The Dynamical Relation Between Individual Needs and Group Performance: A Simulation of the Self-Organising Task Allocation Process

, ,
and
aDalian University of Technology, China; bHanze University of Applied Science, Netherlands; cUniversity of Groningen, Netherlands
Journal of Artificial
Societies and Social Simulation 24 (4) 9
<https://www.jasss.org/24/4/9.html>
DOI: 10.18564/jasss.4715
Received: 13-Apr-2021 Accepted: 04-Oct-2021 Published: 31-Oct-2021
Abstract
Team performance can be considered a macro-level outcome that depends on three sets of micro-level factors: individual workers contributing to the task, team composition, and task characteristics. For a number of reasons, the complex dynamics between individuals in the task allocation process are difficult to systematically explore in traditional experimental settings: the motivational dynamics, the complex dynamics of task allocation processes, and the lack of experimental control over team composition imply an ABM-approach being more feasible. For this reason, we propose an updated version of the WORKMATE model that has been developed to explore the dynamics of team performance. In doing so, we added Deci and Ryan’s SDT theory, stating that people are motivated by three psychological needs, competence, autonomy, and belongingness. This paper is aimed at explaining the architecture of the model, and some first simulation runs as proof of concept. The experimental results show that: 1) an appropriate motivation threshold will help the team have the lowest performance time. 2) the time needed for the task allocation process is related to the importance of different motivations. 3) highly satisfied teams are more likely composed of members valuing autonomy.Introduction
Within the area of organisational psychology, a fundamental question is how individual behaviour mutually relates to group and organisational outcomes. When focusing on this question, based on Aristotle’s notion that the whole is greater than the sum of the parts, we might conclude that group outcomes are a function of both individual actions and social interaction (Cherrington 1989; Furnham 2012; Kozlowski 2018; Kozlowski & Bell 2012). Whilst the relationship between individual, group, and organisational level has been a subject of various studies (Kozlowski & Klein 2000; Ployhart & Moliterno 2011), the underlying dynamical processes in teams, remain less accessible for both experimental and field research.
The mainstream approach, which deals with this kind of multi-level problem in a highly advanced statistical way (Dong et al. 2017; Gong et al. 2013; Hülsheger et al. 2009) contributed largely to the individual factors affecting group performance, however, because of its statistical nature, it cannot capture the dynamics that emerge from the interaction processes between individuals. These emergent phenomena are typical to be explored using Agent-Based Modelling (ABM) (Helbing 2012; Wang et al. 2018; Zoethout et al. 2010). ABM is specifically equipped to study processes among interrelated agents, yet offering a potentially fruitful approach to understand the underlying dynamics of social behaviour as related to group performance. However, although the ABM-approach brought up some psychologically plausible models for team processes and performance (Rendón Rozo et al. 2019; Wang et al. 2017; Zoethout et al. 2008), a simulation model that describes the social dynamics of specific motivational components affecting the task allocation process has not been developed yet.
In studying the group dynamics in work teams, we develop a model that formalises psychological theory into an ABM creating a framework to study the social dynamics that pertain to the mutual relations between the individual and group level of team performance. This framework is based on the WORKMATE-approach (Zoethout et al. 2006), which offers a plain, yet psychologically plausible example of a multi-agent simulation model. This model has been used to describe the mutual relationship between individual and group behaviour by focusing on self-organising processes of task allocation. Although the WORKMATE approach focused on motivational dynamics as well, it made no distinction in various types of motivation affecting the group outcome. For this reason, to this WORKMATE framework, a formalisation of Deci & Ryan’s self-determination theory (SDT) (Gagné & Deci 2005; Ryan & Deci 2000; Ryan & Deci 2017) has been added. The SDT describes three basic human drives: the need for competence, autonomy, and belonging. By implementing the SDT theory into WORKMATE, a framework is created for studying the intertwined relation between individual needs and group performance.
One of the key questions is how the three individual motivations of autonomy, competence, and belonging contribute to team performance. The application of SDT theory to an ABM architecture gives rise to complicated theoretical and modelling issues. Such as, ABM provides a tool to explore micro-macro relations in social systems (Jager 2017), while it is also accompanied by the challenge of how to reasonably use theoretical assumptions to form the rules of agent behaviour. Individual differences and distribution of the three needs have an impact on the collaboration between team members, which will affect productivity. Autonomy and relatedness are affected by prior experiences concerning the interaction with other team members, and an improvement in a specific need does not automatically increase productivity. To integrate psychological theories in an agent-based model, it is essential to figure out those complex relationships mentioned above. In the following theoretical part, we will elaborate more on the complexity of these relationships.
This research is applying SDT theory into an ABM to identify the influence of individual needs on the group dynamics of team performance. Through ABM techniques and methods, we model the psychological and behavioural activities of the team members as agents. This allows for establishing an artificial team to study how the three types of motivation interact with group dynamics through controlled and repeatable experiments. In this way, we want to contribute to the identification of the dynamical processes in teams affecting how successful they are in completing projects.
To elaborate on the research question, this paper follows the consecutive structure. First, we describe the theoretical framework which involves (1) individual components such as motivation and expertise including its dynamics, (2) social components such as mutual influence, especially task allocation processes, and (3) group performance is the most important outcome variable. Next, we propose a formalisation of these components by adding these theories to the existing WORKMATE-framework. Subsequently, we describe a proof-of-concept experiment that we conducted by using this framework. The paper concludes with suggestions for further research and a discussion on applications.
Conceptual Framework
To describe the model that is being used to study the mutual relation between individual, group, and organisational components, we make use of four sets of variables, which can be conceptualised as shown in Figure 1. The main elements of this schematic overview are (1) the input of this system is a project representing a set of tasks, (2) the output of this system is the team performance, which includes performance indicators such as performance time and group satisfaction, (3) the individual workers of which the team is being composed of as well as their behaviour related to task allocation and performance, (4) social interaction of the individual workers involved in the process of task allocation.
By relating these variables, we create a theoretical framework that describes how members organise themselves when allocating and performing a task. This organising process reflects a mutual interaction between individual team members as well as the interaction between the individual and team behaviour. To explain how a team self-organises this process of task allocation, we will elaborate on the different components as described above.
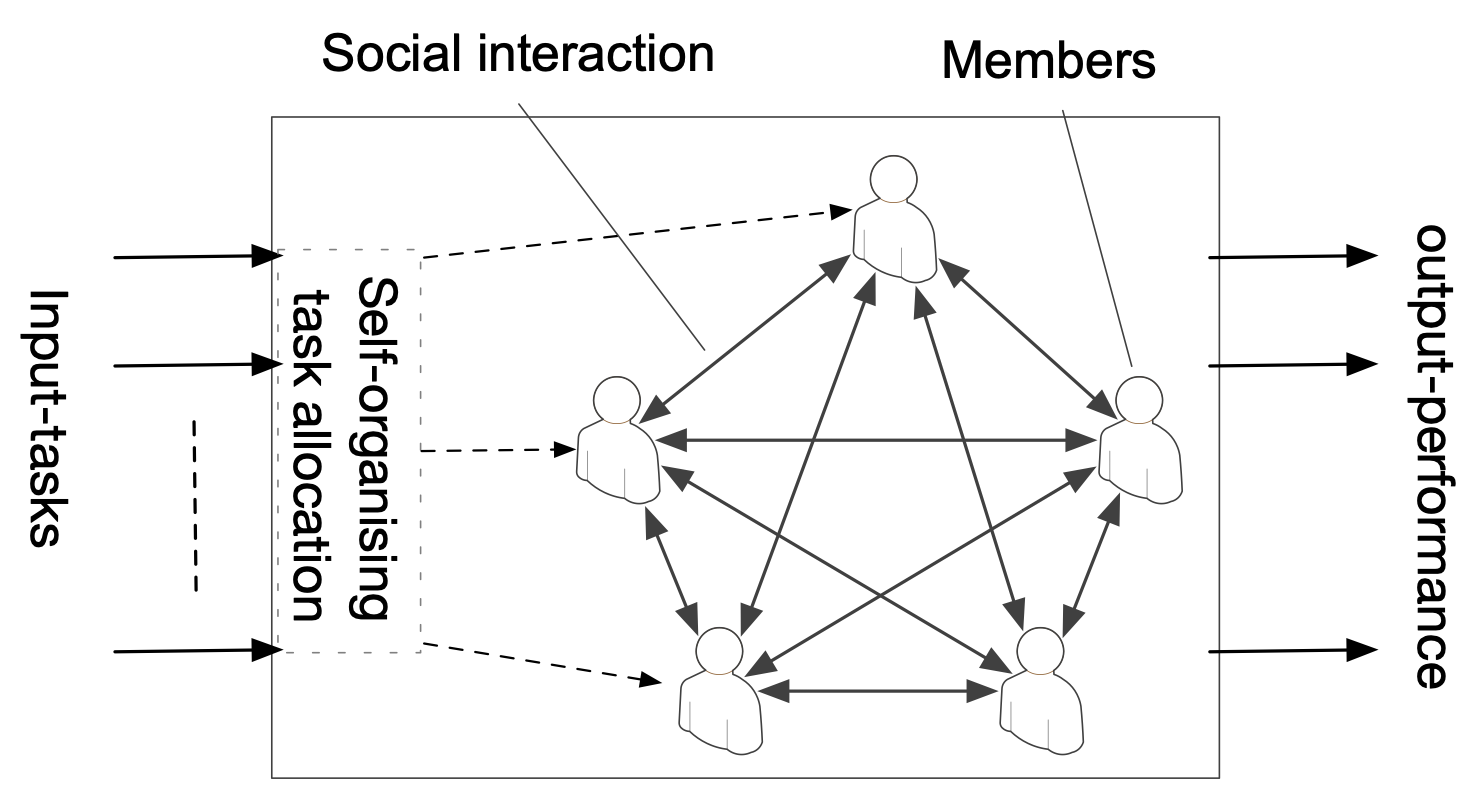
Individual components: Competence and motivation
Our description starts at the moment that a project is being assigned to the team. Every member of the team individually decides whether or not to perform a particular task that a project consists of. According to Wilke & Meertens (1994), the most important individual variables that determine group performance are competence and motivation. On the basis of these variables, each team member individually decides whether or not to perform a particular task (Zoethout et al. 2006, 2010).
Competence refers to one’s ability to complete a task, related to individual knowledge and skills. As an example, in a house building project, team members may differ in their competence, e.g. design, bricklaying, carpentry, electrical installation construction, and the like. Tasks may require different competencies to be performed, and team members may differ concerning the competence they have. In some projects, members perform different tasks that require unique competence, requiring the work of specialist team members. In other projects, the tasks differ less in requiring competence, and team members that are more generalist in terms of their competence can perform the tasks.
Additional to competence, the members’ motivation is also a fundamental determinant of human behaviour (Latham & Pinder 2005), referring to the member’s willingness to perform a task. Organisational research has focused on questions of how team members learn, perform a task, and experience satisfaction when completing a task. Various motivation theories have been used to elaborate on these questions, including goal-setting theory (Locke 2013), expectancy theory (Renko et al. 2012), achievement motivation theory (De Castella et al. 2013; Weiner 1985), and reinforcement theory (Wei & Yazdanifard 2014).
A key theory in this field that is stated as a new impetus to research on human motivation is the Self-Determination Theory (SDT), proposed by Ryan & Deci (2000), this SDT provides a useful conceptual tool for organisational research by addressing the different motivations driving the member to perform a task. SDT identifies three fundamental psychological needs underlying this motivation: competence, autonomy, and belonging (relatedness). Hence, in our model, the individual component of motivation is being described by using these three needs the SDT proposed. Next, we will elaborate on this.
Need for competence
People have a propensity toward feeling competent and having effects on their environment, which shows a person’s capacities and talents to achieve their goals or their ability to solve a problem at hand (Elliot & Dweck 2012). The feeling or expectation that one can successfully perform a task is rightly playing a central role in intentional behaviours. People are often attracted toward tasks for which they can experience competence, and avoid tasks for which they lack competence. The definition of competence as a basic driver of task motivation can be traced back to White’s concept of effectance motivation (White 1959), which was used to connote people’s capacity to interact effectively with their environment. Consistent with White’s conceptualisation of effectance motivation, Ryan & Deci (2000) posit that the need for competence shows people seek and master challenges. The need for competence is satisfied depending on the degree to which people feel and believe that they are effective when performing a task. When a task is perceived as too easy or too difficult to complete, the competence motivation of a person generally is lower (Elliot & Dweck 2012). In terms of Vygotsky’s zone of proximal development, it can be stated that people’s competence motivation is the largest when a new task is just a bit more difficult than their current competence, so learning can take place without the frustration of failure (Chaiklin 2003).
