Identifying the Impact of Artifacts-Based Exploration and Exploitation on Routines’ Formation Dynamics: An Agent-Based Model

and
aShandong Technology and Business University, China; bBournemouth University, United Kingdom
Journal of Artificial
Societies and Social Simulation 26 (3) 5
<https://www.jasss.org/26/3/5.html>
DOI: 10.18564/jasss.5092
Received: 10-Feb-2023 Accepted: 12-Apr-2023 Published: 30-Jun-2023
Abstract
Organizational routines are at the core in capturing the typical way of how organizations accomplish their tasks. This paper primarily summarizes the development of scholars’ understanding of the crucial role that artifacts and the materiality play during the course of routines. We then focus on the material artifacts-based exploration and exploitation carried out by multiple human actors, and create a link between individual situated actions at the micro-level and the collective outcome as patterned routines. This discloses the underlying logic between human actors’ exploration and exploitation of material artifacts on the one hand, and the ‘(re)framing-overflowing’ interaction loop amidst routine performances and artifacts as artifactual representations (D’Adderio, 2008; 2011) on the other. Subsequently, this study uses an agent-based approach to formalize routines formation dynamics from the ‘bottom-up’. Our simulation results highlighted the relationships between the three crucial aspects – which include the interdependences between situated-actions within and between organizational tasks, artifacts-based explorative and exploitative activities carried out by multiple human actors, and organizational structures or the power asymmetry characterizing interpersonal relationships within the routine system. The research work theoretically enriches people’s understanding of routines formation dynamics over time, and provides indications for managers in designing routine performances via the artifacts.Introduction
Organizational routines are commonly defined as ‘recognizable, repetitive pattern of interdependent actions’ carried out by multiple human actors (Feldman & Pentland 2003 p. 96), and they play a crucial role in capturing the typical way of how organizations accomplish their tasks (Becker 2008; Nelson & Winter 1982). Over the last few decades, researchers have engaged practice theory and addressed routines as effortful and emergent accomplishments (Feldman 2000; Feldman & Pentland 2003; Pentland & Rueter 1994). By highlighting the role of human actors and agency, researchers emphasized the generative nature of routines and conceived routines as practices with internal structure, processes and dynamics which contribute to both stability and change in organizations (Feldman et al. 2016; Feldman 2021; Feldman & Pentland 2003). Subsequently, routine dynamics as one branch of research on organizational routines has experienced a rapid growth and become firmly embedded in academic thinking relating to organizational learning and capabilities (Felin & Foss 2009; Parmigiani & Howard-Grenville 2011; Zollo & Winter 2002), organizational change (Nigam et al. 2016), creativity and innovation (Lin et al. 2017, 2021; Sonenshein 2016), and so forth.
Some literature concerned the importance of artifacts and the materiality – i.e., artifactual representations of routines ranging from formal rules and standard operational procedures (SOPs) to machines and technology (Pentland & Feldman 2005, 2008) – that serve as mediators in human cognition and activity, shaping the course of organizational routines in the practice. According to Pentland & Feldman (2005), Pentland & Feldman (2008) and Bapuji et al. (2012), such artifacts and the materiality differ from the specific performance and abstract patterns of routines. However, they provide certain material contexts for multiple human actors to act and interact, enacting the performance of routines repetitively. D’Adderio (2008), D’Adderio (2011) further elaborated on the intricate relationship between procedures inherent in artifacts and actual performances of routines, describing it as interactive cycles of ‘framing, overflowing, and reframing’ of knowledge inputs and actions (D’Adderio 2008 p. 769). Artifacts such as written rules, machines and technology provide guidance and control for the enactment of routines by delimiting and closing search spaces. However, they are incomplete and cannot fully determine actions (D’Adderio 2008, 2011; Reynaud 2005; Yang et al. 2020). On one hand, the material property of artifacts conveys a particular set of symbolic messages facilitate certain courses of actions and make others more difficult at the same time, for participants to accomplish their organizational tasks repetitively (Cacciatori 2012). On the other hand, those symbolic messages embodied in various artifacts need to be interpreted to affect patterned routines in practice.
