Conditions and Effects of Norm Internalization

and
aCenter for Environmental Systems Research (CESR), University of Kassel, Germany
Journal of Artificial
Societies and Social Simulation 26 (1) 6
<https://www.jasss.org/26/1/6.html>
DOI: 10.18564/jasss.5003
Received: 09-Jul-2021 Accepted: 03-Jan-2023 Published: 31-Jan-2023
Abstract
Norm internalization refers to the process of adoption of normative beliefs by individuals, thus representing a link between individual and social change. However, there are several questions regarding norm internalization which need to be answered. These include understanding under which circumstances norm internalization does occur by considering the effects of internalizing either a certain norm or even conflicting norms. To investigate the conditions and effects of norm internalization, we developed a theoretical agent-based model called “DINO”, comprising a norm internalization process grounded on a psychological model of decision-making, considering different types of norms, goals, and habits as well as inter-individual differences. Our conceptualization of personal norms introduces a new level of complexity, allowing for more than one norm to be internalized and either approved or disapproved. Our conceptual model was implemented within the framework of a 3-person prisoner’s dilemma game. Results showed that playing with cooperative others generally facilitated norm internalization. Norm internalization encouraged norm compliance and affected behavioural stability and payoff equality. We discuss how our results relate to empirical findings and theoretical literature, providing a bridge between theory development and empirically testable hypotheses and between psychological micro-level phenomena and social dynamics.Norms, Internalization, Learning, Social Dilemma, Cooperation, Decision-Making
Introduction
Norm internalization is a key mechanism in norm compliance and norm maintenance (Axelrod 1986; Gintis 2004; Horne 2003). In the social sciences, there is a long tradition of theorizing about and studying norm internalization (Parsons 1937; Piaget 1970; Ullmann-Margalit 1977). Norm internalization is considered crucial for learning social values and norms, being an important link between society and the individual (Hoffman 2000; Kohlberg 1984; Vygotsky 1930). This makes norm internalization not only relevant for the individual but also for social and societal change. Internalization has been associated with selfless behaviour (Durkheim 1893), taming the egoistic individual through socialization (Freud 1932), while social influences may arguably lead to “the creation of a storm trooper, a Buddhist monk or a civil rights activist” (Kohlberg & Hersh 1977 p. 53). Empirical studies have shown the importance of personal norms for behavioural decisions (e.g., Harland et al. 1999; Shin et al. 2018). However, there is little research about how internalization proceeds (Neumann 2010b). This is where social simulation comes into play (Jager & Ernst 2017).
In social simulation, few researchers have studied the norm internalization process (Andrighetto et al. 2010; Villatoro et al. 2015). So far, our understanding remains “fragmentary and insufficient” (Conte et al. 2010 p. 64). There is a lack of a psychologically plausible, dynamic theory of norm internalization (Hollander & Wu 2011; Neumann 2010b; Saam & Harrer 1999). Social simulation is a suitable means for developing and rigorously testing a dynamic theory. It enables exploring behavioural effects of internal changes as well as their interaction with social dynamics of change. As norm internalization is challenging to pin down methodologically (Neumann 2010b), the additional use of simulation methods to empirical approaches seems especially promising. Modelling norm internalization demands for cognitively rather complex and heterogeneous agents, since internalization is considered a higher mental function that is individually specific (Piaget 1970; Vygotsky 2004).
Here, we combine psychological theory on norm internalization with agent-based simulation methods. This has several challenges: there is a lack of theories concerning dynamic processes; there are few theoretical superstructures that combine different psychological fields, and simulation demands for a level of precision that psychological theories rarely provide. Moreover, one of the greatest issues for modelling is psychological complexity. We approached these challenges first through relying on psychological concepts and research as well as through integrating assumptions from different psychological and adjacent disciplines. Second, we limited complexity to those areas that are essential to address our research questions. We now will discuss related research on the dynamics of norms, focusing in particular on models that include norm internalization, before presenting the contribution of this work to the field.
Related research
So far, two very different approaches to simulating norms in agent-based systems have been taken. In short, the first is mainly concerned with the emergence of behavioural conventions, treating norms solely as macro level epiphenomena (e.g., Axelrod 1986). In this line of research, social convention norms are often the only quality of norms considered (Mahmoud et al. 2012; Savarimuthu et al. 2007; Sen & Airiau 2007). In the second line of research, norms are modelled as social constraints to agent’s decision-making (Shoham & Tennenholtz 1992, 1995). This idea of built-in behavioural laws was advanced by emphasizing the cognitive representations of social norms and building intelligent, autonomous agent architectures (Castelfranchi et al. 2000; Conte & Castelfranchi 1995; Saam & Harrer 1999). In the Belief-Obligations-Intentions-Desires (BOID) architecture, social norms are represented as perceived obligations and personal goals as desires (Broersen et al. 2001). Through differentiating between individual and social desires, a new complexity was introduced (Neumann 2010b).
Regarding the importance of norm internalization (Axelrod 1986; Hollander & Wu 2011; Mahmoud et al. 2014; Neumann 2008, 2010b; Saam & Harrer 1999), surprisingly few agent-based systems include such a process. Verhagen (2001) presented a model of norm internalization, defining internalization as the matching of personal norms to social norms. Whereas this operationalizes the effect of norm internalization, it “does not represent the process of norm internalization” (Neumann 2008 Section 7.6). Andrighetto et al. (2010) introduced a rich cognitive model of norm internalization, the EMIL-I-A architecture (see also Conte et al. 2010), in which, a norm may be prohibiting, prescribing, or permitting. EMIL Internalizer Agents internalize a norm depending on two conditions: a salient social norm and a cost-benefit-computation exceeding a threshold. Based on a dichotomous parameter, successful internalization stops the EMIL-I-A agent’s normative deliberation and starts a decision-making automatism, disregarding other normative and non-normative motivators. The internalized norm has become a goal in itself. As long as the social norm is still salient, the agent complies with its internalized norm. This conceptualizes an internalized norm similar to a habit that saves time and calculation effort in decision-making and is in line with Epstein (2006) assumption of internalization being blind conformism with a norm. Villatoro et al. (2015) enhanced the EMIL-I-A architecture by characterizing norm internalization as a multi-step process, whereas only the deepest level of internalization corresponds to Epstein’s assumption of thoughtless conformity. This allows agents to have partly internalized a norm and still violate it, while there is always just one norm internalized at any one time.
The present research
The current state of research leaves several questions open that existing models on norm internalization do not address. First, it seems important to investigate facilitating conditions of norm internalization to eventually be able to promote internalization of cooperation norms. Whereas norm internalization is assumed a universal process, when and how a norm is internalized depends on a person’s personality (Ryan & Deci 2017; Vygotsky 2004) interacting with its environment, but how? Which conditions facilitate norm internalization? As working hypotheses, we assumed that individuals’ inherent cooperativeness and the cooperativeness of the social surrounding positively influence internalizing the cooperation norm as appropriate.
Second, we are interested in the effects of norm internalization, assuming an imperfect relation between personal norms (the product of norm internalization) and behaviour. Whereas personal norms have been shown to influence behaviour, empirical investigations mostly found small to medium sized relations (e.g., Bamberg et al. 2007; Bamberg & Schmidt 2003; Hines et al. 1987). Hence, personal norms do not seem to translate one-to-one into behaviour (Bandura 2001; Schönbach 1990; Schwartz 1977a). One possible explanation could be that multiple internalized norms influence behavioural decisions at the same time. Assuming that there are several, potentially conflicting personal norms at work, what are the effects of norm internalization? As working hypotheses, we first hoped to replicate the finding that norm internalization increases norm compliance (Axelrod 1986; Gintis 2004). We secondly expected that individuals become more determined and persistent through norm internalization (Andrighetto et al. 2010) and therefore less flexible in their behaviour. Regarding the macro level social effects of norm internalization, there is little existing research. Since social norms have been proposed as solutions to social inequality (Saam & Harrer 1999; Ullmann-Margalit 1977), we thirdly assumed similar effects for norm internalization, decreasing inequality.
For the next step towards a better understanding of norm internalization, we aimed to disentangle conceptually distinct constructs and incorporate them separately. We did so by endorsing a psychological view that regards personal norms as conceptually distinct from habits. This enabled us to study norm internalization independently of other normative and non-normative factors driving decision-making. Based on this, we had two aims. First, we accounted for inter-individual differences that affect norm internalization to investigate individually specific facilitating conditions. Second, we allowed for more than one personal norm to be internalized and influence decision-making to examine the effects of norm internalization given multiple, potentially conflicting internalized norms. On this basis, we aimed to address the two following research questions and test the corresponding hypotheses:
- What are the mechanisms and conditions that facilitate norm internalization?
- Cooperative agents tend to internalize that it is appropriate to cooperate; defective agents tend to internalize that it is appropriate to defect.
- Cooperative settings facilitate internalizing the appropriateness of cooperation; defective settings facilitate internalizing the appropriateness of defection.
- What are the effects of norm internalization?
- Stronger norm internalization is associated with stronger norm compliance.
- Stronger norm internalization is associated with more behavioural inflexibility.
- Stronger norm internalization is associated with less payoff inequality.
The paper is structured as follows: First, we present the theoretical framework, starting with our definitions of norms and proceeding with our conceptual model of norm-based decision-making. Subsequently, we describe the game-theoretical scenario the conceptual model was applied to. We then introduce the implemented agent-based DINO model – Dynamics of Internalization and Dissemination of Norms. Next, we document the simulation results addressing our research questions and hypotheses. Finally, we discuss the results and conclude.
Theoretical Framework
Taxonomy of norms
We define a norm as a behavioural rule for a specific situation (Dannenberg et al. 2023). Social norms are shared norms between several individuals, and they can have different qualities (Cialdini et al. 1990). A social descriptive norm contains information regarding the observable regularity of a behaviour in a certain situation (i.e., “what most others do”) and is expected to affect behaviour through conformism. It “motivates by providing evidence as to what will likely be effective and adaptive action” (Cialdini et al. 1990 p. 1015). A social injunctive norm refers to the (in)appropriateness of a behaviour in a certain situation (i.e., “what most others consider (in)appropriate”; Dannenberg et al. 2023). An important mechanism explaining their influence is social (dis)approval (Ajzen 1991; Jacobson et al. 2011). Apart from social norms, personal norms describe the norms that an individual holds (Cialdini et al. 1990; Farrow et al. 2017). Personal norms are of an injunctive quality, defining an individual’s belief about (in)appropriate behaviour. They are associated with feelings of moral obligation as well as guilt and shame when violated (Schwartz 1977a; Schwartz & Howard 1981, 1982), which is why they are sometimes also referred to as “moral norms” (Bicchieri & Dimant 2019; Nyborg 2018; Thøgersen 1999). The process of how personal norms develop and change, we call norm internalization (Hoffman 2000; Kohlberg 1984).
Two more considerations are important for our research. First, we consider a norm of any type as behaviour-specific. For example, we assume that there is one personal norm relating to the (in)appropriateness of riding the bike in a specific situation and another personal norm relating to the (in)appropriateness of driving the car, whereas these two personal norms may develop independently from each other. Second, we consider each norm to vary along the dimension of encouragement to discouragement of a behaviour. This relates to prescriptive and proscriptive norms (Bendor & Swistak 2001; Bicchieri 2006; Ullmann-Margalit 1977). Hence, we suggest that, for instance, the personal norm of riding the bike can be approved of (representing a belief of appropriateness) or disapproved of (representing a belief of inappropriateness).
A conceptual model of norm-based decision-making
To investigate the dynamics of norms and their influence on behaviour, one needs theoretical assumptions on how behavioural decisions are made. Based on psychological literature, we developed a conceptual model of norm-based decision-making. Here, we first present relevant literature and then our conceptual model.
One of the most influential and best-validated theories in psychological literature is the theory of reasoned action (TRA hereafter, Fishbein & Ajzen 1975). The TRA was later expanded to the theory of planned behaviour (Ajzen 1991), explaining actions that are not under volitional control. In the present decision scenario that the conceptual model was applied to, we regard behavioural control as given and therefore applied the TRA. Both theories have been extremely successful in explaining behaviour (e.g., Sheeran 2002; Steg & Vlek 2009; Webb & Sheeran 2006). They are based on an expectancy-value model (Ajzen 1991; Atkinson 1957), consisting of expectancies and values. Expectancies are situationally adapted anticipations of behavioural outcomes. Values are a person’s individual importance of motivational factors. A multi-attribute utility calculation of expectancy and value factors determines a person’s intention. The intention is the only direct determinant of behaviour (Fishbein & Ajzen 1975, 1981). In the TRA, the authors considered two motivational factors influencing the intention: the attitude as a person’s inner strivings and the subjective norm as outer, social influence.
The full range of a person’s inner strivings evaluating behavioural outcomes in conflictual or mixed-motive situations is represented in Deutsch’s social-value orientations: individualistic, cooperative, and competitive (cf. Deutsch 1958; Messick & McClintock 1968; Murphy et al. 2011; Murphy & Ackermann 2014). While there are numerous theories describing basic drivers of behaviour under the terms of goals (e.g., Lindenberg & Steg 2007) or needs (e.g., Maslow 1943), two terms often used similarly in social simulation (Kangur et al. 2017; Schlüter et al. 2017), above mentioned social-value orientations are particularly well researched in socially interdependent decision-making situations. The TRA’s subjective norm corresponds to the above given definition of a social injunctive norm. Research has shown that social descriptive norms as well as habits influence decision-making over and above the TRA constructs (for social descriptive norms: Rivis & Sheeran 2003; White et al. 2009; for habits: Bamberg & Schmidt 2003; Perugini & Bagozzi 2001; Whitmarsh & O’Neill 2010). Investigating the additional influence of personal norms, some studies have shown (e.g., Conner & Armitage 1998; Harland et al. 1999; Shin et al. 2018) and some have failed to show their independent effects, raising the question whether their effects are separable from those of other behavioural influences (Bamberg & Schmidt 2003; Kaiser & Scheuthle 2003). Theorists provided a possible explanation for the inconclusive empirical results, assuming norm internalization to be a higher-level process, qualitatively different from the lower-level processes of social norm imitation (Kohlberg & Hersh 1977; Piaget 1970; Vygotsky 1930).
Figure 1 depicts our conceptual model of norm-based decision-making, being an adaptation and extension of the TRA, including all factors presented above. Referring to the TRA’s attitude, we assume goals to represent a person’s basic strivings. Like Deutsch (1958), we consider three goals, driving decision-making: the individualistic, cooperative, and competitive goal. The individualistic goal is defined as pure self-interest, not caring about the benefit or loss of others. The cooperative goal describes a combined interest in the well-being of the self and others. The competitive goal depicts concern for the self, while improving the relative gain compared to others. Analogous to the TRA’s subjective norm, we consider social influences in social norms. Along with the above presented taxonomy of norms, we differentiate social descriptive norm (“what most others regularly do”) and social injunctive norms (“what most others consider (in)appropriate”). Furthermore, our decision-making model includes habits, being a person’s usual behaviour. All the motivational factors (depicted on the left of Figure 1) are comprised of situational expectations and a personal value factor. We assume that personal norms affect decision-making on a higher level, influencing the importance of motivational factors. The intention is an action-specific multi-attribute utility calculation, determining the behavioural choice. The behaviour changes the situation.