Need for autonomy
In SDT, autonomy is conceptualised as the feeling that a person’s decisions and actions are self-integrated and not being influenced or coerced by external power, which concerns a sense of willingness and volition concerning one’s behaviours (Niemiec & Ryan 2013). Hence autonomy does not mean being independent of others, nor being socially isolated. As demonstrated in SDT, autonomy is theorised as a need, and the sense of autonomy (perceived autonomy) is indeed a very personal experience. The need for autonomy describes the need of individuals to experience self-endorsement and ownership of their actions to be self-regulated in the technical sense of that term (Ryan & Deci 2017).
Individuals differ in their desire for autonomy, some people may have certain types of experiences more than others, and the satisfaction of basic needs will bring different levels of satisfaction to different people (Schüler et al. 2016). The experience of autonomy is subjective. It depends upon the perception of three interrelated components, namely (1) an internal perceived locus of causality (the causal source of actions ranges from internal to external), (2) volition (actions are endorsed by the self), and (3) perceived choice (decision-making flexibility) (Reeve 2014). Conditions that increase the sense of choice or freedom to perform tasks have been found to improve experienced autonomy (Evans & Boucher 2015). Behaviours are self-determined when our preference leads to our decision to perform or not perform a particular task. People are not self-determined when another person, e.g. a manager, takes away their sense of choice by coercing them into performing a particular task. The need for autonomy is satisfied by being offered a choice and the opportunity for self-determination (Legault 2016).
Although the need for autonomy is a personal innate feeling, their sense of autonomy depends on how their individual choices are affected by interactions with other team members coercing them to perform a different task than initially preferred. In the context of collaborating in projects, autonomy refers to the capacity of a team member to control the choices of what to do and what not to do. The need for autonomy will be fulfilled by letting team members perform tasks of their choices. When team members are able to perform tasks in accordance with their selection and preference, their perceived autonomy is high, and accordingly, their need for autonomy is highly satisfied.
Need for belonging
Belonging refers to the human need to be accepted as a member of a group, indicating the extent to which a person feels connected with those around them (Pavey et al. 2011). Regardless of the type of group, individuals incline to be a part of a social collective that is greater than themselves. The need for belonging can be considered as a prerequisite for actual social satisfaction, which strongly depends on how a team collaborated in the past and present (Wilczyńska et al. 2015).
The sense of belonging is addressing a person’s experience of being involved and feeling to be part of a group (Hagerty et al. 1992). Baumeister & Leary (1995) recognised that individuals have a pervading drive to form and maintain group memberships and interpersonal bonds. People satisfy their desire to connect with those around them by forming interpersonal bonds and interacting with them. People have different desires concerning their preferred level of social connectedness (Walton et al. 2012), which can be related to their personality (Lin 2016).
The need for belonging is satisfied when a person feels involved in a larger group and connected with others, hence in understanding team performance, it is relevant to consider the factors and processes that allow for social interaction, as these produce feelings of participation and connectedness that affect the overall motivation of team members. Whereas different theories of motivation exist, in the context of team performance it seems a logical choice to address the motivation of individual team members as a combination of their satisfaction of the need for autonomy, competence, and belonging. In performing tasks, sometimes a trade-off will have to be made between these different motivational needs, acknowledging that team members may differ concerning the importance they attach to these three motivational drivers.
Task components
Tasks generally refer to activities carried out to achieve certain goals in social life, engineering technology, and manufacturing. Much research has focused on how people perform tasks, how tasks are allocated (Chen & Wei 2009) and how teams can be supported in performing tasks more effectively (Chae et al. 2015). Some scholars propose three essential components of tasks, namely products, acts, and information cues (Naylor et al. 2013; Wood 1986). Products refer to the measurable and purposeful results of the performance of a task. Acts and information cues refer to the knowledge, skills, and resources needed by an individual to complete a task.
Task complexity is often used as an explanatory variable in discussing factors affecting task performance. Wood defined three types of task complexity: component complexity, coordinative complexity, and dynamic complexity (Wood 1986). Component complexity refers to the number of distinct acts that need to be executed. As the number of acts associated with a task increases, the demand for knowledge (expertise) and skills to perform the task also increases. Hence tasks can be differentiated based on the expertise and/or skills that are required to perform them (Wood 1986). Coordinative complexity refers to the interdependence between the required activities, and as such it captures the precedence relations between the required acts. Dynamic complexity refers to changes that may happen over time concerning the component and coordinative complexity.
Following the description above, we define a team project as a collection of tasks that need to be completed by the team members. A team project thus can be decomposed into a collection of interdependent tasks that need to be allocated over the team members. Each task requires a certain skill level and an available time slot to be completed by a team member. Following Wood (1986), task interdependence refers to the order in which different tasks need to be completed, and dynamic complexity refers to situations where the skill levels and time required to perform a task change over time.
Social interaction
Initial choice and final allocation
The advantage of teams in working on projects lies in their ability to better integrate and utilise the strength of each member (Kozlowski 2018; Kozlowski & Ilgen 2006). Managers can allocate the tasks over the team members based on their estimation of the members’ capacity to perform the tasks well. However, task allocation can also be self-organised, where no central management is needed to come to a good task allocation. The theoretical framework WORKMATE (Zoethout et al. 2006) is for simulating the self-organising process of allocation. In the course of task allocation, the team members choose the tasks they want to undertake based on their competence and motivation and rely on the interaction with the other members to complete the process of task allocation.
Each team member may choose to do a particular task, and not do other tasks based on its competence and motivation for this task. We formulate this as the initial choice of each member, which represents if he/she wants to perform a particular task or not, it could be "I-Do" (I want to do it) or "Y-Do" (I do not want to do it, I want you to do it).
On the basis of the initial choice, the agents start influencing each other. The influencing process aims to reach a complementary situation in which the agent can actually do the task that it wants while influencing the other agents to do the tasks it doesn’t want to do. This process applies to all tasks and all agents involved in a project. This finally leads to a situation in which all the tasks in a project have been allocated to the agents in a self-organising way, based on their competence and motivation related to each skill. The final situation being reached is called: final allocation.
Connections between agents
Every agent has afferent (incoming) connections from other agents and efferent (outgoing) connections to other agents. The connections between agents are directed and heterogeneous. According to Hebb’s (1949) learning rule, the simultaneous activity of two elements increases the likelihood for the emergence of a new or strengthening an existing connection between those elements, in our case the task of performing agents in a team. When agents work on the same project, the strength of the connection between them will increase, and when agents cannot perform tasks according to their preference due to interaction with other agents, then the connection between this agent and another agent accountable for this change will be weakened accordingly. The connections between agents may vary in strength: if a connection is stronger, the proportion of excitation and inhibition is larger.
As Figure 2 depicts, every pair of agents has two directed connections that are distinguishable from each other, like the connections between agent A and agent B, one from agent A to agent B and one vice versa.
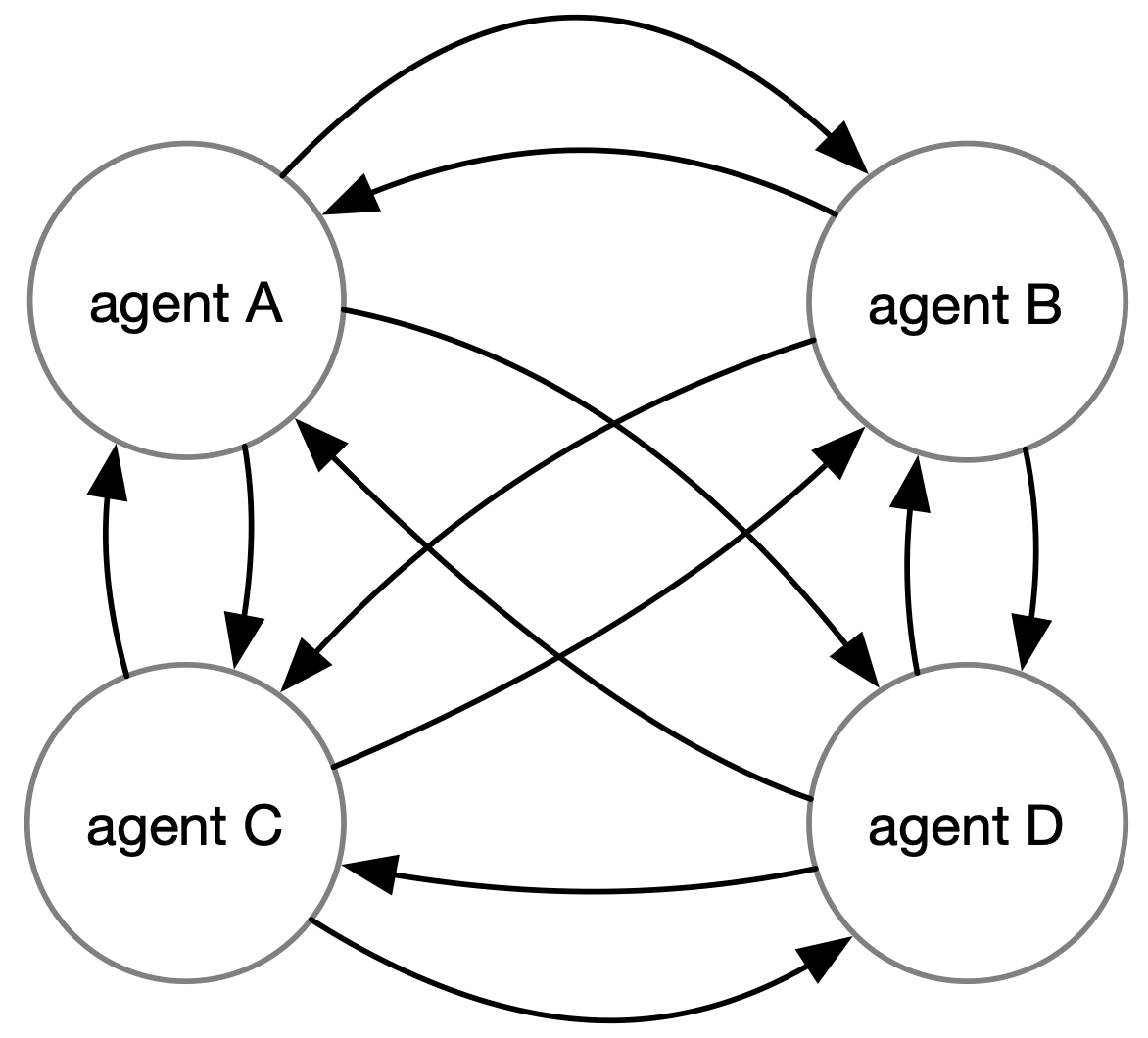
Group performance
Scholars have widely proven that there is a significant relationship between motivation and task performance in many groups, including teachers, students (Inayatullah & Jehangir 2012; Kusurkar et al. 2013), and employees (Zahra et al. 2015). Whether at the organisational, team, or individual level, motivation has been shown to have a significant impact on task performance (Chaudhary et al. 2012).
People’s task performance is determined by what motivates them, and their performance is a product of both ability and motivation (Heavey et al. 2011; Mullins 2007). Ability relates to performance primarily through job knowledge, such that workers having a higher ability tend to perform better because they are better able to use job-relevant knowledge. Motivation relates to task performance by influencing the choices workers to make about whether or not to devote effort towards a certain task (van Iddekinge et al. 2018). The interaction between ability and motivation affects individual task performance, so the relationship between ability and task performance relies on motivation (Mullins 2007). Besides, scholars stated that for a team, in addition to ability and motivation, coordination is a third important factor affecting team performance (Janicik & Bartel 2003). Coordination in teams is considered a key process for team effectiveness, and good coordination is considered to be necessary to ensure that a team functions as a whole in managing a project.
