction and removal based on certain criteria; and 28 models (22.2%) were used for the simulation of exit strategies and removal of NPIs. In total, 35 of the 126 analyzed models (27.8%) have multiple purposes and can simulate different combinations of interventions and applications. As most of the investigated models were developed in the beginning or early phase of the pandemic, the focus on the introduction of NPIs is not surprising. During this time, it became clear that the rapid spread of the virus was a challenge to hospital capacity and, thus, had to be contained by means of interventions. However, the small number of models for simulating the effects of vaccinations on the spread of the virus is surprising. Even though WHO stated already in February 2020 that a vaccine might be available within 18 months, we could not identify any agent-based models that can be used for simulating different vaccination strategies.
There are two different PIs whose effects were investigated by the analyzed models: preventive vaccinations and acute treatments. In contrast, there is a variety of NPIs that have been simulated. As shown in Figure 3, most articles (60, 47.6%) analyze the effects of quarantining and isolation of (potentially) infected individuals, followed by 56 articles (44.4%) that analyze social distancing. For both these NPIs, about 90% of the articles indicate that a clear positive effect is achieved with respect to limiting the transmission. This also includes, for instance, voluntary home quarantine once an individual experiences symptoms or when a certain number of personal contacts have been infected. Testing (33.3%) and tracing (31.0%) are often analyzed together, such that tracing of contacts results in them being tested and quarantined if necessary. More than half of the articles that implement either of these two NPIs (54 articles, 42.9%) include both of them (28 of 54, 51.9%). Testing, usually in combination with quarantining, seems to be the NPI with the clearest positive effect, according to the reviewed papers.
The closure of different types of facilities – such as schools, universities, workplaces, leisure, and shopping – is another well-studied NPI. In total, 32 articles (25.4%) analyze closure of at least one type of facility and 18 articles (14.3%) the closure of multiple types of facilities. The closure of schools, including preschools, is most common (19.1%), followed by all work-places (9.5%), universities (7.1%), and offices (7.1%). A distinction is made between workplaces in general and offices where employees can work from home. As a compromise between remote learning and opening schools for all children, some countries have discussed shift operation. Here, the class will be divided into groups and that alternate between remote learning and being at school. Through this, keeping social distance is facilitated, the number of potential contacts is reduced, and the consequences of a potential infection are lower. In our study, we found that most interventions are simulated in an “all or nothing” manner, i.e. schools or workplaces are either entirely closed or opened for all. Hybrid forms or shift operations, which seem most promising, are usually not modeled. This is, probably, due to the differentiated and more sophisticated modeling that is required. It is also interesting to note that some interventions that have been frequently applied in reality, such as curfews and limiting the size of public and private gatherings, were studied in very few articles. A reason for this could be that the modeling of individual behavior needs to be more detailed and the time step resolution finer than in most of the analyzed simulation models.
Apart from the simulation model itself, the quality and credibility of the results also strongly depend on the quality of input data that are used to configure and adapt the model to the circumstances or the environment that is to be simulated (Bonabeau 2002). Viral transmission between individuals depends on socio-demographic attributes such as age and household size as well as on movement behavior. It can be assumed that simulations making use of real data on the behavior and characteristics of individuals are more successful in generating credible results. In total, 55 of the analyzed models (43.7%) apply real-world census data for generating an artificial population such that the socio-demographic features of the modeled individuals correspond to those of the population of the simulated region or country. Overall, 28 models (22.2%) make use of real-world mobility data, e.g. cellphone data, for generating movement profiles between different locations, such as home, workplace, and leisure activities. To adequately model neighborhoods and distances, 13 models (10.3%) use GIS data for generating a realistic model of the environment. It should be noted, however, that simulations not using real input data may still be useful for getting a general understanding of the effects of different interventions under different circumstances.
For modeling disease states, most of the analyzed models adapt variations of the SIR compartment model (Kermack & McKendrick 1927). As shown in Figure 4, the progress of the disease is described by a number of discrete states, i.e. susceptible individuals that can be infected, different incubation and severity states after an infection occurred, and potential outcomes after an infection such as recovery or dead. Altogether, 123 (97.6%) of the analyzed models include a susceptible state whereas the remaining simulations only focus on already infected individuals. In total, 80 models (63.5%) consider an incubation period after being exposed to the virus in which individuals carry the virus but neither infect others and nor show symptoms. When becoming infectious, 60 models (47.6%) distinguish between being symptomatic or asymptomatic, which can either be consecutive or exclusive. In addition to the classical SIR model, 43 models (34.1%) define states for severely ill and 29 models (23.0%) for critically ill individuals that require hospital or ICU treatment. A state for deceased individuals is considered by 51 models (40.5%).
Due to the identified differences in the applied disease models, the purpose and application area of the models differ. When investigating the effects of different interventions, one might also be interested in the impact on the healthcare system. However, the majority of the models do not consider individuals becoming severely ill such that they require hospital or ICU treatment. Moreover, most models do not include the risk of reinfections. In the early phase of the pandemic, it was assumed that antibodies would prevent a second infection, yet new studies show that there is a risk of reinfection, which might be due to mutations of the virus, a mild first infection, or as antibodies disappear.
Traditionally, state transition in SIR models is implemented by means of transition rates and probabilities of disease transmission. In agent-based simulations, personal attributes or circumstances can be used to calculate individual transition probabilities. Figure 5 provides an overview of attributes used in the transmission models of the investigated simulations. In 34 models (27.0%), the likelihood of infecting others upon contact varies depending on the specific disease state of the infecting individual, e.g. whether an individual is asymptomatic or symptomatic, or on the current location where the contact takes place. The time since the infected individual itself was infected or the age or age-group of either the infecting or infected individual both affect the transmission probability in 22 models (17.5%) respectively. Other common factors that affect the likelihood of transmission are the distance between the individuals (17.5%), the density of people at a location (13.5%), or contact time (7.1%) once a contact occurs. It should be noted, however, that when investigating the effect of social distancing, some models only consider encounters as contacts within a certain distance. Here, the actual distance between the individuals does not necessarily affect the transmission probability. To simplify and combine different factors that might affect the infection probability at specific locations, e.g. at workplaces or during outside activities, 35 models (27.8%) include location-specific transition probabilities. In 37 models (29.4%), uniform transmission probabilities are applied such that the likelihood of infecting others is always the same, despite individual factors such as the location, health condition, or contact duration, or no detailed description of the transmission models are provided. The effect that different mutations of the virus might have on the transmission process has not been investigated or discussed in any of the articles. The stage of infection and the time since infection obviously have a major impact on the likelihood of infecting other individuals. However, other widely discussed factors, such as the distance between individuals, which is also used as an indicator for tracking contacts using smartphone apps, are only considered by a smaller number of models. This is because most models lack a fine-grained representation of the actual position of individuals. Instead, most models make use of contact networks or gathering points to simulate interactions between individuals. This limits the models’ suitability to simulate some types of interventions, e.g. the introduction of tracing apps.
In addition to the described individual disease states, many models add further traits to individuals to make the population more heterogeneous and similar to an actual population. As shown in Figure 6, common attributes include age or age group (44.4%), assigned household (42.1%), workplace (35.7%), and the individual’s current location (35.7%). Some models define specific networks of other individuals that the agent can or will have contact with (39.7%). This contact network is sometimes stratified into household contacts, workplace contacts, or random encounters. Despite 15.9% of the articles claiming to simulate face masks or other personal protection, only 13 models (10.3%) include an attribute whether or not individuals are wearing protection. In the remaining models, the simulation of the effect of protective equipment consists of changing global transmission probability parameters or assuming that the entire population is wearing protective equipment. In 16 models (12.7%), no description of personal attributes is provided.
For interventions to be successful, it is essential that individuals comply with them. In most simulations, it is assumed that all individuals will comply with any given intervention without exceptions. In reality, however, individuals tend to violate restrictions, such as limitations of gatherings, lockdowns, or the requirement to wear a face mask. To simulate obedience and disobedience to norms, more advanced models are required that consider personality traits as well as needs of individuals. However, our results show that personality traits are not among the attributes that are used to describe and characterize individuals.
As outlined by Squazzoni et al. (2020), the modeling of realistic human social behavior is crucial for simulating the dynamics of the Covid-19 pandemic. One major challenge is the modeling of decision-making processes and the actions that result from an individual’s perception of a situation, as well as the individual’s attributes. Approaches and architectures that can be used for modeling agent behavior differ greatly and range from homogeneous reactive behavior patterns, which are more rule-based, to sophisticated deliberation processes that are based on individual needs or the perceived utility of possible actions (Russell & Norvig 2016). As shown in Figure 7, there are differences in how the behavior of the individuals is modeled. This includes how sophisticated the underlying decision-making is and whether or not a uniform behavior model is used for all individuals. Of the articles studied, both Kai et al. (2020) and Pollmann et al. (2020) present two different versions of their models with different agent behavior, which have also been included in this analysis.