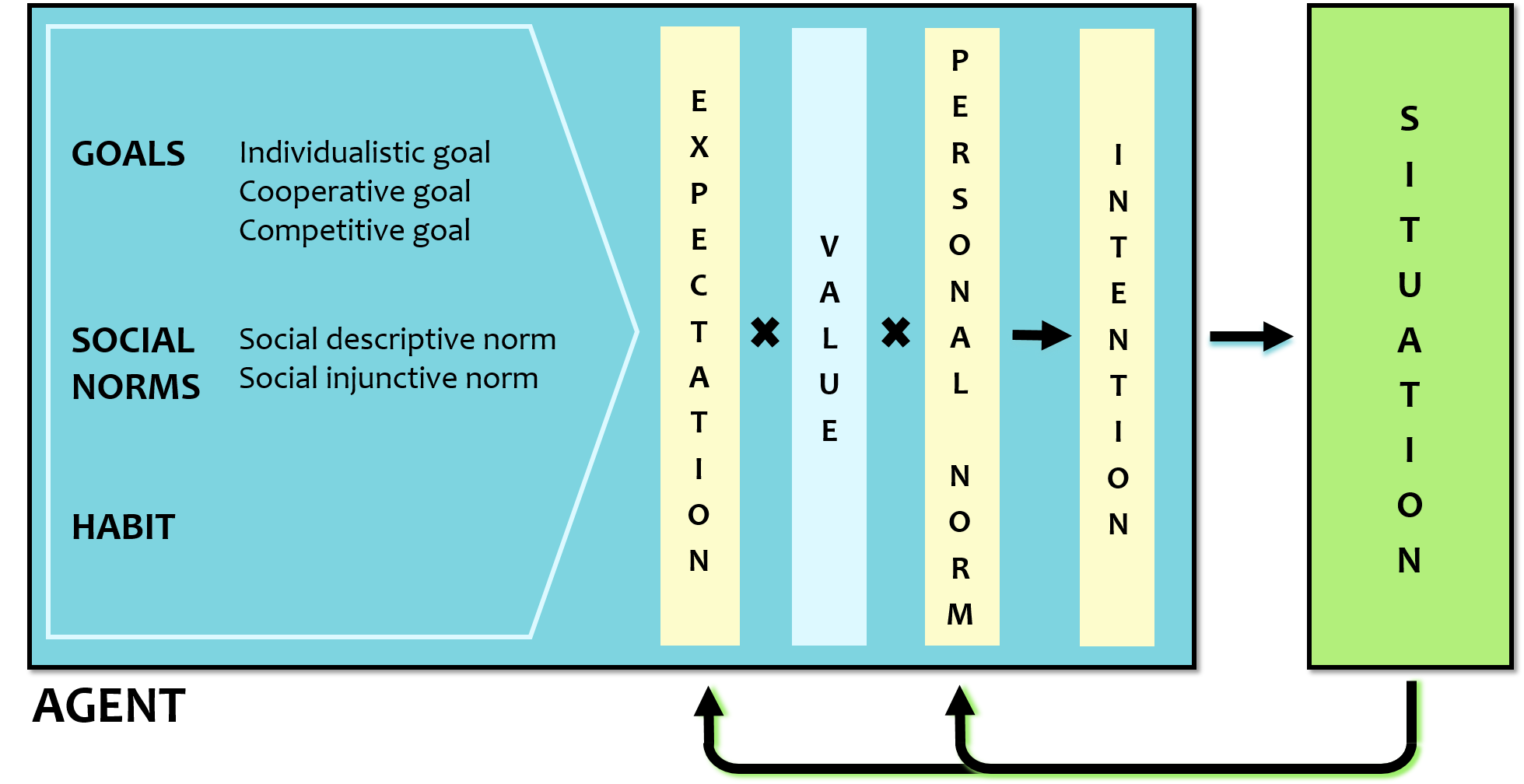
Situational consequences serve as feedback to the agent for different learning processes. We assume that learning processes differ in their speeds. On the one hand, we expect situational expectations to change in a fast adaptation process, representing an individual’s subjective perception of the situation. On the other hand, we expect the norm internalization process to be of a slow adaptation speed, storing and abstracting part of the situational learning. We assume that personal norm adaptations are the in-between of quick situational adaptations and rather stable personal values. Values represent the individual’s importance of motivational factors manifested over a long time, accounting for personality differences, which we consider static for our purposes. In the following, we present the specifics on fast adaptation of expectations, slow adaptation of personal norms (i.e., norm internalization) and personality differences in values.
Fast adaptation of expectations
Expectations are situationally adapted outcome anticipations of a specific behaviour (Bandura 2001). According to learning theory, expectations rise through repeated observations, being influenced by own experiences (i.e., reinforcement learning, Postman 1947; Sutton & Barto 2018) as well as experiences of others (i.e., observational learning, Bandura 1971, 1999). The fit of behaviour and outcome shows in stronger expectations similar to the logic of need satisfaction (Jager 2000; Kangur et al. 2017). Social descriptive norms are influenced by what most others do (Bendor & Swistak 2001; Cialdini et al. 1990; Hollander & Wu 2011). The perception of social injunctive norms is based on more complex, also societal dynamics (Andrighetto, Campenni, et al. 2010; Dannenberg et al. 2023) and will not be further discussed in this paper. Habits are affected by a person’s own behaviour (e.g., Ouellette & Wood 1998; Perugini & Bagozzi 2001) and have therefore, similar to social descriptive norms, also been conceptualized as personal descriptive norms (Batzke & Ernst 2022; Dannenberg et al. 2023).
Here, we assume that goal expectations are learned through reinforcement and observational learning. This implies that one can realize quickly whether a behaviour is useful for a specific goal. A match of goal and behaviour increases goal expectations. Social descriptive norm expectations are adapted through observing behaviour of others and habit expectations through perceiving own behaviour. We suggest that these observations are made rather quickly. Whereas social injunctive norms in a group or a society may change rapidly at times, we focus on the long periods of stability in between events of change. Therefore, we regard social injunctive norm expectations as static.
Slow adaptation: Norm internalization
We now address (1) how norm internalization proceeds and (2) how it affects decision-making, building on assumption formulated in Dannenberg et al. (2023). According to dissonance theoretical approaches, norm internalization is considered an internal reasoning process about one’s past behaviour (Bem 1967; Festinger 1957; Rozin 1999). Reviewing psychological literature revealed some key factors affecting the normative evaluation of appropriateness or inappropriateness of a chosen action. First, the process is influenced by a person’s perception of (in)appropriate behaviours in the social world (e.g., Bandura 2001; Kohlberg 1964), being represented within their social injunctive norms. Second, observations of other people’s behaviours influence internalization (e.g., Miller & Dollard 1941; Sherif & Sherif 1953), pointing to the importance of people’s social descriptive norms. Third, a person’s habitual choices affect their perception of appropriateness (cf. self-perception theory, Bem 1967, 1972). Fourth and last, the internalization process is influenced by a person’s goals, serving as feedback to the individual about how it performs in the environment, regarding its personal preferences (cf. self-determination theory, Deci & Ryan 1985; Ryan & Deci 2017).
Here, all presented factors influence norm internalization, namely: both social norms, habits, and goals, with internalization depending on their situational expectations and personal values. This makes norm internalization a more abstract process, representing an individual’s learning effect, abstracting and storing part of the situational learning. We therefore assume it to be of a slow speed. What is enough support for a behavioural decision to approve of the respective behavioural norm? We suggest that if there is more support for a behavioural decision (rather than against it), the respective personal norm is approved of (i.e., internalized as a belief of appropriateness). Otherwise, it is disapproved of (i.e., internalized as a belief of inappropriateness).
Regarding the effects of internalized norms on decision-making, motivated reasoning approaches predict that once an individual has acquired a certain belief, it searches for reasons that support it, trying to maintain an “illusion of objectivity” (Pyszczynski & Greenberg 1987 p. 302), see also Kunda (1990) and Markus & Kunda (1986). Hence, people are generally inclined to confirm a conclusion rather than to disconfirm it (cf. confirmation bias, Synder 1984). Dissonance theory states that these justification processes reduce psychologically uncomfortable cognitive dissonance, which arises when a new normative belief is formed that conflicts with existing norms or goals (Festinger 1957; Voisin & Fointiat 2013). Highlighting arguments that favour the belief or trivializing those that oppose it, reduces dissonance (Simon et al. 1995).
Here, we assume that personal norms affect decision-making by influencing the importance of motivational factors, namely social norms, goals, and habits. We suggest that a personal norm of approval highlights the importance of other norm-consistent motivational factors. Conversely, a personal norm of disapproval trivializes norm-dissonant motivational factors, making norm internalization a motivational source for dissonance reduction phenomena.
Personality differences in values
Whereas norm internalization is a universal process, it is assumed to be influenced by a person’s personality (Ryan & Deci 2017; Vygotsky 2004). Personality differences arise through personal values of motivational factors (Ajzen 1991). Research suggests that personality variables are rather stable, fundamental patterns of people (Costa & McCrae 1986; Harris et al. 2016; Terracciano et al. 2010). Although they may change over long periods of time, we consider personal values as static, since our research focus lies on norm internalization and personality’s influence on it rather than personality change. To reduce possible combinations of different personal values for the model, we developed seven psychologically plausible personality types. The types are ordered along their cooperativeness, ranging from strong cooperators (types 1 and 2) to strong defectors (types 6 and 7), with more internally conflicted and socially sensible conditional cooperators in between (types 3 to 5). Relevant psychological literature for developing these types and their description is presented in the Appendix.
The Scenario: A Social Dilemma Game
Generally, we assume that norm internalization occurs in every situation. However, many societal challenges arise from people acting selfishly in interdependent and mixed-motive situations, resulting in collective costs. Numerous authors have addressed the issues of how and when individuals act pro-socially and cooperation collectively emerges (e.g., Axelrod 1986; Nowak et al. 2004). One of the most promising factors for long-term behavioural change is motivational change (Otto & Kaiser 2014). Therefore, we were particularly interested in investigating the role of norm internalization in those situations, in which a selfish action has to be refrained from in favour of a collectively advantageous action. What makes decisions in these situations so difficult is that they are made against the backdrop of a perceived conflict, putting individual’s and collective’s interests at odds and interdependent of each other (Dawes 1980). The most basic game-theoretical model that reflects this arguably very common social conflict is the prisoner’s dilemma game (PDG, Luce & Raiffa 1957). Thus, each player has only two behavioural options: cooperation and defection. The conflict is described within the properties that a person receives a higher payoff for a socially defecting choice; however, all individuals in the society are better off, if all cooperate rather than defect (Axelrod 1984).
As previously mentioned, we here focused on agent dynamics. We aimed to minimize dynamics based on interactions between scenario and agents by limiting complexity in the decision scenario. This allowed us to attribute resulting dynamics to agents. Therefore, we chose one of the simplest game-theoretical scenarios for a first implementation of our conceptual model: an iterated 3-person PDG. In the 3-person game, we are already studying a group, which employs a different logic than the 2-person game (Dawes 1980). In future model extensions, the number of agents can easily be increased, and the same logic still applies. In the game, each time step an agent \(i \in I = \{1, 2, 3\}\) chooses between two behavioural actions \(a_{i} \in \{0, 1\}\): cooperation \(a_{i} = 1\), representing the pro-social choice, and defection \(a_{i} = 0\), representing the egoistic choice. Cooperation is beneficial to every individual. Hence, everyone receives benefits \(b\) when individual i cooperates. However, cooperation comes with a cost \(c\) for the cooperating individual. The individual’s payoff (\(P_{i}\)) is a function of its action and the actions of the others given by:
\[\label{equation1} P_{i} = 1 + (a_{1} + a_{2} + a_{3}) b - a_{1} c \; \; with \; 3b > c > b >0\] | \[(1)\] |
Number of cooperators | Payoff to defectors | Payoff to cooperators | Collective payoff |
---|---|---|---|
3 | - | 2 | 6 |
2 | 3 | 1 | 5 |
1 | 2 | 0 | 4 |
0 | 1 | - | 3 |
The Agent-Based DINO Model
In the following, the agent-based DINO model, Dynamics of Internalization and Dissemination of Norms, is presented. The model was programmed in NetLogo (Wilensky 1999) version 6.2.2.
Agent’s decision-making
Figure 2 illustrates agents’ decision-making. Motivational factors are depicted in the rows. Each motivational factor is represented by an expectation and a value factor. Agents’ dynamic expectations express the situational fit of a motivational factor and a specific action. An exception is the social injunctive norm expectation, which we consider as static. Agents’ static values ascribe importance to a motivational factor. Values are set depending on agent type. Personal norms are dynamically internalized, influencing the importance of motivational factors. Agents use a weighted multi-attribute subjective utility matrix to calculate the intention to show an action as described in function (2). Agents perform the action with the highest intention. The model is purely deterministic.
\[\label{equation2} \begin{split} I_{i,t} = IND_{i,t} \times v_{IND,i} \times PN_{i,t} + COOP_{i,t} \times v_{COOP,i} \times PN_{i,t} + \\ COMP_{i,t} \times v_{COMP,i} \times PN_{i,t} + SDN_{i,t} \times v_{SDN,i} \times PN_{i,t} + \\ SIN_{i,t} \times v_{SIN,i} \times PN_{i,t} + HA_{i,t} \times v_{HA,i} \times PN_{i,t} \end{split}\] | \[(2)\] |
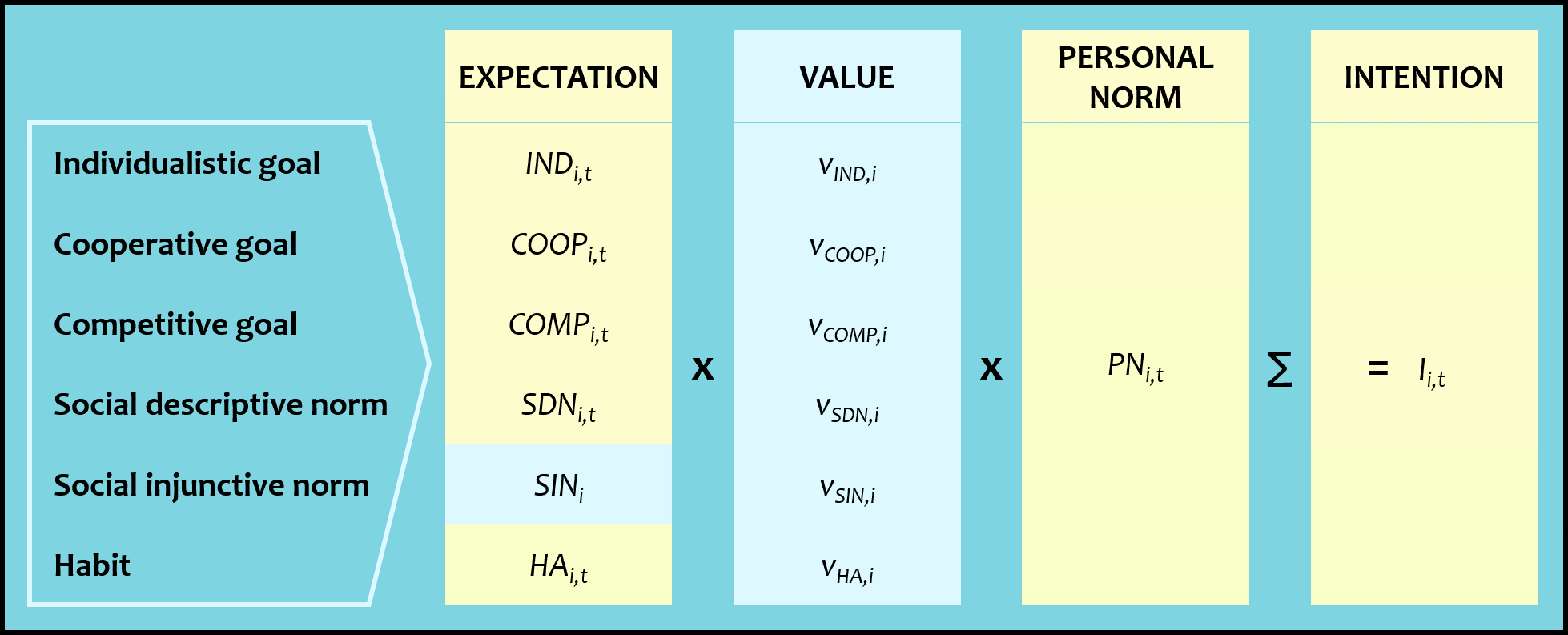
Perception, knowledge, and memory
As can be expected in the 3-person PDG, we assumed that agents have knowledge about the payoff matrix and thus know the minimum and maximum achievable individual and collective payoffs. Moreover, agents can observe the other agents’ actions and remember their own and the others’ payoffs from the previous round.