Researchers have converged on a reflection of teams as complex, adaptive, and dynamic systems. Teams exist and perform in a context and they perform across time. Teams and their members constantly adjust over time and contexts through interactions among their members. Coordination of these social interaction processes and dynamics is considered to be an indispensable process that affects team performance (Rico et al. 2008).
Summarised, there are three main elements related to group performance, namely ability, motivation, and coordination. The former two are components of individual team members, and individual expertise represents the specific abilities of a team member. The concept of coordination costs is defined in terms of the time needed to allocate the different tasks over the team members. The following Figure 3 summarises the conceptual model, positioning the psychological components and behavioural processes in the performance of a team.
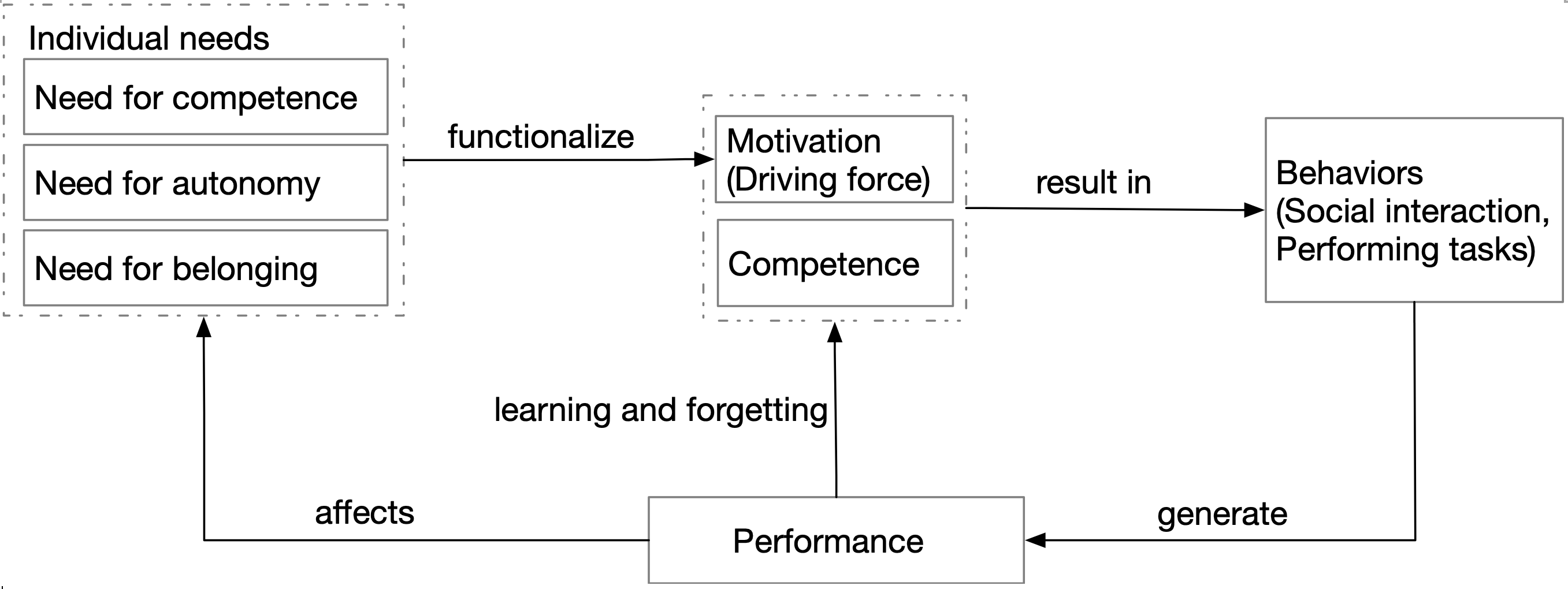
This model will serve as the basic starting point for the development of an Agent-Based Model for simulating a self-organising task allocation process.
The Agent-Based Model Applying the ODD Protocol
The ODD protocol is proposed for describing IBMs (individual-based models) and ABMs (agent-based models) in a complete and comprehensible way. The ODD is designed to ensure the model description is readable and understandable, which was first discussed by Grimm et al. (2006). There are seven elements of the ODD protocol, which can be categorised into three block groups: Overview, Design Concepts, and Details. A complete, detailed model description following the ODD protocol (Grimm et al. 2020) is provided in its supplementary files.
The overall purpose of our model is to explore the dynamical relationship between individual needs and group performance when focusing on self-organising task allocation. Specifically, we attempt to address the question of how three individual needs (need for competence, autonomy, and belonging) affect group performance. The model includes the following five entities: teams and projects, tasks, individuals, connections among individuals. The state variables characterising these entities are listed in Table 1, and the flowchart of the task-performing process is shown in Figure 4.
Entity | State Variable | Description | Possible Value |
---|---|---|---|
Team | Num_Members | The number of individuals or members | Natural number |
Project | Num_Tasks | The number of tasks | Natural number |
Task | ID | The identity number of each task | Natural number |
Task Knowledge | The amount of knowledge required to complete the task | [0,1] | |
Task Duration | The time needed to execute the task | [0,10] | |
Individual | ID | The identity number of each member | Natural number |
Competence | All the knowledge possessed by the individual | [0,1] | |
Motivation | The willingness of individals to perform a task, concerning three needs: need for competence, autonomy, and belonging | [0,1] | |
Learning and forgetting | The ability to adapt and respond to the organisational environment | [0,1] | |
Connection | Connection strength | The influence relationship among individuals | [0,1] |
The fundamental process of the model is the self-organising task allocation process, which is based on the social interaction among individuals (the individual and interactional levels in Figure 4). Firstly, individuals make an initial choice based on their level of competence and motivation, and they have to interact with each other to reach the final allocation when there is no complementary allocation happening until it reaches a complementary situation in which there is only one individual who wants to do a particular task. Individuals will adjust their competence and motivation after performing the tasks allocated to them. When the next project comes, the self-organising allocation and performing process will be repeated, and the individuals involved will constantly adapt according to the coming tasks.
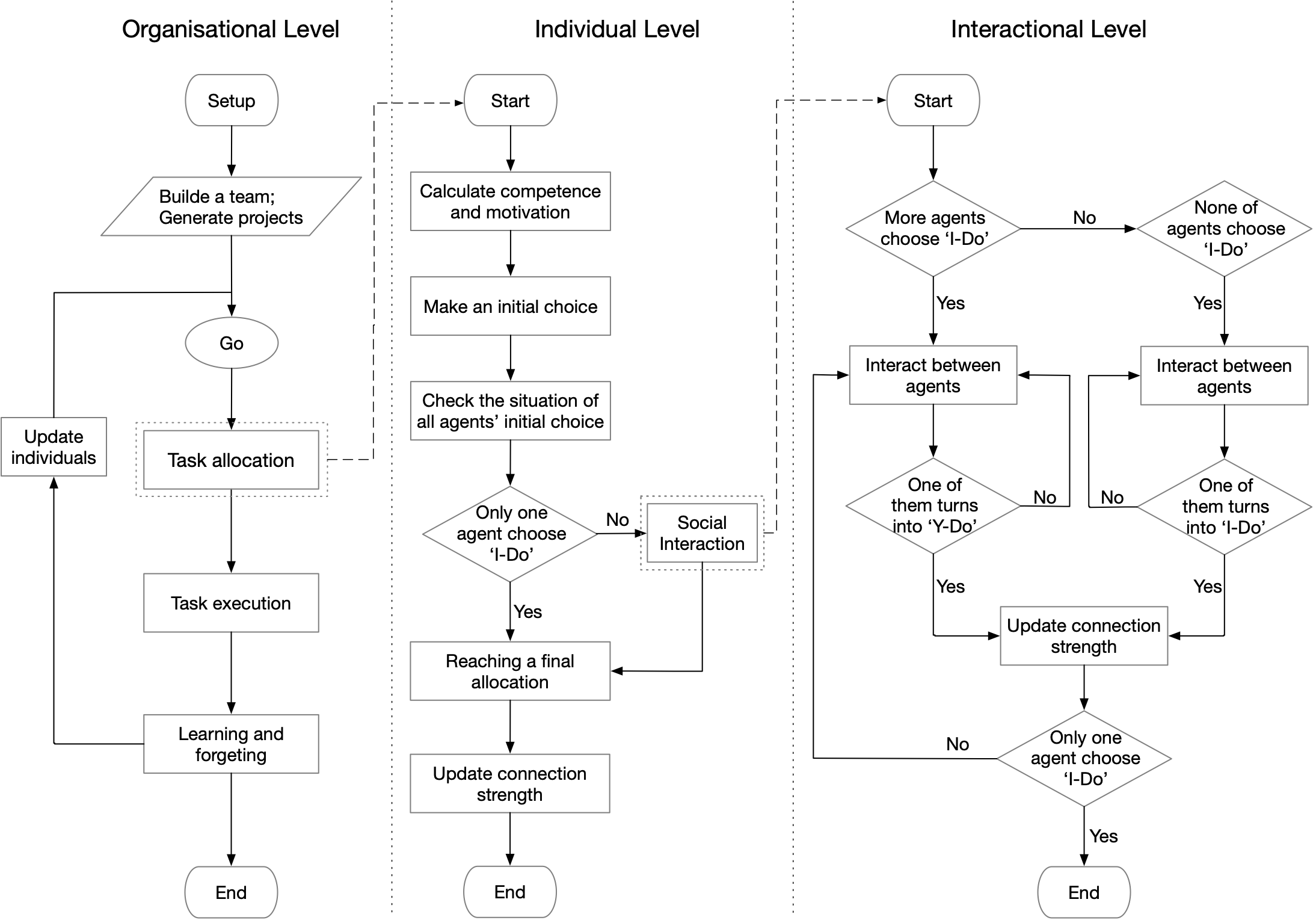
The most important design concepts of the model are adaptation and interaction. The adjustment of motivation is the key adaptive behaviour, it is modelled according to the SDT theory (Ryan & Deci 2017), which addresses three working motivational needs, including (1) the need for competence, which is often considered to be contextual. An agent would be most motivated if the knowledge possessed by this agent is close to the task requirement (Schiepe-Tiska 2013). Hence, an inverted U-shape curve is needed for explaining the relation between the need for competence and individual knowledge concerning task requirements, (2) the need for autonomy, agents want to be autonomous and have individual liking to use a certain skill, expertise, or knowledge, the more agents are capable of doing the tasks they like, the more perceived sense of choice ability, the more satisfied they are with the need for autonomy, and (3) the need for belonging, referring to the need of agents for being a part of a larger whole. The sense of belonging of each individual depends on its feelings of connectedness, in this paper, we will state the individual motivation driven by belonging from social connectivity. Basically, this social connectivity refers to connectivity to the other agents, the larger the connectivity, the more satisfied the need for belonging. Additionally, the interaction happens in the course of self-organising allocation, individuals will interact with each other until they reach a final allocation.
Agent-Based Simulation Results
In the discussion, we will elaborate on the experimental designs we want to explore using the WORKMATE model. In this section, we limit ourselves to demonstrate what team dynamics can be simulated with WORKMATE, and what types of output can be generated for further analysis. As a setting for the proof-of-concept experiment we use the following settings:
- The team consists of three individuals;
- The project is composed of three tasks, and the cycle (repetition) of the project is 40;
- Each member has three types of knowledge.
A complete and detailed set of parameters are described in the supplementary files. All data generated or analysed during this study are included in this article and its supplementary files.
To start the experiment, we followed common sense and educated guessing to select the values of some of the parameters involved in the model. For simplicity, we use the middle value, i.e. 0.5, for \(\lambda\) indicating that competence and motivation are equally important when forming the initial choice of individuals. We set the parameters of the psychological characteristics, namely \(\sigma\), \(\varrho\), \(\beta\), \(\psi_{exc}\), \(\psi_{inh}\) at the value 1, making them equally important. In addition, we assume that the agents’ ability to learn is faster than the rate at which they forget (Zoethout et al. 2010). In this experiment, we set agents’ learning ability \(\gamma=0.3\) and forgetting rate \(\zeta=0.1\).
We conducted a sensitivity analysis to explore how the team behaviours change when varying parameters related to the overall motivation and the three needs, which are the four aforementioned parameters (\(T_{am}\), \(I_c\), \(I_a\), \(I_b\)).
