How Availability Heuristic, Confirmation Bias and Fear May Drive Societal Polarisation: An Opinion Dynamics Simulation of the Case of COVID-19 Vaccination

and
aHuazhong Agricultural University, China; bUniversity of Groningen, Netherlands; cGroningen Center for Social Complexity Studies, University of Groningen, Netherlands
Journal of Artificial
Societies and Social Simulation 26 (4) 2
<https://www.jasss.org/26/4/2.html>
DOI: 10.18564/jasss.5135
Received: 09-Dec-2022 Accepted: 11-May-2023 Published: 31-Oct-2023
Abstract
The stark contrast between different opinions on COVID-19 vaccination suggests that the underlying opinion dynamics is a topic worth studying. Here we build a comprehensive agent-based model by incorporating fear into the HUMAT framework to study how the availability heuristic and confirmation bias influence information processing and impact the opinion dynamics and vaccination rate in the case of the COVID-19 pandemic. Three scenarios are designed to explore the impact of the disease severity, confirmation bias, and authorities on COVID-vaccination opinion polarisation and the vaccination rate. Main simulation results indicate that: 1) Increased fear of disease raises the vaccination rate and accelerates people’s uptake of COVID vaccines. 2) Confirmation bias fuels opinion polarisation through the mechanism of information selection and improves the satisfaction of different groups. 3) Opposing authorities and availability heuristic promote opinion polarisation, while more moderate authorities reduce polarisation but do not make it disappear. Implications of these results are discussed for science communication related to complex societal problems.Introduction
Many difficult societal problems give rise to fears in people with respect to their own health and well-being and that of their family or even the future of mankind and life on Earth in general. The loss of biodiversity, climate change, nuclear power, migration and most lately the COVID-19 pandemic, are all examples of such societal problems that are heavily debated in the media and on which also radical opposing opinions have been being ventilated.
Without underestimating the seriousness and impact of these problems, it may well be that biases in perception may give rise to fear and translate into very strong opinions, which contribute to polarisation in society, making meaningful dialogue difficult. Such processes of polarisation may even jeopardise democratic institutions, when large groups of people are not on speaking terms anymore.
In this paper, we will specifically focus on the opinion dynamics related to the COVID-19 outbreak, where strong polarisation can be observed. For example, Bernacer et al. (2021) observed the polarisation of beliefs based on political ideology as a consequence of the COVID-19 pandemic. Much of the polarisation addresses the importance of supporting the mass vaccination program of the state to combat the spread of a feared disease, versus the fear of side effects of an experimental vaccine that is forcefully administered by an untrustworthy state. In this opinionated situation, the role of social media is undoubtedly critical (Stjernswärd & Glasdam 2021). The perception of disease and health risks is influenced by the media (Clarke 2011; Lupton 2013), and especially on social media people share, evaluate and even attack information provided by authorities, which is facilitated by different perspectives amongst the authorities themselves.
The media played a critical role in the sharing of information on COVID-19. Not only do regular news media succeed in getting readers and viewers by sharing “shocking news” (as an example, in the Netherlands a large article with picture was published in a main newspaper on the fact that a former “strong man”-competitor was not vaccinated and died from COVID-19 – Quekel 2021), but the algorithms operating on social media increasingly share information that AI algorithms have identified as raising your emotions (e.g., McStay 2018). This implies that information on shocking cases is functioning as click-baits in the media and can give a distorted image of the actual situation (availability heuristic).
Wide attention in the media for infectious diseases leads to an overestimation of risks (Young et al. 2013) and it seems clear that the enormous (social) media attention on COVID-19 has had an impact on risk perceptions. For example, in the United States, a study showed that the risk of death in a COVID case was overestimated, as the general public estimated that people under the age of 55 would account for 43% of deaths, while actually it was only 7% (Ladapo et al. 2022). The availability heuristic (Tversky & Kahneman 1974) is a psychological mechanism that explains how the reporting of incidents can result in an overestimation of the chances of such incidents.
Very interestingly, the analysis of COVID-19 risk perception by Macdonald (2021) displays a bimodal distribution, indicating that most people either have a low or high-risk perception of COVID-19 vaccination. This indicates that a polarised risk perception has emerged. A survey conducted by Gallup (Gallup 2022) identifies an overestimation of the perceived risks of COVID-19, with 92% overstating the risk of hospitalisation for the unvaccinated, and 62% overstating the risk for the vaccinated. In the context of polarised perceptions, this study also identified different risk estimations between republicans (low risk) and democrats (high risk), which is in line with Bernacer et al. (2021) concerning the observed polarisation of COVID-19 beliefs based on political ideology.
In the context of a population-wide vaccination program, we must realise that the perception of the risks can be influenced by being vaccinated. The confirmation bias (Wason 1960) explains that people are biased towards information that confirms their beliefs or behaviours, which comes in a variety of guises (Nickerson 1998). In particular, with vaccination, this bias can be strong because one cannot un-vaccinate oneself, so it is likely that information stressing the seriousness of the disease is accepted by vaccinated people, whereas information on limited risks and side effects of vaccinations is neglected. In the context of mass vaccination, this may cause polarisation between a large majority that has been/is in favour of vaccination versus a smaller group that is hesitant about vaccination and has fears of possible side effects (e.g., myocarditis and heart-failure).
Both fears of the disease and fear of side effects may result in a tendency to listen more to authorities (experts, politicians and opinion leaders having a large exposure), who are communicating through (social) media. As reported by Bigo et al. (2021), government messages may play on people’s existential fear of death to make them obey coercive measures. This fits with the idea of Agamben (1998) of ‘homo sacer’, where regimes lead people like a herd by politics of fear. In the context of contagious disease, research on pandemic influenza recommended interventions which highlight the risk posed by pandemic influenza while simultaneously advocating vaccination to ameliorate this risk (Bish et al. 2011). With the outbreak of COVID-19, these insights were used in communication campaigns that were designed to create fear in the population. Members of the Scientific Pandemic Influenza Group on Behaviour (SPI-B) later expressed their regret about using such unethical methods used to control behaviour and considered these tactics to be totalitarian (The Telegraph 2021; Trimble 2021).
However, on social media, it is also easy to find alternative authorities with different perspectives on COVID-19 and vaccination, emphasising for example that the disease was not serious at all, and that the mRNA injections imposed a serious health risk. Obviously, social media also offer a plethora of conspiracy theories, for example creating fear of a genocide aimed at decimating the human population on Earth (e.g., COVID-19 Adverse Reactions Group on Facebook, or news on the conviction for genocide of the Canadian Prime Minister Trudeau, Britain’s Queen Elizabeth, and Pope Francis over their support for COVID-19 vaccination). Because people like confirming their existing fears and the associated best policies to reduce the observed risks, there is always a possibility to find an authority that supports your position. Hence a person with a high fear of COVID-19 may appreciate an authority that stresses a very stringent vaccination and social distancing policy, whereas a person with a high fear of side effects of vaccinations may appreciate an authority that warns of side effects and a mass vaccination strategy. This authority-following tendency in times of fear can further contribute to the polarisation of opinions.
In this study, we would like to explore to what extent the availability heuristic, the confirmation bias, and the role of authorities are on the polarisation of opinions on COVID-19. We hypothesise the effects of these factors contribute to a strengthening of polarisation processes. The results may be important to reflect on the communication of scientific information on risks in societal problems to the wider population.
Conceptual Framework
HUMAT description
Agent-based modelling is gaining momentum in the social sciences as the necessary method for studying social dynamics in many contexts. Whereas the early social simulation models were built using oversimplifications concerning behavioural assumptions, increasingly social scientific and psychological theories are being used for the implementation of more plausible agent rules (Jager 2021).
Often, many different theoretical perspectives are important in understanding the social dynamics of empirical cases. For example, people have different needs, interests and perspectives, are more or less involved in topics, may be more or less susceptible to norms and receptive to information from other people, and their actions may be more or less publicly visible. This leaves the social simulator with a double challenge. First, formalising verbal theories from the social sciences into computational agent rules confronts the modeller with difficult decisions on the implementation of the causal mechanisms and the appropriate level of detail (see e.g., Schlüter et al. 2017). Second, drivers and processes described in specific theories often have to be combined in studying the socially complex dynamics of the phenomenon of interest.
For example, in the case of vaccination to protect against COVID-19, different drivers and processes play a role, such as the fear of the disease and possible side effects of vaccination, personal values on vaccination, information from different sources, normative influence from friends and family, trust in the government and other sources of information. Different theories are relevant in developing a model capturing the social dynamics of opinions on vaccination and the decision to get vaccinated or not. Whilst these two challenges of formalisation and integration are not a focus in the regular social sciences, within the social simulation community there is an increased demand for frameworks that formalise and integrate different mechanisms and processes to support the development of social simulation models for various cases.
Within the context of the EU SMARTEES project, the HUMAT framework has been developed as a generic platform that formalises and integrates different behavioural drivers and processes to support model development and data collection for model parameterisation (Antosz et al. 2019). This HUMAT platform has now been used to simulate a variety of empirical cases of social innovation, such as a referendum on closing a park for car traffic, joining heat network projects, establishing local city blocks banning transit traffic and islands transitioning towards sustainable energy.
The HUMAT socio-cognitive architecture (Antosz et al. 2019) constitutes artificial populations in which agents have dynamic beliefs about how satisfying behavioural alternatives are for their needs and values and have social networks to communicate with one another about these beliefs. The HUMAT framework represents social influence in the context of the (dis)satisfaction of different needs and values as motives for action. In this case, an agent may, for example, fear the side effects of vaccination but experience normative pressure to get vaccinated because the majority of their friends have been vaccinated. This results in an internal conflict, or cognitive dissonance (Festinger 1954; Harmon-Jones & Harmon-Jones 2018). Multiple needs can be grouped into three basic categories of (1) experiential needs related to short-term outcomes, (2) social needs related to fitting in the group, and (3) values. Needs and values vary with respect to their importance to the individual. Moreover, alternative choices differently satisfy those needs and values. For example, the agent in the example before may experience more social satisfaction by complying with the group by getting vaccinated, but this may go at the cost of fear of side effects.
As a consequence, the agent will experience cognitive dissonance in making this choice. From the perspective of that agent, the preferred alternative is internally inconsistent (has pros and cons), and the dissonant state will inhibit action and motivate the agent to reduce the inconsistency: try to convince other agents in its ego-network to change their minds. We introduce an important extension to the social theory of normative influence: the idea of a perceived local norm. This perceived local norm is the belief about what is the most followed behaviour in one’s ego-network. It does not represent the global norm, which may differ. For example, a local perceived norm may be that most people are not vaccinated, whilst the global majority are. HUMAT also emphasises individual differences in sensitivity to social normative influence – agents vary with respect to the importance of social need.
Depending on satisfaction of the social need, information exchange can take two forms: signalling and inquiring. If the slightly preferred option is not popular enough among alters, the ego signals to his/her most gullible alter with an opposite preference and tries to convince/inquire them to change their mind. For example, an agent may try to convince others not to get vaccinated if it prefers to be unvaccinated itself. If the strategy of signalling is not suitable to decrease the dissonance experienced by an agent, the agent chooses to inquire about the slightly preferred option, in this case not taking the vaccination. When inquiring, agents can ask the most persuasive alter in their social network for advice. Persuasiveness depends on the perception of the communicating agent as being trustworthy as an information source and is based on the similarity between the communicating agents. Decisions on whether to adopt a certain behaviour result from a cumulative satisfaction/dissatisfaction of needs/motives weighed by the importance of each of those needs/motives.
HUMAT offers a basic integrated framework and offers possibilities for extensions with e.g., emotional drivers such as hope and fear, and heuristics in informational processing, such as availability heuristic or confirmation bias, depending on the specific social dynamics being targeted. For an extensive description of the precise formalisations of the generic HUMAT framework, we refer to Antosz et al. (2019).
Implementing fear, availability heuristic, and confirmation bias
HUMAT as a basic framework is flexible to incorporate additional components to describe the dynamic opinion formation process. In the context of societal problems such as the COVID-19 situation, emotions like fear and the role of authorities could be incorporated as relevant additions to the basic HUMAT architecture. Epstein (2014) notes in Agent_Zero that fear as a neurocognitive concept consists of three parts – affective, cognitive, and social components, which in combination define the agent’s behavioural disposition. In light of this decomposition, an agent’s opinion depends on its perceived fear as well as its social links with others, such as friends and authorities in our model. In the context of COVID-19, the fear of the virus and the fear of vaccines can not only affect the psychological satisfaction of individuals, which translates into the experiential need in terms of the HUMAT framework but trigger social processes and thus affect individuals’ social needs as well. On the one hand, if one is more fearful of the vaccine than of the disease, then getting vaccinated will detriment its experiential need. On the other hand, if most of one’s friends are fearful of COVID-19 vaccines, it is more socially satisfactory to conform to the majority. As Epstein et al. (2008), Epstein et al. (2021) mentioned, the spread of fear can be independent of the spread of disease. They even generated a pure fear epidemic with no underlying disease in the model, which emphasises the characteristics of fear spreading among the population. To simplify the model, we focused on investigating the role of pure fear of disease and vaccination in the absence of actual disease transmission. Assuming a higher perceived disease severity leading to a higher fear of disease and a lower fear of vaccination, we propose the first hypothesis as follows.
H1. Higher perceived disease severity leads to a higher vaccination rate.
Similarly, heuristics and biases of processing complex information should be added to the basic HUMAT model, which is considered in our conceptual framework to make components more empirically grounded. As a sharing platform, social media changes the proportion of scientific information relative to conspiracy narratives and facilitates heuristics and biases in vaccination risk estimation (Luz et al. 2020; Meppelink et al. 2019), which also makes it easy to get information from authorities such as experts or pseudo-experts that function as opinion leaders.