Adaptation of expectations
We now present the adaptation processes underlying expectations. Expectations are action-specific: one motivational factor is represented in two expectations, one for each action, that is, cooperation or defection.
Goal expectations
Goal expectations determine how promising an agent considers an action in order to achieve a specific goal. Agents evaluate their goal expectation in two successive processes: reinforcement and observational learning. In reinforcement learning, only the goal expectations of the action an agent has taken before are positively or negatively reinforced. After each action an agent evaluates whether it was useful for goal achievement given the circumstances. For that, an agent uses its knowledge about the game. Goal expectations are adapted through subtracting or adding a relative amount of the expectation-change-rate (see section on parametrization). The three goals are evaluated according to the following rules.
- An agent considers the individualistic goal as achieved, if either the individual payoff peaked (\(P_{i,t}\) = [maximum individual payoff]) or improved compared to the last round (\(P_{i,t} > P_{i,t-1}\)). If either of the two conditions apply, the individualistic goal expectation of the last action increases; otherwise, it decreases. The degree of strengthening or weakening is relative to the goal (non-)achievement: it depends on the achieved amount of the individual payoff in relation to the maximum achievable individual payoff (i.e., change in individualistic goal expectation = \(P_{i,t}\) / [maximum individual payoff] \(\times expectation-change-rate\)).
- An agent considers the cooperative goal as met, if either the collective payoff peaked (\(P_{c,t}\) = [maximum collective payoff]) or improved compared to the last round (\(P_{c,t} > P_{c,t-1}\)). Again, the degree of strengthening or weakening depends on the achieved collective payoff in relation to the maximum achievable collective payoff (i.e., change in cooperative goal expectation = \(P_{c,t}\) / [maximum collective payoff] \(\times expectation-change-rate\)).
- The competitive goal answers to the question whether the agent’s individual payoff is higher than the individual payoff of at least one other agent \(j\) ([\(P_{i,t} > P_{j,t}\)] \(\geq 1\)). In case the condition is met, the relative increase of the competitive goal expectation is defined as the number of outperformed others (i.e., increase in competitive goal expectation = [\(P_{i,t} > P_{j,t}\)] / [total number of other agents] \(\times expectation-change-rate\)). In case the condition is not met, the relative decrease depends on the number of others, having outperformed the agent (i.e., decrease in competitive goal expectation = [\(P_{i,t}\leq P_{j,t}\)] / [total number of other agents] \(\times expectation-change-rate\)).
Observational learning is based on observing the other agents’ actions and adapting goal expectations according to their behavioural consequences. Analogous to the rules of reinforcement learning, an agent evaluates the other agents’ actions and their consequences and adapts its goal expectations accordingly. Compared to reinforcement learning, observational learning is of a minor importance, with adaptations being one fifth as large (Ernst 2003).
Social descriptive norm expectations
Social descriptive norm expectations convey information regarding how unequivocally a behaviour matches a social descriptive norm. Due to having only two behavioural alternatives in the PDG, we assumed that in this specific scenario the two expectations of social descriptive norms are negatively associated. Thus, if an agent observes one behaviour, it also notices the absence of the other. Hence, agents update both expectations at each time step 1. Whereas this seems redundant in the PDG, the DINO model was designed to also fit more complex interdependence structures, where norm information regarding one behaviour cannot necessarily be derived from other behaviours. Expectations were adapted according to the following condition:
- If the majority of agents (\(\geq 2\)) cooperated, the cooperative social descriptive norm expectation increases by the \(expectation-change-rate\) and the defective counterpart decreases accordingly (vice versa, if the majority of agents defected).
Habit expectations
Habit expectations express the fit of an action with a behavioural habit. Analogous to social descriptive norms, the two habit expectations are interdependent and adapted according to the following condition:
- If the agent cooperated, the cooperative habit expectation increases by the \(expectation-change-rate\) and the defective counterpart decreases accordingly (vice versa, if the agent defected).
Adaptation of personal norms and their influence on decision-making
As presented in our norm taxonomy, we assumed that agents have two personal norms, one for each action. Norm internalization is an agent’s normative judgement regarding the (in)appropriateness of the chosen action and the following approval or disapproval of the according personal norm. To make the judgement, an agent takes its three goals, descriptive and injunctive social norms, and habits into account. All these motivational factors are collected in agents’ intentions. Therefore, agents evaluate the strength of their intention of the last action through dividing it by the maximum intention that could be achieved regarding their personal values. The maximum achievable intention equals the sum of agents’ values. The multiplication of values by expectations is unnecessary since their maximum is 1. If the reasons in favour of a behaviour outweigh the ones against, the personal norm corresponding to the last action is approved of and increases by the \(internalization-change-rate\) (see section on parametrization). Otherwise, it is disapproved of and decreases as described in the following adaptation rule:
\[\label{equation3} \begin{split} if \; [I_{i,t} / (v_{IND,i} + v_{COOP,i} + v_{COMP,I} + v_{SDN,i} + v_{SIN,i} + v_{HA,i}) ] > 0.5 \\ then \; PN_{i,t} + internalization-change-rate \\ otherwise \; PN_{i,t} - internalization-change-rate \end{split}\] | \[(3)\] |
Personal norms are a multiplier for each motivational factor in the decision-making calculation. That way, personal norms reinforce norm-consistent and inhibit norm-inconsistent motivational factors. More precisely, agents check which action a motivational factor supports best, through comparing the two action-specific expectations of a motivational factor. The personal norm, which supports the same action, is multiplied by value and expectation of a motivational factor. A personal norm of approval (\(PN_{i,t} > 1\)) strengthens a motivational factor that favours a behaviour. Conversely, a personal norm of disapproval (\(PN_{i,t} < 1\)) weakens a motivational factor that favours a behaviour. Taking the individualistic goal as an example, personal norms affect the influence of all motivational factors in decision-making according to the following rule:
\[\label{equation4} \begin{split} if \; IND_{C,i,t} > IND_{D,i,t} \\ then \; IND_{i,t} \times v_{IND,i} \times PN_{C,i,t} \\ otherwise \; IND_{i,t} \times v_{IND,i} \times PN_{D,i,t} \end{split}\] | \[(4)\] |
Agent heterogeneity in values: Seven agent types
Table 2 illustrates the implementation of the seven agent types, which is based on (1) the presented literature (see the Appendix), (2) the authors’ psychological expertise, and (3), whenever (1) and (2) did not give a clear decision, the matching of the resulting agent’s behaviour with the personality type. As presented in the conceptual model, DINO agents possess six motivational factors, shown in the rows of Table 2. Agent types differed in their values, shown in the cells, defining the importance of motivational factors.
Agent Types | |||||||
Cooperators | Conditional cooperators | Defectors | |||||
Type 1 | Type 2 | Type 3 | Type 4 | Type 5 | Type 6 | Type 7 | |
individualistic goal | 0 | 1 | 1 | 2 | 2 | 3 | 1 |
cooperative goal | 3 | 3 | 2 | 2 | 1 | 0 | 0 |
competitive goal | 0 | 0 | 1 | 2 | 2 | 1 | 3 |
social descriptive norm | 0 | 2 | 2 | 2 | 2 | 1 | 0 |
social injunctive norm | 1 | 3 | 2 | 1 | 3 | 1 | 0 |
habit | 3 | 2 | 0 | 0 | 0 | 1 | 3 |
Parametrization, initialization, and model execution
All expectations varied between [0-1]. Higher values indicate a better fit of a motivational factor and a specific action. They were initialized to a neutral midpoint of 0.5. Agents’ personal values ranged between [0-3] and were initialized depending on agent type (see Table 2). Personal norms ranged from [0-2] and were initialized with 1. Social injunctive norm expectations were static, being set to slightly support cooperative behaviour with 0.6 for the cooperative and 0.4 for the defective social injunctive norm expectation.
As presented in our conceptual model of norm-based decision-making, the different adaptation processes differed in their speeds. First, we assumed that goal expectations, social descriptive norm expectations and habit expectations are adapted in a fast adaptation process. The according \(expectation-change-rate\) was set to 0.2, making expectations adaptable from one extreme to the other within about five rounds of the game. Second, we assumed that personal norms are adapted in a slow adaptation process. We conducted a sensitivity analysis for the \(internalization-change-rate\), keeping the \(expectation-change-rate\) stable at 0.2 (see Appendix). The model showed relatively stable results for change rates between 0.01 and 0.07. We set the \(internalization-change-rate\) to 0.02, allowing personal norms to change from full norm approval to full norm disapproval within 100 rounds of the game.
In each time step, agents first calculate their intentions for each action and then act on the strongest one. Agents observe the actions of others and receive their payoff. Next, agents adapt their goal expectations through first reinforcement learning and second observational learning. Then, social descriptive norm and habit expectations are adapted. Lastly, agents adapt their personal norms through norm internalization and update their memory concerning payoffs. Model execution was terminated after 200 time steps, as this is a period in which lock-in phenomena have occurred and agents’ internal dynamics have stabilized in most model runs. The first five time steps were excluded from the analyses.
Experiments and Results
We now address our two research questions. First, we investigated which conditions facilitate norm internalization, analysing which agents internalize what norm under which circumstances. Second, we examined the effects of norm internalization by manipulating agents’ personal norms. In the present work, we studied norm internalization as an additional influence in decision-making over and above the ones of other normative and non-normative behavioural drivers. As a proof-of-concept simulation, we additionally tested its independent effects by comparing the model with norm internalization to the model without. Results are presented and discussed in the Appendix.
Personal norms to cooperate and to defect are hereafter called C-PN and D-PN, both of which agents internalize (i.e., approve of or disapprove of). A model run relates to a specific group composition of three agents playing the 3-person PDG. Since the model is purely deterministic, no repetitions of the same model runs were conducted. Generally, we analysed 84 different group compositions, because the seven agent types can form 84 different groups of three.
Which conditions facilitate norm internalization?
Figures 3 and 4 illustrate agent dynamics of norm internalization, depending on agent type, type of norm (C-PN or D-PN), and group composition. Norm internalization is shown as the absolute value of the personal norm of one agent at a time step (0-200), ranging from norm approval (in turquoise), across indifference (in white) to norm disapproval (in purple). Group compositions are shown as three-digit numbers, indicating the three agent types in the group (e.g., group composition “123” \(=\) agent types 1, 2, and 3), ordered along group cooperativeness.
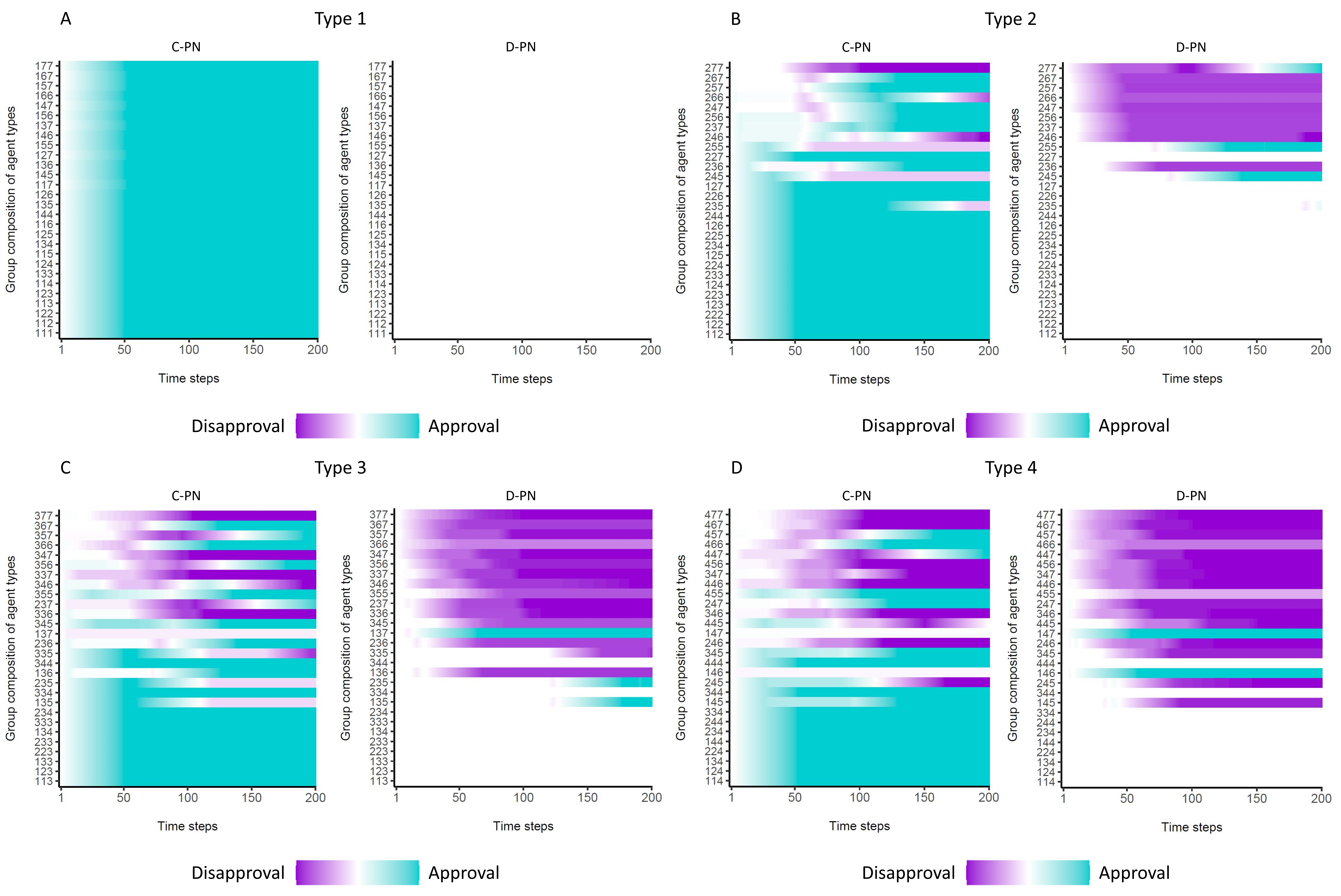
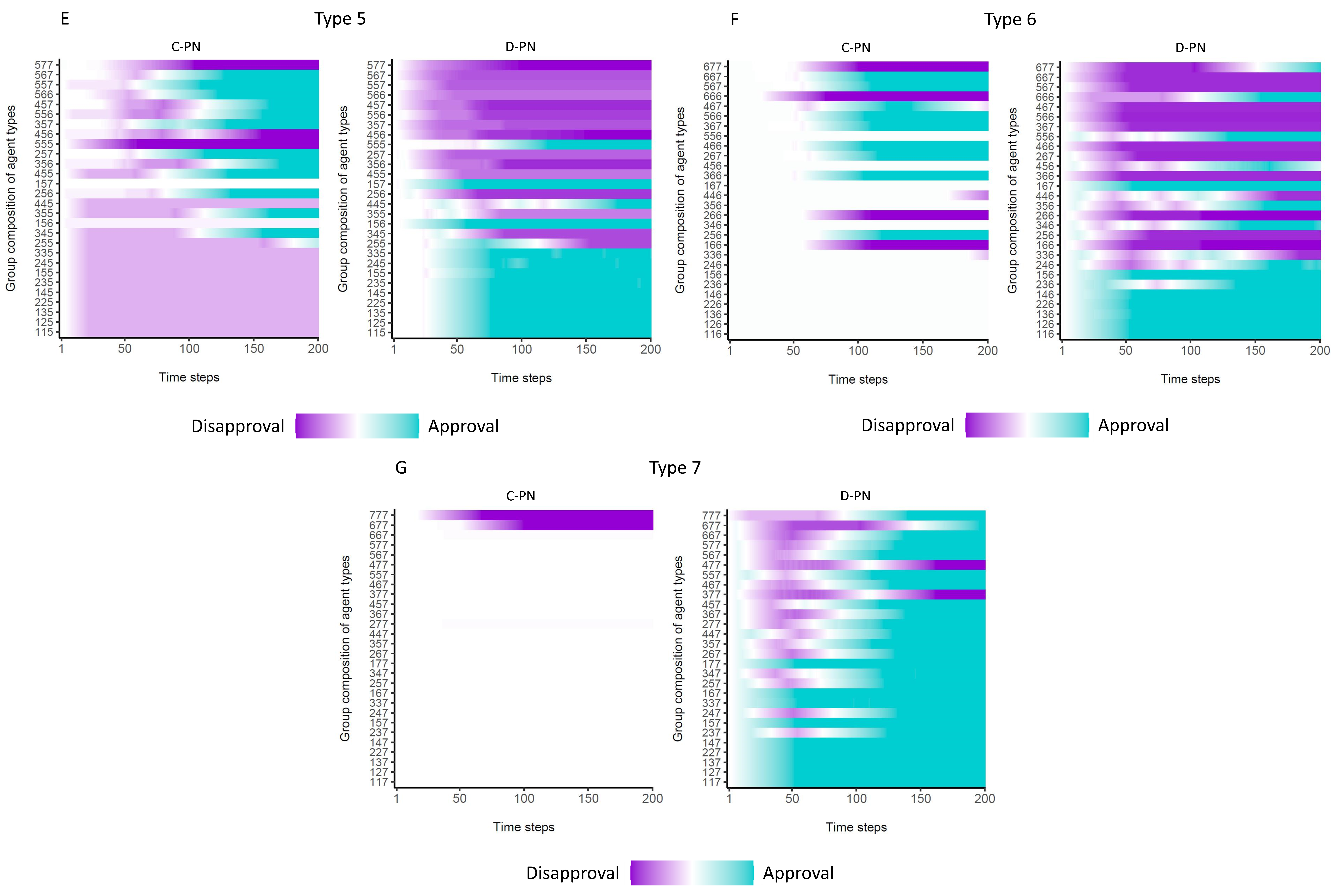
Generally, all agent types might at least develop one personal norm of approval. Highly intrinsically driven agents (i.e., types 1 and 7) approved of the norm according to the behaviour they inherently prefer under (almost) all circumstances (see Figures 3A and 4G). The other agent types (i.e., types 2 – 6) might temporarily approve and disapprove of both norms, depending on the group composition (see Figures 3B-D and 4E-F). Agent types 2 – 4 approved of the C-PN in the majority of group compositions (see Figures 3B-D), types 5 and 6 approved of the C-PN or D-PN in roughly equal parts (see Figures 4E-F), and agent type 7 mostly approved of the D-PN (see Figure 4G). This supports Hypothesis 1a that cooperative agents tend to approve of the C-PN and defective agents tend to approve of the D-PN.