In this paper, we aim to explore the dynamical relationship between three individual needs and group performance. While many experiments can be conducted with our proposed model, as a proof of concept we explore the impact of two critical factors on this dynamical relationship. First, motivation threshold (\(T_{am}\)) is the variable that is related to motivation and directly affects the individuals’ belief whether or not it is willing to undertake a certain task. When an agent’s motivation is higher than this \(T_{am}\), the agent is motivated and willing to choose to do a particular task, and vice versa. The second key factor is the importance of three needs (\(I_c\),\(I_a\),\(I_b\)) affecting the relative importance of the needs. We intend to figure out the impact of the motivation threshold and relative importance of the three individual motivational needs on performance time (\(T_{perf}\)) and satisfaction (\(GS\)).
The effect of motivation threshold on performance time and motivation
To begin with, we analyse the sensitivity to \(T_{am}\), the performance time of team change due to the varying motivation threshold, which showing how critical the members are concerning their motivation to perform the different tasks. Here, the key variable that affects whether a member is critical or not is the motivation threshold, the higher the larger the threshold, the more critical, and vice versa. In Figure 5, we show how the performance time of teams with a high (\(T_{am}=0.7\)), moderate (\(T_{am}=0.4\)), or low motivation (\(T_{am}=0.1\)) evolves over a series of cycles.
In Figure 5, it can be observed that under conditions of moderate motivation the team performs the task the fastest. When the motivation threshold is very high, where agents are hard to motivate, the team performs the slowest in the early cycles. When workers are very critical (high \(T_{am}\)) of being motivated to perform a task, they will be unmotivated to perform the task. If nobody is motivated to do the task, the agents try to convince each other (Y-do) to do the tasks, which requires a lot of coordination time. Hence the higher \(T_{am}\), the more reluctant an agent is to take on the task, and the more \(T_{coor}\) is required to complete a project. When the motivation threshold of the team members is low, the team members are easily motivated to perform a task. If all three team members are motivated to perform the three tasks (I-do), also more negotiation is needed on deciding who is going to do what. Hence the lower \(T_{am}\), the more motivated agents are to do the task, and more time \(T_{coor}\) is required to complete a project.
In the following Figure 6, we show for teams having different motivation-thresholds how the performance time evolves, indicating with colour how much percentage of this time is used for the allocation process. Figure 6 shows the existence of an optimal interval for motivation threshold (\(T_{am}\in[0.3,0.5]\)) leading to the lowest performance time \(T_{perf}\). This interval can be considered as the aforementioned moderate motivation threshold, where the agents do not spend too much time on the allocation process, as indicated with the blueish colour.
When the motivation threshold is low (\(T_{am}\)<0.3) or high (\(T_{am}\)>0.5), all agents are either motivated or unmotivated to perform the task, resulting in a lot of coordination time, as indicated with the greenish colour. The total performance time is thus high. At the optimal interval of motivation threshold \(T_{am}\), the threshold is the closest to the individual motivations of the agent. This implies that it is more likely that one agent is just enough motivated to perform a task (I-do), whilst the other(s) are just not motivated enough, resulting in an easy allocation process. As a consequence, the performance time is low.
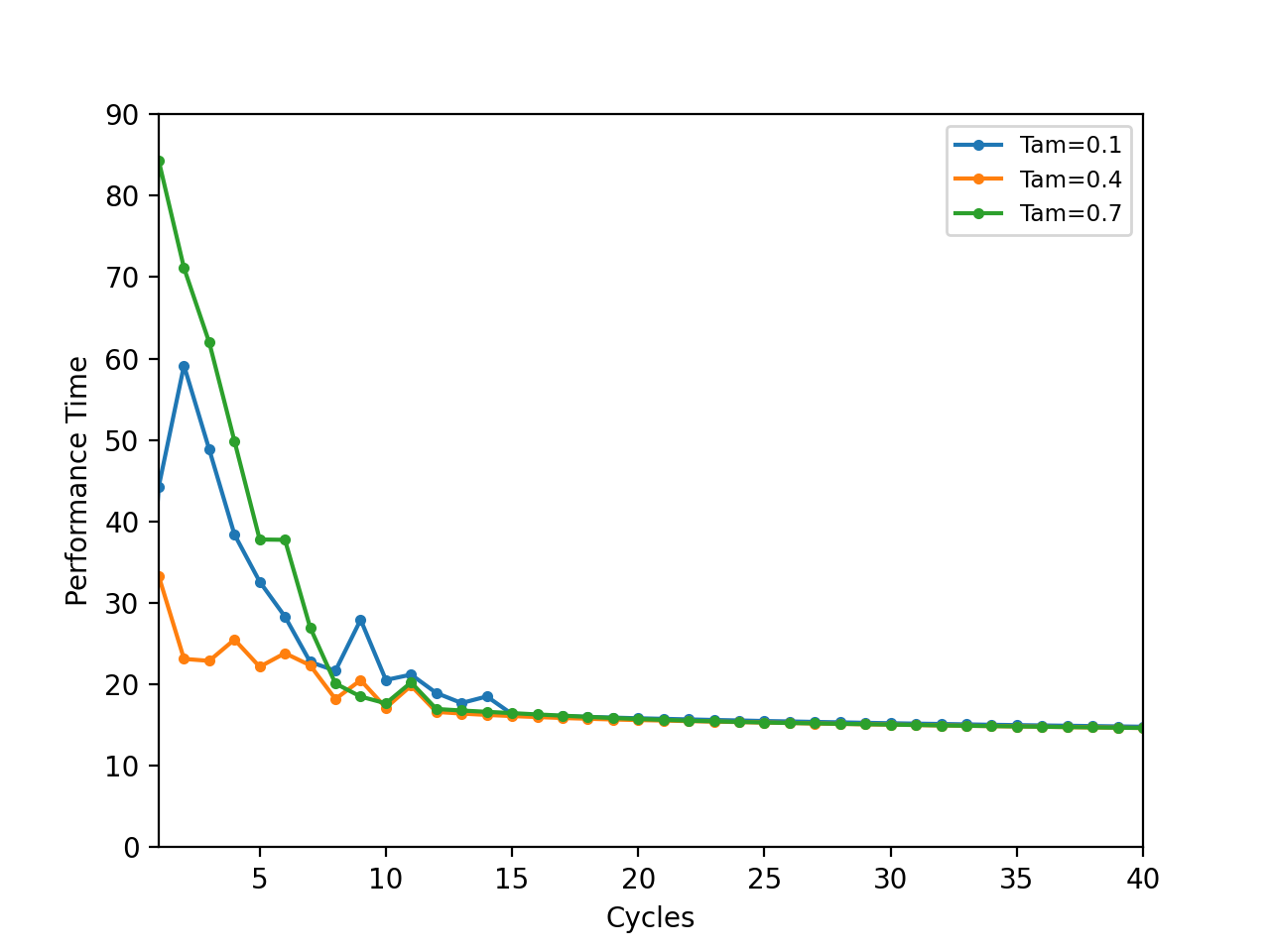
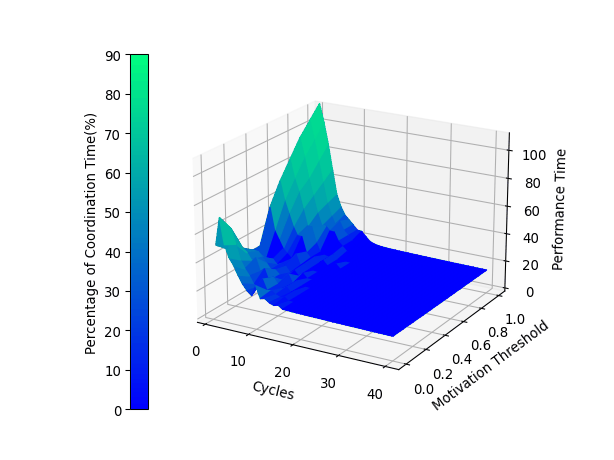
The key conclusion out of this experiment is that under conditions of equally important needs, having highly motivated team members may cause the performance time to become much longer, due to a lot of negotiating on who is doing what. Also, we observe that over time all teams perform equally concerning performance time. Because the agents improve their skills when performing a particular task, and forget tasks not being performed, in due time the motivations for different tasks will adjust accordingly, resulting in a more effective allocation process.
Whereas task performance is important in evaluating projects, with WORKMATE we can also track developments in the motivation of the team members. In the following Figure 7, we display the development of the overall satisfaction of the team members over time.
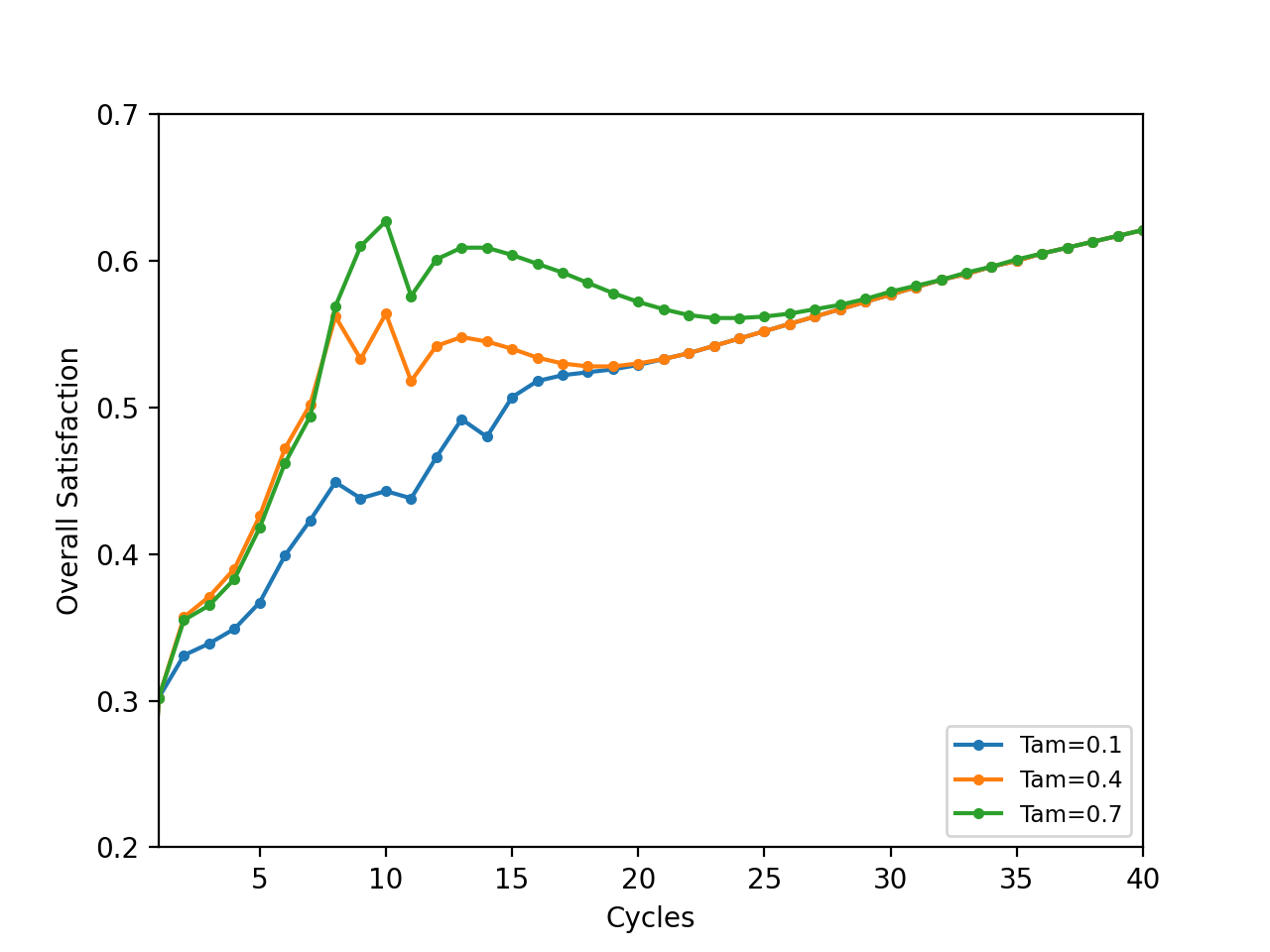
Figure 7 shows that at the beginning of the project the group overall satisfaction (\(GS\)) differs for different motivation thresholds. The highest general satisfaction (\(GS\)) can be observed for the workers with the highest motivation threshold \(T_{am}\). Hence the more critical the workers are concerning their motivation for a task, the higher their general satisfaction (\(GS\)) is. This is because when agents are more critical, their final task allocation is more likely to be consistent with their initial selection. As a consequence, their needs for autonomy and belonging are more satisfied, while at the same time their satisfaction of competence remains unchanged, as this is merely related to the difficulty of projects, and as a result, the \(GS\) will be higher.