We posit that confirmation bias as a general human cognitive disposition plays an active role in people’s COVID-19 vaccination uptake. This confirmation bias implies that when people have taken a vaccination, they show a tendency to reject information on the negative impacts of vaccination. Whereas this bias can also work in the other direction, when non-vaccinated people reject information on the benefits of the vaccination, there is a fundamental skewness, as non-vaccinated people always have the choice to get vaccinated, but vaccinated people have no option to get un-vaccinated. As such, the confirmation bias is likely to be stronger in vaccinated people, as they have no choice. The confirmation bias can be very strong, as people biased in this way can hold a wrong, non-truth-convergent opinion (Kahan 2015) and can show a tendency to actively reject even objectively correct information (Sobkowicz 2018). The HUMAT model is expanded when inducing a confirmation bias mechanism, that is, individuals tend to selectively collect or refine more information that really confirms their decision. In our model, the confirmation bias mechanism implies that vaccinated people are more likely to accept information that justifies their choice and reject information about possible negative side effects of vaccination, which tends to reinforce the opinion polarisation between the vaccinated and unvaccinated groups. The second hypothesis, therefore, focuses on the effect of confirmation bias on the opinion polarisation.
H2. Confirmation bias promotes opinion polarisation.
Authorities function as opinion leaders and play a critical role in shaping social attitudes (Katz 1957; Van Eck et al. 2011), which has not yet been thoroughly studied in the field of epidemics and vaccination. Authorities are often well connected to mass-media such as informational programs on television and newspapers, and specific informational channels such as YouTube, and can have a large base of followers on social media such as Twitter, Weibo, Facebook, and Telegram. Being authorities in the social network, authorities have a larger number of connections than ordinary people, and as a result, are much more heard and can exert a much greater influence upon others broadly. The often emotional tone of narratives from authorities is often louder online as compared with more objective statistical data (Blumenthal-Barby & Krieger 2015). In most cases, individuals do not need to work out opinions for themselves because their favourite authorities’ opinions are readily available. This helps when an individual is confronted with oftentimes incongruent information from many sources which requires some action, e.g., taking a vaccination (Rabin & Schrag 1999). However, the interactions between ordinary individuals (imagine offline meeting with one’s friends or family members) as a source of social influence are also very relevant and discussions on how to respond to the COVID-19 pandemic took place in families, at work, choirs, sports clubs, places of worship and many other places where groups of people gathered. We introduce the bias of availability heuristic, by which people give more weight to information from authorities, especially the extreme authorities they are following in comparison to their private connections such as friends or family members, to capture the extent to which individuals are impacted by authorities versus their local interactions. We put out the following hypotheses to reflect the connection between the authority figure’s role and the polarisation of opinions.
H3a. More moderate authorities lead to less polarisation.
H3b. The availability heuristic promotes polarisation.
We develop a conceptual framework that formalises the cognitive elements based on the HUMAT framework into an agent-based model related to vaccination opinion formation and dynamics. Figure 1 summarises the psychological components and behavioural processes at the individual level.
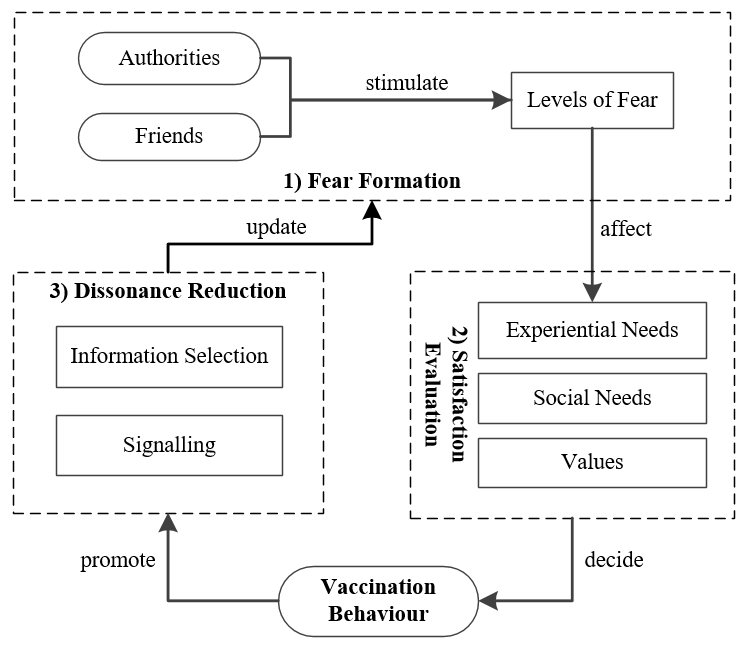
Contributions
Overall, we will build an integrated conceptual model that allows for simulating how psychological mechanisms impact the opinion dynamics related to vaccination. The impact will be studied for the mechanisms separately and in combination to understand the interaction between mechanisms. This model may contribute in several aspects to our understanding of opinion dynamics in this domain. The key novelty of this work resides first in the architecture of the model that we present. Our study uses the new HUMAT framework, which has been developed as an integrative framework connecting different behavioural theories in a formal model. We show how additional processes, that we identified as relevant in the case of opinion dynamics related to vaccination, can be implemented within this framework as “add-on modules”. Second, while most existing research on COVID-19 focuses on the spread of the disease, little work has been done to investigate the social dynamics related to the decision-making process of getting vaccinated or not. This model bridges the gap by looking into the cognitive and social processes behind vaccination choice, as well as the mechanism of how disease severity and fears will impact these processes and the resulting vaccination degree. Third, the results obtained with this model relate the process of polarisation to the effect of fear and the functioning of biases. This is in our view a new and relevant finding, as it also relates to polarisations happening in other domains where people can be afraid for future developments. The consequences of these findings are relevant for the communication of scientific findings to society, and demonstrate that fear-mongering, as discussed in the paper, may have destabilising effects on society. Lastly, by applying the HUMAT framework to a concrete case of COVID vaccination, we present an integrated dual-decision model that can be extended to other contexts.
The Model
The model description follows the ODD (Overview, Design concepts, Details) protocol for describing individual- and agent-based models (Grimm et al. 2006), as updated by Grimm et al. (2020). The protocol helps to make model descriptions complete and accurate, as well as standardise descriptions for the benefit of users and reviewers. The model itself was implemented with NetLogo 6.0.4 (Wilensky 1999). A complete, detailed model description following the ODD protocol is provided in Appendix 1.
The overall purpose of the model is to clarify the opinion dynamics of COVID-19 vaccination. Specifically, we want to answer whether and how individual fears, biased information processing, and the role of authorities contribute to opinion polarisation and vaccination rate.
Main elements
The main elements of the model are as follows: There are \(N\) susceptible individuals in a random network. The average degree of networks, \(d\), determines the number of friends who will influence an individual’s decision-making process. A larger \(d\) indicates a denser network. There are \(N_{a}\) authorities, who transmits information as hubs in the model. Each authority is fully linked to all other individuals, and therefore, it has the potential to influence the fear value of every individual. Each individual has two types of fear, the fear of side effects of vaccination, \(F_{v} \in [0,1]\), and the fear of disease, \(F_{d} \in [0,1]\). Individuals update their fears by interacting with friends and authorities with whom they are connected. Interactions between individuals and authorities are based on the principle of similarity and are implemented through social networks.
The network among individuals is structured following the spatial random graph algorithm (Wong et al. 2006). The core idea is that an individual is more likely to form links with geographically nearby individuals than with those who are farther away. At first, individuals disperse randomly in the world and then create numbers of undirected links with close others based on the level of \(d\), which takes the value 5 in our model. Figure 2 depicts the basic social network structure. The total number of connections is determined by \(d \times N\), but the number of each individual’s links is not necessarily \(d\). In other words, the link amount can vary from individual to individual. The linked individuals can be regarded as friends who can exert normative influence on each other. All individuals are connected to all authorities by incoming links, which reflects that ordinary individuals “follow” hubs and receive information from them, but not the other way around. However, authorities’ influence on their followers is regulated by the weighted importance of the links between them, which is determined by the similarity principle with respect to the fears of vaccines and disease. Lastly, all the links are dynamical in the sense that the strength of a link depends on individuals having (dis)similar fears, and if they are very dissimilar, the link can even be abandoned and rewired due to a social conflict.
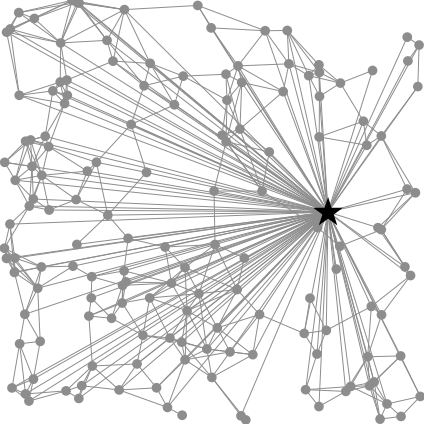
One of the attributes of individuals is the availability heuristic, \(AvH\), which measures how individuals process information from authorities. In our model, the \(AvH\) mechanism plays two roles. First, the higher the \(AvH\) value, the more likely that an individual relies on information from authorities rather than from friends, especially when the individual has high fears. In our model, such individuals preferentially communicate with authorities that are most similar to them. Second, authorities holding extreme positions can often make the deepest and most lasting impressions upon ordinary people. Therefore, in addition to the principle of similarity, we introduce another rule: with a probability equal to \(AvH\), individuals are influenced by extreme authorities, which amplifies personal risk estimation. To sum up, on the one hand, \(AvH\) modulates the influence on individuals by authorities relative to friends. On the other hand, it modulates the influence on individuals by extreme authorities relative to similar authorities.
Another attribute of individuals is called opinion, denoted as \(O \in [-1, 1]\), which is calculated according to two kinds of fears. Positive or negative opinion values represent those for or against vaccination and absolute opinion values indicate attitude strength.
Each individual can assume one of four states, denoted by yellow, red, green and white in the model, and belong to one of two groups, vaccinated and unvaccinated groups (Figure 3). In the beginning, all individuals are not vaccinated. Then, with the elapse of time, some individuals’ satisfaction of vaccination exceeds the threshold, thereby choosing to receive the injection. After that, their status change from \(vaccination?\) \(= false\) to \(vaccination? = true\), meanwhile, their colour changes from yellow to green. With a probability equivalent to the incidence of side effects, the vaccinated population will change into white, with increased \(F_{v}\) and decreased \(F_{d}\). All individuals who do not receive vaccination have the same status, namely \(vaccination? = false\). However, we distinguish two kinds of individuals in this unvaccinated group. Some of them meet two conditions simultaneously, that is, not only their satisfaction of being not vaccinated (\(Suv\)) is above the threshold, but they also hold extreme anti-vaccination attitudes (\(O < - 0.8\)). We thus set their status as \(anti-vaccination? = true\), and change their colours from yellow to red, to directly observe the extent of polarisation. In short, yellow individuals may turn green or red; a minority of green individuals may turn white because of side-effects but can never change into yellow or red; finally, the red individuals have the chance to convert into green ones. It should be noted that all these status changes, except for those involved in side effects, do not change fears or opinions, but help agents to better find similar others in their cognitive dissonance reduction behaviours. Although the vaccinated group cannot unvaccinate themselves, nevertheless, possibly they can accept social influence and become very negative toward vaccines (\(O < 0\)). On the other hand, it is also possible for the unvaccinated group to become quite positive due to social influence (\(O > 0\)). More parameters used in our model are listed in Table 1.
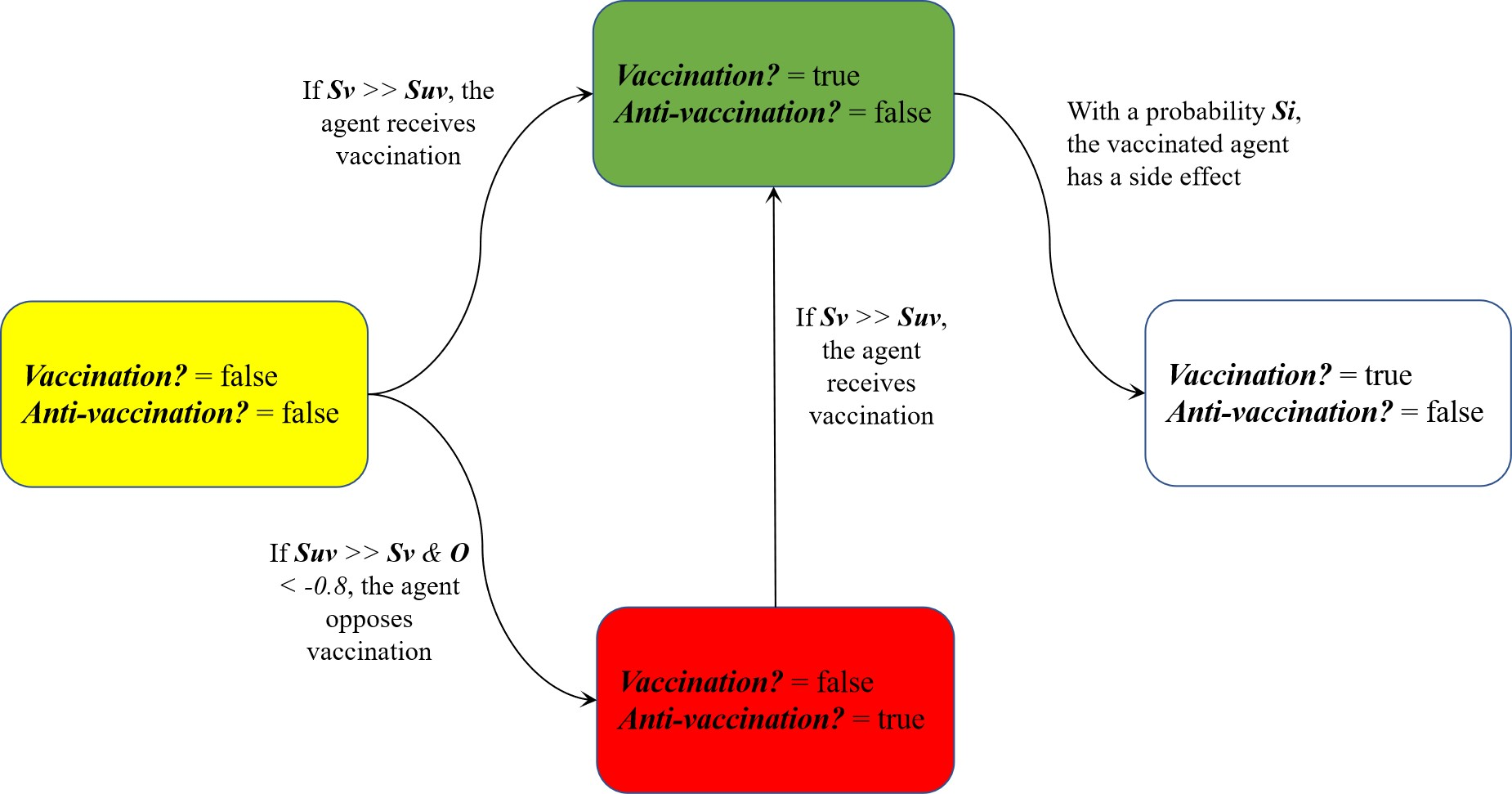
Parameter | Description | Dynamic | Range |
---|---|---|---|
\(N\) | The number of individuals | No | Natural number |
\(N_{a}\) | The number of authorities | No | Natural number |
\(d\) | Network degree, describing the density of network connections | No | Natural number |
\(F_{v}\) | The level of fear of vaccination | Yes | [0, 1] |
\(F_{d}\) | The level of fear of disease | Yes | [0, 1] |
\(O\) | The attitudes toward vaccination | Yes | [-1, 1] |
\(\mu\) | Degree of change in opinion | No | [0, 1] |
\(E\) | Probability of interacting with others | No | [0, 1] |
\(\beta\) | Perceived disease severity | No | [0, 1] |
\(S_{i}\) | probability of side effects in vaccinated individuals. | No | [0, 1] |
\(\alpha\) | The relative importance of fear of vaccines in the similarity function | No | [0, 1] |
\(I_{e}\) | The importance of experiential needs | No | [0, 1] |
\(I_{s}\) | The importance of social needs | No | [0, 1] |
\(I_{v}\) | The importance of value needs | No | [0, 1] |
\(T\) | Threshold of cognitive dissonance tolerance | No | [0, 1] |
\(AvH\) | Level of availability heuristics | No | [0, 1] |
\(vaccination?\) | Vaccination status | Yes | True/false |
\(anti-vaccination?\) | Anti-vaccination status | Yes | True/false |
\(CmB\) | Switch on/off confirmation bias | No | True/false |
Model flowchart
The flowchart of the vaccination decision-making process for an individual is shown in Figure 4. The main idea is that the decision to get vaccinated or not depends not only on oneself, but also others, such as friends in your social network and authorities with greater influence.