For norm approval, a cooperative setting was generally beneficial. It allowed rather cooperative agents to achieve their cooperative goal when cooperating. Interestingly, it also allowed defecting agents to be better than others (satisfying their competitive goal) or to accumulate payoff (individualistic goal) through defection. Achieving goals and complying with norms leads DINO agents to approve of a norm. More defective settings made the norm internalization process longer, in which one or both personal norm(s) were approved or disapproved of before a stable state was reached. This especially applied to conditional cooperators.
For example, in highly heterogeneous settings consisting of a cooperator and a defector, conditional cooperators tended to (partly) disapprove of a one norm before approving of the other (see Figures 3C-D and 4E). This often ended in D-PN approval. While this phenomenon was especially typical to conditional cooperators, it was observable in many agent types (2 – 7). For conditional cooperator types 3 and 4, playing with another (similar or more cooperative) conditional cooperator and a defector resulted in disapproval of both norms (see Figures 3C-D). For types 5 and 6, however, norm internalization in these settings resulted in C-PN approval (see Figures 4E-F). Too cooperative settings led agent types 5 and 6 to approve of the D-PN and types 3 and 4 of the C-PN. Highly defective settings led all conditional cooperators to disapprove of both C-PN and D-PN. Hence, Hypothesis 1b was only partly supported. While cooperative settings did facilitate approval of the C-PN, they facilitated approval of any norm, and defective settings did not facilitate approval of the D-PN.
What are the effects of norm internalization?
To examine the effects of norm internalization, we conducted two series of experiments. In both, we varied the degree to which agents have internalized personal norms. First, we manipulated one norm, keeping the other constant. This shows the effects of having internalized a certain norm depending on the group composition. Second, we varied both norms independently from each other, representing aggregated results across group compositions, showing the effects of internalizing multiple, potentially conflicting norms. To address our hypotheses, we looked at three different outcome variables: cooperation, behavioural changes, and payoff inequality.
Figure 5 shows the effects of agents having internalized a certain norm, the C-PN, to varying degrees, depending on group composition of agent types. Group compositions are again ordered along group cooperativeness, defined by the cooperativeness of the single agents. In the beginning of a model run, we once manipulated agents’ personal norms within their boundary values ranging from full disapproval to full approval. In between full approval and disapproval manipulations, manipulation strengths decrease towards the framed “Baseline”, showing the standard model runs in which no manipulations were conducted. Outcomes were aggregated across agents and time.
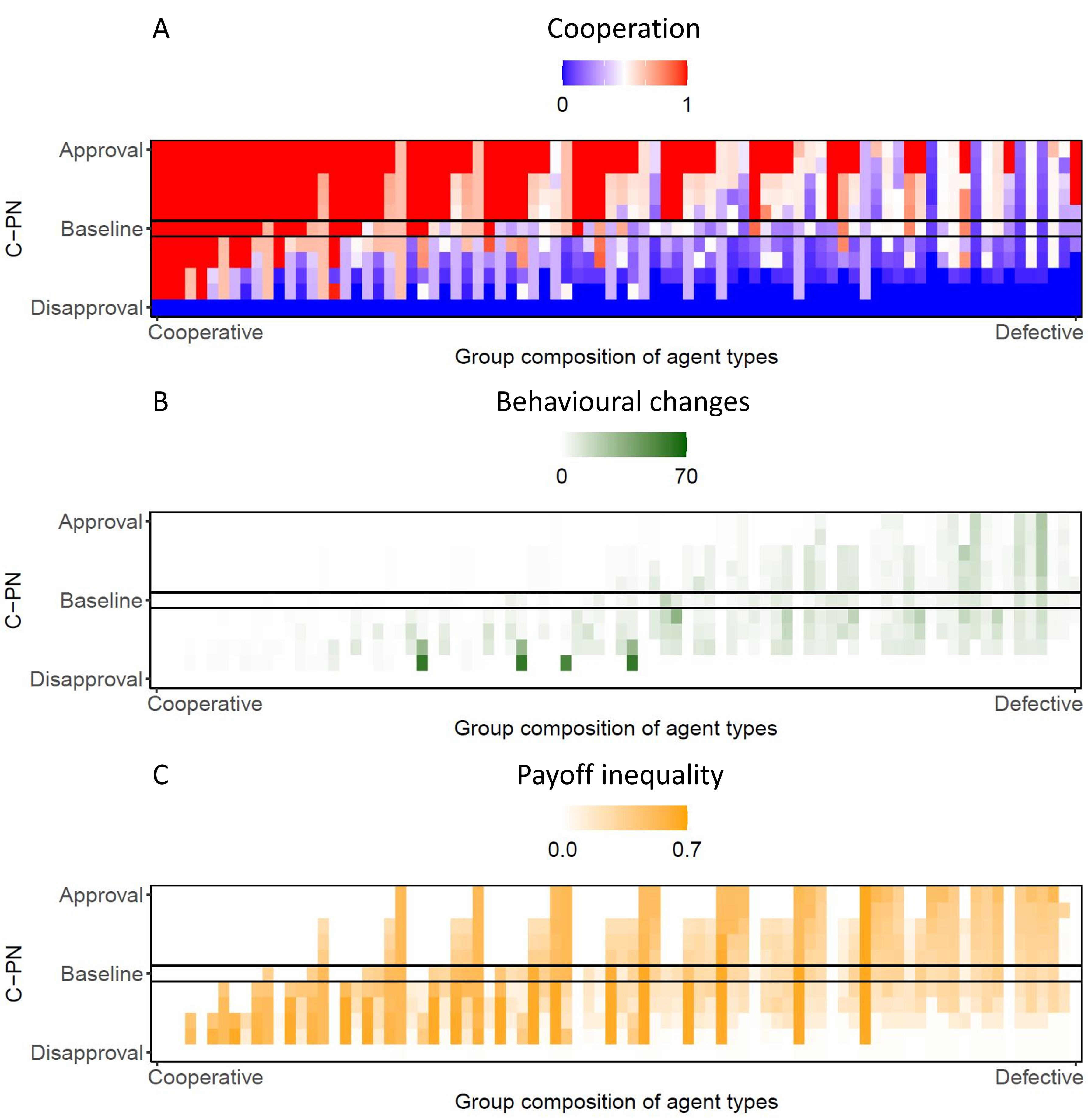
Figure 5A shows that agents’ cooperation increased with stronger approval of the C-PN and decreased with its disapproval compared to the baseline model runs, supporting Hypothesis 2a. In rather cooperative group compositions, slight manipulations towards approval of the C-PN led to pure cooperation of all agents. Strong manipulations achieved the same result even in more defective group compositions. Although approval of the C-PN increased average cooperation in all group compositions, some groups of agents were not tipped over to pure cooperation. Conversely, full disapproval of the C-PN led to pure defection in all group compositions, even those consisting of solely cooperators. Figure 5B indicates that high numbers of behavioural changes were generally associated with defective group compositions as well as (partial) disapproval of the C-PN. A similar pattern was found in Figure 5C regarding payoff inequality, whereas inequality increased especially with C-PN disapproval in mixed, heterogeneous groups. In case of full disapproval of the C-PN, behavioural changes disappeared, and equality was established due to pure defection of all agents, supporting Hypotheses 2b and 2c. The effects of full norm approval partly supported the hypotheses as well, but effects were less univocal.
To investigate the effects of having internalized both, potentially conflicting personal norms, we varied C-PN and D-PN independently. Figure 6 shows the effects of full disapproval to full approval of both norms regarding cooperation, behavioural changes, and payoff inequality. Again, disapproval and approval manipulations decrease towards “Baseline” model runs without manipulation. Results were aggregated across agents, time, and group compositions.
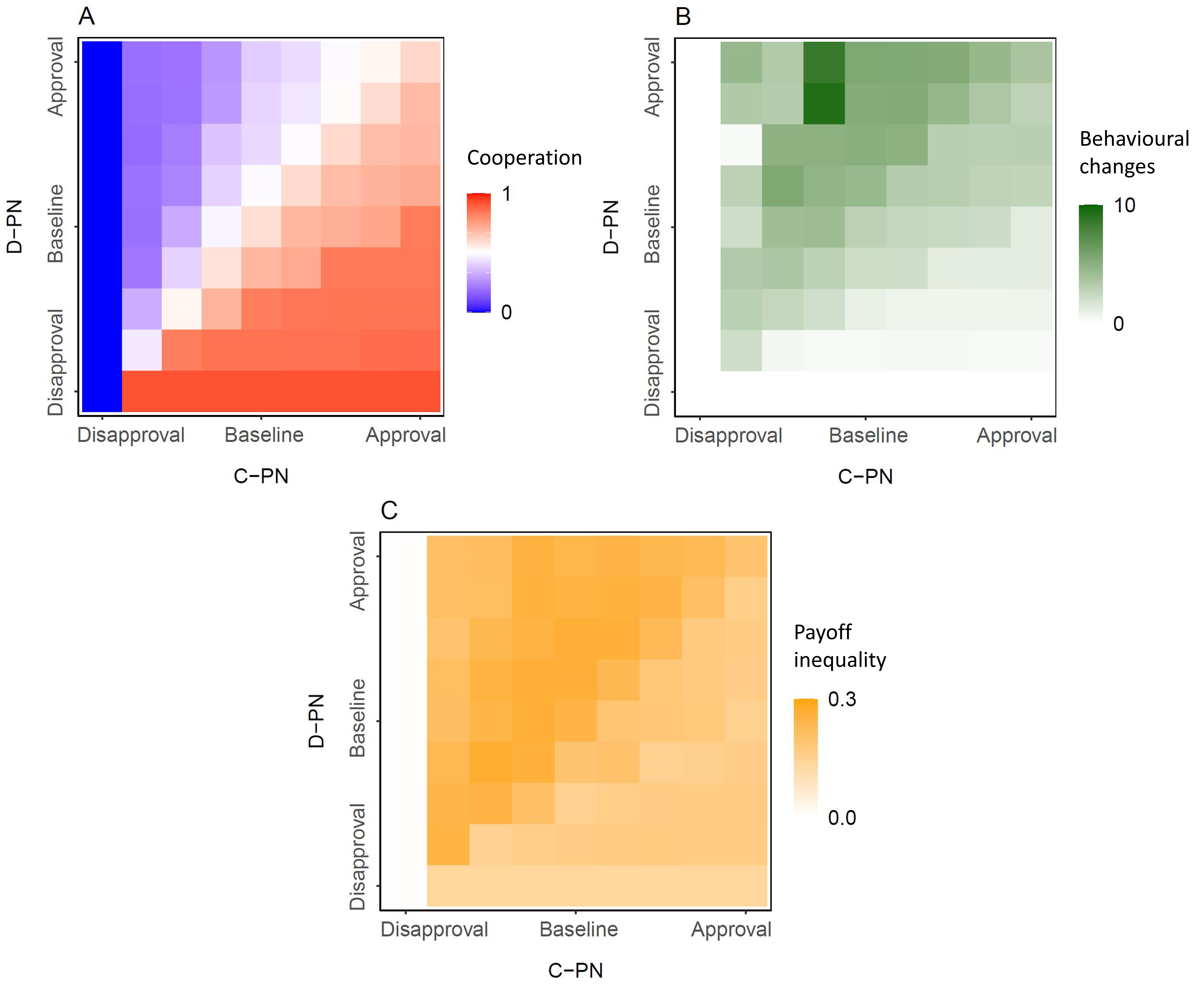
Figure 6A shows that norm approval and disapproval were generally behaviourally effective, which again supported Hypothesis 2a. However, it also shows that a personal norm of disapproval had even stronger behavioural effects than a norm of approval. Full disapproval of the C-PN led to pure defection. As a result, behavioural changes and payoff inequality disappeared (see Figures 6B and 6C), supporting Hypotheses 2b and 2c. Full disapproval of the D-PN led to cooperation in large parts, however, not pure cooperation. The diagonal from the lower left corner (full disapproval of both personal norms) to the upper right corner (full approval of both personal norms) indicates that equally strong norm disapproval was associated with defection. Equally strong norm approval did not affect cooperation, behavioural changes (see Figure 6B), and payoff inequality (see Figure 6C). Dominance of the C-PN over the D-PN tended to decrease behavioural changes and payoff inequality, which supports Hypotheses 2b and 2c. Contrary to the hypotheses, dominance of the D-PN tended to increase behavioural changes and payoff inequality.