In due time the motivation of the workers in all conditions will reach a high level. Again, this is the logical consequence of the agents improving their skills when performing a particular task, and unlearning tasks not being performed.
Task performance concerning single need dominance
Since we discern three different types of needs, teams can differ concerning the focus of their motivation. With WORKMATE we can create teams where one of the three needs is dominantly present, and explore how this affects the team performance. In the following Figure 8, the performance time of four different teams is presented, one team composed of workers for whom all needs are equally important, and 3 teams where respectively the need for competence, autonomy, or belonging dominates their behaviour.
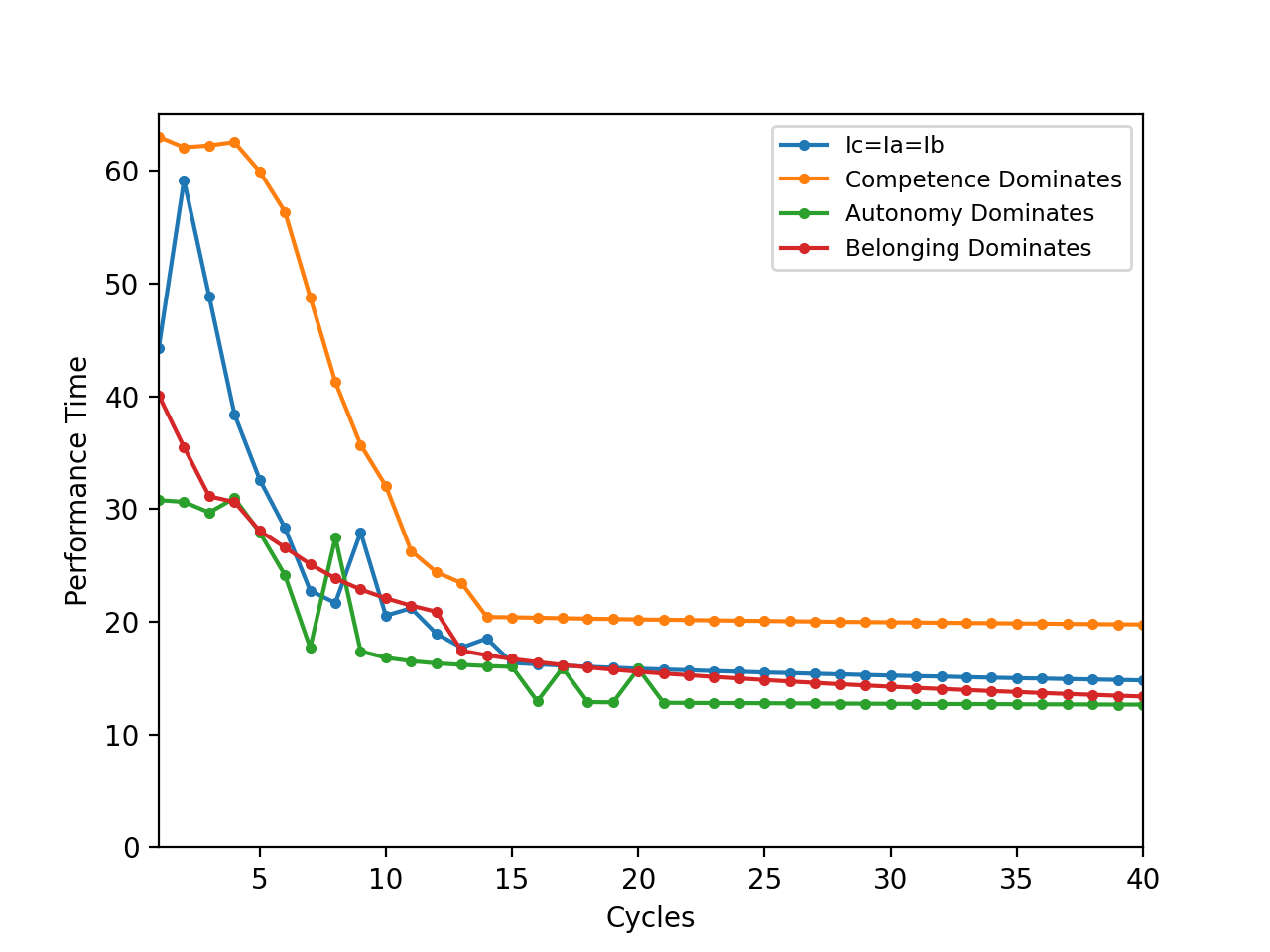
Given that under all conditions the motivation threshold \(T_{am}\) is equal for the teams, we observe different performance times with different needs dominating. Interestingly, we find that the team with a dominating-need for autonomy has the lowest performance time (green curve, Figure 8). The explanation for this phenomenon is that when autonomy dominates the team, there is a big difference in the willingness of members to undertake specific tasks, which leads to less time spent on coordination, thereby reducing the performance time \(T_{perf}\). When competence is the dominating motivation in the team (orange curve), the motivation of all agents to perform a specific task will be high causing that more time is needed for the task allocation process.
In the following Figure 9. we plot the development of the general need satisfaction of the four-team conditions. We observe that the team with the focus on competence starts with the highest motivation, and ends with the lowest motivation. When autonomy or belonging dominates, we see an increase in motivation over time. For the equal needs’ importance group, we see also an increase in general satisfaction, albeit more moderate. This moderation is caused by the need for competence, which is less satisfied.
According to the setting of this experiment, agents’ knowledge and task requirements have initially little discrepancy, so the motivation of competence is the highest at the beginning since an agent would be most motivated if the knowledge of itself close to the task requirement (Schiepe-Tiska 2013). As a consequence, the satisfaction of competence accounts for the vast majority of the general satisfaction ( \(GS\)) in this case. After a while, the autonomy dominating team starts to have the highest level of general satisfaction. This is because in a later stage (after 10 cycles) of task processing, agents are more likely to perform the task they want, causing that their need for autonomy is more satisfied, leading towards the highest general satisfaction.
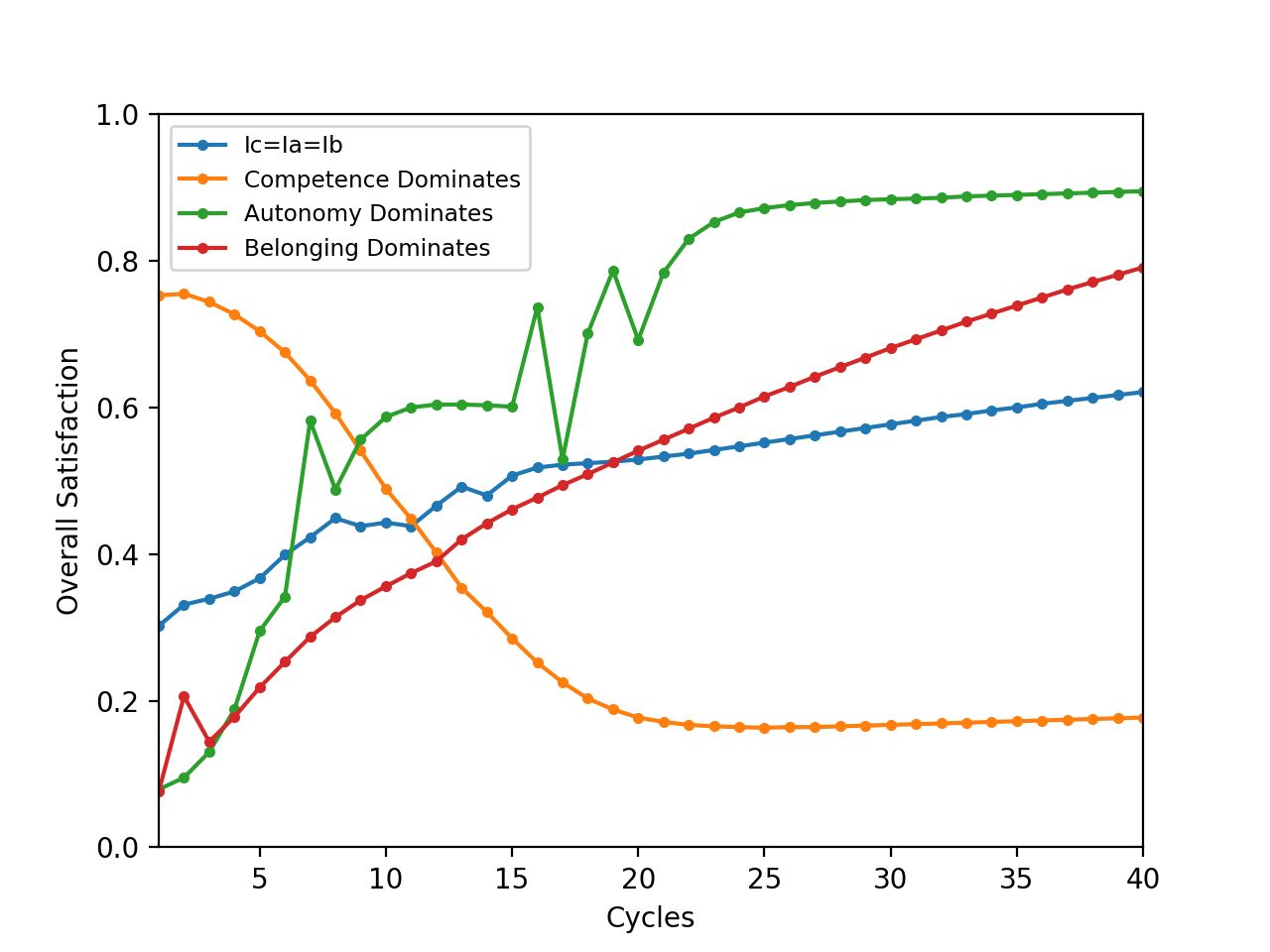
In further exploring the impact of need importance on team performance we systematically vary the importance of respectively the need for competence, autonomy, and belonging, whilst also varying the motivation threshold. In the following Figure 10 and Figure 11, it can be observed how performance time changes for different conditions of need dominance and motivation threshold.
To explore the impact of the importance of the three needs on performance time, we consider one of the three needs’ importance gradually increases from 0.1 to 1, the other two needs’ importance remains the same. For example, \(I_c=0.1\), and \(I_a=I_b=0.5\) initially, then \(I_c\) is increasing from 0.1 to 1, \(I_a\) and \(I_b\) are constant. It can be observed that a more complex 3-way interaction effect exists between need dominance, motivation threshold, and time. The motivation threshold of the team members and the relative importance of needs may have different effects on the performance over time. The "blue channels" in the landscape that are visible in the first-time step of the simulations show that (a) when the importance of competence increases, also motivation threshold should increase for the team to perform good, (b) when the importance of autonomy increases, motivation threshold should decrease for the team to perform well, and (c) when the importance of belonging increases, motivation threshold should decrease for the team to perform well.