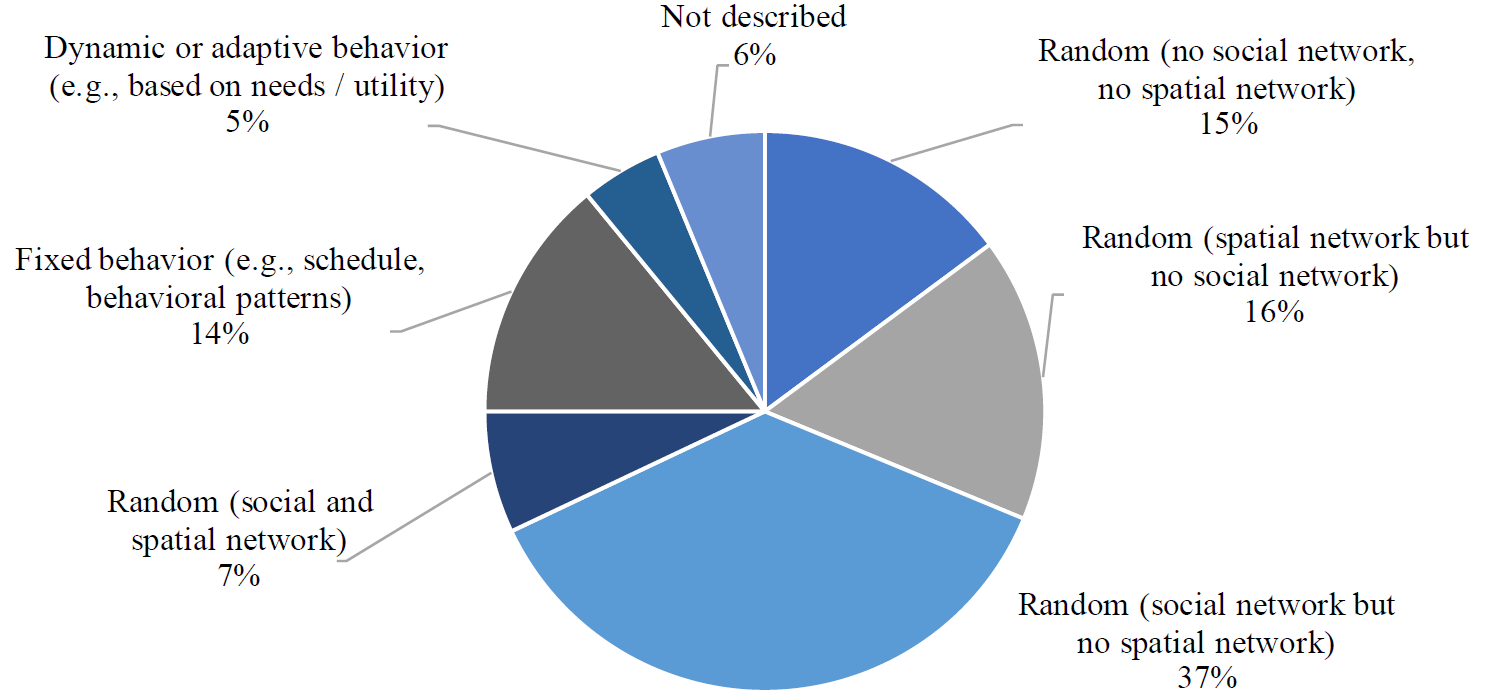
In total, 19 models (15.1%) consist of very simplistic behavior models where individuals randomly infect other individuals in the population. In these models, there are neither social networks nor spatial networks. Hence, there is an equal probability of infecting any individual within the population as there is no representation of locations, contexts, or interpersonal relationships. Overall, 95 models (75.4%) make use of either social networks (37.3%), spatial networks (16.7%), or both (7.1%) to model the individual behavior. Here, agents can only infect other individuals, either when they meet due to a social relation (e.g. household members or co-workers), or as they visit the same location at the same time (e.g. gatherings at home, at work, or at a shop). Most of the solely spatial models are random walk models, where individuals randomly wander in a specific area and transmission might occur once their distance falls under a certain threshold value (e.g. 2 meters). In all, 18 models (14.3%) make use of more explicit behavioral patterns that are mostly derived from empirical data or pre-defined (static) schedules for each individual, for instance, based on its age, personal status, or employment status. Only 6 models (4.8%) include more advanced dynamic or adaptive behavior models. Here, the agents perceive their current situation and assess potential actions to identify the one that is most rewarding or suitable.
The range of interventions that can be analyzed using the different models is strongly limited by design. A high degree of randomness in contacts between individuals facilitates the simulation of large populations. However, in the real world, the probability of people meeting or interacting depends greatly on their location, their routines, and their contact networks. Simple random walk models might be sufficient to get a first impression of the potential dynamics of the pandemic. However, it limits the possibilities of simulating interventions such as quarantining or closure of certain facilities. Models consisting only of social networks are well-suited for simulating transmission processes in households or at workplaces. However, it cannot be simulated when two individuals are at the same location at the same time, thus being able to infect each other. Likewise, limiting the model to the representation of spatial networks does not allow for the representation of fixed households or work colleagues, which are relevant transmission hotspots. For an in-depth analysis of how different interventions affect the behavior and individuals and thus the spread of the virus, more sophisticated behavioral models are required that adequately represent daily routines and activities. However, this is only provided by a few models.
In 82 articles (65.1%), a specific country or region is simulated. As shown in Figure 8, the most studied countries are the United States of America (22 articles), the United Kingdom (11), China (7), and Italy (6). There might be a bias towards English-speaking countries due to the limitation of the study to articles that are written in English. Other than geographical locations, settings studied included hospitals (Huang et al. 2020; Martos et al. 2020), campuses and universities (D’Orazio et al. 2021; Gressman & Peck 2020), general indoor areas (Cuevas 2020), and the Diamond Princess (Fang et al. 2020; Jenness et al. 2020), a cruise ship that was quarantined in the Port of Yokohama in Japan in February 2020 due to a Covid-19 outbreak on board.
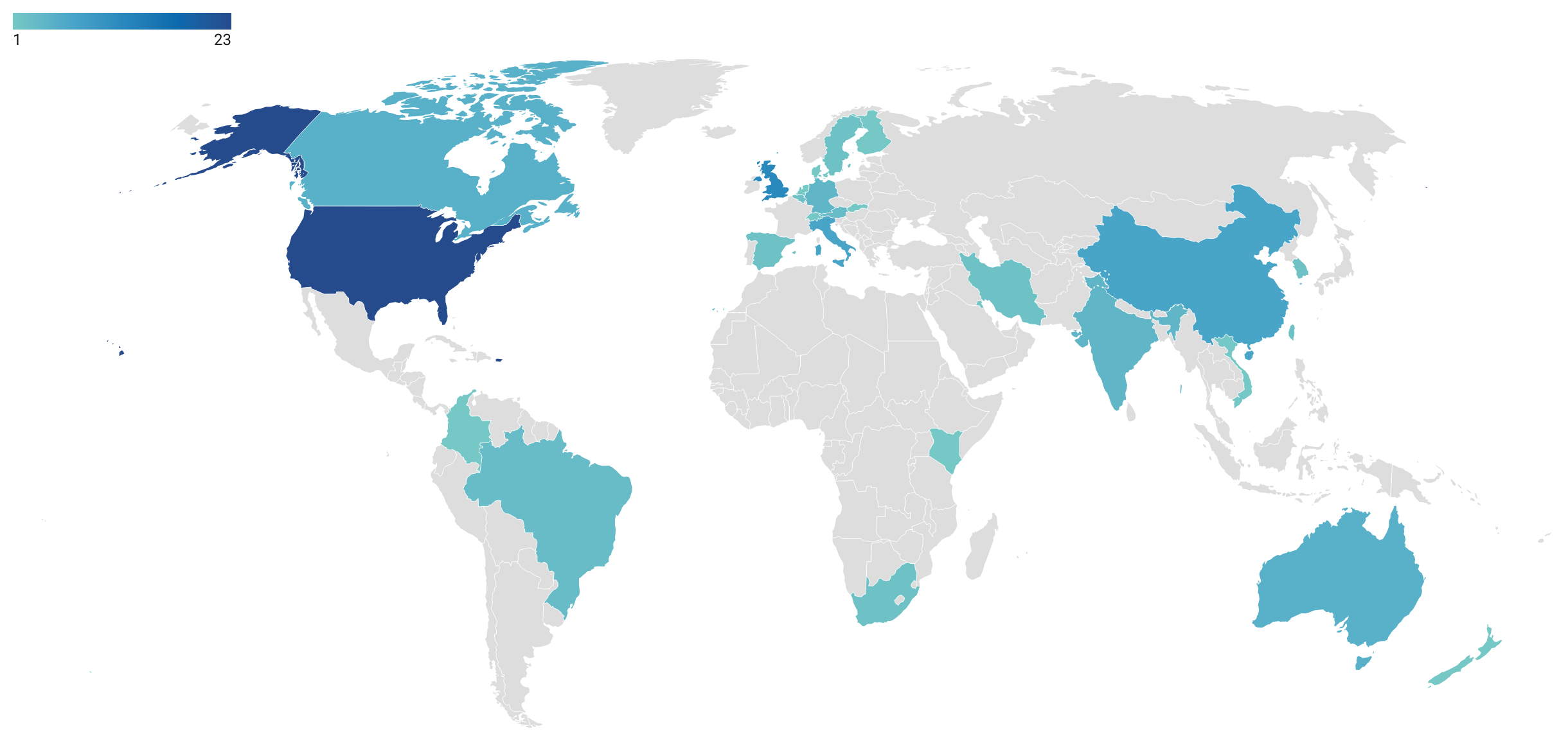
In Figure 9, an overview of the number of simulated agents is provided. Altogether, 94 articles (74.6%) state how many agents were simulated and the number ranges from six to 60 000 000. From those models that provide information on the population size, seven models (7.3%) consist of less than 500 agents and 12 models (12.8%) of more than 5 000 000. The median of simulated agents is 30 000. The considerable difference in population size results from a multiple of the aforementioned aspects. Usually, a trade-off needs to be made between the level of detail of the modeled individuals, as well as the complexity of their behavior, the time step size, and the number of simulated individuals. A simulation of individuals with a sophisticated needs model that consists of rather narrow time-steps, e.g. 1 hour ticks, needs to be limited in the number of individuals with respect to the time and resources required to execute the model. Finding a suitable balance between level of detail and number of individuals strongly depends on the interventions that are studied.
In addition to the described attributes, some other observations were made during the analysis of the included articles. For instance, there are significant differences in the extent and rigor of the models’ documentation, such that some articles had to be excluded from this review due to a lack of information on the model’s fundamental functionalities. This concerns both peer-reviewed and non-peer-reviewed articles, and includes the assumptions that were made regarding the behavior of individuals or Covid-19 transmission dynamics. Standardized protocols that describe the structure of the model, e.g. the ODD protocol (Grimm et al. 2020), are rarely used and only 29.4% of the models are available for download. Though some studies reused existing models, the majority of articles developed new models.
In a literature study by Heath et al. (2009), it was shown that 65% of the investigated articles presenting agent-based models were lacking a thorough validation. Thus, with respect to the trustworthiness of the models and the generated results, we also investigated whether and to what extent the simulations were validated. As shown in Figure 10, the majority of the articles do not elaborate on how the presented model or the generated results were validated. The remaining articles (16.7%) primarily make use of real-world data for assessing the validity of their models by, for instance, comparing the transmission dynamics and the course of the pandemic as observed in the simulation to data from reality. This includes data from reports or surveys, e.g. on the number of infections, hospitalization, or death rates, as well as other epidemic parameters, such as the doubling period or reproduction number (R0). When analyzing phenomena that have not been broadly studied, the availability of real-world data might be limited; thus, it is challenging to validate the models. Hence, four models (3.17%) compare the behavior of their model against other models. The suitability of this approach for validating simulation models has been, for instance, described by Axtell et al. (1996) and Sargent (2015). Finally, three models (2.4%) apply systematic testing and experimentation for assessing the quality of their results and two models (1.6%) involve domain experts, e.g. epidemiologists, for validating their models and results.
However, as stated by Beisbart (2019), even results of models that do not comply with the highest validation standards can still provide valuable insights for decisions makers. This is especially relevant in crisis situations, where other approaches for generating insights are limited. Here, simulations can provide different potential explanations for specific phenomena or observations, that can be used by experts to conclude the real explanation.