Individuals first modify their fears when interacting with others and form initial opinions on vaccination accordingly. But whether to take action to vaccinate is ultimately determined by the overall satisfaction of the three types of needs.
The update of satisfaction, the key adaptive process, is modelled according to the HUMAT framework (Antosz et al. 2019). An update of experiential, social and value satisfactions depends on initial opinions generated from fears, the vaccination level among friends and innate personality. Then individuals take the most satisfying action.
The HUMAT framework regards cognitive dissonance as the motive for post-decision actions. In this model, we assume that individuals hope to minimise their cognitive dissonance in making decisions. In addition, they consider two kinds of dissonance-reduction strategies. First, individuals may signal their satisfaction with the decision to their friends through the network, even with exaggeration, to persuade more people in their social network to take the same actions. Second, individuals may adopt biased information processing methods, including searching for information that supports their decisions and avoiding communication with those who make different decisions by adjusting personal network structures. In particular, vaccinated individuals have a larger motivation to act in a confirmation bias since they are unable to unvaccinate themselves.
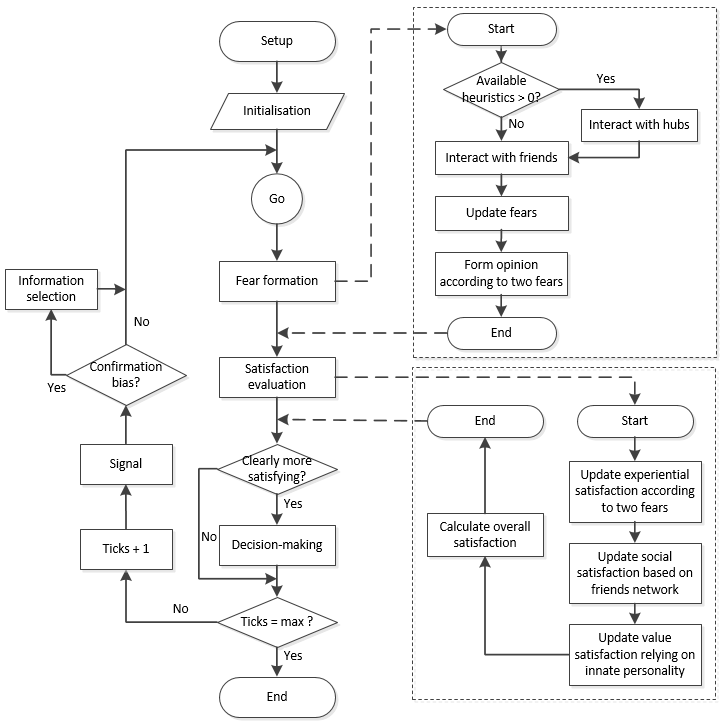
Simulation experiment
We present a series of simulation experiments to explore how social interactions between individuals and authorities influence the vaccination rate and opinion dynamics. By varying parameters related to the agent’s information processing (micro-level), such as the presence of the availability heuristic, the confirmation bias, and the type of authority that the individual is following, we will observe the societal opinion dynamics (macro-level) outcomes of our model including the obtained vaccination rate and the distribution of opinions.
Three scenarios are constructed to analyse the sensitivity of the opinion dynamics for different information processing mechanisms, as shown in Table 2. The default settings of the model are as follows:
- There are 500 individuals and each of them has five friends on average (\(d = 5\));
- Each of the three needs has equal importance;
- The model runs for 200 steps in each experiment.
A sample size of 500 individuals was used to explore the problem, as it provides sufficient representation, while considering model running time constraints. More detailed settings of parameters are described in Appendix 1.
Parameter | Description | Scenario 1 | Scenario 2 | Scenario 3 |
---|---|---|---|---|
\(\beta\) | Perceived disease severity | (0, 1) | 0.5 | 0.5 |
\(CmB\) | Confirmation bias | False | True | False |
\(N_{a}\) | Type of authorities | 0 | 0 | [1, 5] |
\(AvH\) | Level of availability heuristic | 0 | 0 | [0, 1] |
We conducted a sensitivity analysis for different importance of disease severity and the side effects of vaccination in Scenario 1 to test H1. The importance of perceived disease severity was measured by \(\beta \in (0,1)\). If \(\beta > 0.5\), individuals believe the disease is more dangerous than the possible side effects of vaccination. If \(\beta < 0.5\), they believe the opposite. If \(\beta = 0.5\), the dangers are regarded as equal and their impacts on individuals’ current decisions will be equal too. However, in the next step, individuals with high values of fear were more likely to follow authorities, as described in Scenario 3. To simplify, we call \(\beta\) disease severity, but in fact it measures the relative risk of disease and vaccine side effects. This parameter setting can help us to understand the effect of changes in disease severity, as can be resulted from virus mutation. For example, when the virus gets weaker, people were less scared of the disease than of the side effects of vaccinations.
In Scenario 2, we intended to find the impact of confirmation bias (\(CmB\)) and test H2. Confirmation bias implies that after deciding to vaccinate or not, an agent tends to interact with those other agents who hold the same position and avoid dissonant information from different others. In the model, this produced network dynamics, as individuals may form new links with similar others and break links with different others. Confirmation bias, for simplicity, was modelled as a binary in this study, although it could also be modelled differently (e.g., Vogrin et al. 2023). \(CmB\) was designed as a true/false parameter. When \(CmB = true\), vaccinated individuals will disconnect from random anti-vaccination friends and connect with a random vaccinated stranger and anti-vaccination individuals will act just the opposite. However, when \(CmB = false\), individuals will not alter their network voluntarily or interact with others selectively.
Scenario 3 focused on the effect of authority, including the number/type of authorities (\(N_{a}\)) and the influence of authorities (\(AvH\)), which is related to H3a and H3b. The opinions of authorities are evenly distributed between -1 and 1, or from extremely anti-vaccination to extremely pro-vaccination. Specifically, when there is only one authority, its opinion is on either of the two extremes. When there are two authorities, they will stand opposite at the two extremes. Otherwise, when there are more than two authorities, their opinions will distribute from -1 to 1 at equal intervals. Therefore, a larger number of authorities, means there are more moderate opinions. Table 3 shows the types and opinions of the authorities in this experimental design.
\(N_{a}\) | 1 | 2 | 3 | 4 | 5 |
---|---|---|---|---|---|
Opinions | \(\{-1\}\) or \(\{1\}\) | \(\{-1, 1\}\) | \(\{-1, 0, 1\}\) | \(\{-1, -0.33, 0.33, 1\}\) | \(\{-1, -0.5, 0, 0.5, 1\}\) |
Next, we were interested in the relationship between opinion dynamics and the influence the authorities exerted on individuals, which is mediated by the availability heuristic (\(AvH\)). An important mechanism here is about dynamics between availability heuristic, fears, and the tendency to follow an authority with a similar (consonant) opinion. We assumed that, on the one hand, the more fearful agents were more likely to follow authorities, as fear is a factor inducing obedience to authority (Jørgensen et al. 2021). Additionally, the principle of similarity determines that everyone tends to agree and follow foremost the authority whose opinion is closest to herself. On the other hand, (social) media tended to emphasise incidents, which may cause that information from authorities may result in people over-emphasising the seriousness of the disease or side effects of the vaccine, which is significantly related to availability bias (Brezis et al. 2014). In our model, if \(AvH\) was set to 0, interactions between authorities and individuals were totally neglected. At a higher level of \(AvH\), authorities, especially the extreme authorities, could exert more extensive influence on agents.
The output of the simulation experiments is the evolution of the vaccination rate, the distribution of opinions regarding the risks of COVID and vaccination and group satisfaction. The vaccination rate is measured by the proportion of vaccinated agents. Polarisation can be measured in a variety of ways. Inspired by the study of Gestefeld et al. (2022), a graphical approach to the distribution of opinions was adopted to compare the degree of polarisation according to the following principles. First, other things being equal, polarisation becomes higher when the centre opinion loses people to the opposite extremes (Esteban & Ray 1994). In this situation, a normal distribution of opinions indicates little polarisation while a bimodal distribution indicates a high polarisation. Second, polarisation increases as the distance between opinion peaks becomes larger in the sense of divergence. The average satisfaction of all members of each group was used as an indicator of the overall satisfaction of vaccinated (\(S_{v}\)) and unvaccinated groups (\(S_{uv}\)).
Results
Scenario 1: The effect of disease severity
We first investigated whether changes in disease severity (\(\beta\)) affect vaccination rates and opinion dynamics. We assumed that the greater the severity of the disease, the greater the likelihood that fear of the disease’s health consequences outweighs fear of vaccination’s side effects. To reduce the influence of random factors on the results, the experiment was repeated 20 times and the results were summarised by marking the 95% confidence interval around the mean in Figure 5.
Figure 5 depicts the evolution of the vaccination rate for three disease severity levels (\(\beta = 0.3\), \(0.5\), and \(0.7\)). It suggests that when the disease is more severe, the vaccination rate rises, consistent with H1. At a disease severity of 0.7, approximately 90% of individuals choose to be vaccinated at some point, and the vaccination process is relatively quick. A lower disease severity (\(\beta = 0.3\)) results in lower motivation for vaccination and a lower vaccination rate (less than 60%). We ran the experiments when \(\beta\) varied from 0 to 1 to get a comprehensive picture of the impact of \(\beta\) on vaccination degree. Figure 16 in Appendix 2 depicts a similar picture.
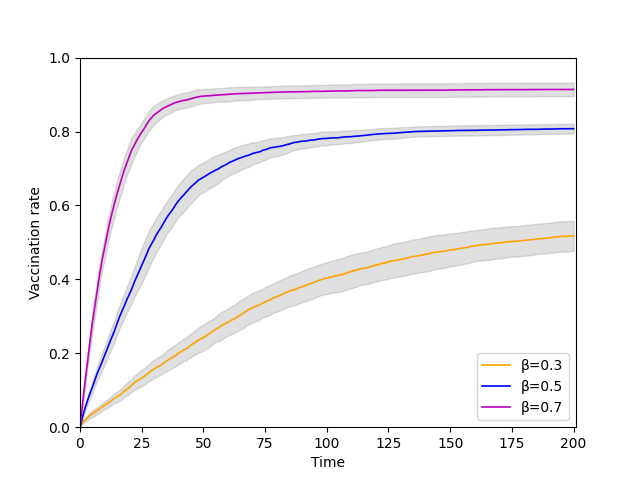
Following that, we investigated the effect of disease severity on opinion distribution in a single run. Figure 6 shows that when \(\beta\) is extremely low (or extremely high), the majority of opinions gravitate toward the anti-vaccination (or pro-vaccination) extreme. When the value of \(\beta\) was in the middle range, the severities of the disease and vaccination are comparable, resulting in more diverse opinions.
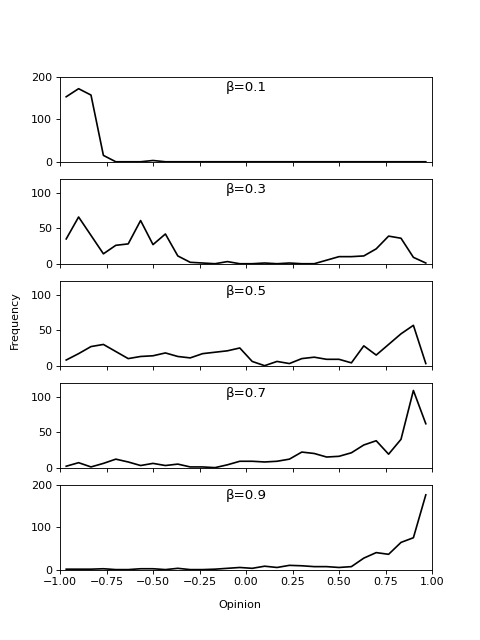
The satisfaction of the three types of needs in HUMAT was then examined for both vaccinated and unvaccinated groups. Figure 7 shows that as \(\beta\) increases, the overall satisfaction of the vaccinated group (\(S_{v}\)) rises while that of the unvaccinated group (\(S_{uv}\)) falls slightly. When \(\beta = 0.4\), the two groups’ overall satisfaction is equal (see the red dot on the black line, \(S_{v} = S_{uv}\)). When \(\beta < 0.4\), the vaccinated group has lower overall satisfaction than the unvaccinated group (\(S_{v} < S_{uv}\)); when \(\beta > 0.4\), the result is reversed (\(S_{v} > S_{uv}\)).