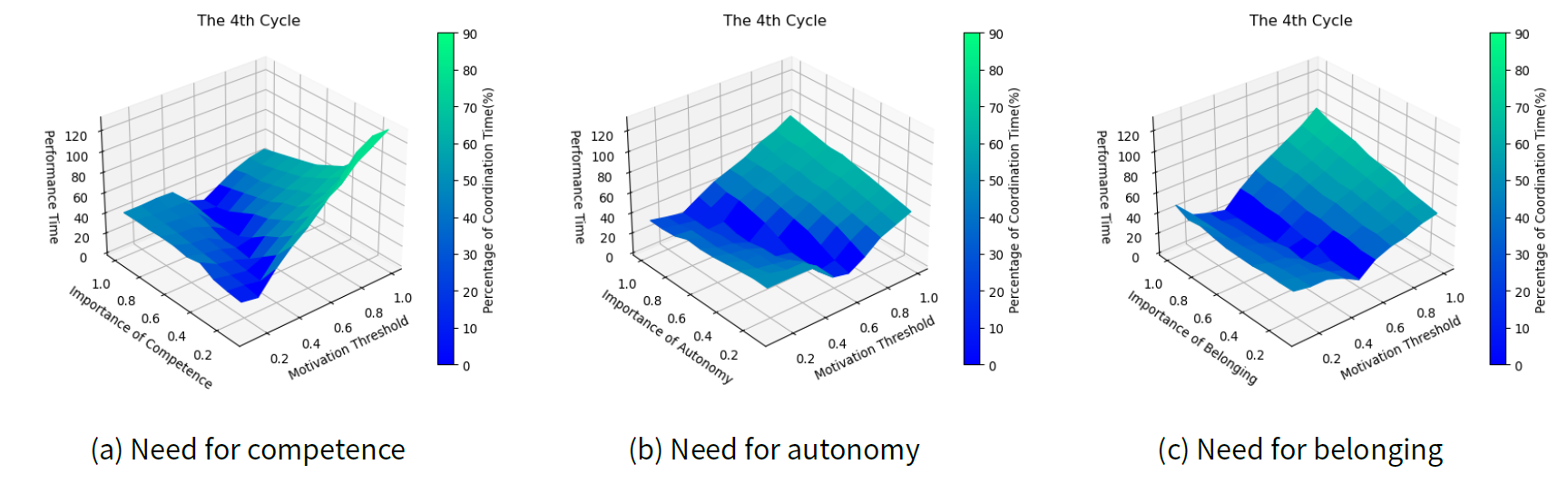
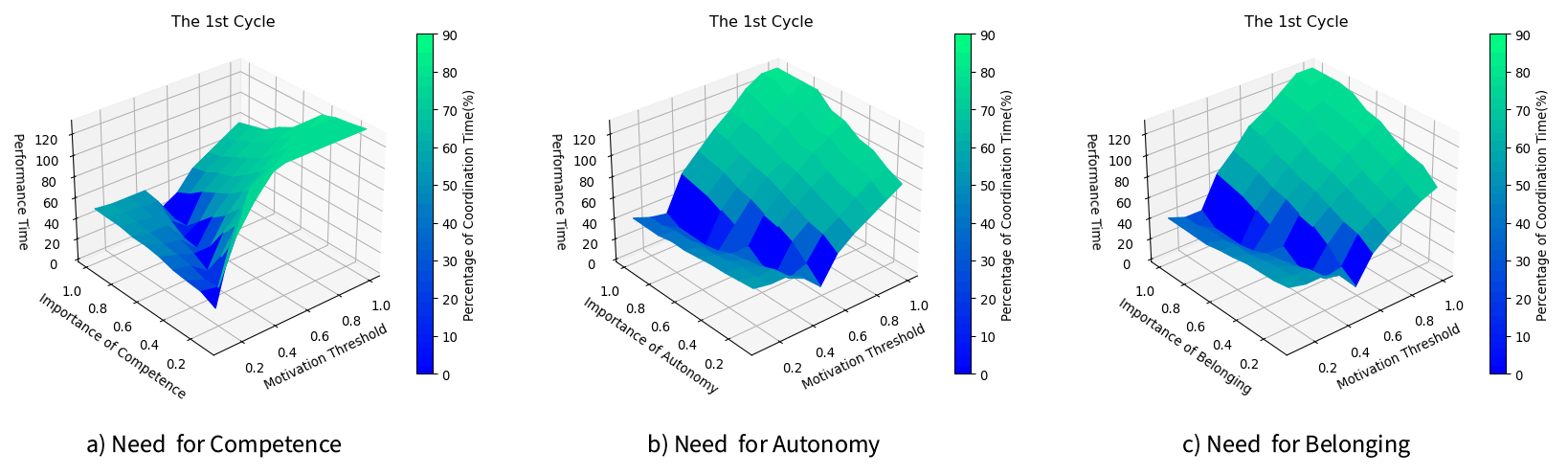
We now focus on how the importance of three needs influences the model behaviour. Looking at Figure 10 a and Figure 11 a, we observe that when the importance of competence \(I_c\) is increasing from 0.1 to 1, the optimum \(T_{am}\) increases, and vice versa. An explanation for that is, in this case, the motivation of competence is larger than the motivation of the other two needs, when \(I_c\) decreases, the overall motivation also decreases, and so does the optimal \(T_{am}\).
Figure 10 b and Figure 11 b show that if the importance of autonomy \(I_a\) is increasing from 0.1 to 1, the optimum \(T_{am}\) goes down as the \(I_a\) goes up, and vice versa. The reason for this result is, when reduces \(I_a\), the overall motivation improves, which requires a higher \(T_{am}\) to help agents complete the allocation process more effectively.
Figure 10 c and Figure 11 c show that when the importance of belonging \(I_b\) is increasing from 0.1 to 1, there still exists an optimum \(T_{am}\) for the lowest \(T_{perf}\). The reason for this phenomenon is similar to that of when autonomy is dominating, namely when \(I_b\) increase, the overall motivation drops, and thus a lower \(T_{am}\) helps the allocation process.
These experiments show that with a clearly defined agent-based model we can formally identify the complex interactions between multiple factors determining team performance. For different combinations of factors, we can identify what dimension is the most relevant to improve performance, not only in the short run but also considering the team develops over time.
Top-down management versus self-organisation
One of the interesting possibilities to experiment with is the impact of managerial strategies on team performance, both in the short and in the long run. In the following Figure 12, we compare 3 conditions of self-organisation with one condition where the task allocation is not being self-organised, but top-down allocated based on competence. In this condition, the tasks are allocated to the team member with the highest skill.
Figure 12 shows for the current conditions that a top-down decision allocating people to tasks solely based on their competence is most effective in the short run. Whereas the more complicated bottom-up allocation process requires more negotiation in the beginning, especially with a team having a high motivation threshold team (\(T_{am}=0.7\)), in due time they will surpass the efficiency of the top-down management. These results suggest that under the current conditions top-down management to speed up the performance indeed will produce effects, and may be considered to be a success. It even is a success as we observe the performance time improving over time. However, top-down management may be counterproductive in the longer run, and management having trust in the self-organising capacities of a team may be better off.
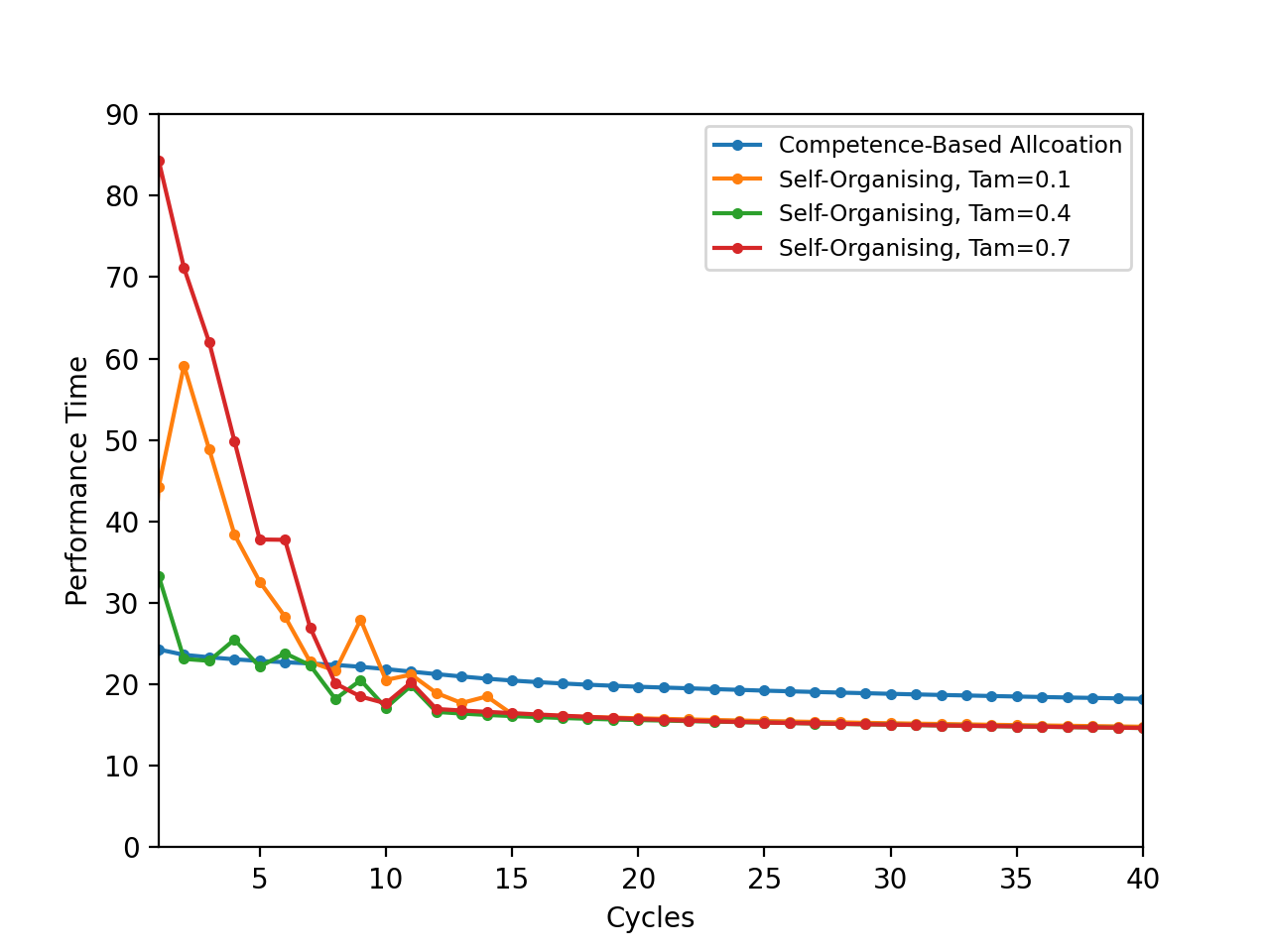
Whereas this run demonstrates that the top-down strategy, despite its higher efficacy at the beginning of the teamwork, is inferior to self-organising under these conditions, we can also observe the impact on the satisfaction of the team members. In Figure 13, we plot the development of the satisfaction of the team members. As can be observed, the satisfaction levels under the top-down condition are the lowest.