Discussion
Challenges and opportunities
To support decision making, the results generated by simulation experiments need to be reliable. In ABSS, the design of the simulated population may be a threat to the reliability of the results. In case where the characteristics or behavior of the artificial population do not represent those of the real population in an adequate way, the simulation results’ ability to draw conclusions regarding mechanisms in the real-world system might be limited. Our review showed that 71 articles (56.3%) mention the inclusion of any real-world input datasets, e.g. on individuals, mobility behavior, or geodata. Due to reasons of privacy, for instance, such data are often not available on the individual level, and artificial populations need to be generated based on aggregated data instead. Still, to adequately represent the real-world environment and to be able to draw conclusions regarding the real world, socio-demographic, and behavioral data for the simulated population are required for the model development. However, the sole use of real-world data is not sufficient to ensure the transferability and applicability of the simulation results, as it depends strongly on the quality, source, relevance, and extent of the used data.
In addition to data on the simulated phenomenon, expert knowledge is valuable in order to verify model assumptions and the generated results. In this review, some simulation models were developed solely by computer scientists, engineers, or experts from other technical disciplines. To calibrate the model to observations from the real world, indicators like the basic reproduction number are used. Cross-disciplinary author collectives and the incorporation of medical experts for verifying the plausibility of assumptions made regarding the transmission process seem to be promising steps towards the development of credible models. However, a certain degree of uncertainty remains as some of the underlying mechanisms of the pandemic are still unknown and data are not available.
In contrast to humans, artificial agents are usually modeled to behave rationally. When simulating the introduction of interventions, results might be misleading due to such rational obedience to restrictions. In the real world, it can be observed that humans disregard and violate restrictive interventions such as curfews or the obligation to wear masks. Such deviations from the intended behavior can either be modeled by means of probability distributions or through the explicit representation of personality traits. Either way, data are required concerning under what circumstances and to what extent individuals tend to disobey restrictions.
When developing an ABSS model, a trade-off has to be made between complexity of the individuals and the number of individuals that are simulated. Incorporating multiple personal and environmental variables into the individual decision-making process requires additional computation time. This can be counteracted by reducing the number of simulated individuals or by increasing the size of the time steps. In this study, the analyzed models consist of six to 60 000 000 agents. More sophisticated models of human behavior were mainly found in the models that consist of a smaller number of individuals. The decision regarding how many agents to include depends on the purpose of the simulation. When simulating a specific city to identify a suitable intervention, one might want to simulate individuals living together in households, commuting to workplaces, and leisure activities combined with a sophisticated transmission model. In contrast, when analyzing the effect of travel restrictions for a country, individual commuting routines might not be of high relevance. Still, the effect they have on the overall movement of individuals needs to be modeled correctly to generate realistic and applicable results. With respect to the scalability of the model and the efficiency of computation, such models might choose to implement contacts between individuals based on a network approach. Moreover, different models can be combined so that results from more detailed simulation at city level can serve as inputs for country-wide or transnational simulations.
With respect to improving the transparency, access, and rigor of simulation models that are used to understand the pandemic and to assist policy and decision makers, Squazzoni et al. (2020) formulate three major challenges that need to be addressed: the Covid-19 prediction challenge, the Covid-19 modeling human behavior challenge, and the Covid-19 data calibration and validation challenge.
In their prediction challenge, the authors outline difficulties that might arise when trying to predict the behavior of complex systems. It is the modelers’ goal to minimize limitations of their models by scientifically grounding their assumptions and by calibrating their models with the most accurate data. However, data are often not available, and it is difficult to validate the assumptions made. Our study showed that nearly all investigated models aim at predicting how different interventions might affect the transmission dynamics. This includes the simulation of a certain time period, in which one or multiple interventions can be activated at a specific point in time or when a certain threshold of an epidemic parameter, e.g. a certain number or ratio of infected individuals, is exceeded. It further showed that the simulated time period, i.e. the horizon of prediction, differs greatly between the models. It ranges from a few days up to two years. Most models, however, predict over a time period between three months and one year.
The modeling human behavior challenge addresses the modeling of complex social dynamics, which is of particular relevance when simulating infection dynamics of the Covid-19 pandemic, as well as the effects of interventions. That is, because such dynamics emerge from the individual behavior of humans. However, it is challenging to build cognitive models of human decision making as actions might not always be rational and the heterogeneity of individuals needs to be considered. Building sound models requires not only socio-demographic data on the particular attributes, e.g. age, gender, or household, but also cross-disciplinary expertise. Squazzoni et al. (2020) argue that models that cannot be used to examine social dynamics are lacking a crucial aspect for analyzing and predicting Covid-19 transmissions.
Most models that were investigated as part of this study include rather simplistic models of human behavior. To this end, the actions taken by individuals and the transmission of the virus between the individuals take place randomly. Socio-demographic data are often used to generate a population that corresponds to the real-world population with respect to specific features, e.g. age distribution. However, this information is then primarily used for the global organization of the model, e.g. the composition of households, determining their occupation, or as factors in the transmission model for determining the individual probability of infection. Moreover, interactions in most models are predetermined as a result of social or spatial network graphs. Only a few models include more sophisticated models of human behavior, where schedules, utility, or need models are used for proactively determining suitable actions based on a given situation and personal features. Social dynamics, as described by Squazzoni et al. (2020), are in fact part of many models. However, they are mostly not the result of the individual assessment of a given situation; rather, they are the consequence of a predefined social interaction network. Especially with respect to the simulation of whether or not individuals comply with interventions, more sophisticated decision-making models are required. In particular, compliance with different measures depends on personal characteristics and circumstances, all of which need to be taken into account.
Finally, the data calibration and validation challenge especially arises when using simulations for ex ante analyses with the aim of predicting future developments. This is a result of a lack of data and possibilities for gathering it, e.g. as experiments are either impossible or unethical. To this end, the authors suggest the retrospective validation of models. However, this might not always be feasible when simulation results are required for making timely decisions. Squazzoni et al. (2020) also discuss the quality of available data on the Covid-19 pandemic as metrics, e.g. the number of cases, might be defined and collected differently among different countries. This affects both calibration and validation and it might lead to biased estimations.
Nearly half of the investigated articles outline how their models have been calibrated. This includes determining feasible probabilities for transmission processes as well as for the progress of the disease, e.g. the likelihood of being infected, the transmission rate, the probability of developing a severe progress of disease that requires hospital admission, or the likelihood of dying as a result of the disease. Moreover, calibration is used to adjust social interactions between individuals, that potentially result in infections and the transmission of the virus. This is achieved by, for instance, calibrating the overall contact probability and even the specific weights of edges in network graphs that represent individual transmission or contact probabilities. Approaches that are used for calibrating models include optimization techniques such as Bayesian optimization, Sequential Monte Carlo, maximum likelihood estimation, minimization of the sum of squared residuals, and experimental designs such as Latin Hypercube Sampling. However, the large number of potentially interdependent model parameters makes the calibration challenging.
In accordance with the study by Heath et al. (2009), we also found that the majority of articles (75%) are lacking a description of how the model was validated. Due to the pandemic situation and as most models aim at the ex ante analysis of the effects of different interventions, the availability of data is strongly limited. In addition, the use of early available data, whose quality might be questionable, another approach is the comparison of different models among each other, e.g. in accordance with Axtell et al. (1996) and Sargent (2015). However, as the validity of these models also is uncertain, the results of these comparisons need to be assessed carefully.
Limitations
As the Covid-19 pandemic is still ongoing, the work on novel models and simulations continues, thus resulting in new publications. This literature review analyzes and discusses the state of publications as of October 1, 2020, approximately nine months after the outbreak of the first Covid-19 cases. Due to the high number of preprints that are included in this study, it can be assumed that some of the presented models are relatively quick responses to the pandemic that were developed without comprehensive funding. However, the researchers should still be responsible for the validity and completeness. It can also be assumed that the number of quality-assured publications will increase in the future as a result of extensive peer review processes. In addition, these future articles might present an updated or extended version of the models.
Implications for practice and future research
To simulate transmission processes, the underlying mechanisms that lead to the spread of the disease between individuals need to be modeled. Due to the novelty of Covid-19, the understanding of the infection mechanisms is still limited. Thus modelers are required to make assumptions on how certain mechanisms work and how different parts of the model are interconnected. At a later point, these assumptions might prove to be imprecise or even incorrect, and thus need to be revised. In cases where they have been hard-coded into the model, future users are not able to adjust them, which severely limits the applicability of the model. Instead, modelers and developers should aim at mapping assumptions to model parameters so that they can be easily modified. This is also relevant with respect to the interpretation of the generated results, as it allows for the analysis and comparison of different assumptions.
Instead of explicitly programming all parts of the simulation model or manually setting parameters, these may also be induced from observed data using machine learning techniques. This includes, for instance, information on where infections occur, personal factors that influence the susceptibility, and the likelihood of transmission when having contact with an infected individual. However, data on these mechanisms are still being collected and only limited information is available.
To promote the use of simulation results in policy making, it is not only the model building but also the model description that needs to be improved. In fact, some papers were excluded from the study because of an incomplete or shallow description of the model. To be able to adequately interpret simulation results and to use them as evidence in decision-making processes, the assumptions made by the model, as well as the underlying mechanisms, need to be transparently communicated. This includes not only the possibility to download the model but also a comprehensive description of its entities and their interactions. This can, for instance, be achieved by using the ODD protocol (Grimm et al. 2020). In addition, the thorough documentation of a model’s mechanisms, validation of the model – e.g. by including epidemiological experts in the project team – seems necessary to ensure the correctness and plausibility of model assumptions.
When comparing interventions that are supported by the simulation models with those interventions that are discussed in reality, a discrepancy can be observed. Many simulations model lockdown scenarios, however, complete lockdowns often are considered a last resort. Instead, curfews and limitations of public and private gatherings are common interventions, but only a few models analyze these interventions. This might be due to the increased complexity of the simulation models needed, for instance, in terms of the size of the simulated time steps or the used human behavior models. Simulating the effects of some NPIs requires a more realistic and individual representation of human decision making and norm obedience as well as a more fine-grained time step size. However, most of the investigated models consist of rather simplistic behavior models and simulate on a daily basis. This does not allow for the analysis of more advanced NPIs such as curfews or preventive quarantine.
The great variety of models identified in this study as well as the small number of reused models, also suggests that collaborations and model combinations might allow for the development of more complex models. Combinations of different models were not identified in this literature review.
Conclusions
In this systematic review on ABSS of Covid-19 transmission, we identified and analyzed 126 articles that propose relevant simulation models. The models can be used to investigate different aspects of the ongoing Covid-19 pandemic, including transmission dynamics and interventions for containing the spread of the virus. However, these models differ in the interventions that can be simulated, in the extent of the transmission model, the attributes of the individuals, and the states used for representing the progress of the disease.