Further examination of the contribution of the three needs to the overall satisfaction revealed that first, social needs had the greatest impact on the satisfaction for both groups, particularly the unvaccinated group. Among the three types of needs, social needs played the most important role not only in promoting vaccination for the vaccinated group but in inhibiting vaccination for the unvaccinated group. This is because, as disease severity increases, more people in their social networks were vaccinated (see Figure 5) and the unvaccinated individuals felt social pressure, which lowered their social satisfaction. Second, when \(\beta < 0.5\), fear-based experiential satisfaction had an inhibiting effect on \(S_{v}\). When \(\beta > 0.5\), however, the effect is reversed. Experiential satisfaction was always positive and higher than \(S_{uv}\), indicating that despite the increasing severity of the disease, the unvaccinated group’s fear of the disease is lower than their fear of the side effect of vaccination. However, as social pressure for vaccination grows, their overall satisfaction decreased. Third, because one’s sense of value is difficult to change, value-related parameters in our model were set to be stable. As a result, it is not surprising that the value satisfaction did not change significantly with \(\beta\).
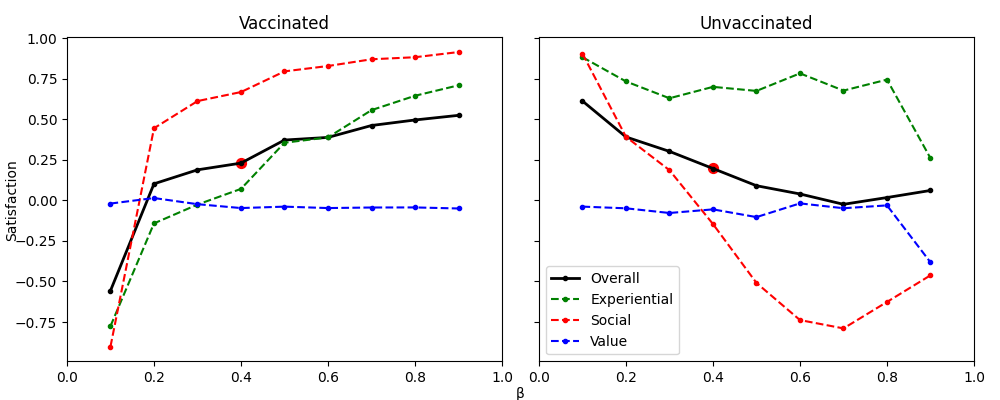
Another relevant question is how viral mutation affects opinion polarisation. We assumed that the virus was weakened through mutation, leading to a reduction in an individual’s perceived fear of disease (\(\beta\)). Accordingly, a supplementary experiment was conducted by setting disease severity to decrease over time. Figure 8 (left) shows that when the disease severity decreases, the peak opinion gradually shifted from positive to negative, with the level of polarisation first increasing and then decreasing. In contrast, Figure 8 (right) shows that the opinion distribution consistently skews towards the negative end when disease severity is constant.
Looking at the curve of overall satisfaction in different groups in Figure 9, we found that the satisfaction of the vaccinated group first increases briefly and then decreased over time, while the satisfaction of the unvaccinated group increased slowly and eventually exceeded that of the vaccinated group.
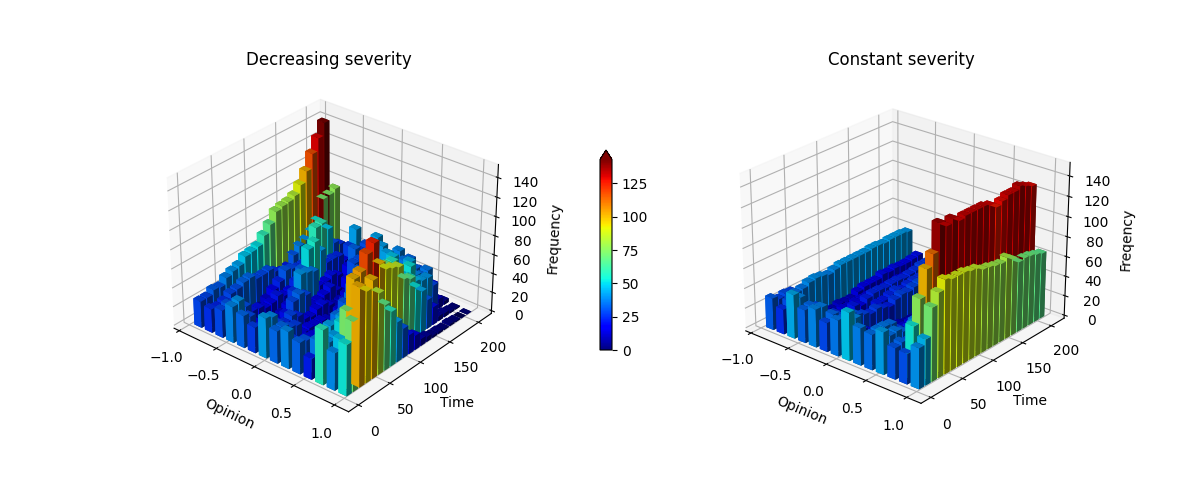
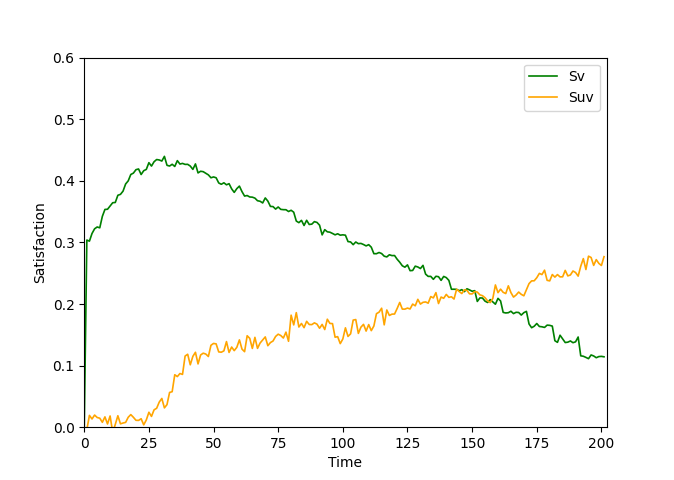
Scenario 2: The effect of confirmation bias
To examine the effect of confirmation bias on vaccination rates, we compared biased individuals (\(CmB = true\)) and unbiased individuals (\(CmB = false\)). The experiment was repeated 100 times, and the results from 200 steps using a 95% confidence interval are shown in Figure 10. It is clear that confirmation bias reduced vaccination rates. Without confirmation bias, the average vaccination rate was 87%, which is nearly 10% higher than when confirmation bias was present.
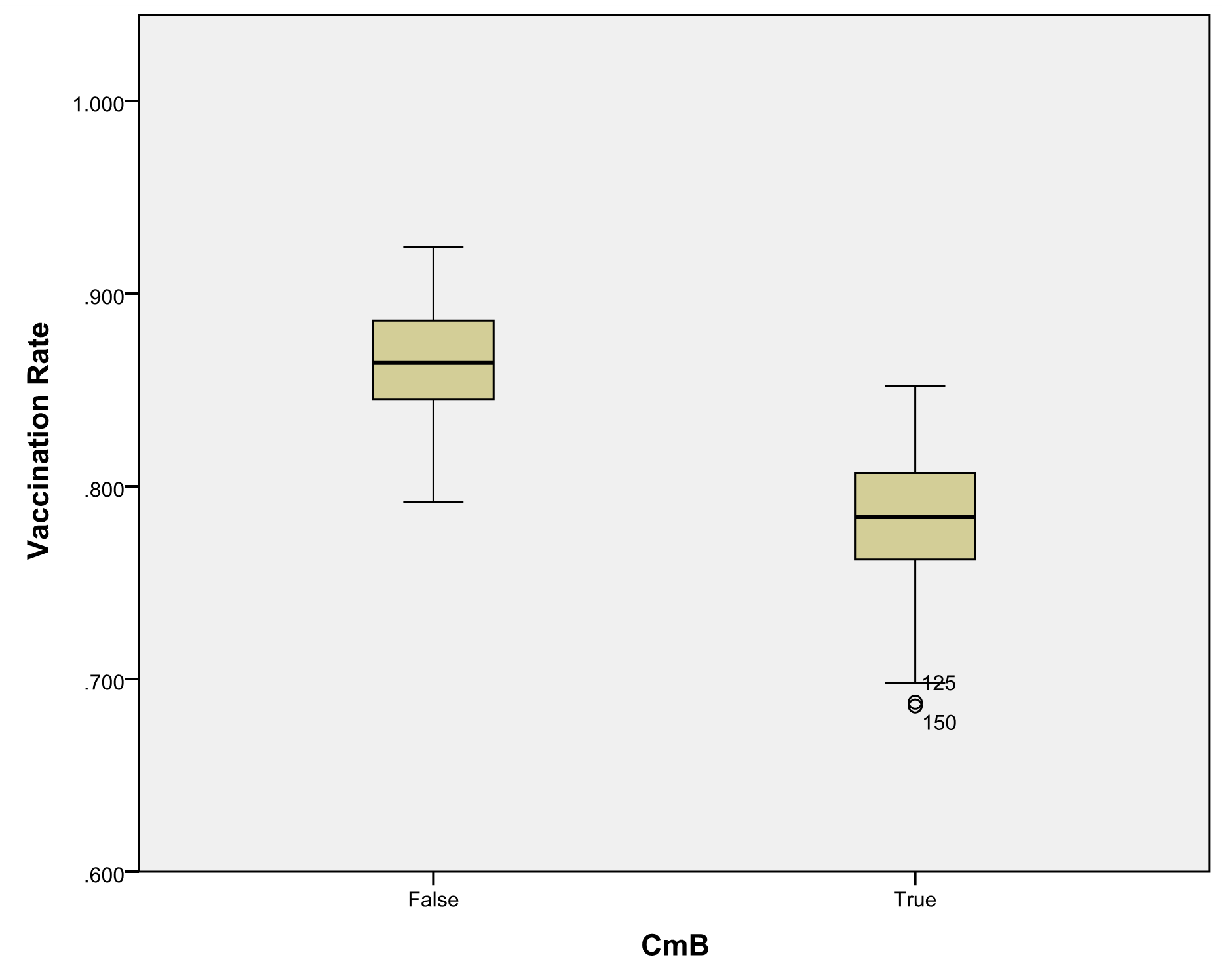
Figure 11 depicts the experimental results of the effect of \(CmB\) on opinion polarisation. When \(CmB = true\), opinions were more polarised in the end, whereas when \(CmB = false\), the distribution of opinions was more diverse, which supports H2. Confirmation bias, in particular, caused a greater proportion of moderate individuals to gravitate toward the opposite extremes, and the overall distance between opinion peaks was greater than it would be without confirmation bias. Figure 17 depicts a 3D evolution diagram that allows us to fully observe the process by which opinions evolve over time. Individuals’ opinions change from the initial random distribution to the final state of polarisation with confirmation bias, confirming the promoting impact on opinion distribution.
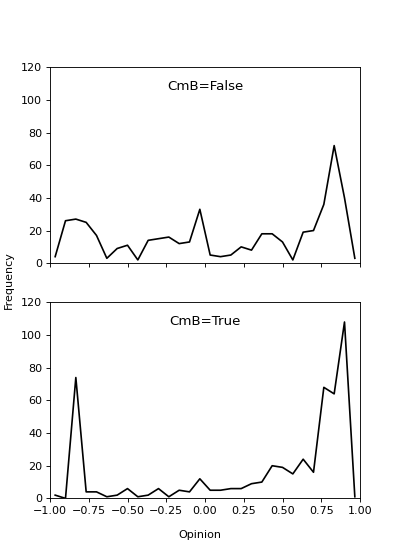
Subsequently, we continued to investigate satisfaction in various groups. Figure 12 depicts the evolution of overall satisfaction in the vaccinated (\(S_{v}\)) and unvaccinated (\(S_{uv}\)) groups in the presence or absence of \(CmB\), while Figure 13 depicts satisfaction on three needs in each case.
Figure 12 shows that the addition of \(CmB\) (green curve) increased overall satisfaction in both groups by about 20% when compared to a no-\(CmB\) situation (red curve). To some extent, this confirms that confirmation bias actions do, as expected, reduce cognitive dissonance. Furthermore, by looking at the evolution of the satisfaction of three needs in Figure 13, we can see that the increased overall satisfaction was primarily due to increased social satisfaction. When \(CmB\) exists, individuals actively seek out and connect with other individuals who have made similar decisions. As a result, neighbours became more similar than they were previously, better meeting their social needs.
The vaccinated individuals with confirmation bias had the highest level of overall satisfaction, while the unvaccinated individuals with no confirmation bias had the lowest. We investigated the interesting results about the satisfaction of experiential needs further because personal subjective satisfaction is probably a better indicator of individual feelings than overall satisfaction. Because experiential satisfaction is derived from personal fear of disease and fear of vaccine side effects, it more accurately reflects individuals’ subjective feelings and initial opinions as individuals. For example, when the fear of side effects was higher, the vaccinated group’s experiential satisfaction was lower; however, the unvaccinated group’s experiential satisfaction could be higher. As shown in Figure 13, vaccinated agents’ experiential satisfaction decreased over time, implying that while more people choose to be vaccinated, their personal satisfaction was, compared to unvaccinated individuals, low. The unvaccinated group, on the other hand, had higher experiential satisfaction, indicating that they were satisfied on a personal level despite high social pressure.