D’Adderio (2008) asserted that multiple human actors can always operate discretion in interpreting the symbolic messages embodied and displayed in artifacts and ‘ultimately decide whether, how and when to abide by, work around or altogether reject them’ (p.773). In other words, human actors can either explore or exploit artifacts such as written rules, machines, and technology to pursue new knowledge on how to perform their organizational work (Pentland & Feldman 2008). Following March's (1991) exploration-exploitation framework, we view exploitation here as involving utilization, or local search to traverse for the ‘best’ solutions through the search space predetermined by human actors’ situation-specific (re)interpretation in the use and development of existing artifacts (D’Adderio 2008, 2011; Geiger & Schröder 2014), and exploration as a distant or long jump of the strategical search process to get new understandings through organizational learning. For example, human actors communicate with and imitate from partners either consciously with some strong incentives or not (Hodgson 2008; Knudsen 2008). Although exploitation is perceived to yield predictable increments in performance, exploration is often associated with uncertain results on the enactment of routines (den Hamer & Frenken 2021; Gupta et al. 2006; Lavie et al. 2011). Since March’s (1991) groundbreaking paper, there have been substantial publications on exploration-exploitation within and across organizations (Almahendra & Ambos 2015; Wilden et al. 2018). However, our understanding of the role of artifacts-based exploration and exploitation in routine dynamics is still limited.
To fill the research gap, we utilized an agent-based approach to model the intricate relationship between artifacts-based explorative and exploitative activities carried out by multiple human actors on the one hand, and the interactive process between artifacts and routine performance – i.e., the ‘framing, overflowing and reframing’ cycle – on the other. Our study is built on the concept community for a couple of reasons. Firstly, as Cohendet & Llerena (2003) noted that routines are usually shaped and determined at an intermediate level, rather than individual or organizational levels; Secondly, researchers in the routines field have highlighted the importance of the manner and quality of interactions between human actors distributed across the organization (Feldman & Rafaeli 2002; Turner & Rindova 2012). Hence, (re)focusing on the performing actors could provide a useful lens to enhance people’s understanding of routine dynamics (Danner-Schröder 2021; Sargis-Roussel et al. 2017). The third reason is that the literature on learning theory also examined how the structure of social networks among individuals can affect the organizational performance through exploration and exploitation regarding human-made artifacts (den Hamer & Frenken 2021; Lazer & Friedman 2007).
Our main objective of this study is to apply the agent-based modeling (ABM) method (Gao & Akbaritabar 2022; Gómez-cruz et al. 2017; Kahl & Meyer 2016) to formalize the internal structure and processes underpinning the formation dynamics of organizational routines. Positioning organizational routines as an effortful and emergent property (Feldman 2000; Feldman & Pentland 2003), the agent-based model focuses on the artifacts-based exploration and exploitation at the micro level and investigates the collective outcome of patterned routines from the ‘bottom-up’. Based on the simulation data, we analyze and identify the impact of such artifacts-based explorative and exploitative activities on routines’ formation dynamics. In doing so, we aim to enrich the extant literature at understanding of how social and material settings affect routines’ formation dynamics, hence theoretically contribute to organizational routines literature.
The paper is structured as follows. Section 2 describes the relevant literature and formulates a conceptual framework serving as theoretical foundations of the research work. Section 3 then shows the design of the agent-based model in detail. Thereafter, we design the simulation experiments, test and verified the simulation outputs, and present simulation results in Section 4. This is followed by discussions and conclusion parts in section 5 and 6, respectively.
Theoretical Foundations
Artifacts at the center of routines
It has been widespread consensus that routines are certain generative systems consisting of two distinct aspects (Feldman et al. 2016; Feldman & Pentland 2003). One is the ostensive, referring ‘the ideal or schematic form’ of a routine that shapes people’s perception of what the routine is’, or ‘the routine in principle’; the other one is the performative, referring ‘the specific actions taken by specific people at specific times’, or ‘the routine in practice’ (Feldman & Pentland 2003 pp. 101–102). These two aspects are recursive and mutually constitutive, implying that the ostensive not only guides performances, but also is created and recreated from them (Pentland & Feldman 2005, 2008). In any practical setting, Pentland & Feldman (2005) asserted that the two aspects may ‘be codified or prescribed, as well as enabled and constrained’, by various artifacts (P.795). Those artifacts as physical manifestations reflect and influence both the ostensive and performative aspects of routines. Pentland & Feldman (2005), Pentland & Feldman (2008) placed artifacts outside of the routine itself to illustrate the interactive influence of artifacts and routines (see in Figure 1a), stating that artifacts were ‘different from either the specific performance or the abstract patterns’ and that they ‘can be incorporated into the routine in a variety of ways’ (Pentland & Feldman 2008 p. 244).
Drawing from recent advances in routines and performative theory, D’Adderio (2008), D’Adderio (2011) proposed artifacts as mediators in human cognition and activity, which brings artifacts and materiality from the periphery to the core of routines (as shown in Figure 1b). This view considers artifacts as integral parts of, rather than resting outside the routines (D’Adderio 2011), acknowledging the mutual shaping relationship between artifacts as human made objects on the one hand, and the ostensive patterns and specific performances of routines on the other. This perspective offers a potential for researchers to capture the internal dynamics underpinning routines’ emergence and evolutionary processes.