Over 90% of the analyzed articles present simulations of at least one NPI and investigate how this affects the dynamics of the pandemic in terms of infected individuals, mortality, or demand on the healthcare system. In addition to the parameters that can be used to adapt the model, more than half of the models include some real-world input datasets of individuals, mobility behavior, or geodata, which allows for adapting the model to the real-world conditions of the area that is simulated. By this means, local circumstances can be modeled and different scenarios of interventions can be investigated prior to their actual introduction. The results generated by the simulations can provide new information on how transmission dynamics are affected under different circumstances. Thus they provide policy makers with additional valuable insights to be used in their decision-making process.
For the conducted literature review on ABSS models for the Covid-19 pandemic, we can summarize the following key messages:
- ABSS offers a more powerful tool to investigate Covid-19 transmission processes between individuals and the effects of interventions compared to traditional macro-level models, e.g. system dynamics or mathematical models. Nevertheless, although we identified 126 published ABSS models for Covid-19 in this review, the full potential of ABSS is yet to be realized.
- A discrepancy can be identified between the needs of policy makers and what the simulation models implement. There is, for instance, a need to consider different configurations of curfews and restrictions of gatherings. The analyzed simulations, however, mainly implement tougher general interventions such as lockdowns and quarantines, which are easier to model. Moreover, the effects of pharmaceutical interventions are rarely studied.
- The effects of the studied interventions are often estimated to be positive, with the exception of lockdowns and closing of schools and universities, where the effects are unclear or negative in 25% or more of the studies.
- Few models include factors that are generally assumed to affect the transmission probability, such as the distance between the individuals (17.5%), density of individuals at the location (13.5%), wearing protection (10.3%), and contact time (7.1%).
- Although age is the most commonly modeled attribute of individuals, more than half of the analyzed models do not consider this attribute in the transmission model, despite it being widely regarded as a critical factor.
- Regarding the modeling of disease states, more than 50% of the models do not distinguish between being symptomatic or asymptomatic. Moreover, less than 25% of the models have a state for being critically ill and requiring hospital or ICU treatment.
- Most models (90.5%) include simplistic models of human behavior, where decisions and actions are mainly random with predefined spatial or social networks. Some models, however, include more sophisticated models of human decision making that make use of individual schedules, needs, or utility functions.
- As the trustworthiness of the ABSS models is a key factor for policy making, the transparency needs to be improved. This can, for instance, be achieved by providing more detailed information about the model’s underlying mechanisms and assumptions made using a standardized protocol (e.g. ODD), and by making the model available for download.
- The validation of simulation models whose purpose is prediction is challenging. This is, as required data are usually not available. In 75% of the investigated articles, there is no information provided on whether or how the model has been validated. This is a drawback with respect to the trustworthiness of the generated results. However, even without sufficient data, validation can consist of systematic testing of the model, comparing it against other models, or expert assessment. Nevertheless, only a few models pursue such approaches.
Our analysis showed that simulation can be used to identify potential effects or side-effects of different interventions against the spread of Covid-19 in an artificial population. However, the results presented by the articles mostly allow for understanding the dynamics of the pandemic and to identify factors that potentially affect these dynamics. Thus the generated results need to be interpreted thoroughly, and their capability of predicting future developments is limited.
This study gives an overview of models and their features but does not assess their trustworthiness. This is, to identify shortcomings in existing models with respect to future research endeavors, as well as to support decision makers in the identification of appropriate models for a given scenario. We conclude that ABSS is a powerful tool to investigate Covid-19 transmission processes and to analyze potential interventions. However, the full potential of ABSS is yet to be realized.
Acknowledgements
This work was supported by Malmö University via the project “Towards More Reliable Predictions: Multi-model Ensembles for Simulating the Corona Pandemic” (Fo 2020/299).Notes
- The exact search string used for searching COVIDScholar.org: "+simulation +agent +transmission".
Appendix
To access the supplementary information file accompanying the manuscript please click here.References
ABDOLLAHI, E., Haworth-Brockman, M., Keynan, Y., Langley, J. M., & Moghadas, S. M. (2020). Simulating the effect of school closure during COVID-19 outbreaks in Ontario, Canada. BMC Medicine, 18(2020), 230. [doi:10.1186/s12916-020-01705-8]
AHMED, D. A., Ansari, A. R., Imran, M., Dingle, K., AHMED, N., & Bonsall, M. A. (2020). Mechanistic modelling of coronavirus infections and the impact of confined neighbourhoods on a short time scale. MedRxiv preprint. Available at: https://www.medrxiv.org/content/10.1101/2020.07.28.20163634v1 [doi:10.20944/preprints202007.0577.v1]
AKAY, H., & Barbastathis, G. (2020). Markovian random walk modeling and visualization of the epidemic spread of COVID-19. MedRxiv preprint. Available at: https://www.medrxiv.org/content/10.1101/2020.04.12.20062927v1. [doi:10.1101/2020.04.12.20062927]
AKBARPOUR, M., Cook, C., Marzuoli, A., Mongey, S., Nagaraj, A., Saccarola, M., Tebaldi, P., Vasserman, S., & Yang, H. (2020). Socioeconomic network heterogeneity and pandemic policy response. NBER, National Bureau of Economic Research. Working Paper 27374. [doi:10.3386/w27374]
ALAGOZ, O., Sethi, A., Patterson, B., Churpek, M., & Safdar, N. (2021). Impact of timing of and adherence to social distancing measures on COVID-19 burden in the US: A simulation modeling approach. MedRxiv preprint. Available at: https://www.medrxiv.org/content/10.1101/2020.06.07.20124859v1. [doi:10.1101/2020.06.07.20124859]
ALETA, A., Martín-Corral, D., Pastore y Piontti, A., Ajelli, M., Litvinova, M., Chinazzi, M., Dean, N. E., Halloran, M. E., Longini Jr, I. M., Merler, S., Pentland, A., Vespignani, A., Moro, E., & Moreno, Y. (2020). Modelling the impact of testing, contact tracing and household quarantine on second waves of COVID-19. Nature Human Behaviour, 4(9), 964–971. [doi:10.1038/s41562-020-0931-9]
ALMAGOR, J., & Picascia, S. (2020). Exploring the effectiveness of a COVID-19 contact tracing app using an agent-based model. Scientific Reports, 10(22235). [doi:10.1038/s41598-020-79000-y]
ALSING, J., Usher, N., & Crowley, P. J. D. (2020). Containing Covid-19 outbreaks with spatially targeted short-term lockdowns and mass-testing. MedRxiv preprint. Available at: https://www.medrxiv.org/content/10.1101/2020.05.05.20092221v2. [doi:10.1101/2020.05.05.20092221]
AXTELL, R., Axelrod, R., Epstein, J. M., & Cohen, M. D. (1996). Aligning simulation models: A case study and results. Computational & Mathematical Organization Theory, 1(2), 123–141. [doi:10.1007/bf01299065]
AZZIMONTI, M., Fogli, A., Perri, F., & Ponder, M. (2020). Pandemic control in ECON-EPI networks. NBER, National Bureau of Economic Research. Working Paper 27741. [doi:10.3386/w27741]
BAHL, R., Eikmeier, N., Fraser, A., Junge, M., Keesing, F., Nakahata, K., & Wang, L. Z. (2020). Modeling COVID-19 spread in small colleges. arXiv preprint. Available at: https://arxiv.org/abs/2008.09597.
BARRETT, K., Khan, Y. A., Mac, S., Ximenes, R., Naimark, D. M. J., & Sander, B. (2020). Estimation of COVID-19-induced depletion of hospital resources in Ontario, Canada. CMAJ, 192(24), 640–646. [doi:10.1503/cmaj.200715]
BEISBART, C. (2019). 'What is validation of computer simulations? Toward a clarification of the concept of validation and of related notions.' In C. Beisbart & N. J. Saam (Eds.), Computer Simulation Validation: Fundamental Concepts, Methodological Frameworks, and Philosophical Perspectives (pp. 35–67). Berlin Heidelberg: Springer International Publishing. [doi:10.1007/978-3-319-70766-2_2]
BHATTACHARYYA, C., & Vinay, V. (2020). Suppress, and not just flatten: Strategies for rapid suppression of COVID19 transmission in small world communities. Journal of the Indian Institute of Science, 100(4), 849–862. [doi:10.1007/s41745-020-00209-x]
BICHER, M. R., Rippinger, C., Urach, C., Brunmeir, D., Siebert, U., & Popper, N. (2020). Agent-Based simulation for evaluation of contact-Tracing policies against the spread of SARS-CoV-2. medRxiv preprint. Available at: https://www.medrxiv.org/content/10.1101/2020.05.12.20098970v2. [doi:10.1101/2020.05.12.20098970]
BISIN, A., & Moro, A. (2020). Spatial-SIR with network structure and behavior: Lockdown policies and the Lucas critique. arXiv preprint. Available at: https://arxiv.org/abs/2103.13789.
BISWAS, K., & Sen, P. (2020). Space-time dependence of corona virus (COVID-19) outbreak. arXiv preprint. Available at: https://arxiv.org/abs/2003.03149.
BONABEAU, E. (2002). Agent-based modeling: Methods and techniques for simulating human systems. Proceedings of the National Academy of Sciences, 99(3), 7280–7287. [doi:10.1073/pnas.082080899]
BOSSERT, A., Kersting, M., Timme, M., Schröder, M., Feki, A., Coetzee, J., & Schlüter, J. (2020). Limited containment options of COVID-19 outbreak revealed by regional agent-based simulations for South Africa. arXiv preprint. Available at: http://arxiv.org/abs/2004.05513. [doi:10.12688/f1000research.28250.1]
BOUCHNITA, A., & Jebrane, A. (2020). A hybrid multi-scale model of COVID-19 transmission dynamics to assess the potential of non-pharmaceutical interventions. Chaos, Solitons & Fractals, 138, 109941. [doi:10.1016/j.chaos.2020.109941]
BRAUER, F. (2008). 'Compartmental models in epidemiology.' In F. BRAUER, P. van den Driessche, & J. Wu (Eds.), Mathematical Epidemiology (pp. 19–79). Berlin Heidelberg: Springer. [doi:10.1007/978-3-540-78911-6_2]
BRAUN, B., Taraktas, B., Beckage, B., & Molofsky, J. (2020). Simulating phase transitions and control measures for network epidemics caused by infections with presymptomatic, asymptomatic, and symptomatic stages. PloS ONE, 15(9), e0238412. [doi:10.1371/journal.pone.0238412]
BROOTHERHOOD, L., Kircher, P., Santos, C., & Tertilt, M. (2020). An economic model of the COVID-19 epidemic: The importance of testing and age-Specific policies. CESifo Working Paper Series 8316, CESifo.