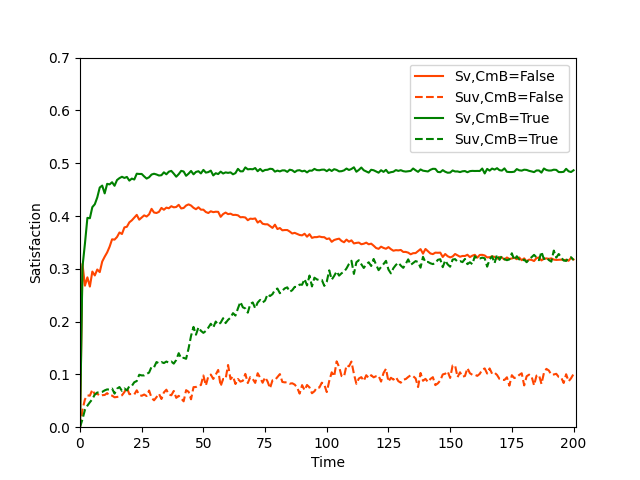
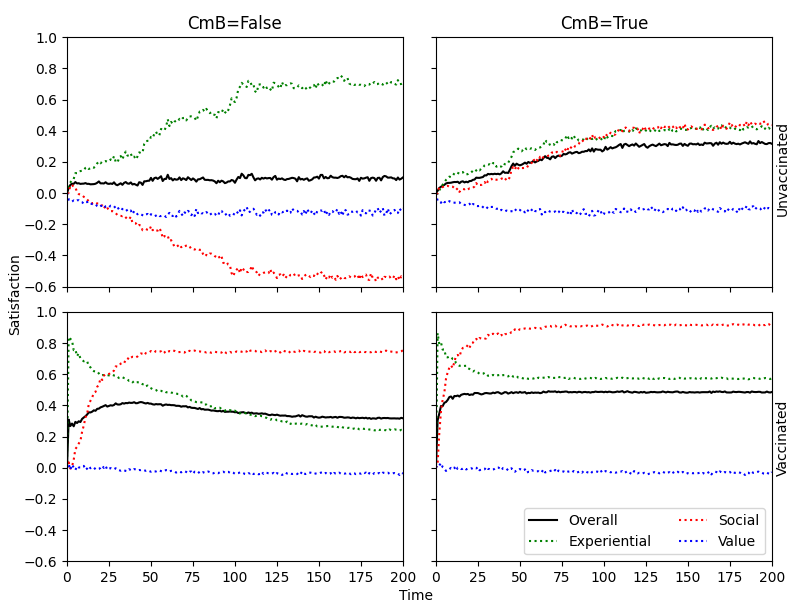
Scenario 3: The effect of authority
We introduced authorities in this scenario to investigate how they contributed to the opinion distribution. Our approach was to investigate the impact of authorities with heterogeneous positions, such as moderate or more extreme positions, on opinion dynamics.
Figure 14 depicts the distribution of opinions under different numbers/types of authorities (\(N_{a}\)) when \(AvH\) is low (a) or high (b). Figure 14(a) shows a single anti-vaccine authority causes more polarisation than a single pro-vaccine authority. When there was only one pro-vaccine authority, social opinions tended to focus on the right end of the spectrum, indicating a pro-vaccination consensus. In contrast, if the only authority was of the anti-vaccine type, while most agents end up with pro-vaccination opinions, there were a significant number of anti-vaccine agents. When there are two authorities with strong and opposite opinions, the most polarised opinions in the population emerge. While increasing the authority of moderate opinion, polarisation decreased, which supports H3a.
Figure 14(b) shows the distribution of individual opinions as the value of \(AvH\) is increased. A comparison of Figure 14(a) and (b) shows that a higher \(AvH\) promotes opinion polarisation when there are more moderate authorities (\(N_{a} = 5\)), which supports H3b. Heuristic information processing manifests itself in the sensitivity to extreme opinions, as assumed in our model. The greater the \(AvH\), the greater the influence of authorities, particularly extreme authorities, on individuals, promoting opinion polarisation. To fully observe the process by which opinions evolve with time, a 3D evolution diagram is presented in Figure 18.
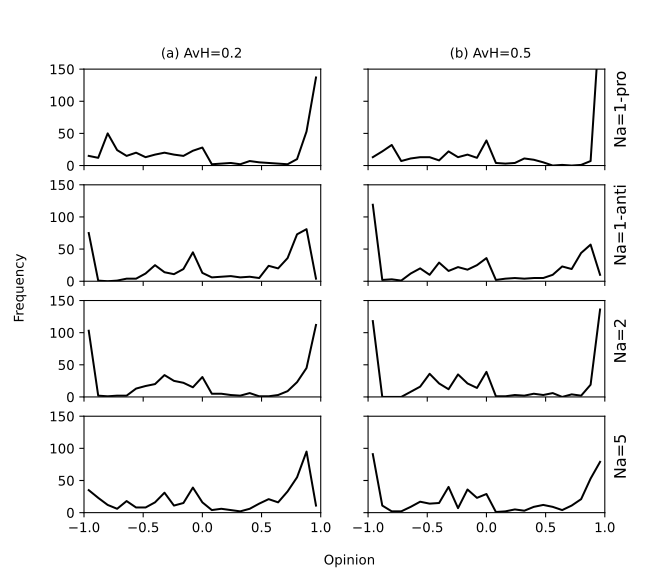
As the previous experiment (Figure 11) shows, confirmation bias promotes polarisation. Here, Figure 14 indicates that moderate authorities tended to reduce polarisation compared with extreme ones. This raises a question: to what extent does the number of moderate authorities offset or cancel out the effect of polarisation caused by confirmation bias? With this in mind, we conducted an experiment with confirmation bias turned on and the number of authorities (\(N_{a}\)) gradually increased from 2 to 9. The result in Figure 15 shows that, as the number of moderate authorities rises, polarisation becomes mild but still exists.
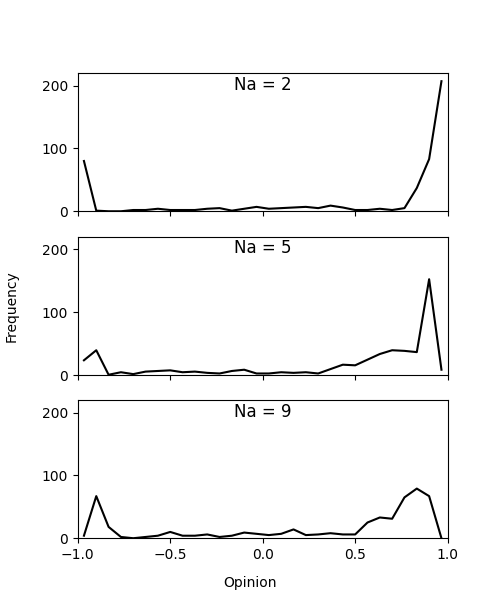
We demonstrate that more experiments are possible when considering, for example, the effectiveness of vaccines and the number of agents in our model. The scenarios we presented here serve primarily as proof of concept. Supplementary experimental results are shown in Appendix 2.
Discussion and Conclusions
We investigated how fear, availability heuristics, the tendency to follow authorities, and confirmation bias, which causes people to lean toward information that confirms their choice, affect the dynamics of opinion about COVID-19 vaccination. The effects on vaccination rates were also investigated. We added mechanisms for availability heuristics and confirmation bias, as well as a relationship between fear and obedience to authority, to the HUMAT framework (Antosz et al. 2019).
The findings of our simulation study suggest that the empirical overestimation of risks of both COVID-19 and the side effects of vaccination can be explained by the impact of fear on information processing and availability heuristics. Furthermore, such mechanisms can contribute to social polarisation. Confirmation bias, for example, was responsible for an unbalanced polarisation: while a majority group supports vaccination a minority group strongly opposed it.
Scenarios and results
We simulated three scenarios to get a clear insight into how the implemented mechanisms affect the opinion dynamics and degree of vaccination.
Scenario 1 investigated how the perceived severity of the disease affects the outcomes. This is practically significant because the virus’s perceived danger varies as it evolves. According to intuition, as disease severity decreases, vaccination becomes less urgent and individuals tend to get vaccinated less. Furthermore, the results showed that as the virus mutates, the satisfaction of the vaccinated people eventually declines. This resembles the observation that increasingly people report in (social) media to regret having been vaccinated.
Scenario 2 examines the effect of confirmation bias, as demonstrated by agents’ proclivity to avoid contradictory information and seek supportive evidence to back up their decisions. This was accomplished in the model through post-decision behavioural settings. Confirmation bias, it turned out, lowers vaccination rates while increasing opinion polarisation. This could be attributed to the fact that as more people are vaccinated, the social pressure to vaccinate grows stronger, but such a norm is constrained by confirmation bias, which encourages individuals to adjust their network by connecting with similar others. As a result, segregation will most likely establish and obstruct the further spread of vaccination as a social norm among unvaccinated groups. When confirmation bias exists, the satisfaction of both vaccinated and unvaccinated groups increases significantly, indicating that while confirmation bias improves personal satisfaction, it exacerbates societal opinion polarisation in society.
In Scenario 3, we introduced authorities to investigate their impact on the distribution of opinions. The level of availability heuristic was used to calculate the frequency of individuals interacting with authorities at each step. We discovered that when there were two authorities with opposing opinions, the individuals’ opinions were most polarised. When more (moderate) authorities are added to the model, opinion polarisation decreases but does not disappear. Furthermore, simulation results revealed that availability heuristic, regardless of the number or type of authorities, promotes opinion polarisation.
When the availability heuristic, confirmation bias, and tendency to follow informative authorities interact in the context of fear eliciting societal problems, the model exhibits complex social dynamics. The findings revealed that sharing emotions and fear-inducing content on social media can exacerbate social division, and that risk misperception is to blame for social polarisation.
Opinion and behaviour
We first identify fear as one of the key psychological drivers for opinion formation and dynamics by referring to the Agent_Zero theory (Epstein 2014). According to the correlation between the media and COVID-19 risk perception (Rivas et al. 2021), authorities influence people’s fears of disease and vaccines. We assumed that individual opinions are formed directly from two types of fears: disease fear and vaccination fear. Our model in particular, distinguishes between opinions and behaviour. The former are fear-related attitudes, while the latter includes final vaccination decisions and decisions to reduce cognitive dissonance. Vaccination decisions are influenced by overall satisfaction, whereas dissonance-reduction actions are motivated by confirmation bias, which causes people to seek information that supports their decisions.
Implications for science communication
The results of the simulation experiments showed that due to biases there is a strong tendency towards ending up in a polarised situation, and that opinion leaders play an important role in this process. The results also demonstrated that having more opinion leaders with a moderate position reduces the polarisation in society. Here, we wanted to remark that many media stimulated debate over complex matters, where often conflicting perspectives are being debated, which emphasises the polarised perspectives. However, many complex societal problems are uncertain by nature and presenting a more nuanced perspective on such issues and stimulating a dialogue between people with different perspectives may contribute to a reduced polarisation in society on complex problems. This raises questions on how science communications could be improved regarding the communication of complex and uncertain societal problems.
Limitations and future work
Our model aims to explain the opinion dynamics and polarisation surrounding COVID-19 vaccination, and it is adaptable to other social contexts such as public discussions on climate change, transitioning to a plant-based diet, elections and opinions on conflict and war.
Unlike previous infectious disease models, we do not take virus spread or human immunity into account. Instead, we focused on the spread of fear of disease and fear of vaccines. Although it is a simplified model, more mechanisms could be in the future. This model, for example, only considers one-time vaccination, but in practice, booster shots and multiple vaccinations are also common and these can be discussed further. In addition, the waning effectiveness of vaccines can also be an interesting question worth exploring. It may also be interesting to couple the current model with an interdisciplinary policy model like ASSOCC (Dignum 2021) to explore how policies might interfere with biased information processing.
Further research should consider how different policies affect opinion dynamics and vaccination rates in different cultural settings. For example, more collectivistic (Eastern) cultures may react differently to certain policies (e.g., authoritarian) and information than more individualistic (Western) cultures. Hofstede’s cultural dimensions theory (Hofstede 1980) may be a useful extension here, particularly because it has been formalised in agent-based models (Hofstede et al. 2012). Additionally, the model opens possibilities for exploring how people respond to repeated vaccination schemes under changing virulence and vaccination side effects.
Acknowledgments
Work in this paper has been supported by the European Commission’s Horizon 2020 project SMARTEES – Social Innovation Modelling Approaches to Realising Transition to Energy Efficiency and Sustainability (grant agreement no. 763,912) and partly supported by the China Scholarship Council (grant agreement no. 202206760072). The reviewers of the European Social Simulation Conference are thanked for their valuable comments on an early version of this paper. Finally, the anonymous reviewers of JASSS are being thanked for their rich and valuable comments on a previous version of this paper.
Model Documentation
The model was implemented with NetLogo 6.0.4. The full code, model, and the ODD protocol are openly available on the CoMSES Computational Model Library at: https://www.comses.net/codebase-release/75cc6242-a040-4dd3-b712-1085bad7ce45/.
Appendix 1: Agent-Based Model Applying the ODD Protocol
Overview of the model
Purpose
The purpose of the model is to demonstrate the fear-driven opinion dynamics of COVID-19 vaccination, especially how fears, cognitive biases, and the role of authorities contribute to the social polarisation and vaccination rate. For this, we develop an integrated model that comprises dynamic decision-making processes, such as information exchange, fear formation, satisfaction evaluation, and dissonance reduction. Specifically, we carry out simulation experiments to analyse three scenarios that are designed by different target mechanisms. Scenario 1 analyses the mechanisms of disease severity, Scenario 2 focuses on the impact of confirmation bias, and finally, Scenario 3 explores the role of authority.