Discussion
The aim of our research was to provide the next step towards understanding the dynamics of norm internalization better. Based on psychological literature, we developed a conceptual model of norm-based decision-making, made assumptions regarding the mechanisms of norm internalization, and implemented both into the agent-based DINO model. In the conceptual model, personal norms, being the product of norm internalization, are regarded as an additional motivational force in decision-making over and above the situational expectations and personal values of goals, habits, and social norms. Thus, the model accounts for inter-individual differences in norm internalization. The conceptualization of personal norms introduces a new level of complexity, being behaviour-specific rules. This implies the existence of a personal norm for each behavioural alternative. In the DINO model, they may develop independently from each other, while each personal norm may be approved of, representing a normative belief of appropriateness, or disapproved of, representing a normative belief of inappropriateness. In the present work, we tested the model regarding facilitating conditions and independent effects of norm internalization.
Conditions of norm internalization
A cooperative setting was generally beneficial to develop a personal norm of approval, which partly supports Hypothesis 1b. Cooperative settings allowed cooperators and conditional cooperators to approve of the norm to cooperate (as predicted). However, they also allowed defectors to approve of the defection norm. In highly defective settings, agents tended to disapprove of both norms. Hence, for norm approval most agents were dependent on the cooperation of others. In line with Hypothesis 1a, cooperators mostly approved of the cooperation norm and defectors of the defection norm. These highly intrinsically driven agents mostly approved of the norm they inherently preferred even in defective settings.
Conditional cooperators were more flexible regarding which norm they internalized. Empirical studies showed that conditional cooperators are highly influenced by the social setting (e.g., Burlando & Guala 2005; Kurzban & Houser 2005), increasing cooperation in cooperative settings (Fischbacher & Gächter 2010) and decreasing it in the presence of defectors (de Oliveira et al. 2015). This strongly relates to the DINO agent types 3 and 4. They approved of the cooperation norm in cooperative settings, which was associated with cooperation, and disapproved of both norms in more defective settings, associated with defection (see the Appendix). The model also replicated the empirical finding of conditional cooperators siding with the defector in highly heterogeneous settings (Hartig et al. 2015; Lucas et al. 2014), showing that this behaviour goes along with approval of the defection norm.
Relating to more individualistic and competitive DINO agent types 5 and 6, Fischbacher and colleagues (2001) found a behavioural pattern in 14% of participants characterized by conditional cooperation and a decay of cooperation above a certain contribution level of other players; a pattern they called “hump-shaped”. In the DINO model, these types approved of the defection norm in more cooperative settings. However, when playing with a defector and a conditional cooperator (rather than a pure cooperator), they eventually started cooperating and internalizing accordingly. It prevented them from following through on their individualistic and competitive goals, making it difficult to exploit each other. Similarly, Gächter & Thöni (2005) reported that their less cooperative subjects cooperated more when playing with similar others. They concluded that when “there are no cooperators around to free ride on […] they understand that they need to cooperate among themselves if they want to earn money.” (pp. 310–311). The DINO model showed that defective conditional cooperators’ approval of the cooperation norm is facilitated by other conditional cooperators when defectors are present. On the contrary, this facilitated disapproval of both norms in more cooperative conditional cooperators (types 3 and 4). These types were dependent on a cooperative setting to approve of the cooperation norm.
Interestingly, conditional cooperators as well as type 6 generally approved of the cooperation norm in many group compositions. Regarding, for instance, the balanced goal structure of type 4 or the strong individualistic goal of type 6, this result was rather surprising. It suggests that approval of the cooperation norm is achievable for many types with different goal structures. This relates to empirical evidence that cooperative behaviour, such as pro-environmental behaviour, may result from different motivations including environmental and economic concerns (Brandon & Lewis 1999; Thøgersen 2003). Moreover, experimental research showed that even people that are predominantly motivated by gain rarely act completely egoistically (Camerer 2003).
The DINO model also suggests that the relative importance of personal norms matters. In ambiguous settings and generally in conditional cooperators, agents’ motivations were often too ambiguous for developing any norm of approval. As norm internalization was modelled as a motivational source for dissonance reduction phenomena, disapproval of one norm reduced agents’ internal conflicts and consequently could facilitate approving of another norm. This finding relates to Lindenberg & Steg (2007) goal-framing theory, wherein the authors argued for the importance of the relation between multiple goals. Since in the DINO model personal norms influence goal importance, one may assume that not only the relative importance of goals but also of personal norms is of significance.
Effects of norm internalization
In the DINO model, agents’ behaviour was generally associated with approval of the according norm, supporting the idea formulated in Hypothesis 2a that norm internalization increases norm compliance (Andrighetto et al. 2010; Axelrod 1986; Deci & Ryan 2000; Gintis 2004). The model also showed that norm approval is only behaviourally effective in those agents that are not overly disinclined towards the behaviour. For agents that are highly intrinsically driven, norm disapproval was more effective in achieving behavioural change. Hence, the motivational strengthening via norm approval of an inherently undesirable action did not exceed the motivational support that an inherently desirable action had in these agents. For example, to make defectors cooperate, disapproving of the defection norm was more effective than approving of the cooperation norm. This is an interesting point, relating to prospect theory (Tversky & Kahneman 1992). Therein the authors suggest that people give more weight to avoiding something undesirable than to achieving something desirable. Similarly, recent socio-political developments suggest the power of norm disapproval, such as the 2017 emerging Swedish “flight shame” movement to reduce flying drastically lowered the number of aircraft passengers in Sweden.
However, norm-based intervention studies predominantly focus on norm approval, often not testing the effects of norm disapproval (e.g., Hamann et al. 2015; Terrier & Marfaing 2015). As has been proposed before (Schwartz & Fleishman 1982), our results suggest that the effects of norm disapproval are potentially underestimated and might be worth further investigating. In case of conflict between the two personal norms, the model showed that disapproval of both tends to be associated with defection. This seems plausible with defection being the dominant strategy in the PDG (Dawes 1980). Equally strong norms of approval did not affect overall cooperation, supporting again to the idea that the relative importance of personal norms matters for behavioural decisions (Lindenberg & Steg 2007).
The DINO model presented mixed results for Hypotheses 2b and 2c, showing that norm internalization may increase or decrease behavioural inflexibility and payoff equality. The hypothesized effects of norm internalization increasing inflexibility and payoff equality held true for collective approval of the cooperation norm and disapproval of the defection norm. Hence, the model supports the idea that internalization has the potential to make agents more persistent (Andrighetto et al. 2010). Moreover, it illustrated that persistence is limited to the behavioural option that is collectively beneficial. In these cases, norm internalization resulted in agents developing strong habits, making the two difficult to differentiate on a behavioural level, which relates to Epstein (2006) assumed connection of internalization and habit formation.
However, approval of the defection norm increased behavioural flexibility and payoff inequality. As Bendor & Swistak (2001) have famously demonstrated, pure defection is an unstable state. The DINO model suggests that the instability of defection is related to collective approval of the defection norm or disapproval of the cooperation norm. Norm disapproval may have counterintuitive effects on agents, making them more flexible and adaptive (see the Appendix), which shows their dissimilarity from habits. Very strong norm disapproval again effectively promoted behavioural consensus and thus stability and equality. Similarly, research has shown that social norms may have diverse and contradictory effects (see the Appendix), also regarding social inequality (Conte & Castelfranchi 1995; Saam & Harrer 1999; Ullmann-Margalit 1977). The DINO model showed that equality can be fostered through collective internalization of the same norm except for approval of the defection norm.
Limitations and future research
This work has a number of limitations, offering various leverage points for future research. The DINO model was implemented within the framework of an iterated 3-person PDG. Real-world situations are often characterized by more than two behavioural alternatives, larger and dynamic groups, changing payoff matrices, outcome uncertainty, et cetera. The focus of our work was to advance the cognitive representation of internalized norms in agent-based models. We considered it important to account for multiple personal norms, effects of their approval and disapproval as well as personality differences, in order to better understand norm internalization processes.
For an initial implementation of these aspects, we tried to limit complexity in the situational framework. Our choice fell on the PDG, describing the core of a conflict that is common to many situations (Dawes 1980) and that we consider particularly interesting for studying norm internalization. Compared to the 2-person game, the 3-person game entails a different logic (Dawes 1980), allowing to easily increase the agent number later-on. In the 3-person game, emerging norms are specific for the small group context. In larger groups, one would expect that subgroups may emerge and develop their own social norms – a process that is not represented so far.
In developing our conceptual model, we aimed for principles and structures that also fit a more complex game with more behavioural alternatives, such as a commons dilemma (Ernst 2010; Hardin 1968; Ostrom et al. 2002). Applying the conceptual model to different behavioural domains may require different/additional goals as Jager (2000) similarly described for needs. It remains for future research to investigate norm internalization in larger, dynamic groups, test the effects of multiple group memberships, and take outcome uncertainty into account. Moreover, effects of situational influences through norm interventions, changes in the payoff matrix, et cetera, are promising approaches for influencing norm internalization and should further be investigated.
Whereas the developed conceptual model of norm-based decision-making is an extension of the frequently used theory of reasoned action, it still simplifies in various aspects. For instance, it does not consider all psychological factors that have been proven to significantly influence people’s behaviour, such as values in the sense of higher-order beliefs (Schwartz 1977b). Psychological constructs sometimes lack a clear definition and differentiation with concepts even being used interchangeably. To improve (dynamic) theories of decision-making, further research is needed investigating the distinct mechanisms and independent effects of motivational factors – something social simulation can greatly contribute to (Jager 2017; Jager & Ernst 2017).
Furthermore, the model so far considers only one process in decision-making, being a subjective utility-maximizing decision calculation in the sense of deliberation. Scholars have argued for the existence of two distinct processes (cf. dual-process theories, Kahneman 2003; Petty & Cacioppo 1986) with the second being a fast and intuitive decision process. Implementing the conceptual model into a more complex game with more situational information will facilitate including heuristic shortcuts that agents use when specific situational cues appear (Gigerenzer 2001). Additionally, we simplified by considering social injunctive norms and personal values as static. To better understand the implications of norm internalization on the dynamics of social norms, it is an important step to endogenize social injunctive norms. Including personal value change would enable the study of norm internalization in the larger context of personality development.
There is a long tradition in studying normative agent-based systems, focusing on various aspects that are relevant to norms (Neumann 2010a). Whereas the DINO model does by far not represent all important mechanisms, missing for example social enforcement (Andrighetto et al. 2010), we aimed at advancing the study of norms in agent-based systems on different levels. First, our representation of norms as (1) behaviour-specific and (2) ranging from approval to disapproval introduces a new level of complexity. The DINO model suggests that these aspects matter. Second, by implementing the norm internalization process in a generic decision-making context, it is applicable to a variety of decision-making models – also those not primarily focusing on norms. Prerequisite for applying the DINO internalization process is an expectancy-value model of decision-making, such as approaches based on the theory of planned behaviour which include models of voting behaviour (Kottonau & Pahl-Wostl 2004), recycling behaviour (Scalco et al. 2017), resource use (Briegel et al. 2012; Nerb et al. 1997), and innovation diffusion (Schwarz & Ernst 2009). The theory of planned behaviour offers an integrative decision framework that can easily be expanded, and micro theories applied (Jager 2000).
The DINO model is a theoretical model, developed on the grounds of theoretical and empirical research. Some model results are well relatable to existing literature. Some assumptions, such as on the adaptation speed of different norms, remain free parameters and thus to be empirically tested and validated. Presently, there is very little empirical time-series data on internalization processes, while first shining examples show that they can be assessed (Szekely et al. 2021). In any case, there is much value in a formalized, coherent, and dynamic psychological theory (Jager 2017; Neumann 2010b). Simulated theories mean they can be experimented on (Dowling 1999; Troitzsch 2017). They allow us to investigate the relations between psychological phenomena on the micro level, such as norm internalization, and social/sociological ones on the macro level (Lorenz et al. 2021). They allow for the induction of hypotheses that may then be tested empirically and – in a recursive process – for improvement of theory, simulation, and data. The DINO model presents various hypotheses that may stimulate future empirical research. For instance, it suggests that norm approval is facilitated by a cooperative setting. This could be a starting point for investigating conditions of norm internalization empirically. The model also implies that fostering norm disapproval can be a powerful means to achieve norm-consistent behaviour.
Conclusion
Norm internalization is considered a fundamental principle in human development (Vygotsky 1930) and an essential element of socialization (Hoffman 1977). A better understanding of norm internalization seems crucial (Neumann 2010b). In this work, we presented an agent architecture for studying the conditions and independent effects of norm internalization within a decision-making framework. The DINO model demonstrated several main theoretical assumptions and empirical findings. It complements existing research by providing insights into the interactions of internal and external processes that underlie conditions of cooperation and highlights the potential of social simulation to provide causal explanations for empirically observed phenomena. Furthermore, it provides useful conceptualizations for advancing normative agent-based systems and promising theoretical conclusions that may serve as hypotheses for future research.
Acknowledgements
The present work was developed within the ZumWert project. We acknowledge the funding of the University of Kassel in their profile building initiative. We thank three anonymous reviewers for their valuable and detailed comments. We also thank Georg von Wangenheim and Fabian Mankat for insightful discussions on model development.
Model Documentation
The model is implemented in NetLogo version 6.2.2. The code and ODD protocol are available at: https://www.comses.net/codebases/1bb193d4-6c9e-4f19-84d1-b95e2780e9ed/releases/1.0.0/.
Appendix
A1: Personality differences in values
First, we now briefly present relevant literature to developing the seven personality types and second introduce them. People’s goal structure, relating to their social value orientation, translates into their willingness to cooperate (Murphy et al. 2011; Murphy & Ackermann 2013, 2014). Several authors categorized people along that continuum. Frank (1988) suggested two types: cooperative individuals, who always decide to maximize joint payoff, and defecting individuals, who strive to maximize their own payoff. Fischbacher et al. (2001) added a third type: conditional cooperators (see also Fehr & Fischbacher 2002). They have been described as a melting pot for all kinds of motivations such as “’sucker aversion’, ‘conformity’ or ‘miming’ […]” (Burlando & Guala 2005 p. 36). Their motivation “depends directly on how others behave or are believed to behave” (Fischbacher & Gächter 2010 p. 542), being social descriptive norms. Being prone to social influence is connected to the personality trait openness (McCrae 2000). Openness is linked to flexibility (Deyoung et al. 2002), relating to a low importance of relying on habits.
We adopted the classification along the willingness to cooperate continuum with people at the extremes inherently favouring either cooperation or defection. Conditional cooperators in between the extremes are inherently more conflicted and sensible to their social environment. Based on the literature, we assumed that highly cooperative and highly defective individuals score lower on openness than conditional cooperators. Hence, they are less affected by situational changes, such as social influences, and rely more on habits in decision-making. To represent more complexity than the conventional three categories of cooperators, conditional cooperators, and defectors, we distinguished seven personality types along their motivations. While the number of seven is in principle arbitrary, it allowed us a more fine-grained differentiation within each category similar to a Likert scale. Moreover, each type possesses stereotypical characteristics relevant in the context of norm internalization. Of course, types do not represent the empirical reality of personalities, which one could consider as being continuous rather than categorical. However, for our purpose types were expedient, reducing complexity.
We now introduce our seven types, ordered from most to the least cooperative. Cooperator type 1 is intrinsically highly willing to cooperate and thus to sacrifice the own well-being for the group. It is strongly guided by its goals, inflexible, and barely influenced by social norms. Cooperator type 2 is similarly high motivated to contribute to the benefits of the group. However, type 2 also has some self-interest in mind and derives its motivation from its goals as well as from social norms. Empirically, cooperators represent between 1% and 18% of the population (1-4% in Noosey et al. 2020; 18% in Burlando & Guala 2005).