BUHAT, C. A. H., Lutero, D. S. M., Olave, Y. H., Torres, M. C., & Rabajante, J. F. (2020). Transmission of respiratory infectious diseases between neighboring cities using agent-based model and compartmental model. medRxiv preprint. Available at: https://www.medrxiv.org/content/10.1101/2020.06.24.20138818v1. [doi:10.1101/2020.06.24.20138818]
CENCETTI, G., Santin, G., Longa, A., Pigani, E., Barrat, A., Cattuto, C., Lehmann, S., Salathe, M., & Lepri, B. (2021). Digital proximity tracing on empirical contact networks for pandemic control. Nature Communications, 12(1655). [doi:10.1038/s41467-021-21809-w]
CHANG, M. C., Kahn, R., Li, Y. A., Lee, C. S., Buckee, C. O., & CHANG, H. H. (2020). Variation in human mobility and its impact on the risk of future COVID-19 outbreaks in Taiwan. medRxiv preprint. Available at: https://www.medrxiv.org/content/10.1101/2020.04.07.20053439v2. [doi:10.1101/2020.04.07.20053439]
CHANG, S. L., Harding, N., Zachreson, C., Cliff, O. M., & Prokopenko, M. (2020). Modelling transmission and control of the COVID-19 pandemic in Australia. Nature Communications, 11(1), 5710. [doi:10.1038/s41467-020-19393-6]
CHAO, D. L., Oron, A. P., Srikrishna, D., & Famulare, M. (2020). Modeling layered non-pharmaceutical interventions against SARS-CoV-2 in the United States with Corvid. Available at: https://www.medrxiv.org/content/10.1101/2020.04.08.20058487v1. [doi:10.1101/2020.04.08.20058487]
CHEN, J., Li, K., Zhang, Z., Li, K., & Yu, P. S. (2020). A survey on applications of artificial intelligence in fighting against Covid-19. Available at: https://arxiv.org/abs/2007.02202.
CHU, D. K., Akl, E. A., Duda, S., Solo, K., Yaacoub, S., Schünemann, H. J., El-harakeh, A., Bognanni, A., Lotfi, T., Loeb, M., & COVID-19 Systematic Urgent Review Group Effort (SURGE). (2020). Physical distancing, face masks, and eye protection to prevent person-to-person transmission of SARS-CoV-2 and COVID-19: A systematic review and meta-analysis. The Lancet, 395(10242), 1973–1987. [doi:10.1016/j.jvs.2020.07.040]
CHURCHES, T., & Jorm, L. (2020). Flexible, freely available stochastic individual contact model for exploring COVID-19 intervention and control strategies: Development and simulation. JMIR Public Health and Surveillance, 6(3), e18965. [doi:10.2196/18965]
COLETTI, P., Libin, P., Petrof, O., Willem, L., Steven, A., Herzog, S. A., Faes, C., Wambua, J., Kuylen, E. J., Beutels, P., & Hens, N. (2020). A data-driven metapopulation model for the Belgian COVID-19 epidemic: Assessing the impact of lockdown and exit strategies. Available at: https://www.medrxiv.org/content/10.1101/2020.07.20.20157933v1. [doi:10.1101/2020.07.20.20157933]
CREMONINI, M., & Maghool, S. (2020). The unknown of the pandemic: An agent-Based model of final phase risks. Journal of Artificial Societies and Social Simulation, 23(4), 8: https://www.jasss.org/23/4/8.html. [doi:10.18564/jasss.4426]
CUEVAS, E. (2020). An agent-based model to evaluate the COVID-19 transmission risks in facilities. Computers in Biology and Medicine, 121, 103827. [doi:10.1016/j.compbiomed.2020.103827]
CURRIE, C. S. M., Fowler, J. W., Kotiadis, K., Monks, T., Onggo, B. S., Robertson, D. A., & Tako, A. A. (2020). How simulation modelling can help reduce the impact of COVID-19. Journal of Simulation, 14(2), 1–15. [doi:10.1080/17477778.2020.1751570]
DAVIDS, A., Rand, G. D., Georg, C. P., Koziol, T., & Schasfoort, J. A. (2020). SABCoM: A spatial agent-Based Covid-19 model. medRxiv preprint. Available at: https://www.medrxiv.org/content/10.1101/2020.07.3020164855v1. [doi:10.2139/ssrn.3663320]
DAVIDSSON, P. (2002). Agent based social simulation: A computer science view. Journal of Artificial Societies and Social Simulation, 5(1), 7: https://www.jasss.org/5/1/7.html
DIGNUM, F., Dignum, V., Davidsson, P., Ghorbani, A., Hurk, M. van der, Jensen, M., Kammler, C., Lorig, F., Ludescher, L. G., Melchior, A., Mellema, R., Pastrav, C., Vanhee, L., & Verhagen, H. (2020). Analysing the combined health, social and economic impacts of the coronavirus pandemic using agent-Based social simulation. Minds and Machines, 30(2), 177–194. [doi:10.1007/s11023-020-09527-6]
DONG, E., Du, H., & Gardner, L. (2020). An interactive web-based dashboard to track COVID-19 in real time. The Lancet Infectious Diseases, 20(5), 533–534. [doi:10.1016/s1473-3099(20)30120-1]
D’ORAZIO, M., Bernardini, G., & Quagliarini, E. (2021). A probabilistic model to evaluate the effectiveness of main solutions to COVID-19 spreading in university buildings according to proximity and time-based consolidated criteria. Building Simulation. [doi:10.1007/s12273-021-0770-2]
EILERSEN, A., & Sneppen, K. (2020). Cost-benefit of limited isolation and testing in COVID-19 mitigation. medRxiv preprint. Available at: https://www.medrxiv.org/content/10.1101/2020.04.09.20059790v4. [doi:10.1038/s41598-020-75640-2]
ELBANNA, A., Wong, G. N., Weiner, Z. J., Wang, T., Zhang, H., Liu, Z., Tkachenko, A. V., Maslov, S., & Goldenfeld, N. (2020). Entry screening and multi-layer mitigation of COVID-19 cases for a safe university reopening. medRxiv preprint. Available at: https://www.medrxiv.org/content/10.1101/2020.08.29.20184473v1. [doi:10.1101/2020.08.29.20184473]
ESPANA, G., Cavany, S., Oidtman, R. J., Barbera, C., Costello, A., Lerch, A., Poterek, M., Tran, Q., Wieler, A., Moore, S. M., & Perkins, A. (2020). Impacts of K-12 school reopening on the COVID-19 epidemic in Indiana, USA. medRxiv preprint. Available at: https://www.medrxiv.org/content/10.1101/2020.08.22.20179960v2.
FANG, Z., Huang, Z., Li, X., Zhang, J., Lv, W., Zhuang, L., Xu, X., & Huang, N. (2020). How many infections of COVID-19 there will be in the "Diamond Princess" - Predicted by a virus transmission model based on the simulation of crowd flow. arXiv preprint. Available at: https://arxiv.org/abs/2002.10616.
FERGUSON, N., Laydon, D., Nedjati Gilani, G., Imai, N., Ainslie, K., Baguelin, M., Bhatia, S., Boonyasiri, A., Cucunuba Perez, Z., Cuomo-Dannenburg, G., Dighe, A., Dorigatti, I., Fu, H., Gaythorpe, K., Green, W., Hamlet, A., Hinsley, W., Okell, L., Van Elsland, S., … Ghani, A. (2020). Report 9: Impact of non-pharmaceutical interventions (NPIs) to reduce COVID19 mortality and healthcare demand. Available at: https://www.imperial.ac.uk/mrc-global-infectious-disease-analysis/covid-19/report-9-impact-of-npis-on-covid-19/. [doi:10.12688/wellcomeopenres.15805.1]
FIORE, V. G., DeFelice, N., Glicksberg, B. S., Perl, O., Shuster, A., Kulkarni, K., O’Brien, M., Pisauro, M. A., Chung, D., & Gu, X. (2020). Containment of future waves of COVID-19: Simulating the impact of different policies and testing capacities for contact tracing, testing, and isolation. medRxiv preprint. Available at: https://www.medrxiv.org/content/10.1101/2020.06.05.20123372v1. [doi:10.1101/2020.06.05.20123372]
FIRTH, J. A., Hellewell, J., Klepac, P., Kissler, S., Kucharski, A. J., & Spurgin, L. G. (2020). Using a real-world network to model localized COVID-19 control strategies. Nature Medicine, 26(10), 1616–1622. [doi:10.1038/s41591-020-1036-8]
GARDNER, J. M., Willem, L., Van Der Wijngaart, W., Kamerlin, S. C. L., Brusselaers, N., & Kasson, P. (2020). Intervention strategies against COVID-19 and their estimated impact on swedish healthcare capacity. medRxiv preprint. Available at: https://www.medrxiv.org/content/10.1101/2020.04.11.20062133v1. [doi:10.1101/2020.04.11.20062133]
GASPAREK, M., Racko, M., & Dubovsky, M. (2020). A stochastic, individual-based model for the evaluation of the impact of non-pharmacological interventions on COVID-19 transmission in Slovakia. Available at: https://www.medrxiv.org/content/10.1101/2020.05.11.20096362v1. [doi:10.1101/2020.05.11.20096362]
GAUDOU, B., Huynh, N. Q., Philippon, D., Brugiére, A., Chapuis, K., Taillandier, P., Larmande, P., & Drogoul, A. (2020). COMOKIT: A modeling kit to understand, analyze, and compare the impacts of mitigation policies against the COVID-19 epidemic at the scale of a city. Frontiers in Public Health, 8, 563247. [doi:10.3389/fpubh.2020.563247]
GERMAN, R., Djanatliev, A., Maile, L., Bazan, P., & Hackstein, H. (2020). Modeling exit strategies from COVID-19 lockdown with a focus on antibody tests. medRxiv preprint. Available at: https://www.medrxiv.org/content/10.1101/2020.04.14.20063750v1. [doi:10.1101/2020.04.14.20063750]
GIACOPELLI, G. (2020). A full-scale agent-based model of Lombardy COVID-19 dynamics to explore social networks connectivity and vaccine impact on epidemic. medRxiv preprint. Available at: https://www.medrxiv.org/content/10.1101/2020.09.13.20193599v1. [doi:10.2196/preprints.24630]
GILBERT, N. (1999). Simulation: A new way of doing social science. American Behavioral Scientist, 42(10), 1485–1487. [doi:10.1177/0002764299042010002]
GOLDENBOGEN, B., Adler, S. O., Bodeit, O., Wodke, J. A. H., Korman, A., Bonn, L., Escalera, X. M. de la, Haffner, J. E. L., Krantz, M., Karnetzki, M., Maintz, I., Mallis, L., Torres, R. U. M., Prawitz, H., Segelitz, P., Seeger, M., Linding, R., & Klipp, E. (2020). Geospatial precision simulations of community confined human interactions during SARS-CoV-2 transmission reveals bimodal intervention outcomes. medRxiv preprint. Available at: https://www.medrxiv.org/content/10.1101/2020.05.03.20089235v2. [doi:10.1101/2020.05.03.20089235]
GOMEZ, J., Prieto, J., Leon, E., & Rodríguez, A. (2020). INFEKTA: A general agent-based model for transmission of infectious diseases: Studying the COVID-19 propagation in Bogotá - Colombia. medRxiv preprint. Available at: https://www.medrxiv.org/content/10.1101/2020.04.06.20056119v2. [doi:10.1101/2020.04.06.20056119]
GOPALAN, A., & Tyagi, H. (2020). How reliable are test numbers for revealing the COVID-19 ground truth and applying interventions? arXiv preprint. Available at: http://arxiv.org/abs/2004.12782. [doi:10.1007/s41745-020-00210-4]
GRAUER, J., Löwen, H., & Liebchen, B. (2020). Strategic spatiotemporal vaccine distribution increases the survival rate in an infectious disease like Covid-19. arXiv preprint. Available at: http://arxiv.org/abs/2005.04056. [doi:10.1038/s41598-020-78447-3]
GRESSMAN, P. T., & Peck, J. R. (2020). Simulating COVID-19 in a university environment. Mathematical Biosciences, 328, 108436. [doi:10.1016/j.mbs.2020.108436]
GRIMM, V., Railsback, S. F., Vincenot, C. E., Berger, U., Gallagher, C., DeAngelis, D. L., Edmonds, B., Ge, J., Giske, J., Groeneveld, J., Johnston, A. S. A., Milles, A., Nabe-Nielsen, J., Polhill, G. J., Radchuk, V., Rohwäder, M. S., Stillman, R. A., Thiele, J. C., & Ayllón, D. (2020). The ODD protocol for describing agent-based and other simulation models: A second update to improve clarity, replication, and structural realism. Journal of Artificial Societies and Social Simulation, 23(2), 7: https://www.jasss.org/23/2/7.html. [doi:10.18564/jasss.4259]
GUTIN, G., Hirano, T., Hwang, S. H., Neary, P. R., & Toda, A. A. (2020). The effect of social distancing on the reach of an epidemic in social networks. arXiv preprint. Available at: http://arxiv.org/abs/2005.03067.