Entities, state variables and scales
There are two types of individual-level entities. One is the susceptible individuals. Each individual is denoted as \(a_{i}\). Individuals are characterised by a set of state variables: fear, needs, and cognitive biases. Fears, including fear of vaccines (\(F_{v}(a_{i})\)) and fear of disease (\(F_{d}(a_{i})\)), are formed by risk information from authorities and friends. Individuals will initially have a random fear value, and then be influenced by them in every interaction with friends and authorities, eventually forming a new fear value. \(F_{v}(a_{i}) \in [0,1]\), indicates how much danger \(a_{i}\) would feel if vaccinated, and \(F_{v}(a_{i}) = 0\) represents \(a_{i}\) doesn’t care about vaccine side effects at all, while \(F_{v}(a_{i}) = 1\) means \(a_{i}\) is extremely afraid of side effects of the vaccine. Similarly, fear of disease (\(F_{d}(a_{i})\)) describes the risk-estimation for contracting COVID-19, which has the same formation mechanism as \(F_{v}(a_{i})\). In addition, individual needs are also the key drivers of decision-making as per HUMAT (Antosz et al. 2019), providing a measure of decision satisfaction. Three categories of needs or values are considered in the model: experiential needs (\(Nexp(a_{i})\)), social needs (\(Nsoc(a_{i})\)), and values (\(Nval(a_{i})\)). Correspondingly, three kinds of satisfaction with a specific decision are calculated. An individual will act only when the satisfaction of action is sufficiently great, that is, vaccination will occur when the overall satisfaction of three needs is greater than the threshold. Cognitive biases in this model focus on the mechanisms of availability heuristics (\(AvH\)), which measure the degree of influence by authorities on decision-making, and confirmation bias (\(Confirmation-bias?\)), which comes into play after taking action to vaccinate or not vaccinate as a means of reducing cognitive dissonance. \(AvH\) is a continuous variable between 0 and 1 and \(CmB\) is set to a binary variable. Table 1 shows details of parameters for individuals.
The other is the authorities, which act as hubs in the network. Each authority is denoted as hj, the authority in the social network representing the authority with large influence through (social) media. These authorities disseminate different risk information that evokes different fear of vaccines \(F_{v}(h_{j})\) and fear of disease \(F_{d}(h_{j})\) among individuals, but their attitudes towards vaccines and diseases are relatively stable. Here the role of authorities in social networks is similar to that of the fifth neighbour mentioned in Shibanai’s paper (Shibanai et al. 2001), with a different chance of selection from other neighbours or friends at each step. The chance, denoted as \(P_{h}\), is controlled by the collective effect of the level of the availability heuristic (\(AvH\)) and total fears of an individual, measuring the probability of interaction of individuals with the authorities. We set \(AvH\) to be uniform but the total number of fears of an individual is dynamic. Therefore, we assume that agents can either interact with authorities with a probability of \(P_{h}\) or with friends with a probability of (\(1 - P_{h}\)) in the network, both of which are based on the similarity principle (Axelrod 1997).
The societal-level entities are links that describe the social interaction relationships among individuals and relationships between individuals and authorities. How individuals interact with others depends on whom they are connected with through the social network. Figure 2 is a simplified illustration of the network structure that is to be used in this study to connect a large number of agents. Individuals in the model fully contact all authorities by incoming links but contact limited other agents by undirected links. One state variable of links is the weighted importance indicating the chance of agents to interact with each other. The weighted importance is measured by the similarity principle with respect to fear of vaccines and fear of disease, assuming that the more similar a pair of agents are, the more likely they are to influence each other. In our similarity function, the greater the sum of the differences in two fears of a pair of agents, the lower the similarity calculated, and therefore, the weighted importance of the link will be low, leading to a small probability of their interaction. When the sum of the differences in two fears is 0, the weighted importance of the link equals 1, which means the two agents will influence each other with a probability of 100%, but as they are already congruent with each other, there is no room for further change. When the sum of the differences in two fears is 1, the weighted importance is 0, indicating that the two agents will not influence each other although they are linked. One of the dynamics of the model is that updating either of these fears creates a dynamic network.
Process overview and scheduling
When receiving the risk information of diseases and vaccines from authorities and linked agents, the agents evaluate the information and estimate the risk with a certain degree of bias. Based on the HUMAT framework (Antosz et al. 2019), the process of forming an opinion about vaccination and taking adaptive action can be divided into the following stages and the basic structure of the decision-making flowchart is given in Figure 4.
Fear formation
The level of fear each individual has about vaccines and disease is related to the risk information they receive from authorities and connected friends. For each step, the individual interacts with the authorities at a probability of \(P_{h}\) and with the friend at a probability of (\(1-P_{h}\)).
Interact with authorities. There are different authorities with different opinions that will influence susceptible individuals. When interacting with authorities rather than friends at this step, individuals decide which authority to finally follow based on the similarity of the authority’s risk information to the individual’s risk perception. An individual is more likely to listen to an authority who expresses a similar opinion than one who has a dissimilar opinion. But this similarity is threatened by the availability heuristic, that is, the larger the availability heuristic, the more affected by extreme authority than similarity authority.
Interact with friends. When an individual agent chooses to interact with friends, it will randomly select a friend from its social network without the similarity principle. This is because we assume that there is an inherently more consistent similarity between friends.
Form an initial opinion on vaccination
When the two fears are updated, the opinion of vaccination can be formed accordingly. And vaccination opinion is positively correlated with fear of disease and negatively correlated with fear of the vaccine. Here we also take into account the role of virus severity on the relative weight of the two fears in opinion formation. We assume that the more severe the disease, the higher the fear of the disease affects opinion formation, and the more positive attitudes toward vaccination.
Satisfaction evaluation of three needs
The decision-making process will continue until action is taken to get vaccinated or not. Referring to the HUMAT framework (Antosz et al. 2019), the initial fear-driven opinion is related to the individual’s experiential need \(Nexp(a_{i})\), that is, the degree to which the experiential needs are satisfied determines the attitudes toward vaccination initially. But ultimately the behaviour of vaccination also depends on the satisfaction of the individual’s social need \(Nsoc(a_{i})\) and value needs \(Nval(a_{i})\). For example, individuals will also consider the opinions of friends and whether vaccination is in line with their personal values. Actions could only be taken after comprehensive consideration of the satisfaction of all three needs. Once an agent individual evaluates the satisfaction level of all available behavioural alternatives, it chooses the most satisfactory one.
Decision-making
Three types of decisions are taken into account by the model, including vaccination, against vaccination, and hesitation. If getting vaccinated is unambiguously more satisfying than not vaccinating, the individual will vaccinate at this step, otherwise, if the vaccination is unambiguously less satisfying and the opinion is very negative (opinion \(< -0.8\)), the individual will choose anti-vaccination. However, if these two alternatives are similarly satisfactory, the individual will be hesitant and postpone the decision.
Dissonance reduction
No matter what decisions people make, they tend to take more effort to reduce the cognitive dissonance that comes with the decision.
Signal and persuade others. One of the actions that require the least effort to reduce the dissonance is to actively signal satisfaction with the decision to the outside (or inform others about the dissatisfaction of not taking similar behaviour), which can be implemented in the model by convincing a friend to modify their fears with a certain probability (10%, for instance).
Information selection. Here we consider the dissonance reduction strategies triggered by confirmation bias, that is, whether individuals finally get vaccinated or not, they will try to find more supportive information to confirm their decision. A strategy is to alter the current network to avoid discussion with those friends who act differently and interact with others who have similar decisions. In the model, for the vaccinated individuals, if the proportion of vaccinated agents around them is less than 50%, an existing link is randomly disconnected and a link is established with one of the other vaccinated agents; the same is true for anti-vaccination agents.
Agents enter the next step in an updated state.
Design concepts
Basic principles: the basic principle of this model is the idea that it was designed to illustrate that opinion polarisation is related to fears and biased information processing. Whether or not to get a COVID-19 vaccination should be considered by each agent according to the overall satisfaction of experiential needs, social needs, and values (Antosz et al. 2019). To make the final decision with the highest satisfaction and keep looking for more supporting information to confirm this decision is critical for outcomes.
Emergence: the key outcomes of the model are opinion polarisation and vaccination rate, especially, what the peaks are in these outcomes and how quickly to reach the peak. Satisfaction levels for different groups are also exported to better understand vaccination rates and opinion polarisation. These outcomes emerge from how much fear of vaccines and fear of disease agents have and how they deal with risk information from authorities and friends.
Heterogeneity: both authorities and susceptible individuals are heterogeneous, and their social networks and opinions are dynamic. In particular, the cognitive dissonance reduction actions cause agents to keep modifying their state valuables.
Adaptation: the main adaptive mechanism of individuals is from their confirmation bias: deciding whether to get vaccinated and if so, signalling ego feelings to friends or contact more with others that make the same decisions. And accordingly, individuals’ fears and satisfaction will update adaptively.
Objectives: agents decide whether to get vaccinated by an objective measure that represents the overall satisfaction of three needs. Polarisation can be seen from the time evolution plot of opinions. Similarly, the objective measure used by the model to calculate the vaccination rate is the percentage of agents that have been vaccinated.
Learning: in each step, an agent chooses an authority or friend to interact with. This is an adaptive behaviour involving learning. After each step, agents update their fear of vaccination and fear of disease, which adjusts the importance of connections with authorities and friends accordingly. For example, if the fear of vaccination is much higher than the fear of disease at the current time step, then the agent is more likely to trust those authorities who declare the vaccines are dangerous and interact more often with similar friends in the next step.
Interaction: The asymmetric interaction between individuals and authorities is based on the similarity principle. These interactions will not only affect individuals’ attitudes towards vaccination, but also the strength of connections between agents.
Stochasticity: two ways of stochasticity are implemented in the model. First, the model is initialised in such a way that the level of fear of vaccine and fear of disease, the network structure, and the number of agents. Second, interaction happens mainly based on the similarity effect but it is possible to select an agent randomly. The purpose of stochasticity is to avoid the effect of the fixed start of a simulation on results and to increase the variety of interactions.
Observation: summary statistics on vaccination rate and opinion distribution are recorded with time via plots on the interface to show the dynamic evolution processes.
Details
Initialisation
The initial state of the model at the time \(t = 0\) is a virtual world composed of two kinds of entities, individuals and authorities, with random locations by default. Each individual’s state variables, such as fear of disease, fear of vaccine, and availability heuristic, are initialised by drawing randomly from [0, 1]. The individual’s opinion is initialised based on the existing fears, which represents the attitude towards the vaccination. Each individual creates five links to other individuals who are closer to them, representing their friends. At the same time, individuals are also linked to all authorities, indicating that everyone can receive information from authorities on social media. The number of individuals, authorities, and friends can be adjusted to serve different research purposes.
In order to examine the effects of different mechanisms, we implement different initialisation conditions for some parameters in different scenarios. Scenario 1 explores how disease severity may influence the vaccination rate and opinion polarisation by adjusting the value of \(\beta\). In this scenario, we focus on social influence among friend networks without authorities and biases, which is achieved by setting the number of authorities (\(N_{a}\)) to 0 and confirmation bias (\(CmB\)) to \(false\). Scenario 2 intends to examine the impact of confirmation bias by turning on this mechanism. Scenario 3, includes the influence of authorities in two ways: varying the value of \(N_{a}\) and adjusting \(AvH\) to understand their effects on outcomes. For example, when there are two authorities, they will send opposite risk information about the disease and vaccine to individuals. When \(N_{a}\) is more than 2, their opinions follow a uniform distribution, which means more neutral information is introduced. The details are shown in Table 4.
Parameter | Value |
---|---|
\(N\) | 500 |
\(N_{a}\) | 0 |
\(D\) | 5 |
\(M\) | 0.15 |
\(E\) | 0.15 |
\(I_{e}\) | 0.5 |
\(I_{s}\) | 0.5 |
\(I_{v}\) | 0.5 |
\(T\) | 0.5 |
\(vaccination?\) | False |
\(anti-vaccination?\) | False |
\(S_{i}\) | 0.05 |
\(R_{e}\) | 0.1 |
Input data
The model does not use input data to represent time-varying processes.
Sub-models
Fear. Fears are related to the perceived health risk of getting the disease and the perceived risk of side effects of the vaccine, both of which are associated with vaccination behaviour (Brewer et al. 2007; Epstein et al. 2021). We consider two kinds of fears in this model that are related to Epstein’s Agent Zero (Epstein 2014) saying that fear consists of three parts - affective, cognitive, and social components. Inspired by this theory, the affective fear in our model is from the agent’s perceived risk estimation, the cognitive fear is influenced by authorities’ information sharing and the social component depends on the friends’ opinions.
An agent’s perceived fear comes from the initial random distribution and then adjusts the fear level through interactions with authorities and friends.
The probability of contact with authorities is denoted by \(P_{h}\), whereas contact with friends occurred at a probability of \(1 - P_{h}\). \(P_{h}\) is a function of available heuristics, \(AvH\), and total fears, \(F_{v}(a_{i}) + F_{d}(a_{i})\), simply expressed as Equation 1.
\[ P_{h} = f(AvH, (F_{v}(a_{i}) + F_{d}(a_{i}))) = AvH (F_{v}(a_{i}) + F_{d}(a_{i})) / 2\] | \[(1)\] |
Once interactions with authorities occurred in this step, the agents update their current fears following similarity rules, that is, the more similar they are, the more likely they interact with each other. At the same time, individuals choose the latter between the similarity authority and the extreme authority with the max probability of \(AvH\). For example, when interacting with authorities at this step, if \(AvH = 0.2\), the individual will choose extreme authority with a probability less than 0.2 and similar authority with a probability greater than 0.2. We formalise the agent \(i\)’s perceived similarity with an authority \(h_{j}\) as Equation 2:
\[ S_{i,h_{j}} = 1 - (\alpha \lvert F_{v}(a_{i}) - F_{v}(h_{j})\lvert + (1 - \alpha) \lvert F_{d}(a_{i}) - F_{d}(h_{j})\lvert)\] | \[(2)\] |
Where \(S_{i,h_{j}}\) measures the similarity between individuals and authorities and represents the frequency of interaction with authorities. When agents contact authorities, they discuss over the fear of vaccine \(F_{v}\) and fear of disease \(F_{d}\). Here, the chances of discussing \(F_{v}\) depend on the relative importance of \(F_{v}\), denoted by \(\alpha\). When agent \(a_{i}\) interacts with authority \(h_{j}\) with a probability of \(S_{i,h_{j}}\), the agent ai modifies its \(F_{v}\) (or \(F_{d}\)) to move closer to the authority \(h_{j}\), but only if its \(F_{v}\) (or \(F_{d}\)) is sufficiently similar at the current step. Equation 3 represents the updated fear value after interacting with authorities.
\[ F_{v, t+1}(a_{i}) = F_{v,t}(a_{i}) + \mu (F_{v,t}(h_{j}) - F_{v,t}(a_{i})), \; if \; \lvert F_{v,t}(h_{j}) - F_{v,t}(a_{i}) \lvert \leq \epsilon\] | \[(3)\] |
If \(\lvert F_{v,t}(h_{j}) - F_{v,t}(a_{i}) \lvert > \epsilon\), then \(\mu = 0\). The parameter \(\epsilon \in [0,1]\) defines the agent’s tolerance of differences in fears called confidence level (Hegselmann & Krause 2002). The parameter \(\mu \in [0, 1]\) defines the intensity of the change in fears during the interaction.