The three conditional cooperators (types 3 to 5) are more conflicted, having contradictory motivations. Type 3 is still highly willing to cooperate. However, it has equally strong other goals and is highly susceptible to social pressures. This makes type 3 inconsistent and malleable by its social surroundings. Type 4 is highly driven by what others do. Having a rather balanced goal structure, it is highly flexible and can jump on any bandwagon. Type 5 is worried about getting one’s share from the cake (through individualistic and competitive goals), which is why cooperation seems too risky. At the same time, it is highly susceptible to social norms. Empirically, conditional cooperators are the largest fraction, constituting between 35% and 63% of the population (35% in Burlando & Guala 2005; 63% in Kurzban & Houser 2005).
Defector type 6 is a typical individualist, considering above all its own advantage, generally paying little attention to social norms. Defector type 7 represents the exact opposite to type 1 along the willingness to cooperate continuum, which means being highly competitive. Similar to type 1, type 7 is highly motivated by its goals, and little influenced by social pressures, resulting in a highly inflexible defector. Empirically, defectors represent between 4% and 33% of the population (33% in Fischbacher et al. 2001; 4-25% in Martinsson et al. 2009).
A2: Sensitivity analysis of the \(internalization-change-rate\)
We conducted a sensitivity analysis for the adaptation parameter of personal norms, namely the \(internalization-change-rate\). We assumed that the adaptation speed of the personal norms was lower than the one of expectations. We set the \(expectation-change-rate\) to 0.2, assuming that expectations are adapted quickly within few rounds of the game, and varied the \(internalization-change-rate\) from 0.01 to 0.2. Figure 7 shows the effects on (A) cooperation, (B) behavioural changes, (C) payoff inequality, and (D & E) internalization of both personal norms. Figure 7A shows that agents’ cooperation is more similar in several group compositions for \(internalization-change-rates\) between 0.01 and 0.07. Several groups were tipped over to pure cooperation at higher change rates. Lower change rates are were associated with more behavioural changes (Figure 7B) and slightly higher payoff inequality (Figure 7C). Figures 7D and 7E show that internalization dynamics tended to be more similar with low change rates.
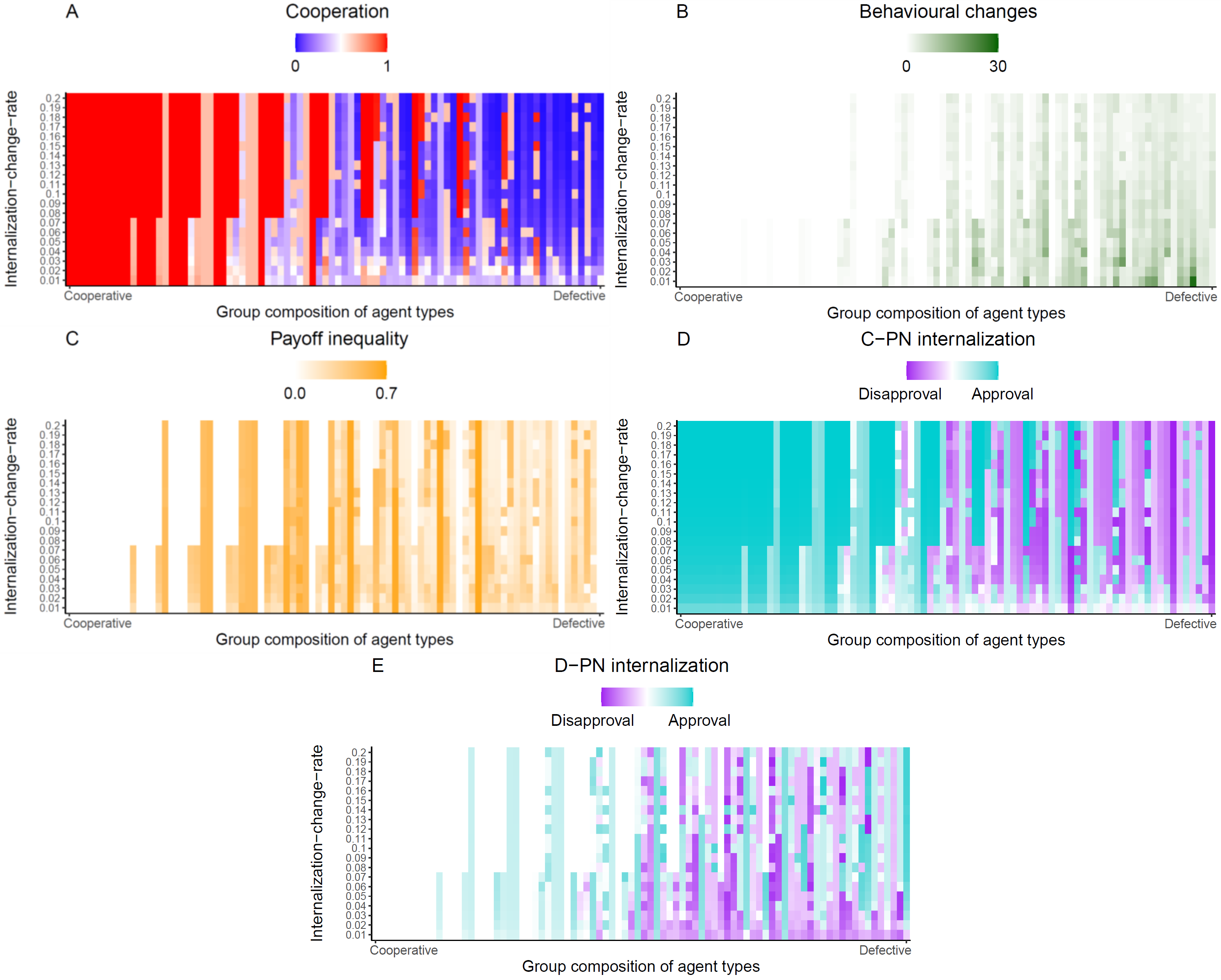
A3: Proof-of-concept simulation: What are the independent effects of norm internalization apart from other normative and non-normative behavioural influences?
Results
To examine the independent effects, we compared the model with norm internalization to the one without. As guidance for this exploratory analysis, we used the same outcome variables as in the other experiments, namely: cooperation, behavioural changes, and payoff inequality. Outcome variables were averaged across time and group compositions. Table 3 shows agents’ cooperation in the model with and without norm internalization, depending on agent type. Most agent types cooperated more in the model with norm internalization, which also caused a general increase in cooperation (see Table 4). Did norm internalization lead to norm-consistent behaviour? Whereas approval of the C-PN was strongly associated with cooperation (\(p_{pb} = 0.93\)), the D-PN was not correlated with defection (\(p_{pb} = -0.05\)), resulting from the fact that defection could result from D-PN approval as well as disapproval of both norms (see Figure 8) 2. Similarly, correlational data showed that approval of the C-PN was associated to greater differences in the two behavioural intentions favouring cooperation (\(p_{pb} = 0.81\)), whereas approval of the D-PN was close to uncorrelated with differences in intentions favouring defection (\(p_{pb} = -0.11\)).
Cooperation | |||||||
---|---|---|---|---|---|---|---|
Type 1 | Type 2 | Type 3 | Type 4 | Type 5 | Type 6 | Type 7 | |
Without norm internalization | 0.98 | 0.67 | 0.56 | 0.52 | 0.53 | 0.00 | 0.00 |
With norm internalization | 0.98 | 0.84 | 0.72 | 0.65 | 0.40 | 0.28 | 0.02 |
Without norm internalization | With norm internalization | |
---|---|---|
Cooperation | 0.51 | 0.59 |
Behavioural changes | 0.12 | 2.93 |
Round of last behavioural change | 1.20 | 54.63 |
Payoff inequality | 0.13 | 0.25 |
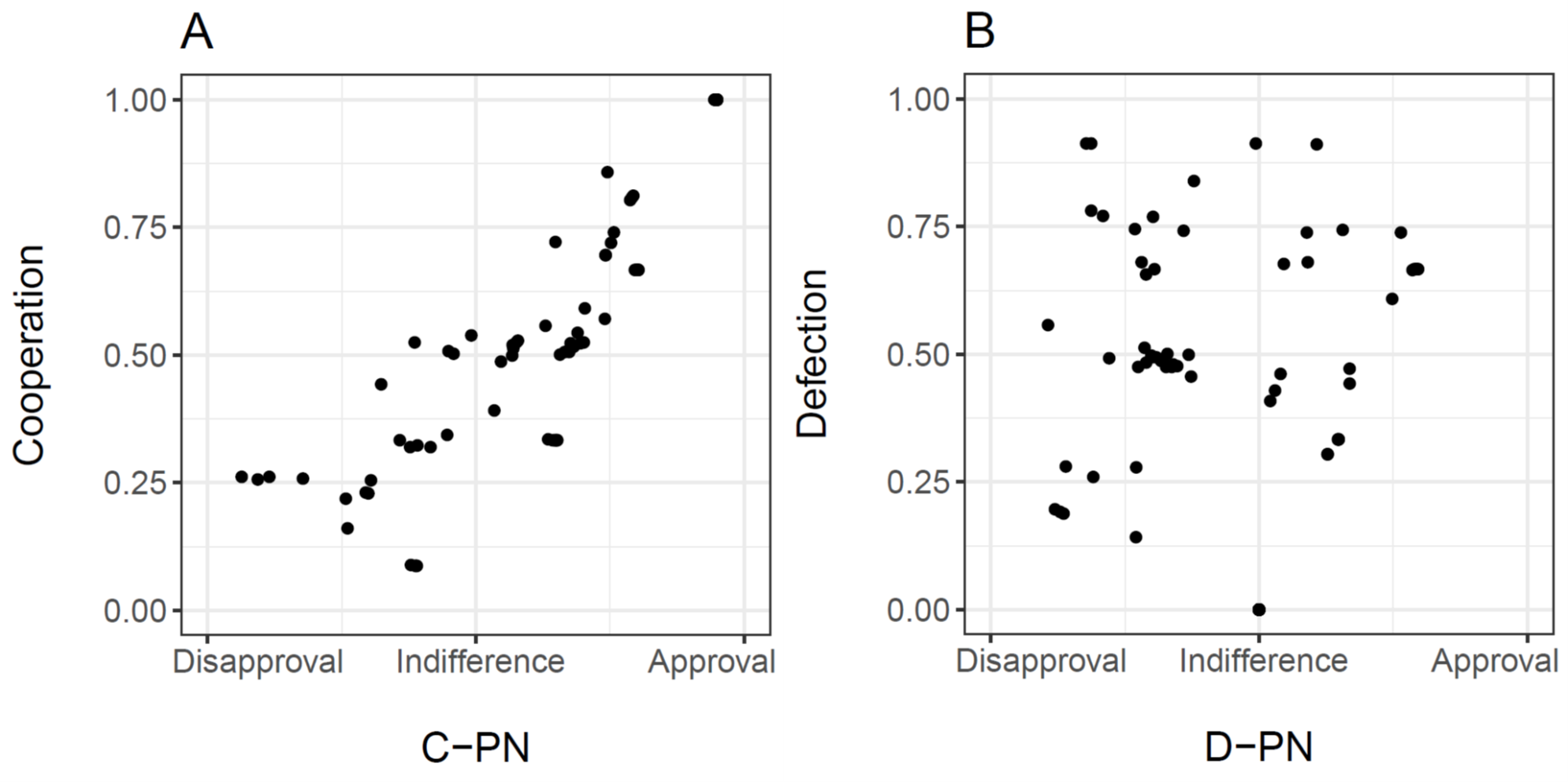
Table 4 shows that agents changed behaviour more often when they internalized norms. Moreover, in the model with norm internalization behaviour stabilized later and lock-in phenomena occurred later in the game. Without norm internalization, model runs were strongly determined by agents’ first few actions and their consequences, while norm internalization made agents’ behaviour more volatile. Norm internalization could be a longer process depending on agent type and social setting. Especially those model runs in which agents’ norm internalization was a longer process differed more strongly between the model without and with norm internalization. Norm internalization was associated with behavioural volatility. Correlations showed that particularly disapproval of a norm was associated with more behavioural changes (\(p_{pb,C-PN} = -0.61\) and \(p_{pb,D-PN} = -0.68\)) and later occurrence of lock-in phenomena (\(p_{pb,C-PN} = -0.83\) and \(p_{pb,D-PN} = -0.56\)).
Payoff inequality emerged when agents behaved differently from one another. Norm internalization changed payoff allocations between agents (see Table 4). Although norm internalization is influenced by social norms, personal norms do did not encourage behavioural conformity and hence payoff equality, but rather increased inequality. Payoff inequality was slightly associated with approval of the D-PN (\(p_{pb} = 0.25\)) and disapproval of the C-PN (\(p_{pb} = -0.37\)), whereas the latter relation is better described by an inverted U-shape (see Figure 9A).
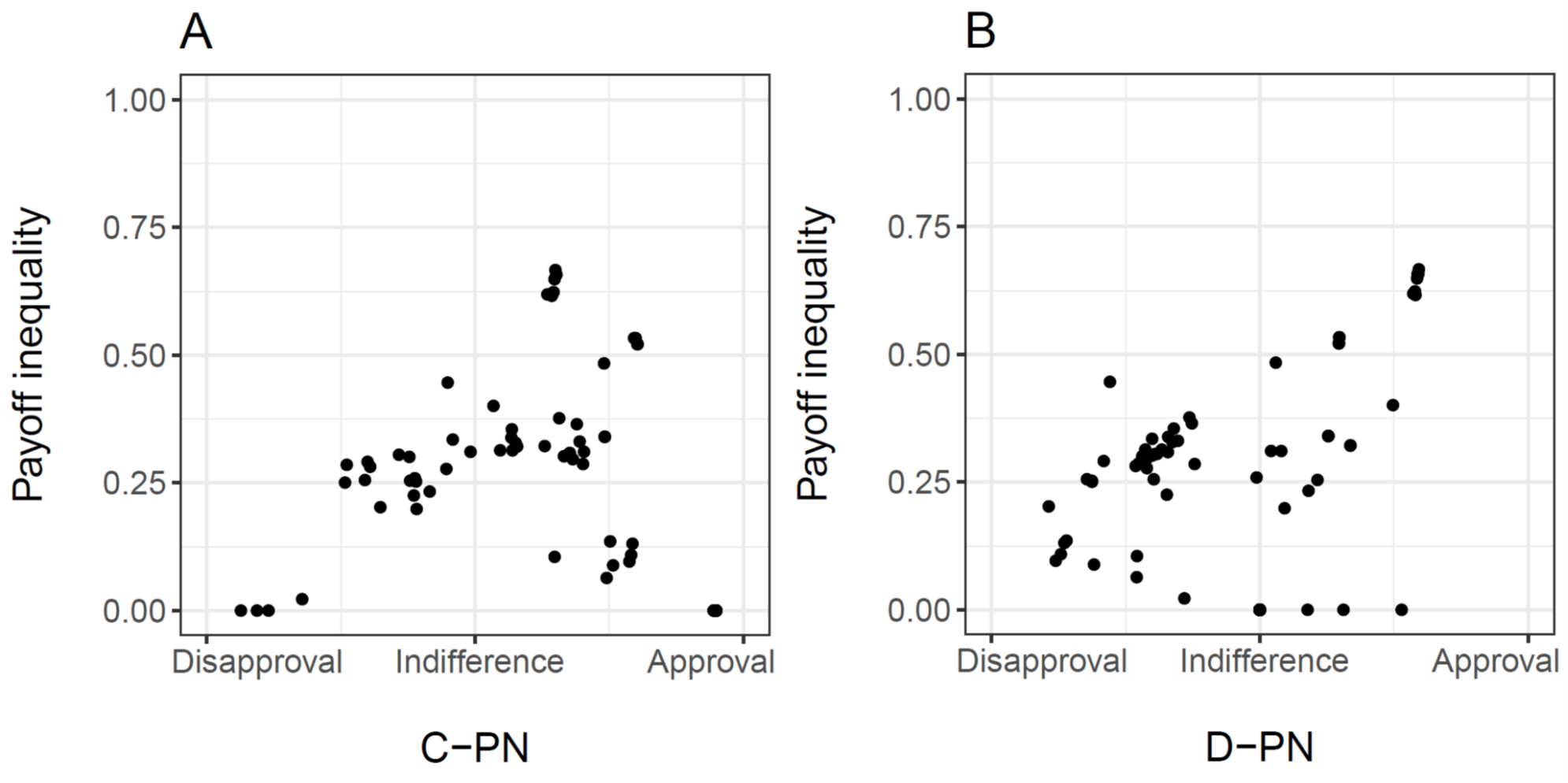
Discussion
In our model, norm internalization overall promoted the emergence of cooperation. Regarding the vast amount of literature stemming from developmental psychology, moral psychology, economics, etc., closely connecting norm internalization to morality, this result may not seem surprising (Bicchieri & Dimant 2019; Haidt 2001; Hoffman 2000; Nyborg 2018; Schwartz 1977a; Thøgersen 1999). While we drew on these approaches to formulate assumptions on internalization mechanisms, our conceptualization differs significantly. We did not employ an ethical conceptualization in the sense of linking personal norms to “moral” or “prosocial” behaviour, but rather described the mechanisms for making a judgment on appropriateness or inappropriateness. Interestingly, the model showed that our conceptualization of norm internalization as a slow adaptation process, storing and abstracting part of the situational learning, promotes cooperation – a result that strongly relates to the above-mentioned literature.