HALE, T., Petherick, A., Phillips, T., & Webster, S. (2020). Variation in government responses to COVID-19. Blavatnik School of Government Working Paper. Available at: https://www.bsg.ox.ac.uk/research/publications/variation-government-responses-covid-19.
HEAD, J. R., Andrejko, K., Cheng, Q., Collender, P. A., Phillips, S., Boser, A., Heaney, A. K., Hoover, C. M., Wu, S. L., Northrup, G. R., Click, K., Harrison, R., Lewnard, J. A., & Remais, J. V. (2020). The effect of school closures and reopening strategies on COVID-19 infection dynamics in the San Francisco Bay Area: a cross-sectional survey and modeling analysis. medRxiv preprint. Available at: https://www.medrxiv.org/content/10.1101/2020.08.06.20169797v1. [doi:10.1101/2020.08.06.20169797]
HEATH, B., Hill, R., & Ciarallo, F. (2009). A survey of agent-based modeling practices (January 1998 to July 2008). Journal of Artificial Societies and Social Simulation, 12(4), 9: https://www.jasss.org/12/4/9.html.
HELLEWELL, J., Abbott, S., Gimma, A., Bosse, N. I., Jarvis, C. I., Russell, T. W., Munday, J. D., Kucharski, A. J., Edmunds, W. J., Sun, F., Flasche, S., Quilty, B. J., Davies, N., Liu, Y., Clifford, S., Klepac, P., Jit, M., Diamond, C., Gibbs, H., … Eggo, R. M. (2020). Feasibility of controlling COVID-19 outbreaks by isolation of cases and contacts. The Lancet Global Health, 8(4), 488–496. [doi:10.1016/s2214-109x(20)30074-7]
HERBRICH, R., Rastogi, R., & Vollgraf, R. (2020). CRISP: A probabilistic model for individual-Level COVID-19 infection risk estimation based on contact data. arXiv preprint. Available at: http://arxiv.org/abs/2006.04942.
HERNÁNDEZ-ORALLO, E., Manzoni, P., Calafate, C. T., & Cano, J. (2020). Evaluating how smartphone contact tracing technology can reduce the spread of infectious diseases: The case of COVID-19. IEEE Access, 8, 99083–99097. [doi:10.1109/access.2020.2998042]
HERRMANN, H. A., & Schwartz, J. M. (2020). Why COVID-19 models should incorporate the network of social interactions. Physical Biology, 17(6), 065008. [doi:10.1088/1478-3975/aba8ec]
HINCH, R., Probert, W. J. M., Nurtay, A., Kendall, M., Wymatt, C., Hall, M., Lythgoe, K., Cruz, A. B., Zhao, L., Stewart, A., Ferritti, L., Montero, D., Warren, J., Mather, N., Abueg, M., Wu, N., Finkelstein, A., Bonsall, D. G., Abeler-Dorner, L., & Fraser, C. (2020). OpenABM-Covid19 - an agent-based model for non-pharmaceutical interventions against COVID-19 including contact tracing. medRxiv preprint. Available at: https://www.medrxiv.org/content/10.1101/2020.09.16.20195925v1. [doi:10.1101/2020.09.16.20195925]
HOERTEL, N., Blachier, M., Blanco, C., Olfson, M., Massetti, M., Limosin, F., & Leleu, H. (2020). Facing the COVID-19 epidemic in NYC: A stochastic agent-based model of various intervention strategies. medRxiv preprint. Available at: http://medrxiv.org/lookup/doi/10.1101/2020.04.23.20076885. [doi:10.1101/2020.04.23.20076885]
HUANG, Q., Mondal, A., Jiang, X., Horn, M. A., Fan, F., Fu, P., Wang, X., Zhao, H., Ndeffo-Mbah, M., & Gurarie, D. (2020). SARS-CoV-2 transmission and control in a hospital setting: An individual-based modelling study. Royal Society Open Science, 8(3). [doi:10.1098/rsos.201895]
JACKSON, M. L. (2020). Low-impact social distancing interventions to mitigate local epidemics of SARS-CoV-2. Microbes and Infection, 22(10), 611–616. [doi:10.1016/j.micinf.2020.09.006]
JALAYER, M., Orsenigo, C., & Vercellis, C. (2020). CoV-ABM: A stochastic discrete-event agent-based framework to simulate spatiotemporal dynamics of COVID-19. arXiv preprint. Available at: http://arxiv.org/abs/2007.13231.
JENNESS, S. M., Willebrand, K. S., Malik, A. A., Lopman, B. A., & Omer, S. B. (2020). Dynamic network strategies for SARS-CoV-2 control on a cruise ship. medRxiv preprint. Available at: https://www.medrxiv.org/content/10.1101/2020.08.26.20182766v2. [doi:10.1101/2020.08.26.20182766]
KAI, D., Goldstein, G. P., Morgunov, A., Nangalia, V., & Rotkirch, A. (2020). Universal masking is urgent in the COVID-19 pandemic: SEIR and agent based models, empirical validation, policy recommendations. arXiv preprint. Available at: http://arxiv.org/abs/2004.13553.
KANO, T., Yasui, K., Mikami, T., Asally, M., & Ishiguro, A. (2020). An agent-based model for interrelation between COVID-19 outbreak and economic activities. arXiv preprint. Available at: http://arxiv.org/abs/2007.11988. [doi:10.1098/rspa.2020.0604]
KARATAYEV, V. A., Anand, M., & Bauch, C. T. (2020). Local lockdowns outperform global lockdown on the far side of the COVID-19 epidemic curve. Proceedings of the National Academy of Sciences, 117(39), 24575–24580. [doi:10.1073/pnas.2014385117]
KARIN, O., Bar-On, Y. M., Milo, T., Katzir, I., Mayo, A., Korem, Y., Dudovich, B., Yashiv, E., Zehavi, A. J., Davidovitch, N., Milo, R., & Alon, U. (2020). Cyclic exit strategies to suppress COVID-19 and allow economic activity. medRxiv preprint. Available at: https://www.medrxiv.org/content/10.1101/2020.04.04.20053579v4. [doi:10.1101/2020.04.04.20053579]
KARTHA, M., & Pathan, H. (2020). Simulation study on effect of lockdown and recovery time on spread of COVID-19 in high and low-Density areas. Social Science Research Network (SSRN). Available at: https://papers.ssrn.com/abstract=3572697. [doi:10.2139/ssrn.3572697]
KERMACK, W. O., & McKendrick, A. G. (1927). A contribution to the mathematical theory of epidemics. Proceedings of the Royal Society of London (Series A), 115(772), 700–721.
KERR, C. C., Stuart, R. M., Mistry, D., Abeysuriya, R. G., Hart, G., Rosenfeld, K., Selvaraj, P., Nunez, R. C., Hagedorn, B., George, L., Izzo, A., Palmer, A., Delport, D., Bennette, C., Wagner, B., Chang, S., Cohen, J. A., Panovska-Griffiths, J., Jastrzebski, M., … Klein, D. J. (2020). Covasim: An agent-based model of COVID-19 dynamics and interventions. medRxiv preprint. Available at: https://www.medrxiv.org/content/10.1101/2020.05.10.20097469v1. [doi:10.1101/2020.05.10.20097469]
KLÔH, V. P., Silva, G. D., Ferro, M., Araújo, E., Melo, C. B., Lima, J. R. P. de A., & Martins, E. R. (2020). The virus and socioeconomic inequality: An agent-based model to simulate and assess the impact of interventions to reduce the spread of COVID-19 in Rio de Janeiro, Brazil. Brazilian Journal of Health Review, 3(2), 3647–3673.
KOLUMBUS, Y., & Nisan, N. (2020). On the effectiveness of tracking and testing in SEIR models. arXiv preprint. Available at: http://arxiv.org/abs/2007.06291.