Moreover, Pentland & Hærem (2015), Feldman et al. (2016) and Pentland et al. (2017) put situated action in the foreground and proposed an action-centric agenda for routine dynamics research, suggesting that ‘patterns of actions offer a useful addition to our repertoire as a unit of analysis’ (Pentland et al. 2017 p. 137). Although concentrating on situated action helps to better understand the internal structure and dynamics of routines, the success of routines to a large extent depends on the interpersonal interactions of human actors (Danner-Schröder 2021). The fact is that multiple actors involved in the routines are often heterogenous and distributed across the organization (Becker 2004). They act and interact with each other, and the manner and quality of such interpersonal interactions help create shared understandings which influence the individuals’ situated actions, ultimately enabling either stability and change in organizational routines (Dionysiou & Tsoukas 2013; Feldman & Rafaeli 2002; Pentland & Feldman 2005; Turner & Rindova 2012). Additionally, the material property of artifacts often creates both constraints and affordances which substantively influence how human actors perform routines (D’Adderio 2008, 2011). In this sense, Gao et al. (2018) and Gao (2019) pointed out that researchers can capture the nexus between individual situated actions and the collective outcome as patterned routines by focusing on the significant role of artifacts in the enactment of routines, as illustrated in Figure 1c. This approach provides a solution to unlock the ‘black box’ of organizational routines and reveal the micro-mechanisms underpinning routine dynamics.
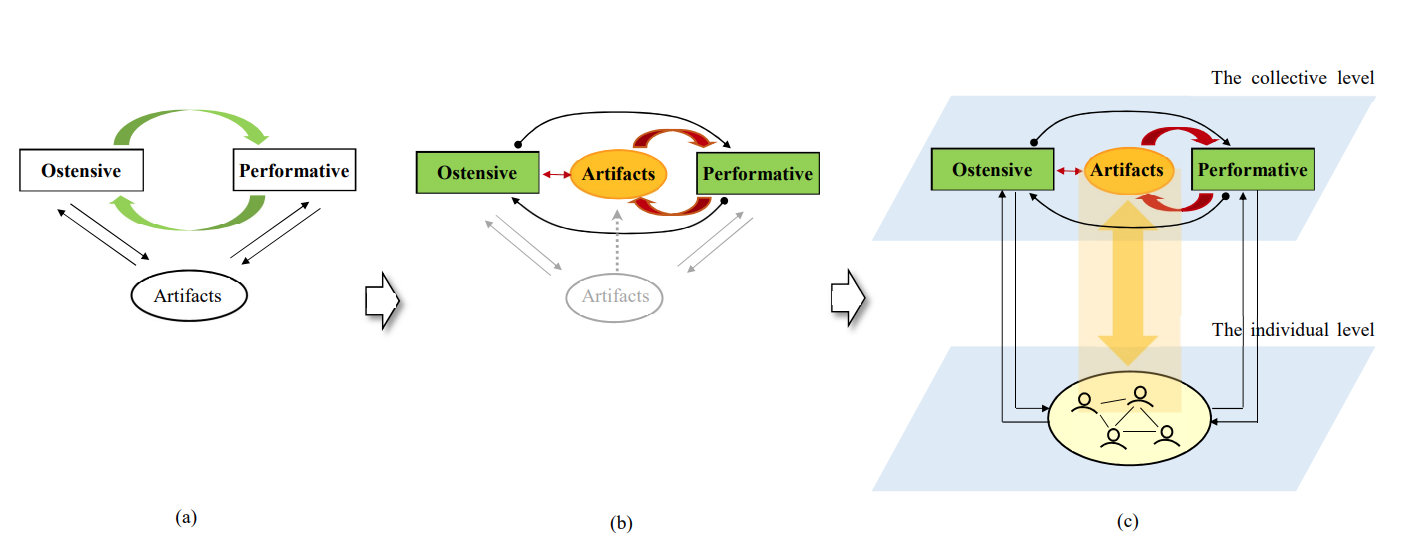
Artifacts-based exploration and exploitation in routine performances
When opening up the ‘black box’ of routines to analyze how the different aspects of routines interact and what the influence of artifacts and agencies is on routine dynamics, D’Adderio (2008), D’Adderio (2011) described the interplay between procedures inherent in artifacts and actual performances of routines as interactive cycles of (re)framing and overflowing of knowledge inputs and actions. The (re)framing action exerted by constraints from artifacts ‘delimits and closes search spaces’ of human actors (D’Adderio 2008 p. 770), providing guidance and control to set ‘the boundaries of what can be done and what cannot be done’ (D’Adderio 2011 p. 219). In this sense, the ‘(re)framing’ brings convergence between the artifacts-embedded description and actual performances of the same routine. However, these (re)framing actions are never complete and there is always room for adaptation and change, which leads to overflowing and opens up new search spaces (D’Adderio 2008). While the ‘(re)framing’ view often defines the role of artifacts as deterministic and equates the course of human practices as being reproduced automatically without adaptation or resistance, the ‘overflowing’ view by contrast highlights the ability of human actors to interpret, modify or even disregard the rules or procedures embedded in artifacts (D’Adderio 2008, 2011; Dionysiou & Tsoukas 2013).