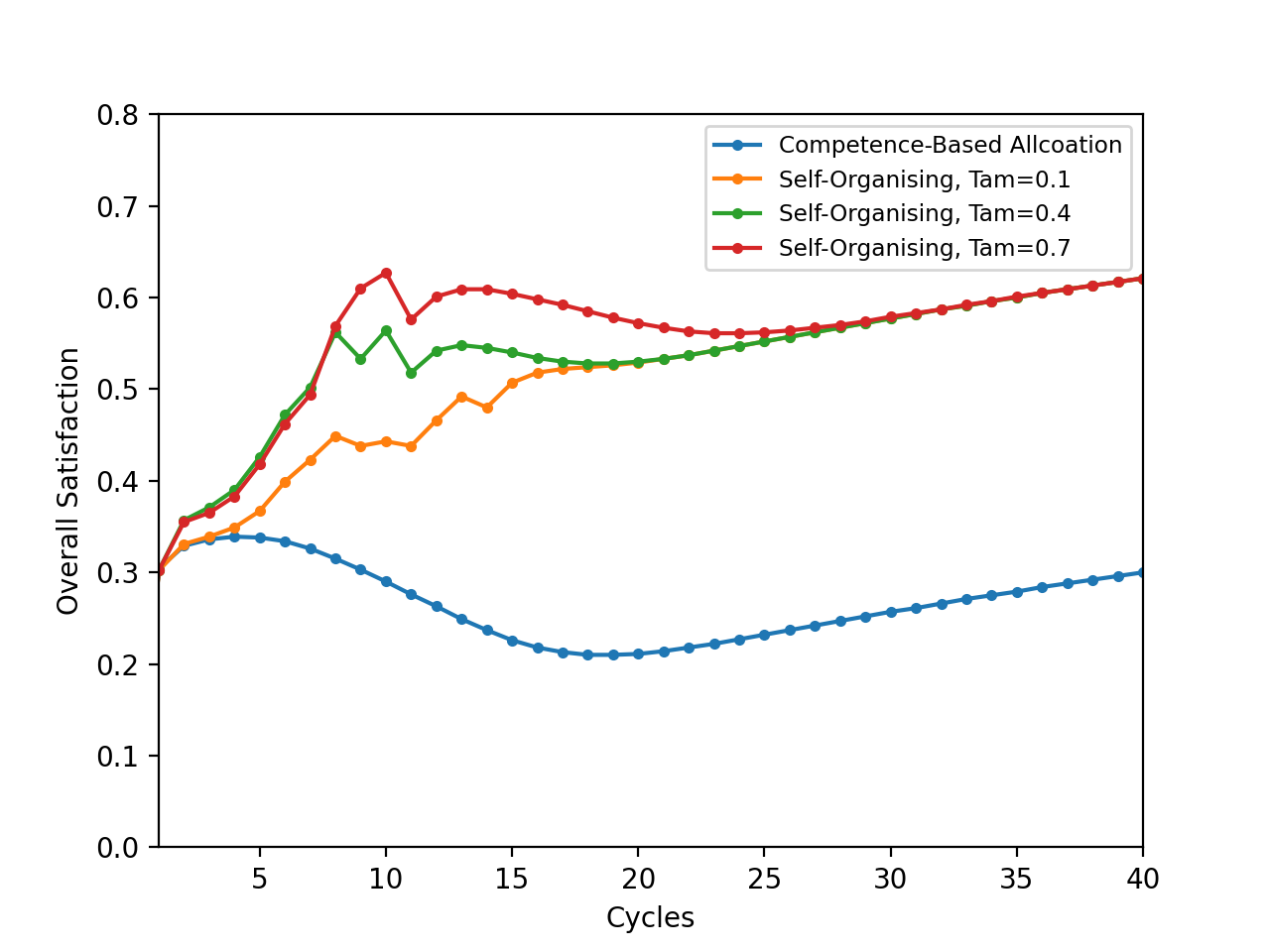
The current experiment is mainly to demonstrate our model’s capacity to experiment with management strategies and identify the impact on team performance and motivation. Many more experiments will have to be conducted to develop a more complete picture of how different management styles interact with the performance of different teams and project conditions.
Further sensitivity analyses can, of course, be conducted. The experiments that we present here serve as proof of concept. We demonstrate that it is probable with our model to explore the dynamical relations between individual characteristics of team members, task complexity, management strategy and overall team performance. Obviously, many experiments are possible where combinations of personal characteristics, the complexity of tasks and different managerial strategies can be systematically explored. Here sensitivity analysis will be important to identify the robustness of effects and to identify interesting instabilities in certain organisational settings.
Conclusions and Discussion
In this paper, we formalise the Self-Determination Theory of Deci & Ryan (2012) to update the WORKMATE model (Zoethout et al. 2006) with a motivational component. This updated WORKMATE model offers a framework to explore social dynamics with respect to the interrelation between individual and group levels. We present some basic experimentation as a proof-of-concept on discovering the dynamical relationship between individual needs and group performance.
With regard to a self-organising task allocation process, the first illustrative results show some interesting phenomena.
First, we studied the impact of the motivation threshold on team performance and team overall satisfaction. Through analysing the first simulation results, we found that a) there exists an optimal motivation threshold that will minimise the team’s performance time, b) The group’s overall satisfaction improves as the motivation threshold increases.
Second, we studied the impact of needs’ importance on team performance and overall satisfaction. We utilise the parameters (\(I_c\), \(I_a\), \(I_b\)) that can affect the balance between the three motivations as an independent variable to explore its effect on team performance. The first results show that a) when the motivation threshold is moderate, the performance time will be lowest when in an autonomy-dominating team and will be highest when in a competence-dominating team. b) the optimal motivation threshold for the lowest performance time will increase as the importance of competence increases, but it will decrease as the importance of autonomy increases. c) when the task performing system has been running for a period of steps, the team that is autonomy-dominated always has the highest group overall satisfaction.
Third, we found that a top-down task allocation focusing on team members’ knowledge and skills might save a lot of time in the beginning because the workers do not spend time on the allocation process. However, in the long run, conditions are possible where self-organisation is both more efficient and results in the higher motivation of the team members.
The experiments as presented in this paper are just an example of the potential of WORKMATE to identify dynamical processes and phenomena in collaborating teams. Because there is no systematic analysis of the robustness of the effects under varying conditions, the results should be interpreted with caution. Nevertheless, we think that the first results show the potential of this model for systematically studying team processes in a novel and systematic manner, and may provide a formal framework supporting the analysis of empirical cases.
As an example, in our experiment on self-organisation versus top-down allocation of tasks based on expertise, the first results indicated the possibility of a "managerial trap". When in a team much time is spent on negotiating who is doing what, an enforced top-down decision allocating the tasks on the basis of the expertise of the team members will result in an immediate improvement in performance time. Additionally, the performance time will further improve due to learning effects. Whereas this suggests managerial success, our model reveals that continuing with the self-organised task allocation not only may prove to be more effective in the long run, but it also causes the motivation of the team members to be higher.
The better performance of self-organisation is an emergent effect. Because the performance time is better than when the tasks are optimised on individual expertise, a "gestalt" effect is emerging. We tend to interpret this as a "team spirit effect". In this paper, using the agent-based simulation method, we studied the effect of individual needs on team performance pertaining to the self-organisation allocation. However, this study does have some limitations. First, the performance time is an important performance indicator, its value depends on individual psychological parameters, therefore, the value has no practical meaning, but the comparison of superiority and the trend in different contexts significantly matter. Second, the heterogeneity of team members and the complexity of the project affect the productivity of the team, but in the experiments of this article, we attempt to eliminate the impact of their differences through the initial settings of the experiment. Third, belonging consists of two parts. The first part is about the connectivity, which we have modelled as connections among agents and the strength of those connections. The second part is related to higher-order of cognitive functions, which are about sharing beliefs, shared values of group identity and feeling a part of a larger group. Since the model we present in this paper is already quite complicated, we decide to utilise the connectivity as a proxy for belonging. It remains a challenge to address the more social cognitive processes related to connectivity in modelling team group identities. We hope in future work to expand our model in this direction.
The proposed computational model in this paper can become a foundation for further scientific advancements. Future exploration directions include introducing the relation between self-interested behaviours and team behaviours, for example, shirking of employees would adversely affect individuals and organisations (Antosz et al. 2020), the free-riding behaviour will make the group work unpleasant and make members feel frustrated (Hall & Buzwell 2013), and social punishment has an impact on cooperative behaviour (Wang et al. 2013). Another possible venue for further experimentation with the model is to explore under what conditions of team composition and task complexity (Liu & Li 2012), different managerial and self-organisational principles perform in terms of team efficiency and team satisfaction. Following Ashby’s law (Ashby 1991), the complexity of the management system needs to be adapted to the complexity of the management object system. Some projects may be better managed top-down, especially when team composition generates a lot of allocation discussions, and the project is short-lived. Other projects may benefit from investing more time in the self-organisational processes because the merits of a well-running team will become manifest at a later stage. Obviously, the complexity of a project and the diversity of skills required will be important factors to investigate.
Model Documentation
A complete and detailed model description following the ODD protocol (Grimm et al. 2006, 2020) is provided in the Appendix. The model itself was implemented with NetLogo 6.1 (Wilensky 1999), which can be found in CoMSES online repository at: https://bit.ly/3uUJhCx.Acknowledgments
This work is partly supported by the China Scholarship Council (CSC) under the Grant CSC No. 201906060101.Appendix
A complete and detailed model description following the ODD protocol (Grimm et al. 2006, 2020) can be accessed here.
References
ANTOSZ, P., Rembiasz, T., & Verhagen, H. (2020). Employee shirking and overworking: Modelling the unintended consequences of work organisation. Ergonomics, 63(8), 997–1009. [doi:10.1080/00140139.2020.1744710]
ASHBY, W. R. (1991). 'Requisite variety and its implications for the control of complex systems.' In Facets of Systems Science (pp. 405–417). Boston, MA: Springer US. [doi:10.1007/978-1-4899-0718-9_28]
ATTNEAVE, F., & Hebb, D. O. (1949). The organization of behavior: A neuropsychological theory. The American Journal of Psychology, 63(4), 633. [doi:10.2307/1418888]
BAUMEISTER, R. F., & Leary, M. R. (1995). The need to belong: Desire for interpersonal attachments as a fundamental human motivation. Psychological Bulletin, 117(3), 497–529. [doi:10.1037/0033-2909.117.3.497]
CHAE, S. W., Seo, Y. W., & Lee, K. C. (2015). Task difficulty and team diversity on team creativity: Multi-agent simulation approach. Computers in Human Behavior, 42, 83–92. [doi:10.1016/j.chb.2014.03.032]
CHAIKLIN, S. (2003). 'The zone of proximal development in Vygotsky’s analysis of learning and instruction.' In A. Kozulin, B. Gindis, V. S. Ageyev, & S. M. Miller (Eds.), Vygotsky’s Educational Theory in Cultural Context (pp. 39–64). Cambridge, MA: Cambridge University Press. [doi:10.1017/cbo9780511840975.004]
CHAUDHARY, R., Rangnekar, S., & Barua, M. K. (2012). Relationships between occupational self efficacy, human resource development climate, and work engagement. Team Performance Management: An International Journal, 18(7–8), 370–383. [doi:10.1108/13527591211281110]
CHEN, Y. M., & Wei, C. W. (2009). Multiagent approach to solve project team work allocation problems. International Journal of Production Research, 47(13), 3453–3470. [doi:10.1080/00207540802356754]
CHERRINGTON, D. J. (1989). The Management of Individual and Organizational Performance: Instructor’s Manual for Organizational Behavior. Boston, MA: Allyn; Bacon.
DE CASTELLA, K., Byrne, D., & Covington, M. (2013). Unmotivated or motivated to fail? A cross-cultural study of achievement motivation, fear of failure, and student disengagement. Journal of Educational Psychology, 105(3), 861–880. [doi:10.1037/a0032464]
DECI, E. L., & Ryan, R. M. (2012). 'Motivation, personality, and development within embedded social contexts: An overview of self-Determination theory.' In R. M. Ryan (Ed.), The Oxford Handbook of Human Motivation (pp. 84–108). New York, NY: Oxford University Press. [doi:10.1093/oxfordhb/9780195399820.013.0006]
DONG, Y., Bartol, K. M., Zhang, Z. X., & Li, C. (2017). Enhancing employee creativity via individual skill development and team knowledge sharing: Influences of dual-focused transformational leadership. Journal of Organizational Behavior, 38(3), 439–458. [doi:10.1002/job.2134]
ELLIOT, A. J., & Carol S. Dweck, D. (2012). Handbook of Competence and Motivation, Second Edition: Theory and Application. New York, NY: Guilford Publications.
EVANS, M., & Boucher, A. R. (2015). Optimizing the power of choice: Supporting student autonomy to foster motivation and engagement in learning. Mind, Brain, and Education, 9(2), 87–91. [doi:10.1111/mbe.12073]
FURNHAM, A. (2012). The Psychology of Behaviour at Work: The Individual in the Organization. Hove: Psychology Press.
GAGNÉ, M., & Deci, E. L. (2005). Self-determination theory and work motivation. Journal of Organizational Behavior, 26(4), 331–362.