KOO, J. R., Cook, A. R., Park, M., Sun, Y., Sun, H., Lim, J. T., Tam, C., & Dickens, B. L. (2020). Interventions to mitigate early spread of SARS-CoV-2 in Singapore: A modelling study. The Lancet Infectious Diseases, 20(6), 678–688. [doi:10.1016/s1473-3099(20)30162-6]
KRETZSCHMAR, M. E., Rozhnova, G., Bootsma, M. C. J., Boven, M. van, Wijgert, J. H. H. M. van de, & Bonten, M. J. M. (2020). Impact of delays on effectiveness of contact tracing strategies for COVID-19: A modelling study. The Lancet Public Health, 5(8), 452–459. [doi:10.1101/2020.05.09.20096289]
KUCHARSKI, A. J., Klepac, P., Conlan, A. J. K., Kissler, S. M., Tang, M. L., Fry, H., Gog, J. R., Edmunds, W. J., Emery, J. C., Medley, G., Munday, J. D., Russell, T. W., Leclerc, Q. J., Diamond, C., Procter, S. R., Gimma, A., Sun, F. Y., Gibbs, H. P., Rosello, A., … Simons, D. (2020). Effectiveness of isolation, testing, contact tracing, and physical distancing on reducing transmission of SARS-CoV-2 in different settings: A mathematical modelling study. The Lancet Infectious Diseases, 20(10), 1151–1160. [doi:10.1101/2020.04.23.20077024]
KWON, O., Son, W. S., Kim, J. Y., & Kim, J. H. (2020). Intervention effects in the transmission of COVID-19 depending on the detection rate and extent of isolation. Epidemiology and Health, 42, e2020045. [doi:10.4178/epih.e2020045]
LENG, T., White, C., Hilton, J., Kucharski, A., Pellis, L., Stage, H., Davies, N. G., Keeling, M. J., Flasche, S., & Centre for Mathematical Modelling of Infectious Disease 2019 nCoV Working Group. (2020). The effectiveness of social bubbles as part of a Covid-19 lockdown exit strategy, a modelling study. Wellcome Open Research, 5, 213. [doi:10.12688/wellcomeopenres.16164.1]
LI, X., Cai, Y., Ding, Y., LI, J. da, Huang, G., Liang, Y., & Xu, L. (2020). Discrete simulation analysis of COVID-19 and prediction of isolation bed numbers. medRxiv preprint. Available at: https://www.medrxiv.org/content/10.1101/2020.07.13.20152330v1. [doi:10.1101/2020.07.13.20152330]
LIBERATI, A., Altman, D. G., Tetzlaff, J., Mulrow, C., Gøtzsche, P. C., Ioannidis, J. P. A., Clarke, M., Devereaux, P. J., Kleijnen, J., & Moher, D. (2009). The PRISMA statement for reporting systematic reviews and meta-analyses of studies that evaluate health care interventions: Explanation and elaboration. Journal of Clinical Epidemiology, 62(10), 1–34. [doi:10.1016/j.jclinepi.2009.06.006]
LORCH, L., Kremer, H., Trouleau, W., Tsirtsis, S., Szanto, A., Schölkopf, B., & Gomez-Rodriguez, M. (2020). Quantifying the effects of contact tracing, testing, and containment measures in the presence of infection hotspots. arXiv preprint. Available at: http://arxiv.org/abs/2004.07641.
MAHDIZADEH Gharakhanlou, N., & Hooshangi, N. (2020). Spatio-temporal simulation of the novel coronavirus (COVID-19) outbreak using the agent-based modeling approach (case study: Urmia, Iran). Informatics in Medicine Unlocked, 20, 100403. [doi:10.1016/j.imu.2020.100403]
MAHESHWARI, P., & Albert, R. (2020). Network model and analysis of the spread of Covid-19 with social distancing. arXiv preprint. Available at: http://arxiv.org/abs/2006.09189.
MAHMOOD, B. M., & Dabdawb, M. M. (2020). The pandemic COVID-19 infection spreading spatial aspects: A network-Based software approach. AL-Rafidain Journal of Computer Sciences and Mathematics, 14(1), 159–170. [doi:10.33899/csmj.2020.164684]
MAHMOOD, I., Arabnejad, H., Suleimenova, D., Sassoon, I., Marshan, A., Serrano-Rico, A., Louvieris, P., Anagnostou, A., Taylor, S. J. E., Bell, D., & Groen, D. (2020). FACS: A geospatial agent-based simulator for analysing COVID-19 spread and public health measures on local regions. Journal of Simulation, 1–19. [doi:10.1080/17477778.2020.1800422]
MANOUT, O., El-Megzari, I., & Ciari, F. (2020). Modeling the COVID-19 pandemic: A sensitivity analysis on input data using agent-based transportation simulation. Available at: https://trid.trb.org/view/1749284.
MANZO, G., & Rijt, A. van de. (2020). Halting SARS-CoV-2 by targeting high-Contact individuals. Journal of Artificial Societies and Social Simulation, 23(4), 10: https://www.jasss.org/23/4/10.html. [doi:10.18564/jasss.4435]
MARQUIONI, V. M., & de Aguiar, M. A. M. (2020). Quantifying the effects of quarantine using an IBM SEIR model on scalefree networks. Chaos, Solitons & Fractals, 138, 109999. [doi:10.1016/j.chaos.2020.109999]
MARTOS, D. M., Parcell, B., & Eftimie, R. (2020). Modelling the transmission of infectious diseases inside hospital bays: Implications for Covid-19. Mathematical Biosciences and Engineering, 17(6), 8084–8104. [doi:10.1101/2020.09.04.20188110]
MCCOMBS, A., & Kadelka, C. (2020). A model-based evaluation of the efficacy of COVID-19 social distancing, testing and hospital triage policies. PLoS Computational Biology, 16(10), e1008388. [doi:10.1371/journal.pcbi.1008388]
MICHAELS, J. A., & Stevenson, M. D. (2020). Explaining national differences in the mortality of Covid-19: Individual patient simulation model to investigate the effects of testing policy and other factors on apparent mortality. medRxiv preprint. Available at: https://www.medrxiv.org/content/10.1101/2020.04.02.20050633v2. [doi:10.1101/2020.04.02.20050633]
MILNE, G. J., & Xie, S. (2020). The effectiveness of social distancing in mitigating COVID-19 spread: A modelling analysis. medRxiv preprint. Available at: https://www.medrxiv.org/content/10.1101/2020.03.20.20040055v1. [doi:10.1101/2020.03.20.20040055]
MOHSEN, A., & Alarabi, A. (2020). The impact of community containment implementation timing on the spread of COVID-19: A simulation study. F1000Research, 9, 452. [doi:10.12688/f1000research.24156.1]
MÜLLER, S. A., Balmer, M., Neumann, A., & Nagel, K. (2020). Mobility traces and spreading of COVID-19. medRxiv preprint. Available at: https://www.medrxiv.org/content/10.1101/2020.03.27.20045302v1.
NADINI, M., Zino, L., Rizzo, A., & Porfiri, M. (2020). A multi-agent model to study epidemic spreading and vaccination strategies in an urban-like environment. Applied Network Science, 5(1), 1–30. [doi:10.1007/s41109-020-00299-7]
NANDE, A., Adlam, B., Sheen, J., Levy, M. Z., & Hill, A. L. (2020). Dynamics of COVID-19 under social distancing measures are driven by transmission network structure. medRxiv preprint. Available at: https://www.medrxiv.org/content/10.1101/2020.06.04.20121673v1. [doi:10.1101/2020.06.04.20121673]
NARASSIMA, M. S., Jammy, G. R., Pant, R., Choudhury, L., Aadharsh, R., Yeldandi, V., Anbuudayasankar, S. P., Rangasami, P., & John, D. (2020). An agent based model for assessing spread and health systems burden for COVID-19 in Rangareddy district, Telangana state, India. medRxiv preprint. Available at: https://www.medrxiv.org/content/10.1101/2020.06.04.20121848v4. [doi:10.1101/2020.06.04.20121848]
NEILAN, A. M., Losina, E., Bangs, A. C., Flanagan, C., Panella, C., Eskibozkurt, G. E., Mohareb, A., Hyle, E. P., Scott, J. A., Weinstein, M. C., Siedner, M. J., Reddy, K. P., Harling, G., Freedberg, K. A., Shebl, F. M., Kazemian, P., & Ciaranello, A. L. (2020). Clinical impact, costs, and cost-Effectiveness of expanded SARS-CoV-2 testing in Massachusetts. Clinical Infectious Diseases, ciaa1418. [doi:10.1101/2020.07.23.20160820]
NG, T. C. V., Cheng, H. Y., Chang, H. H., Liu, C. C., Yang, C. C., Jian, S. W., Liu, D. P., Cohen, T., & Lin, H. H. (2020). Effects of case- and population-based COVID-19 interventions in Taiwan. medRxiv preprint. Available at: https://www.medrxiv.org/content/10.1101/2020.08.17.20176255v1. [doi:10.1101/2020.08.17.20176255]
NG, V., Fazil, A., Waddell, L. A., Bancej, C., Turgeon, P., Otten, A., Atchessi, N., & Ogden, N. H. (2020). Projected effects of nonpharmaceutical public health interventions to prevent resurgence of SARS-CoV-2 transmission in Canada. CMAJ, 192(37), 1053–1064. [doi:10.1503/cmaj.200990]
NGUYEN, T. T., NGUYEN, Q. V. H., NGUYEN, D. T., Hsu, E. B., Yang, S., & Eklund, P. (2020). Artificial intelligence in the battle against coronavirus (COVID-19): A survey and future research directions. arXiv preprint. Available at: https://arxiv.org/abs/2008.07343. [doi:10.36227/techrxiv.12743933.v1]
NICOLA, M., Alsafi, Z., Sohrabi, C., Kerwan, A., Al-Jabir, A., Iosifidis, C., Agha, M., & Agha, R. (2020). The socio-economic implications of the coronavirus pandemic (COVID-19): A review. International Journal of Surgery, 78, 185. [doi:10.1016/j.ijsu.2020.04.018]
NÚÑEZ-CORRALES, S., & Jakobsson, E. (2020). The epidemiology workbench: A tool for communities to strategize in response to COVID-19 and other infectious diseases. medRxiv preprint. Available at: https://www.medrxiv.org/content/10.1101/2020.07.22.20159798v2.
PEAK, C. M., Kahn, R., Grad, Y. H., Childs, L. M., Li, R., Lipsitch, M., & Buckee, C. O. (2020). Individual quarantine versus active monitoring of contacts for the mitigation of COVID-19: A modelling study. The Lancet Infectious Diseases, 20(9), 1025–1033. [doi:10.1016/s1473-3099(20)30361-3]
PESCARMONA, G., Terna, P., Acquadro, A., PESCARMONA, P., Russo, G., & Terna, S. (2020). The contagion sequences of the epidemic S.I.s.a.R. model: A source of suggestions for intervention policies. Report available at: https://terna.to.it/simul/contagionSequences.pdf.