Norm internalization increased the intention to show a behaviour with agents still being able to behave against their internalized norms. This result is highly consistent with empirical research (e.g., Bamberg & Schmidt 2003) and psychological theories (e.g., Schwartz 1977a; Bandura 2001). The DINO model represents, to our knowledge, a first approach towards understanding these empirical norm-behaviour relations. It sheds not only light onto the question when norm internalization does lead to norm-consistent behaviour but also when it does not.
Norm internalization increased behavioural volatility and later occurrence of lock-in phenomena. Especially the ability to disapprove of a norm displayed agents’ multi-dimensional motivational structure, leading to counterintuitive effects of a conditional cooperator (type 5) defecting more and a defector (type 6) cooperating more. In the model, personal norms influence the importance of motivational factors, making individual preferences malleable by the situation and hence agents more adaptive. That way, agents are (slowly) transformed by the situation, making the norm internalization a transformative process of the individual as has been theoretically assumed (Piaget 1970; Vygotsky 1930). Similarly, Gintis (2004) argued that “internalization of norms is thus adaptive because it facilitates the transformation of drives, needs, desires and pleasures” and “altering agents’ goals” (p. 62).
Whereas introducing norm internalization increased cooperation, it decreased payoff equality. The rise in cooperation tended to be driven by single agents approving of the cooperation norm, making agents more persistent cooperators. As a result, they cooperated more even in groups with defecting others. This led conditional cooperators and defectors in mixed groups to approve of the defection norm more easily and defect increasingly. Hence, introducing norm internalization had polarizing effects on motivation as well as payoff distributions. Social norms may to have different, sometimes contradictory macro level social effects, such as reducing social friction and improving coordination (Sen & Airiau 2007; Shoham & Tennenholtz 1992), maintaining or undermining social cohesion (Taylor & Davis 2018), and solving or enforcing social inequality (Conte & Castelfranchi 1995; Saam & Harrer 1999; Ullmann-Margalit 1977). Regarding norm internalization, there is generally little knowledge about its macro level effects. Lorenz et al. (2021) have shown that motivated cognition causes societal attitude polarization, which relates to the DINO norm internalization process, building on motivated reasoning literature (Festinger 1957; Kunda 1990; Rozin 1999). It seems plausible to assume that in social groups with multiple norms, internalization of different norms may lead to increased inequality.
Notes
As the two social descriptive norm expectations are assumed negatively dependent in the present decision scenario, we refrained from representing them as ranging from appropriateness to inappropriateness for simplicity and consistency with other expectations. ↩︎
Based on the nature of the data, we calculated robust correlation coefficients, i.e., the percentage bend correlation \(p_{pb}\), since in standard correlation measures such as Pearson’s correlation outliers and normality violations have strong impacts ↩︎
References
AJZEN, I. (1991). The theory of planned behavior. Organizational Behavior and Human Decision Processes, 50, 179–211.
ANDRIGHETTO, G., Campenni, M., Cecconi, F., & Conte, R. (2010). The complex loop of norm emergence: A simulation model. In K. Takadama, C. Cioffi-Revilla, & G. Deffuant (Eds.), Simulating Interacting Agents and Social Phenomena (pp. 19–35). Springer.
ANDRIGHETTO, G., Villatoro, D., & Conte, R. (2010). Norm internalization in artificial societies. AI Communications, 23(4), 325–339.
ATKINSON, J. W. (1957). Motivational determinants of risk-taking behavior. Psychological Review, 64, 359–372.
AXELROD, R. (1984). The Evolution Of Cooperation. New York, NY: Basic Books.
AXELROD, R. (1986). An evolutionary approach to norms. American Political Science Review, 80(4), 1095–1111.
BAMBERG, S., Hunecke, M., & Blöbaum, A. (2007). Social context, personal norms and the use of public transportation: Two field studies. Journal of Environmental Psychology, 27(3), 190–203.
BAMBERG, S., & Schmidt, P. (2003). Incentives, morality, or habit? Predicting students’ car use for university routes with the models of Ajzen, Schwartz, and Triandis. Environment and Behavior, 35(2), 264–285.
BANDURA, A. (1971). Social Learning Theory. New York, NY: General Learning Press.
BANDURA, A. (1999). Social cognitive theory: An agentic perspective. Asian Journal of Social Psychology, 2(1), 21–41.
BANDURA, A. (2001). Social cognitive theory: An agentic perspective. Annual Review of Psychology, 52(1), 1–26.
BATZKE, M. C. L., & Ernst, A. (2022). Explaining and resolving norm-behavior inconsistencies – A theoretical agent-based model. In M. Czupryna & B. Kamiński (Eds.), Advances in Social Simulation (pp. 41–52). Berlin Heidelberg: Springer.
BEM, D. J. (1967). Self-perception: An alternative interpretation of cognitive dissonance phenomena. Psychological Review, 74(3), 183.
BEM, D. J. (1972). Self-perception theory. In L. Berkowitz (Ed.), Advances in Experimental Social Psychology (Vol. 6, pp. 1–62). Cambridge, MA: Academic Press.
BENDOR, J., & Swistak, P. (2001). The evolution of norms. American Journal of Sociology, 106(6), 1493–1545.
BICCHIERI, C. (2006). The Grammar Of Society: The Nature and Dynamics of Social Norms. Cambridge: Cambridge University Press.
BICCHIERI, C., & Dimant, E. (2019). Nudging with care: The risks and benefits of social information. Public Choice, 191, 1–22.
BRANDON, G., & Lewis, A. (1999). Reducing household energy consumption: A qualitative and quantitative field study. Journal of Environmental Psychology, 19, 75–85.
BRIEGEL, R., Ernst, A., Holzhauer, S., Klemm, D., Krebs, F., & Martínez Piñánez, A. (2012). Social-ecological modelling with LARA: A psychologically well-founded lightweight agent architecture. International Congress on Environmental Modelling and Software. Managing Resources of a Limited Planet. Sixth Biennial Meeting, Leipzig, Germany. Available at: http://www.iemss.org/society/index.php/iemss-2012-proceedings
BROERSEN, J., Dastani, M., Hulstijn, J., Huang, Z., & Torre, L. (2001). The BOID architecture: Conflicts between beliefs, obligations, intentions and desires. Agents ’01: Proceedings of the fifth international conference on Autonomous agents. Association for Computing Machinery
BURLANDO, R. M., & Guala, F. (2005). Heterogeneous agents in public goods experiments. Experimental Economics, 8(1), 35–54.
CAMERER, C. F. (2003). Behavioral Game Theory. New York, NY: Russell Sage.
CASTELFRANCHI, C., Dignum, F., Jonker, C. M., & Treur, J. (2000). Deliberative normative agents: Principles and architecture. In N. R. Jennings & Y. Lesperance (Eds.), Intelligent Agents VI. Agent Theories, Architectures, and Languages (Vol. 1757, pp. 364–378). Berlin Heidelberg: Springer.
CIALDINI, R. B., Reno, R. R., & Kallgren, C. A. (1990). A focus theory of normative conduct: Recycling the concept of norms to reduce littering in public places. Journal of Personality and Social Psychology, 58(6), 1015–1026.
CONNER, M., & Armitage, C. J. (1998). Extending the theory of planned behavior: A review and avenues for further research. Journal of Applied Social Psychology, 28(15), 1429–1464.
CONTE, R., Andrighetto, G., & Campennì, M. (2010). Internalizing norms: A cognitive model of (social) norms’ internalization. International Journal of Agent Technologies and Systems, 2(1), 63–73.
CONTE, R., & Castelfranchi, C. (1995). Understanding the functions of norms in social groups through simulation. In N. Gilbert & R. Conte (Eds.), Artificial Societies: The Computer Simulation of Social Life (pp. 213–226). London: Routledge.
COSTA, P. T., & McCrae, R. R. (1986). Personality stability and its implications for clinical psychology. Clinical Psychology Review, 6(5), 407–423.
DANNENBERG, A., Gutsche, G., Batzke, M., Christens, S., Engler, D., Mankat, F., Möller, S., Weingärtner, E., Ernst, A., Lumkowsky, M., Wangenheim, G., Hornung, G., & Ziegler, A. (2023). The effects of norms on environmental behavior. Review of Environmental Economics and Policy, in press.
DAWES, R. M. (1980). Social dilemmas. Annual Review of Psychology, 31, 169–193.
DECI, E. L., & Ryan, R. M. (1985). The general causality orientations scale: Self-determination in personality. Journal of Research in Personality, 19(2), 109–134.
DECI, E. L., & Ryan, R. M. (2000). The “what” and “why” of goal pursuits: Human needs and the self-determination of behavior. Psychological Inquiry, 11(4), 227–268.
DE Oliveira, A. C. M., Croson, R. T. A., & Eckel, C. (2015). One bad apple? Heterogeneity and information in public good provision. Experimental Economics, 18(1), 116–135.
DEUTSCH, M. (1958). Trust and suspicion. Journal of Conflict Resolution, 2(3), 265–279.
DEYOUNG, C. G., Peterson, J. B., & Higgins, D. M. (2002). Higher-order factors of the Big Five predict conformity: Are there neuroses of health? Personality and Individual Differences, 33(4), 533–552.
DOWLING, D. (1999). Experimenting on theories. Science in Context, 12(2), 261–273.
DURKHEIM, E. (1893). Über soziale Arbeitsteilung. Studie über die Organisation höherer Gesellschaften [The Division of Labour in Society]. Frankfurt am Main: Suhrkamp.
EPSTEIN, J. (2006). Generative Social Science: Studies in Agent-Based Computational Modeling. Princeton: Princeton University Press.
ERNST, A. (2003). Agentenbasierte Modellierung des Handelns in Gemeingutdilemmata. Jahrbuch Ökologische Ökonomik, 3, 139–170.
ERNST, A. (2010). Social simulation: A method to investigate environmental change from a social science perspective. In M. Gross & H. Heinrichs (Eds.), Environmental Sociology (pp. 109–122). Berlin Heidelberg: Springer.
FEHR, E., & Fischbacher, U. (2002). Why social preferences matter – The impact of non‐selfish motives on competition, cooperation and incentives. The Economic Journal, 112(478), 1–33.
FESTINGER, L. (1957). A Theory of Cognitive Dissonance. Palo Alto, CA: Stanford University Press.
FISCHBACHER, U., & Gächter, S. (2010). Social preferences, beliefs, and the dynamics of free riding in public goods experiments. American Economic Review, 100(1), 541–556.
FISCHBACHER, U., Gächter, S., & Fehr, E. (2001). Are people conditionally cooperative? Evidence from a public goods experiment. Economics Letters, 71(3), 397–404.
FISHBEIN, M., & Ajzen, I. (1975). Belief, attitude, intention, and behavior: An introduction to theory and research. Boston, MA: Addison-Wesley.
FISHBEIN, M., & Ajzen, I. (1981). Attitudes and voting behavior: An application of the theory of reasoned action. Progress in Applied Social Psychology, 1(1), 253–313.
FRANK, R. H. (1988). Passions Within Reason: The Strategic Role of the Emotions. W.W. Norton & Co.
FREUD, S. (1932). Neue Folge der Vorlesungen zur Einführung in die Psychoanalyse [New Introductory Lectures on Psychoanalysis]. Fischer.
GÄCHTER, S., & Thöni, C. (2005). Social learning and voluntary cooperation among like-minded people. Journal of the European Economic Association, 3(2–3), 303–314.
GIGERENZER, G. (2001). The adaptive toolbox. In G. Gigerenzer & R. Selten (Eds.), Bounded Rationality: The Adaptive Toolbox (pp. 37–50). Cambridge, MA: MIT Press.
GINTIS, H. (2004). The genetic side of gene-culture coevolution: Internalization of norms and prosocial emotions. Journal of Economic Behavior and Organization, 53, 57–67.
HAIDT, J. (2001). The emotional dog and its rational tail: A social intuitionist approach to moral judgment. Psychological Review, 108(4), 814.
HAMANN, K. R., Reese, G., Seewald, D., & Loeschinger, D. C. (2015). Affixing the theory of normative conduct (to your mailbox): Injunctive and descriptive norms as predictors of anti-ads sticker use. Journal of Environmental Psychology, 44, 1–9.
HARDIN, G. R. (1968). The tragedy of the commons. Science, 162(3859), 1243–1248.
HARLAND, P., Staats, H., & Wilke, H. A. (1999). Explaining proenvironmental intention and behavior by personal norms and the theory of planned behavior. Journal of Applied Social Psychology, 29(12), 2505–2528.
HARRIS, M. A., Brett, C. E., Johnson, W., & Deary, I. J. (2016). Personality stability from age 14 to age 77 years. Psychology and Aging, 31(8), 862.
HARTIG, B., Irlenbusch, B., & Kölle, F. (2015). Conditioning on what? Heterogeneous contributions and conditional cooperation. Journal of Behavioral and Experimental Economics, 55, 48–64.
HINES, J. M., Hungerford, H. R., & Tomera, A. N. (1987). Analysis and synthesis of research on responsible environmental behavior: A meta-analysis. The Journal of Environmental Education, 18(2), 1–8.
HOFFMAN, M. L. (1977). Moral internalization: Current theory and research. In L. Berkowitz (Ed.), Advances in Experimental Social Psychology (Vol. 10, pp. 85–133). New York, NY: Academic Press.
HOFFMAN, M. L. (2000). Empathy and moral development: Implications for caring and justice. Cambridge: Cambridge University Press.
HOLLANDER, C. D., & Wu, A. S. (2011). The current state of normative agent-based systems. Journal of Artificial Societies and Social Simulation, 14(2), 6.
HORNE, C. (2003). The internal enforcement of norms. European Sociological Review, 19(4), 335–343.
JACOBSON, R. P., Mortensen, C. R., & Cialdini, R. B. (2011). Bodies obliged and unbound: Differentiated response tendencies for injunctive and descriptive social norms. Journal of Personality and Social Psychology, 100(3), 433.