GLOBERSON, S., Levin, N., & Shtub, A. (1989). The impact of breaks on forgetting when performing a repetitive task. IIE Transactions, 21(4), 376–381. [doi:10.1080/07408178908966244]
GONG, Y., Kim, T. Y., Lee, D. R., & Zhu, J. (2013). A multilevel model of team goal orientation, information exchange, and creativity. Academy of Management Journal, 56(3), 827–851. [doi:10.5465/amj.2011.0177]
GRIMM, V., Berger, U., Bastiansen, F., Eliassen, S., Ginot, V., Giske, J., Goss-Custard, J., Grand, T., Heinz, S. K., Huse, G., Huth, A., Jepsen, J. U., Jørgensen, C., Mooij, W. M., Müller, B., Pe’er, G., Piou, C., Railsback, S. F., Robbins, A. M., … DeAngelis, D. L. (2006). A standard protocol for describing individual-based and agent-based models. Ecological Modelling, 198(1–2), 115–126. [doi:10.1016/j.ecolmodel.2006.04.023]
GRIMM, V., Railsback, S. F., Vincenot, C. E., Berger, U., Gallagher, C., DeAngelis, D. L., Edmonds, B., Ge, J., Giske, J., Groeneveld, J., Johnston, A. S. A., Milles, A., Nabe-Nielsen, J., Polhill, J. G., Radchuk, V., Rohwäder, M.-S., Stillman, R. A., Thiele, J. C., & Ayllón, D. (2020). The ODD protocol for describing agent-Based and other simulation models: A second update to improve clarity, replication, and structural realism. Journal of Artificial Societies and Social Simulation, 23(2), 7: https://www.jasss.org/23/2/7.html. [doi:10.18564/jasss.4259]
HAGERTY, B. M. K., Lynch-Sauer, J., Patusky, K. L., Bouwsema, M., & Collier, P. (1992). Sense of belonging: A vital mental health concept. Archives of Psychiatric Nursing, 6(3), 172–177. [doi:10.1016/0883-9417(92)90028-h]
HALL, D., & Buzwell, S. (2013). The problem of free-riding in group projects: Looking beyond social loafing as reason for non-contribution. Active Learning in Higher Education, 14(1), 37–49. [doi:10.1177/1469787412467123]
HEAVEY, C., Halliday, S. V., Gilbert, D., & Murphy, E. (2011). Enhancing performance. Journal of General Management, 36(3), 1–18. [doi:10.1177/030630701103600301]
HELBING, D. (2012). Social Self-Organization: Agent-Based Simulations and Experiments to Study Emergent Social Behavior. Berlin Heidelberg: Springer.
HÜLSHEGER, U. R., Anderson, N., & Salgado, J. F. (2009). Team-level predictors of innovation at work: A comprehensive meta-analysis spanning three decades of research. Journal of Applied Psychology, 94(5), 1128–1145.
INAYATULLAH, A., & Jehangir, P. (2012). Teacher’s job performance: The role of motivation. Abasyn Journal of Social Sciences, 5(2), 78–99.
JAGER, W. (2017). Enhancing the realism of simulation (EROS): On implementing and developing psychological theory in social simulation. Journal of Artificial Societies and Social Simulation, 20(3), 14: https://www.jasss.org/20/3/14.html. [doi:10.18564/jasss.3522]
JANICIK, G. A., & Bartel, C. A. (2003). Talking about time: Effects of temporal planning and time awareness norms on group coordination and performance. Group Dynamics: Theory, Research, and Practice, 7(2), 122–134. [doi:10.1037/1089-2699.7.2.122]
KOZLOWSKI, S. W. J. (2018). Enhancing the effectiveness of work groups and teams: A reflection. Perspectives on Psychological Science, 13(2), 205–212. [doi:10.1177/1745691617697078]
KOZLOWSKI, S. W. J., & Bell, B. S. (2012). 'Work groups and teams in organizations.' In I. B. Weiner (Ed.), Handbook of Psychology (Vol. 12). Hoboken, NJ: John Wiley & Sons, Inc.
KOZLOWSKI, S. W. J., & Ilgen, D. R. (2006). Enhancing the efectiveness of work groups and teams. Psychological Science in the Public Interest, Supplement, 7(3), 77–124. [doi:10.1111/j.1529-1006.2006.00030.x]
KOZLOWSKI, S. W. J., & Klein, K. J. (2000). 'A multilevel approach to theory and research in organizations: Contextual, temporal, and emergent processes.' In K. J. Klein & S. W. J. Kozlowski (Eds.), Multilevel Theory, Research and Methods in Organizations: Foundations, Extensions and New Directions (pp. 3–90). Hoboken, NJ: Jossey-Bass.
KUSURKAR, R. A., Ten Cate, T. J., Vos, C. M. P., Westers, P., & Croiset, G. (2013). How motivation affects academic performance: A structural equation modelling analysis. Advances in Health Sciences Education, 18(1), 57–69. [doi:10.1007/s10459-012-9354-3]
LATHAM, G. P., & Pinder, C. C. (2005). Work motivation theory and research at the dawn of the twenty-First century. Annual Review of Psychology, 56(1), 485–516. [doi:10.1146/annurev.psych.55.090902.142105]
LEGAULT, L. (2016). 'The need for autonomy.' In V. Zeigler-Hill & T. K. Shackelford (Eds.), Encyclopedia of Personality and Individual Differences (pp. 1–3). Cham: Springer International Publishing. [doi:10.1007/978-3-319-28099-8_1120-1]
LIN, J. H. (2016). Need for relatedness: A self-determination approach to examining attachment styles, Facebook use, and psychological well-being. Asian Journal of Communication, 26(2), 153–173. [doi:10.1080/01292986.2015.1126749]
LIU, P., & Li, Z. (2012). Task complexity: A review and conceptualization framework. International Journal of Industrial Ergonomics, 42(6), 553–568. [doi:10.1016/j.ergon.2012.09.001]
LOCKE, E. A. (2013). New Developments in Goal Setting and Task Performance. London, UK: Routledge.
MULLINS, L. J. (2007). Management and Organisational Behaviour. London, UK: Pearson Education.
NAYLOR, J. C., Pritchard, R. D., & Ilgen, D. R. (2013). A Theory of Behavior in Organizations. Cambridge, MA: Academic Press.
NIEMIEC, C. P., & Ryan, R. M. (2013). 'What makes for a life well lived? Autonomy and its relation to full functioning and organismic wellness.' In S. David, I. Boniwell, & A. C. Ayers (Eds.), Oxford Handbook of Happiness (pp. 1–16). Oxford, UK: Oxford University Press. [doi:10.1093/oxfordhb/9780199557257.013.0016]
PAVEY, L., Greitemeyer, T., & Sparks, P. (2011). Highlighting relatedness promotes prosocial motives and behavior. Personality and Social Psychology Bulletin, 37(7), 905–917. [doi:10.1177/0146167211405994]
PLOYHART, R. E., & Moliterno, T. P. (2011). Emergence of the human capital resource: A multilevel model. Academy of Management Review, 36(1), 127–150. [doi:10.5465/amr.2009.0318]
REEVE, J. (2014). Understanding Motivation and Emotion. Hoboken, NJ: John Wiley & Sons.
RENDÓN Rozo, K., Arellana, J., Santander-Mercado, A., & Jubiz-Diaz, M. (2019). Modelling building emergency evacuation plans considering the dynamic behaviour of pedestrians using agent-based simulation. Safety Science, 113, 276–284.
RENKO, M., Kroeck, K. G., & Bullough, A. (2012). Expectancy theory and nascent entrepreneurship. Small Business Economics, 39(3), 667–684. [doi:10.1007/s11187-011-9354-3]
RICO, R., Sánchez-Manzanares, M., Gil, F., & Gibson, C. (2008). Team implicit coordination processes: A team knowledge-Based approach. Academy of Management Review, 33(1), 163–184. [doi:10.5465/amr.2008.27751276]
RYAN, R. M., & Deci, E. L. (2000). Self-determination theory and the facilitation of intrinsic motivation, social development, and well-being. American Psychologist, 55(1), 68–78. [doi:10.1037/0003-066x.55.1.68]
RYAN, R. M., & Deci, E. L. (2017). 'Self-Determination theory: Basic psychological needs in motivation, development, and wellness.' In R. M. Ryan & E. L. Deci (Eds.), Self-Determination Theory: Basic Psychological Needs in Motivation, Development, and Wellness. New York, NY: Guilford Press.
RYAN, R. M., & Moller, A. C. (2017). Competence as a necessary but not sufficient condition for high quality motivation: A self-determination theory perspective. Handbook of Competence Motivation, 214–231.
SCHIEPE-TISKA, A. (2013). In the power of flow: The impact of implicit and explicit motives on flow experience with a special focus on the power domain. PhD Thesis. Available at: https://mediatum.ub.tum.de/doc/1128674/1128674.pdf.
SCHÜLER, J., Sheldon, K. M., Prentice, M., & Halusic, M. (2016). Do some people need autonomy more than others? Implicit dispositions toward autonomy moderate the effects of felt autonomy on well-Being. Journal of Personality, 84(1), 5–20.
VAN Iddekinge, C. H., Aguinis, H., Mackey, J. D., & DeOrtentiis, P. S. (2018). A meta-Analysis of the interactive, additive, and relative effects of cognitive ability and motivation on performance. Journal of Management, 44(1), 249–279. [doi:10.1177/0149206317702220]
WALTON, G. M., Cohen, G. L., Cwir, D., & Spencer, S. J. (2012). Mere belonging: The power of social connections. Journal of Personality and Social Psychology, 102(3), 513–532. [doi:10.1037/a0025731]
WANG, S., Dang, Y., & Wu, J. (2017). 'The effect of task allocation strategy on knowledge intensive team performance based on computational experiment.' In J. Chen, T. Theeramunkong, T. Supnithi, & X. Tang (Eds.), Communications in Computer and Information Science (Vol. 780, pp. 227–241). Singapore: Springer Singapore. [doi:10.1007/978-981-10-6989-5_19]
WANG, S., Dang, Y., & Wu, J. (2018). How task allocation strategy affects team performance: A computational experiment. Journal of Systems Science and Systems Engineering, 27(5), 656–676. [doi:10.1007/s11518-018-5387-3]
WANG, Z., Xia, C.-Y., Meloni, S., Zhou, C.-S., & Moreno, Y. (2013). Impact of social punishment on cooperative behavior in complex networks. Scientific Reports, 3(1), 3055. [doi:10.1038/srep03055]
WEI, L. T., & Yazdanifard, R. (2014). The impact of positive reinforcement on employees’ performance in organizations. American Journal of Industrial and Business Management, 04(1), 9–12. [doi:10.4236/ajibm.2014.41002]
WEINER, B. (1985). An attributional theory of achievement motivation and emotion. Psychological Review, 92(4), 548–573. [doi:10.1037/0033-295x.92.4.548]
WHITE, R. W. (1959). Motivation reconsidered: The concept of competence. Psychological Review, 66(5), 297–333. [doi:10.1037/h0040934]
WILCZYŃSKA, A., Januszek, M., & Bargiel-Matusiewicz, K. (2015). The need of belonging and sense of belonging versus effectiveness of coping. Polish Psychological Bulletin, 46(1), 72–81.
WILENSKY, U. (1999). NetLogo. for connected learning and computer-based modeling, Northwestern University.
WILKE, H. A. M., & Meertens, R. W. (1994). Group Performance. London, UK: Routledge.
WOOD, R. E. (1986). Task complexity: Definition of the construct. Organizational Behavior and Human Decision Processes, 37(1), 60–82. [doi:10.1016/0749-5978(86)90044-0]
ZAHRA, i., Xinping, X., Shafi, K., Hua, L., & Nazeer, A. (2015). Effect of salary, training and motivation on job performance of employees. American Journal of Business, Economics and Management, 3(2), 55–58.
ZOETHOUT, K., Jager, W., & Molleman, E. (2006). Formalizing self-organizing processes of task allocation. Simulation Modelling Practice and Theory, 14(4), 342–359. [doi:10.1016/j.simpat.2005.09.004]
ZOETHOUT, K., Jager, W., & Molleman, E. (2008). Task dynamics in self-organising task groups: Expertise, motivational, and performance differences of specialists and generalists. Autonomous Agents and Multi-Agent Systems, 16(1), 75–94. [doi:10.1007/s10458-007-9022-9]
ZOETHOUT, K., Jager, W., & Molleman, E. (2010). When does a newcomer contribute to a better performance? A multi-Agent study on self-Organising processes of task allocation. Journal of Artificial Societies and Social Simulation, 13(3), 7: https://www.jasss.org/13/3/7.html. [doi:10.18564/jasss.1614]