PHILLIPS, B., Browne, D., Anand, M., & Bauch, C. (2020). Model-based projections for COVID-19 outbreak size and student-days lost to closure in Ontario childcare centres and primary schools. medRxiv preprint. Available at: https://www.medrxiv.org/content/10.1101/2020.08.07.20170407v2. [doi:10.1101/2020.08.07.20170407]
POLLMANN, T. R., POLLMANN, J., Wiesinger, C., Haack, C., Shtembari, L., Turcati, A., Neumair, B., Meighen-Berger, S., Zattera, G., Neumair, M., Apel, U., Okolie, A., Mueller, J., Schoenert, S., & Resconi, E. (2020). The impact of digital contact tracing on the SARS-CoV-2 pandemic - A comprehensive modelling study. medRxiv preprint. Available at: https://www.medrxiv.org/content/10.1101/2020.09.13.20192682v1. [doi:10.1101/2020.09.13.20192682]
PULLANO, G., Valdano, E., Scarpa, N., Rubrichi, S., & Colizza, V. (2020). Evaluating the effect of demographic factors, socioeconomic factors, and risk aversion on mobility during the COVID-19 epidemic in France under lockdown: A population-based study. The Lancet Digital Health, 2(12), 638–649. [doi:10.1101/2020.05.29.20097097]
RAJABI, A., Mantzaris, A. V., Mutlu, E. C., & Garibay, I. (2020). Investigating dynamics of COVID-19 spread and containment with agent-based modeling. medRxiv preprint. Available at: https://www.medrxiv.org/content/10.1101/2020.08.18.20177451v1. [doi:10.1101/2020.08.18.20177451]
RECHTIN, M., Feldman, V., Klare, S., Riddle, N., & Sharma, R. (2020). Modeling and simulation of COVID-19 pandemic for Cincinnati Tri-State area. arXiv preprint. Available at: http://arxiv.org/abs/2006.06021.
REICH, O., Shalev, G., & Kalvari, T. (2020). Modeling COVID-19 on a network: Super-spreaders, testing and containment. medRxiv preprint. Available at: https://www.medrxiv.org/content/10.1101/2020.04.30.20081828v1. [doi:10.1101/2020.04.30.20081828]
ROCKETT, R. J., Arnott, A., Lam, C., Sadsad, R., Timms, V., Gray, K. A., Eden, J. S., Chang, S., Gall, M., Draper, J., Sim, E. M., Bachmann, N. L., Carter, I., Basile, K., Byun, R., O’Sullivan, M. V., Chen, S. C. A., Maddocks, S., Sorrell, T. C., … Sintchenko, V. (2020). Revealing COVID-19 transmission in Australia by SARS-CoV-2 genome sequencing and agent-based modeling. Nature Medicine, 26(9), 1398–1404. [doi:10.1101/2020.04.19.048751]
ROSENSTROM, E., Aglar, B. O., Ivy, J. S., Keskinocak, P., Mayorga, M., & Swann, J. L. (2020). High-Quality masks can reduce infections and deaths in the US. medRxiv preprint. Available at: https://www.medrxiv.org/content/10.1101/2020.09.27.20199737v1. [doi:10.1101/2020.09.27.20199737]
RUSSELL, S., & Norvig, P. (2016). Artificial Intelligence: A Modern Approach. Boston, MA: Addison Wesley.
SARGENT, R. G. (2015). 'Model verification and validation.' In M. L. Loper (Ed.), Modeling and Simulation in the Systems Engineering Life Cycle (pp. 57–65). Berlin Heidelberg: Springer.
SHINDE, G. R., Kalamkar, A. B., Mahalle, P. N., Dey, N., Chaki, J., & Hassanien, A. E. (2020). Forecasting models for coronavirus disease (COVID-19): A survey of the state-of-the-art. SN Computer Science, 1(4), 1–15. [doi:10.36227/techrxiv.12101547]
SILVA, P. C. L., Batista, P. V. C., Lima, H. S., Alves, M. A., Guimarães, F. G., & SILVA, R. C. P. (2020). COVID-ABS: An agent-based model of COVID-19 epidemic to simulate health and economic effects of social distancing interventions. Chaos, Solitons & Fractals, 139, 110088. [doi:10.1016/j.chaos.2020.110088]
SITUNGKIR, H. (2020). The pandemics in artificial society: Agent-Based model to reflect strategies on COVID-19. medRxiv preprint. Available at: https://www.medrxiv.org/content/10.1101/2020.07.27.20162511v1. [doi:10.1101/2020.07.27.20162511]
SMALL, M., & Cavanagh, D. (2020). Modelling strong control measures for epidemic propagation with networks - A COVID-19 case study. arXiv preprint. Available at: http://arxiv.org/abs/2004.10396. [doi:10.1109/access.2020.3001298]
SON, W. S., & RISEWIDs Team. (2020). Individual-based simulation model for COVID-19 transmission in Daegu,Korea. Epidemiology and Health, e2020042. [doi:10.4178/epih.e2020042]
SQUAZZONI, F., Polhill, J. G., Edmonds, B., Ahrweiler, P., Antosz, P., Scholz, G., Chappin, É., Borit, M., Verhagen, H., Giardini, F., & Gilbert, N. (2020). Computational models that matter during a global pandemic outbreak: A call to action. Journal of Artificial Societies and Social Simulation, 23(2), 10: https://www.jasss.org/23/2/10.html. [doi:10.18564/jasss.4298]
THOMPSON, J., McClure, R., Blakely, T., Wilson, N., Baker, M., Wijnands, J. S., Sa, T. H. de, Nice, K., Cruz-Gambardella, C., Aschwanden, G., & Stevenson, M. (2020). Modelling the estimated likelihood of eliminating the SARS-CoV-2 pandemic in Australia and New Zealand under public health policy settings: An agent-based-SEIR approach. SSRN Scholarly Paper. Available at: https://papers.ssrn.com/abstract=3588074. [doi:10.2139/ssrn.3588074]
TOPIRCEANU, A., Udrescu, M., & Marculescu, R. (2020). Centralized and decentralized isolation strategies and their impact on the COVID-19 pandemic dynamics. arXiv preprint. Available at: http://arxiv.org/abs/2004.04222.
TUOMISTO, J. T., Yrjölä, J., Kolehmainen, M., Bonsdorff, J., Pekkanen, J., & Tikkanen, T. (2020). An agent-based epidemic model REINA for COVID-19 to identify destructive policies. medRxiv preprint. Available at: https://www.medrxiv.org/content/10.1101/2020.04.09.20047498v2. [doi:10.1101/2020.04.09.20047498]
WAGNER, M., Kombe, I. K., Kiti, M. C., Aziza, R., Barasa, E., & Nokes, D. J. (2020). Using contact data to model the impact of contact tracing and physical distancing to control the SARS-CoV-2 outbreak in Kenya. Wellcome Open Research, 5, 212. [doi:10.12688/wellcomeopenres.16264.1]
WALLENTIN, G., Kaziyeva, D., & Reibersdorfer-Adelsberger, E. (2020). COVID-19 intervention scenarios for a long-term disease management. International Journal of Health Policy and Management, 9(12), 508–516. [doi:10.34172/ijhpm.2020.130]
WANG, Q., Shi, N., Huang, J., Cui, T., Yang, L., Ai, J., Ji, H., Xu, K., Ahmad, T., Bao, C., & Jin, H. (2020). Effectiveness and cost-effectiveness of public health measures to control COVID-19: A modelling study. medRxiv preprint. Available at: https://www.medrxiv.org/content/10.1101/2020.03.20.20039644v2. [doi:10.1101/2020.03.20.20039644]
WELLS, K., & Lurgi, M. (2020). COVID-19 containment policies through time may cost more lives at metapopulation level. medRxiv preprint. Available at: https://www.medrxiv.org/content/10.1101/2020.04.22.20075093v1. [doi:10.1101/2020.04.22.20075093]
WELLS, K., Lurgi, M., Collins, B., Lucini, B., Kao, R. R., Lloyd, A. L., Frost, S. D. W., & Gravenor, M. B. (2020). COVID-19 control across urban-rural gradients. medRxiv preprint. Available at: https://www.medrxiv.org/content/10.1101/2020.09.07.20189597v2. [doi:10.1101/2020.09.07.20189597]
WILDER, B., Charpignon, M., Killian, J. A., Ou, H. C., Mate, A., Jabbari, S., Perrault, A., Desai, A. N., Tambe, M., & Majumder, M. S. (2020). Modeling between-population variation in COVID-19 dynamics in Hubei, Lombardy, and New York City. Proceedings of the National Academy of Sciences, 117(41), 25904–25910. [doi:10.1073/pnas.2010651117]
WILLEM, L., Abrams, S., Libin, P. J. K., Coletti, P., Kuylen, E., Petrof, O., Møgelmose, S., Wambua, J., Herzog, S. A., Faes, C., Beutels, P., & Hens, N. (2020). The impact of contact tracing and household bubbles on deconfinement strategies for COVID-19: An individual-based modelling study. medRxiv preprint. Available at: https://www.medrxiv.org/content/10.1101/2020.07.01.20144444v4. [doi:10.1101/2020.07.01.20144444]
WOHLIN, C. (2014). Guidelines for snowballing in systematic literature studies and a replication in software engineering. Proceedings of the 18th International Conference on Evaluation and Assessment in Software Engineering. [doi:10.1145/2601248.2601268]
XIAO, Y., Yang, M., Zhu, Z., Yang, H., Zhang, L., & Ghader, S. (2020). Modeling indoor-level non-pharmaceutical interventions during the COVID-19 pandemic: A pedestrian dynamics-based microscopic simulation approach. arXiv preprint. Available at: http://arxiv.org/abs/2006.10666.
XUE, L., Jing, S., Miller, J. C., Sun, W., Li, H., Estrada-Franco, J. G., Hyman, J. M., & Zhu, H. (2020). A data-driven network model for the emerging COVID-19 epidemics in Wuhan, Toronto and Italy. Mathematical Biosciences, 326, 108391. [doi:10.1016/j.mbs.2020.108391]
YANG, S., Senapati, P., Wang, D., Bauch, C. T., & Fountoulakis, K. (2020). Targeted pandemic containment through identifying local contact network bottlenecks. arXiv preprint. Available at: http://arxiv.org/abs/2006.06939.
ZHANG, N., Cheng, P., Jia, W., Dung, C. H., Liu, L., Chen, W., Lei, H., Kan, C., Han, X., Su, B., Xiao, S., Qian, H., Lin, B., & Li, Y. (2020). Impact of intervention methods on COVID-19 transmission in Shenzhen. Building and Environment, 180, 107106. [doi:10.1016/j.buildenv.2020.107106]