Agents update their fears through contact with authorities as well as with friends in ego networks. We assume agent \(a_{i}\) selects any one of its friends with equal probability to exchange information and update fears in the same manner as above shown in Equation 4.
\[ F_{v, t+1}(a_{i}) = F_{v,t}(a_{i}) + \mu (F_{v,t}(a_{j}) - F_{v,t}(a_{i})), \; if \; \lvert F_{v,t}(a_{j}) - F_{v,t}(a_{i}) \lvert > \epsilon\] | \[(4)\] |
Opinion
Agent’s opinion is formed by two kinds of fears after they contact external authorities and agents. Specifically, people who perceive as more likely to be harmed by the disease are more likely to be vaccinated (Brewer et al. 2017; Tannenbaum et al. 2015), which is denoted by Equation 5.
\[ O(a_{i}) = \frac{(\beta F_d(a_i) - (1 - \beta) F_v(a_i))}{(\beta F_d(a_i) + (1 - \beta) F_v(a_i))}\] | \[(5)\] |
Where \(O(a_{i}) \in [-1, 1]\) indicates the opinion towards the vaccination, which is positively affected by fear of disease \(F_d(a_i)\), and negatively affected by fear of vaccine \(F_v(a_i)\). Here \(\beta\) represents the perceived severity of the disease, meaning that the more severe the disease, the more positive attitudes toward vaccination. The positive or negative sign of opinions represents either the agent tends to support or oppose vaccination, while the absolute value of opinions indicates the strength of attitudes. Initially, since two types of fears are randomly distributed among all agents, opinions are also randomly distributed. The basic interaction principle for the above model is that updating these two fears of connecting agents as well as the relative importance of fears would also produce opinion dynamics and a dynamically changing network, which in turn affects the frequency of interaction.
Overall satisfaction
Experiential satisfaction refers to comfort and costs related to physical experience. When agents are fearful, their experiential satisfaction decreases. In the model, if an agent chooses to vaccinate, its satisfaction of experiential need \(VN_{exp}(a_i)\) is the normal distribution function of fear-driven opinion in Equation 6:
\[ VN_{exp}(a_i) \sim N(O(a_{i}), \sigma^{2})\] | \[(6)\] |
If the agent chooses not to be vaccinated at the current step, the satisfaction of experiential need \(UVN_{exp}(a_{i})\) is the normal distribution function of (\(-\) opinion) in Equation 7:
\[ UVN_{exp}(a_i) \sim N(-O(a_{i}), \sigma^{2})\] | \[(7)\] |
The agent’s value is set initially so that the percentage of the population, \(R_{e}\), is reluctant to be vaccinated for multiple reasons, including religiosity, private experience, or natural factors such as gender, age, and ethnicity (Troiano & Nardi 2021). So, the satisfaction of value needs to vaccinate \(VN_{val}(a_{i})\) is the normal distribution function of \(R_{e}\) in Equation 8:
\[ VN_{val}(a_{i}) \sim N(R_{e}, \sigma^{2})\] | \[(8)\] |
The satisfaction of value need for not being vaccinated \(UVN_{val}(a_{i})\) is the normal distribution function of \(- R_{e}\) in Equation 9:
\[ UVN_{val}(a_{i}) \sim N(-R_{e}, \sigma^{2})\] | \[(9)\] |
The satisfaction of social need refers to belongingness, relatedness (to feel close and accepted with important others), and social safety. The social norm plays an important role in the model, the more friends in your network are vaccinated, the stronger the norm will be to get vaccinated yourself. Thus, the social satisfaction to vaccinate \(VN_{soc}(a_{i})\) is expressed by the comparative proportion of friends (\(v\)) in the network being vaccinated in Equation 10:
\[ VN_{soc}(a_{i}) \sim N(v, \sigma^{2})\] | \[(10)\] |
The unvaccinated social satisfaction \(UVN_{soc}(a_{i})\) is expressed by the proportion of friends (\(uv\)) in the network not being vaccinated in Equation 11:
\[ UVN_{soc}(a_{i}) \sim N(uv, \sigma^{2})\] | \[(11)\] |
The overall satisfaction from a behavioural alternative is an additive function of the extent of need satisfaction multiplied by the importance of a respective group of needs, shown in Equations 12 and 13:
\[ S_v(a_i) = \frac{(I_e VN_{exp}(a_i) + I_v VN_{val}(a_i) + I_s VN_{soc}(a_i))} {(I_e + I_s + I_v)}\] | \[(12)\] |
\[ S_uv(a_i) = \frac{(I_e UVN_{exp}(a_i) + I_v UVN_{val}(a_i) + I_s UVN_{soc}(a_i))} {(I_e + I_s + I_v)}\] | \[(13)\] |
Decisions
Based on the overall satisfaction of vaccination, individuals will choose the behaviour options that can better satisfy them. There are three behaviour alternatives (\(B(a_{i})\)) in the model: vaccination, anti-vaccination, and hesitation, which are determined by Equation 14.
\[ B(a_{i})= \begin{cases} vaccination \quad if \quad S_v(a_i) - S_uv(a_i) > T,\\ anti-vaccination \quad if \quad S_uv(a_i) - S_v(a_i) > T \quad \& \quad O(a_i) < - 0.8\\ hesitation \quad else \end{cases}\] | \[(14)\] |
where \(B(a_{i})\) is the behaviour alternative of agent \(i\), \(T\) is the decision-making threshold and \(S(a_i)\) is the overall satisfaction for the specific behaviour.
If \(S_v(a_i) - S_uv(a_i) > T\), agent ai chooses to get vaccinated, and some personal attributes change accordingly. The colour of the agent turns green, and the fear of vaccine \(F_v(a_i)\) is reduced. Since there is a certain incidence of side effects, \(S_i\), fear of vaccine \(F_v(a_i)\) rises dramatically once side effects occur for an agent and the colour changes into white. One expectation here is that the higher the vaccination rate, the higher the number of people with side effects.
If \(S_uv(a_i) - S_v(a_i) > T\) and \(opinion < -0.8\), agent ai chooses to be anti-vaccinated. We set the decision of agents who are not vaccinated and have an extreme opinion as anti-vaccination. Their colour turns red.
If \(\lvert S_v(a_i) - S_uv(a_i)\lvert \leq T\) or \(S_uv(a_i) - S_v(a_i) > T\) and \(opinion > -0.8\), agent ai won’t decide at this step and will go directly to the next step. When the satisfaction and dissatisfaction of a decision are similar, the threshold of action \(T\) is used here to measure this similarity, and there is no advantage in taking or not taking a vaccine, so the agent can only choose to hesitate with no state variables to change.
Appendix 2: Supplementary Experimental Results
Supplementary Scenario 1: The effect of disease severity
More comprehensively, Figure 16 confirms the positive impact of \(\beta\) on the vaccination rate. We found that when \(\beta\) is less than 0.5, the vaccination rate rises at a slow pace. However, when \(\beta\) is over 0.5, the vaccination rate increases fast to a high level.
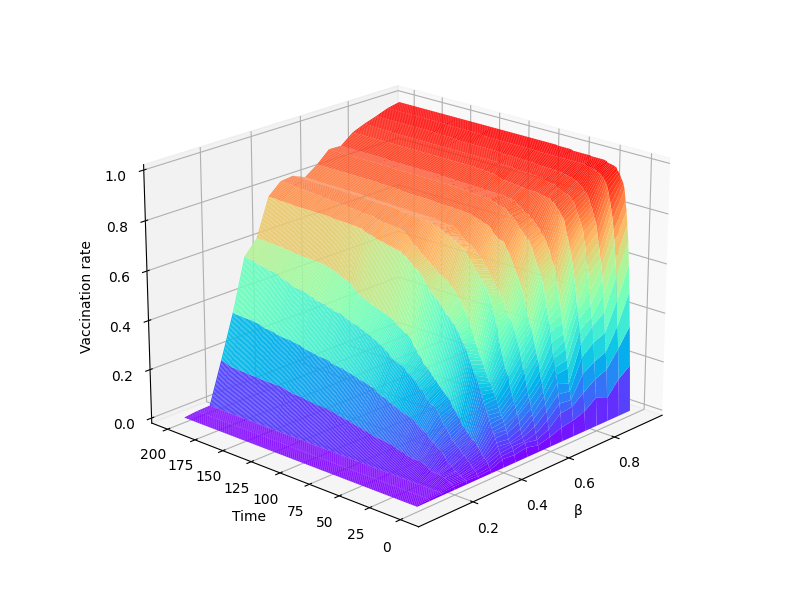
Supplementary Scenario 2: The effect of confirmation bias
Figure 17 shows that with confirmation bias, opinions are more highly polarised in the end than without confirmation bias. With confirmation bias, the frequency of median opinions has decreased significantly over time, and the extreme opinions on both sides have increased. By contrast, the distribution of opinions is more diverse without the confirmation bias mechanism.
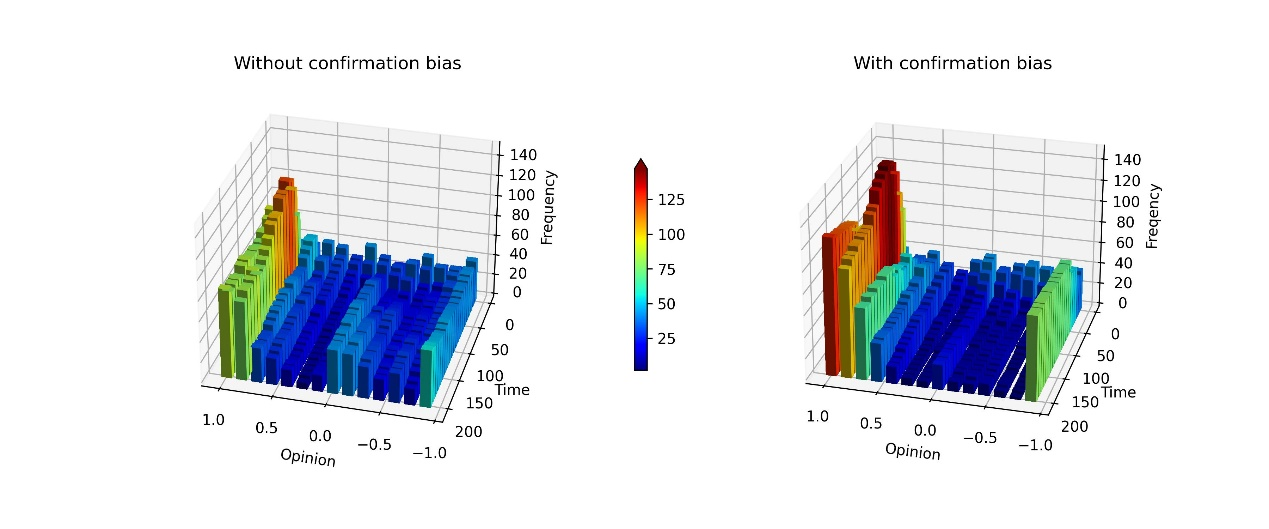
Supplementary Scenario 3: The effect of authority
Here is an example, when \(N_{a} = 5\), to show the effect of \(AvH\). Figure 18 illustrates the evolutionary process of \(AvH\) promoting opinion polarisation. At first, opinions are distributed at random. However, as time passes, a higher \(AvH\) causes more polarisation of opinion.
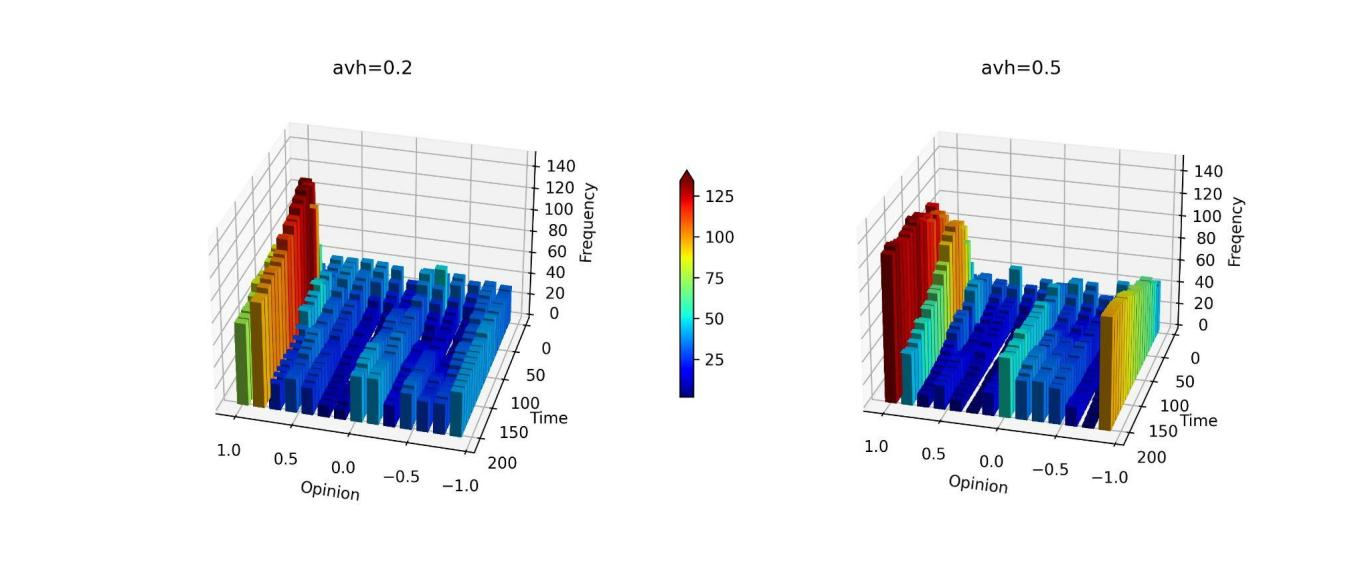
Supplementary experiment: The effect of the number of agents
Figure 19 depicts that when the number of agents is large (\(N = 1000\)), we can still conclude that confirmation bias contributes to opinion polarisation. This shows that increasing the number of individuals in this model has no substantial effect on the main results, therefore, we can say that \(N = 500\) is adequate for simulations of large populations.