JAGER, W. (2000). Modelling consumer behaviour. Available at: https://research.rug.nl/en/publications/modelling-consumer-behaviour
JAGER, W. (2017). Enhancing the realism of simulation (EROS): On implementing and developing psychological theory in social simulation. Journal of Artificial Societies and Social Simulation, 20(3), 14.
JAGER, W., & Ernst, A. (2017). Introduction of the special issue: "Social simulation in environmental psychology". Journal of Environmental Psychology, 52, 114–118.
KAHNEMAN, D. (2003). Maps of bounded rationality: Psychology for behavioral economics. American Economic Review, 93(5), 1449–1475.
KAISER, F. G., & Scheuthle, H. (2003). Two challenges to a moral extension of the theory of planned behavior: Moral norms and just world beliefs in conservationism. Personality and Individual Differences, 35(5), 1033–1048.
KANGUR, A., Jager, W., Verbrugge, R., & Bockarjova, M. (2017). An agent-based model for diffusion of electric vehicles. Journal of Environmental Psychology, 52, 166–182.
KOHLBERG, L. (1964). Development of moral character and moral ideology. Review of Research in Child Development, 1, 383–431.
KOHLBERG, L. (1984). Essays on Moral Development: The Psychology of Moral Development. Skokie, IL: Row Publishers, Inc.
KOHLBERG, L., & Hersh, R. H. (1977). Moral development: A review of the theory. Theory into Practice, 16(2), 53–59.
KOTTONAU, J., & Pahl-Wostl, C. (2004). Simulating political attitudes and voting behavior. Journal of Artificial Societies and Social Simulation, 7(4), 6.
KUNDA, Z. (1990). The case for motivated reasoning. Psychological Bulletin, 108(3), 480.
KURZBAN, R., & Houser, D. (2005). Experiments investigating cooperative types in humans: A complement to evolutionary theory and simulations. Proceedings of the National Academy of Sciences, 102(5), 1803–1807.
LINDENBERG, S., & Steg, L. (2007). Normative, gain and hedonic goal frames guiding environmental behavior. Journal of Social Issues, 63(1), 117–137.
LORENZ, J., Neumann, M., & Schröder, T. (2021). Individual attitude change and societal dynamics: Computational experiments with psychological theories. Psychological Review, 128(4), 623.
LUCAS, P., Oliveira, A., & Banuri, S. (2014). The effects of group composition and social preference heterogeneity in a public goods game: An agent-based simulation. Journal of Artificial Societies and Social Simulation, 17(3), 5.
LUCE, R. D., & Raiffa, H. (1957). Games and Decisions: Introduction and Critical Survey. Hoboken, NJ: John Wiley & Sons.
MAHMOUD, M. A., Ahmad, M. S., Mohd Yusoff, M. Z., & Mustapha, A. (2014). A review of norms and normative multiagent systems. The Scientific World Journal, 2014, 684587.
MAHMOUD, S., Griffiths, N., Keppens, J., & Luck, M. (2012). Norm emergence: Overcoming hub effects in scale free networks. Proceedings of the AAMAS 2012 workshop on coordination, organizations, institutions and norms.
MARKUS, H., & Kunda, Z. (1986). Stability and malleability of the self-concept. Journal of Personality and Social Psychology, 51, 858–866.
MARTINSSON, P., Villegas-Palacio, C., & Wollbrant, C. (2009). Conditional cooperation and social group-experimental results from Colombia. Discussion Paper Series, Environment for Development. Available at: https://www.jstor.org/stable/resrep14913.
MASLOW, A. H. (1943). A theory of human motivation. Psychological Review, 50, 370–396.
MCCRAE, R. R. (2000). Emotional Intelligence from the Perspective of the Five-Factor Model of Personality. Hoboken, NJ: John Wiley & Sons.
MESSICK, D. M., & McClintock, C. G. (1968). Motivational bases of choice in experimental games. Journal of Experimental Social Psychology, 4.
MILLER, N. E., & Dollard, J. (1941). Social Learning and Imitation. New Haven, CT: Yale University Press.
MURPHY, R. O., & Ackermann, K. A. (2013). Explaining behavior in public goods games: How preferences and beliefs affect contribution levels. Available at SSRN: https://ssrn.com/abstract=2244895.
MURPHY, R. O., & Ackermann, K. A. (2014). Social value orientation: Theoretical and measurement issues in the study of social preferences. Personality and Social Psychology Review, 18(1), 13–41.
MURPHY, R. O., Ackermann, K. A., & Handgraaf, M. (2011). Measuring social value orientation. Judgment and Decision Making, 6(8), 771–781.
NERB, J., Spada, H., & Ernst, A. M. (1997). A cognitive model of agents in a commons dilemma. Proceedings of the 19th annual conference of the Cognitive Science Society.
NEUMANN, M. (2008). Homo socionicus: A case study of simulation models of norms. Journal of Artificial Societies and Social Simulation, 11(4), 6.
NEUMANN, M. (2010a). A classification of normative architectures. In K. Takadama, C. Cioffi-Revilla, & G. Deffuant (Eds.), Simulating Interacting Agents and Social Phenomena (pp. 3–18). Berlin Heidelberg: Springer.
NEUMANN, M. (2010b). Norm internalisation in human and artificial intelligence. Journal of Artificial Societies and Social Simulation, 13(1), 12.
NOOSEY, L., Isaac, R. M., Norton, D., & Stinn, J. (2020). Cooperation, contributor types, and control questions. Journal of Behavioral and Experimental Economics, 85, 101489.
NOWAK, M. A., Sasaki, A., Taylor, C., & Fudenberg, D. (2004). Emergence of cooperation and evolutionary stability in finite populations. Nature, 428(6983), 646–650.
NYBORG, K. (2018). Social norms and the environment. Annual Review of Resource Economics, 10, 405–423.
OSTROM, E. E., Dietz, T. E., Dolšak, N. E., Stern, P. C., Stonich, S. E., & Weber, E. U. (2002). The Drama of the Commons. Washington, DC: National Academy Press.
OTTO, S., & Kaiser, F. G. (2014). Ecological behavior across the lifespan: Why environmentalism increases as people grow older. Journal of Environmental Psychology, 40, 331–338.
OUELLETTE, J. A., & Wood, W. (1998). Habit and intention in everyday life: The multiple processes by which past behavior predicts future behavior. Psychological Bulletin, 124(1), 54–74.
PARSONS, T. (1937). The Structure of Social Action. New York, NY: Free Press.
PERUGINI, M., & Bagozzi, R. P. (2001). The role of desires and anticipated emotions in goal‐directed behaviours: Broadening and deepening the theory of planned behaviour. British Journal of Social Psychology, 40(1), 79–98.
PETTY, R. E., & Cacioppo, J. T. (1986). The elaboration likelihood model of persuasion. In R. E. Petty & J. T. Cacioppo (Eds.), Communication and Persuasion (pp. 1–24). Berlin Heidelberg: Springer.
PIAGET, J. (1970). Piaget’s theory. In P. Mussen (Ed.), Carmichaels’ Manual of Child Psychology (Vol. 1, pp. 703–732). Hoboken, NJ: John Wiley & Sons.
POSTMAN, L. (1947). The history and present status of the law of effect. Psychological Bulletin, 44(6), 489–563.
PYSZCZYNSKI, T., & Greenberg, J. (1987). Toward an integration of cognitive and motivational perspectives on social inference: A biased hypothesis-testing model. Advances in Experimental Social Psychology, 20, 297–340.
RIVIS, A., & Sheeran, P. (2003). Descriptive norms as an additional predictor in the theory of planned behaviour: A meta-analysis. Current Psychology, 22(3), 218–233.
ROZIN, P. (1999). The process of moralization. Psychological Science, 10(3), 218–221.
RYAN, R. M., & Deci, E. L. (2017). Self-Determination Theory. Basic Psychological Needs in Motivation, Development, and Wellness. New York, NY: The Guilford Press.
SAAM, N. J., & Harrer, A. (1999). Simulating norms, social inequality, and functional change in artificial societies. Journal of Artificial Societies and Social Simulation, 2(1), 2.
SAVARIMUTHU, B. T. R., Cranefield, S., Purvis, M., & Purvis, M. (2007). Role model based mechanism for norm emergence in artificial agent societies. In J. S. Sichman, J. Padget, S. Ossowski, & P. Noriega (Eds.), International Workshop on Coordination, Organizations, Institutions, and Norms in Agent Systems (pp. 203–217). Berlin Heidelberg: Springer.
SCALCO, A., Ceschi, A., Shiboub, I., Sartori, R., Frayret, J. M., & Dickert, S. (2017). The implementation of the theory of planned behavior in an agent-based model for waste recycling: A review and a proposal. In A. Alonso-Betanzos, N. Sánchez-Maroño, O. Fontenla-Romero, J. G. Polhill, T. Craig, J. Bajo, & J. M. Corchado (Eds.), Agent-Based Modeling Of Sustainable Behaviors (pp. 77–97). Berlin Heidelberg: Springer.
SCHLÜTER, M., Baeza, A., Dressler, G., Frank, K., Groeneveld, J., Jager, W., Janssen, M. A., McAllister, R. R. J., Müller, B., Orach, K., Schwarz, N., & Wijermans, N. (2017). A framework for mapping and comparing behavioural theories in models of social-ecological systems. Ecological Economics, 131, 21–35.
SCHÖNBACH, P. (1990). Account Episodes: The Management or Escalation of Conflict. Cambridge: Cambridge University Press.
SCHWARTZ, S. H. (1977a). Normative influences on altruism. In L. Berkowitz (Ed.), Advances in Experimental Social Psychology (Vol. 10, pp. 221–279). Cambridge, MA: Academic Press.
SCHWARTZ, S. H. (1977b). Universals in the content and structure of values: Theory and empirical tests in 20 countries. In M. Zanna (Ed.), Advances in Experimental Social Psychology (Vol. 25, pp. 1–65). Cambridge, MA: Academic Press.
SCHWARTZ, S. H., & Fleishman, J. A. (1982). Effects of negative personal norms on helping behavior. Personality and Social Psychology Bulletin, 8(1), 81–86.
SCHWARTZ, S. H., & Howard, J. A. (1981). A normative decision-making model of altruism. In J. P. Rushton (Ed.), Altruism And Helping Behaviour: Social, Personality And Developmental Perspectives (pp. 189–211). Lawrence Erlbaum Associates Inc.
SCHWARTZ, S. H., & Howard, J. A. (1982). Helping and cooperation: A self-based motivational model. In V. J. Derlega & J. Grzelak (Eds.), Cooperation and Helping Behavior: Theories and Research (pp. 327–353). Cambridge, MA: Academic Press.
SCHWARZ, N., & Ernst, A. (2009). Agent-based modeling of the diffusion of environmental innovations – An empirical approach. Technological Forecasting and Social Change, 76(4), 497–511.
SEN, S., & Airiau, S. (2007). Emergence of norms through social learning. Proceedings of the Twentieth International Joint Conference on Artificial Intelligence
SHEERAN, P. (2002). Intention-behavior relations: A conceptual and empirical review. European Review of Social Psychology, 12(1), 1–36.
SHERIF, M., & SHERIF, C. W. (1953). Groups in Harmony and Tension. New York, NY: Harper & Brothers.
SHIN, Y. H., Im, J., Jung, S. E., & Severt, K. (2018). The theory of planned behavior and the norm activation model approach to consumer behavior regarding organic menus. International Journal of Hospitality Management, 69, 21–29.
SHOHAM, Y., & Tennenholtz, M. (1992). On the synthesis of useful social laws for artificial agent societies. Proceedings of the AAAI Conference, Stanford, CA
SHOHAM, Y., & Tennenholtz, M. (1995). On social laws for artificial agent societies: Off-line design. Artificial Intelligence, 73(1–2), 231–252.
SIMON, L., Greenberg, J., & Brehm, J. (1995). Trivialization: The forgotten mode of dissonance reduction. Journal of Personality and Social Psychology, 68(2), 247.
STEG, L., & Vlek, C. (2009). Encouraging pro-environmental behaviour: An integrative review and research agenda. Journal of Environmental Psychology, 29(3), 309–317.
SUTTON, R. S., & Barto, A. G. (2018). Reinforcement Learning: An Introduction. MIT Press.
SYNDER, M. (1984). When belief creates reality. In L. Berkowitz (Ed.), Advances in Experimental Social Psychology (Vol. 18, pp. 247–305). Cambridge, MA: Academic Press.
SZEKELY, A., Lipari, F., Antonioni, A., Paolucci, M., Sánchez, A., Tummolini, L., & Andrighetto, G. (2021). Evidence from a long-term experiment that collective risks change social norms and promote cooperation. Nature Communications, 12(1), 1–7.
TAYLOR, J., & Davis, A. (2018). Social cohesion. The International Encyclopedia of Anthropology
TERRACCIANO, A., McCrae, R. R., & Costa Jr, P. T. (2010). Intra-individual change in personality stability and age. Journal of Research in Personality, 44(1), 31–37.
TERRIER, L., & Marfaing, B. (2015). Using social norms and commitment to promote pro-environmental behavior among hotel guests. Journal of Environmental Psychology, 44, 10–15.
THØGERSEN, J. (1999). The ethical consumer: Moral norms and packaging choice. Journal of Consumer Policy, 22(4), 439–460.
THØGERSEN, J. (2003). Monetary incentives and recycling: Behavioural and psychological reactions to a performance-dependent garbage fee. Journal of Consumer Policy, 26, 197–228.
TROITZSCH, K. G. (2017). Axiomatic theory and simulation. A philosophy of science perspective on Schelling’s segregation model. Journal of Artificial Societies and Social Simulation, 20(1), 10.
TVERSKY, A., & Kahneman, D. (1992). Advances in prospect theory: Cumulative representation of uncertainty. Journal of Risk and Uncertainty, 5(4), 297–323.
ULLMANN-MARGALIT, E. (1977). The Emergence of Norms. Oxford: Clarendon Press.
VERHAGEN, H. (2001). Simulation of the learning of norms. Social Science Computer Review, 19(3), 296–306.
VILLATORO, D., Andrighetto, G., Conte, R., & Sabater-Mir, J. (2015). Self-policing through norm internalization: A cognitive solution to the tragedy of the digital commons in social networks. Journal of Artificial Societies and Social Simulation, 18(2), 2.
VOISIN, D., & Fointiat, V. (2013). Reduction in cognitive dissonance according to normative standards in the induced compliance paradigm. Social Psychology, 44(3), 191–195.
VYGOTSKY, L. (1930). The genesis of higher mental functions. He concept of activity in Soviet psychology
VYGOTSKY, L. S. (2004). Analysis of sign operations of the child. In R. W. Rieber & D. K. Robinson (Eds.), The Essential Vygotsky (pp. 557–569). Kluwer Academic/Plenum Press.
WEBB, T. L., & Sheeran, P. (2006). Does changing behavioral intentions engender behavior change? A meta-analysis of the experimental evidence. Psychological Bulletin, 132(2), 249.
WHITE, K. M., Smith, J. R., Terry, D. J., Greenslade, J. H., & McKimmie, B. M. (2009). Social influence in the theory of planned behaviour: The role of descriptive, injunctive, and in‐group norms. British Journal of Social Psychology, 48(1), 135–158.
WHITMARSH, L., & O’Neill, S. (2010). Green identity, green living? The role of pro-environmental self-identity in determining consistency across diverse pro-environmental behaviours. Journal of Environmental Psychology, 30(3), 305–314.
WILENSKY, U. (1999). NetLogo. Center for Connected Learning and Computer-Based Modeling. Northwestern University, Evanston, IL