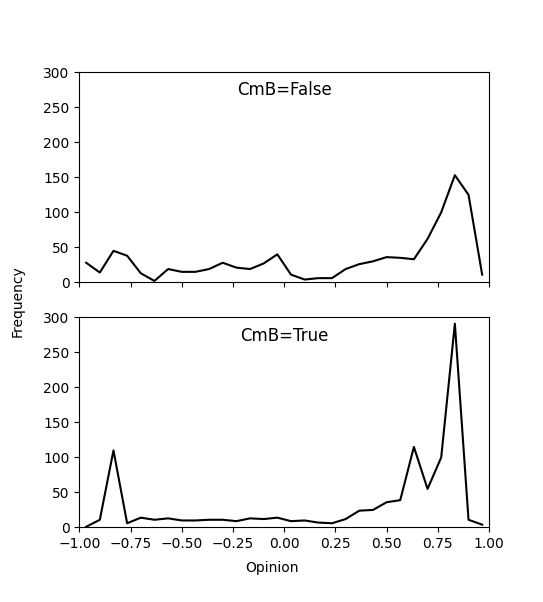
References
AGAMBEN, G. (1998). Homo Sacer: Sovereign Power and Bare Life. Stanford, CA: Stanford University Press.
ANTOSZ, P., Jager, W., Polhill, G., Salt, D., Alonso-Betanzos, A., Sanchez-Marono, N., Guijarro-Berdinas, B., & Rodríguez, A. (2019). Simulation model implementing different relevant layers of social innovation, human choice behaviour and habitual structures - Report describing the theoretical principles of the model and justification and clarification of assumptions used. SMARTEES Deliverable 7.2. Available at: https://local-social-innovation.eu/fileadmin/user_upload/Deliverables/SMARTEES-D7.2_Simulation_model_DR1.pdf
AXELROD, R. (1997). The dissemination of culture: A model with local convergence and global polarization. Journal of Conflict Resolution, 41(2), 203–226. [doi:10.1177/0022002797041002001]
BERNACER, J., García-Manglano, J., Camina, E., & Güell, F. (2021). Polarization of beliefs as a consequence of the COVID-19 pandemic: The case of Spain. PLoS One, 16(7), e0254511. [doi:10.1371/journal.pone.0254511]
BIGO, D., Guild, E., & Kuskonmaz, E. M. (2021). Obedience in times of COVID-19 pandemics: A renewed governmentality of unease? Global Discourse, 11(3), 471–489. [doi:10.1332/204378921x16158113910675]
BISH, A., Yardley, L., Nicoll, A., & Michie, S. (2011). Factors associated with uptake of vaccination against pandemic influenza: A systematic review. Vaccine, 29(38), 264–410. [doi:10.1016/j.vaccine.2011.06.107]
BLUMENTHAL-BARBY, J. S., & Krieger, H. (2015). Cognitive biases and heuristics in medical decision making: A critical review using a systematic search strategy. Medical Decision Making, 35(4), 539–557. [doi:10.1177/0272989x14547740]
BREWER, N. T., Chapman, G. B., Gibbons, F. X., Gerrard, M., McCaul, K. D., & Weinstein, N. D. (2007). Meta-analysis of the relationship between risk perception and health behavior: The example of vaccination. Health Psychology, 26(2), 136. [doi:10.1037/0278-6133.26.2.136]
BREWER, N. T., Chapman, G. B., Rothman, A. J., Leask, J., & Kempe, A. (2017). Increasing vaccination: Putting psychological science into action. Psychological Science in the Public Interest, 18(3), 149–207. [doi:10.1177/1529100618760521]
BREZIS, R. S., Galili, T., Wong, T., & Piggot, J. I. (2014). Impaired social processing in autism and its reflections in memory: A deeper view of encoding and retrieval processes. Journal of Autism and Developmental Disorders, 44(5), 1183–1192. [doi:10.1007/s10803-013-1980-y]
CLARKE, J. N. (2011). Magazine portrayal of attention deficit/hyperactivity disorder (ADD/ADHD): A post-modern epidemic in a post-trust society. Health, Risk & Society, 13(7–8), 621–636. [doi:10.1080/13698575.2011.624178]
DIGNUM, F. (2021). Social Simulation for a Crisis. Berlin Heidelberg: Springer.
EPSTEIN, J. M. (2014). Agent_Zero: Toward Neurocognitive Foundations for Generative Social Science. Princeton, NJ: Princeton University Press.
EPSTEIN, J. M., Hatna, E., & Crodelle, J. (2021). Triple contagion: A two-fears epidemic model. Journal of the Royal Society Interface, 18(181), 20210186. [doi:10.1098/rsif.2021.0186]
EPSTEIN, J. M., Parker, J., Cummings, D., & Hammond, R. A. (2008). Coupled contagion dynamics of fear and disease: Mathematical and computational explorations. PLoS One, 3(12), e3955. [doi:10.1371/journal.pone.0003955]
ESTEBAN, J. M., & Ray, D. (1994). On the measurement of polarization. Econometrica, 62(4), 819–851. [doi:10.2307/2951734]
FESTINGER, L. (1954). A theory of social comparison processes. Human Relations, 7(2), 117–140. [doi:10.1177/001872675400700202]
GALLUP. (2022). U. S. Adults’ Estimates of COVID-19 Hospitalization Risk. Available at: https://news.gallup.com/opinion/gallup/354938/adults-estimates-covid-hospitalization-risk.aspx
GESTEFELD, M., Lorenz, J., Henschel, N. T., & Boehnke, K. (2022). Decomposing attitude distributions to characterize attitude polarization in Europe. SN Social Sciences, 2(7), 1–35. [doi:10.1007/s43545-022-00342-7]
GRIMM, V., Berger, U., Bastiansen, F., Eliassen, S., Ginot, V., Giske, J., Goss-Custard, J., Grand, T., Heinz, S. K., Huse, G., Huth, A., Jepsen, J. U., JNørgensen, C., Mooij, W. M., Müller, B., Pe’er, G., Piou, C., Railsback, S. F., Robbins, A. M., … DeAngelis, D. L. (2006). A standard protocol for describing individual-based and agent-based models. Ecological Modelling, 198(1), 115–126. [doi:10.1016/j.ecolmodel.2006.04.023]
GRIMM, V., Railsback, S. F., Vincenot, C. E., Berger, U., Gallagher, C., DeAngelis, D. L., Edmonds, B., Ge, J., Giske, J., Groeneveld, J., Johnston, A. S. A., Milles, A., Nabe-Nielsen, J., Polhill, J. G., Radchuk, V., Rohwäder, M. S., Stillman, R. A., Thiele, J. C., & Ayllón, D. (2020). The ODD protocol for describing agent-based and other simulation models: A second update to improve clarity, replication, and structural realism. Journal of Artificial Societies and Social Simulation, 23(2), 7. [doi:10.18564/jasss.4259]
HARMON-JONES, E., & HARMON-JONES, C. (2018). Toward an increased understanding of dissonance processes: A response to the target article by Kruglanski et al. Psychological Inquiry, 29(2), 74–81. [doi:10.1080/1047840x.2018.1480691]
HEGSELMANN, R., & Krause, U. (2002). Opinion dynamics and bounded confidence: Models, analysis and simulation. Journal of Artificial Societies and Social Simulation, 5(3), 2.
HOFSTEDE, G. (1980). Culture and organizations. International Studies of Management & Organization, 10(4), 15–41. [doi:10.1080/00208825.1980.11656300]
HOFSTEDE, G. J., Jonker, C. M., & Verwaart, T. (2012). Cultural differentiation of negotiating agents. Group Decision and Negotiation, 21, 79–98. [doi:10.1007/s10726-010-9190-x]
JAGER, W. (2021). Using agent-based modelling to explore behavioural dynamics affecting our climate. Current Opinion in Psychology, 42, 133–139. [doi:10.1016/j.copsyc.2021.06.024]
JØRGENSEN, F., Bor, A., & Petersen, M. B. (2021). Compliance without fear: Individual‐level protective behaviour during the first wave of the COVID‐19 pandemic. British Journal of Health Psychology, 26(2), 679–696.
KAHAN, D. M. (2015). The politically motivated reasoning paradigm. Emerging Trends in Social & Behavioral Sciences, Forthcoming. [doi:10.1002/9781118900772.etrds0417]
KATZ, E. (1957). The two-step flow of communication: An up-to-date report on a hypothesis. Public Opinion Quarterly, 21(1), 61–78. [doi:10.1086/266687]
LADAPO, J. A., Rothwell, J. T., & Ramirez, C. M. (2022). Misperceptions of COVID-19 illness risk and preferences for business and school closures in the united states. Preventive Medicine Reports, 27, 10178. [doi:10.1016/j.pmedr.2022.101780]
LUPTON, D. (2013). Risk and emotion: Towards an alternative theoretical perspective. Health, Risk & Society, 15(8), 634–647. [doi:10.1080/13698575.2013.848847]
LUZ, P. M., Nadanovsky, P., & Leask, J. (2020). How heuristics and cognitive biases affect vaccination decisions. Cadernos De Saúde Pública, 36. [doi:10.1590/0102-311x00136620]
MACDONALD, B. (2021). Attitudes to COVID-19 vaccinations. Available at: https://ourworldindata.org/attitudes-to-covid-vaccinations
MCSTAY, A. (2018). Emotional AI: The Rise of Empathic Media. Thousands Oaks, CA: Sage. [doi:10.4135/9781526451293]
MEPPELINK, C. S., Smit, E. G., Fransen, M. L., & Diviani, N. (2019). "I was right about vaccination": Confirmation bias and health literacy in online health information seeking. Journal of Health Communication, 24(2), 129–140. [doi:10.1080/10810730.2019.1583701]
NICKERSON, R. S. (1998). Confirmation bias: A ubiquitous phenomenon in many guises. Review of General Psychology, 2(2), 175–220. [doi:10.1037/1089-2680.2.2.175]
QUEKEL, S. (2021). Sterkste man-finalist overlijdt aan corona, ook zoon (27) in coma: “Niet te bevatten”. Algemeen Dagblad, 18(2023), 1–10.
RABIN, M., & Schrag, J. L. (1999). First impressions matter: A model of confirmatory bias. The Quarterly Journal of Economics, 114(1), 37–82. [doi:10.1162/003355399555945]
RIVAS, M. N., Ebinger, J. E., Wu, M., Sun, N., Braun, J., Sobhani, K., Van Eyk, J. E., Cheng, S., & Arditi, M. (2021). BCG vaccination history associates with decreased SARS-CoV-2 seroprevalence across a diverse cohort of health care worker. The Journal of Clinical Investigation, 131(2), e145157. [doi:10.1172/jci145157]
SCHLÜTER, M., Baeza, A., Dressler, G., Frank, K., Groeneveld, J., Jager, W., Janssen, M., McAllister, R., Müller, B., Orach, K., Schwarz, N., & Wijermans, N. (2017). A framework for mapping and comparing behavioural theories in models of social-ecological systems. Ecological Economics, 131, 21–35.
SHIBANAI, Y., Yasuno, S., & Ishiguro, I. (2001). Effects of global information feedback on diversity: Extensions to Axelrod’s adaptive culture model. Journal of Conflict Resolution, 45(1), 80–96. [doi:10.1177/0022002701045001004]
SOBKOWICZ, P. (2018). Opinion dynamics model based on cognitive biases of complex agents. Journal of Artificial Societies and Social Simulation, 21(4), 8. [doi:10.18564/jasss.3867]
STJERNSWÄRD, S., & Glasdam, S. (2021). Solidarity and polarisation regarding COVID-19 and related risks - A thematic analysis of comments from an international survey. Social Sciences & Humanities Open, 4(1), 100211.
TANNENBAUM, M. B., Hepler, J., Zimmerman, R. S., Saul, L., Jacobs, S., Wilson, K., & Albarracín, D. (2015). Appealing to fear: A meta-analysis of fear appeal effectiveness and theories. Psychological Bulletin, 141(6), 1178.
THE Telegraph. (2021). Use of fear to control behaviour in Covid crisis was ‘totalitarian’, admit scientists. The Telegraph 14th May 2021. Available at: https://www.telegraph.co.uk/news/2021/05/14/scientists-admit-totalitarian-use-fear-controlbehaviour-covid/
TRIMBLE, M. (2021). Propaganda. Ulster Medical Journal, 90(3), 133–134.
TROIANO, G., & Nardi, A. (2021). Vaccine hesitancy in the era of COVID-19. Public Health, 194, 245–251. [doi:10.1016/j.puhe.2021.02.025]
TVERSKY, A., & Kahneman, D. (1974). Judgment under uncertainty: Heuristics and biases. Science, 185(4157), 1124–1131. [doi:10.1126/science.185.4157.1124]
VAN Eck, P. S., Jager, W., & Leeflang, P. S. (2011). Opinion leaders’ role in innovation diffusion: A simulation study. Journal of Product Innovation Management, 28(2), 187–203. [doi:10.1111/j.1540-5885.2011.00791.x]
VOGRIN, M., Wood, G., & Schmickl, T. (2023). Confirmation bias as a mechanism to focus attention enhances signal detection. Journal of Artificial Societies and Social Simulation, 26(1), 2. [doi:10.18564/jasss.4954]
WASON, P. C. (1960). On the failure to eliminate hypotheses in a conceptual task. Quarterly Journal of Experimental Psychology, 12(3), 129–140. [doi:10.1080/17470216008416717]
WILENSKY, U. (1999). NetLogo. Center for Connected Learning and Computer-Based Modeling, Northwestern University
WONG, L. H., Pattison, P., & Robins, G. (2006). A spatial model for social networks. Physica A: Statistical Mechanics and Its Applications, 360(1), 99–120. [doi:10.1016/j.physa.2005.04.029]
YOUNG, M. E., King, N., Harper, S., & Humphreys, K. R. (2013). The influence of popular media on perceptions of personal and population risk in possible disease outbreaks. Health, Risk & Society, 15(1), 103–114. [doi:10.1080/13698575.2012.748884]