By placing artifacts and materiality at the center, rather than periphery of routines (D’Adderio 2008, 2011), researchers can concentrate on the interaction between human and non-human actants (i.e., the multiple individual actors and artifacts, respectively) involved in the routines (Gao et al. 2018; Pentland & Feldman 2008). We then resort to human actors’ exploration and exploitation regarding certain artifacts to unearth the micro-mechanisms underpinning those translation processes from artifacts as artifactual representations to the performance of routines and vice versa, as shown in Figure 2. Artifacts often predetermine the boundaries of search spaces for human actors to enact routines (D’Adderio 2008, 2011; Geiger & Schröder 2014). This means that, when accomplishing the organizational tasks iteratively, human actors have only finite alternatives based on their situation-specific interpretation regarding existing artifacts. These human actors are bounded rational decision makers (Simon 1997) who pursue to make rational decisions to maximize their own rewards but operate under limited information and cognitive constraints. Hence, exploitation implies that human actors would utilize – e.g., by experimental refinement and reuse of (March 1991; Baum et al. 2000) – their (re)interpretation regarding artifacts. Consequently, the human actors incrementally improve their performances based on the cumulative experience and move step-by-step to the ‘best’ solution during their repetitive enactment of routines.
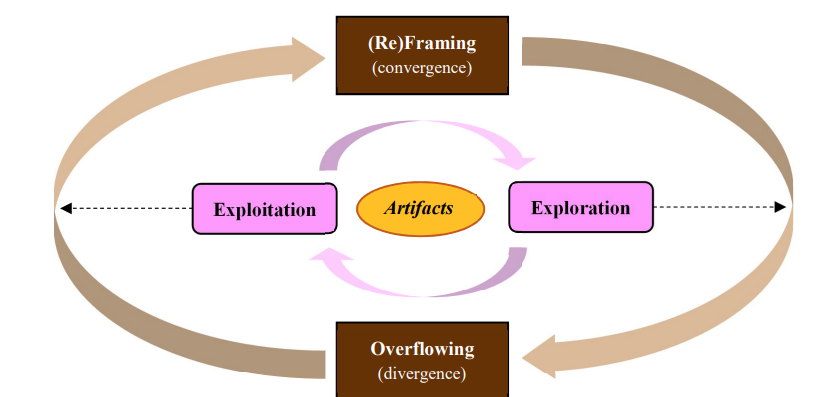
However, participants’ choices within individual search spaces are usually restricted due to their limited capacity for interpretation when using targeted artifacts and cognitive constraints imposed by the material property of artifacts themselves (Geiger & Schröder 2014; Pentland & Feldman 2008). Such exploitative activities of human actors lead to the ‘(re)framing’, or more specifically, the convergence between the artifacts-embedded description of routines, and the routines’ actual performances.
Human actors with bounded rationality intend to explore and acquire new understanding or knowledge in their situated actions (Gupta et al. 2006) through organizational learning. For example, learning could occur through communication with or imitation of partners (Hodgson 2008; Knudsen 2008). This opens individuals’ search spaces and leads to the ‘overflowing’ – that is, the divergence between artifacts as artifactual representations and the actual performances of routines. This creates adaption and change during the course of routines (D’Adderio 2008; Howard-Grenville 2005), ultimately, varying organizational performance in manners (Stadler et al. 2014).
Design of the Model
The task environment
In our setting, the organizational activity is performed simultaneously by \(Q\) agents (i.e., human actors) within a community. Each agent \(q\) (\(1 \leq q \leq Q\)) faces an organizational task that can be expressed as the vector of \(N\) component actions \(action_{q} = (a_{q,1}, \dots, a_{q,n}, \dots, a_{q,N})\). The total tasks of the community can be depicted as that:
\[ TASK_{total} = \{a_{1,1}, \dots, a_{1,n}, \dots, a_{1,N}; \dots; a_{q,1}, \dots, a_{q,n}, \dots, a_{q,N}; \dots; a_{Q,1}, \dots, a_{Q,n}, \dots, a_{Q,N}\}\] | \[(1)\] |
Here, the variable \(a_{q,n}\) represents the nth component action carried out by agent \(q\) (\(1 \leq q \leq Q\); \(1 \leq n \leq N\)). For each of those component actions, there are two discrete states being noted as 0 or 1 representing different choices undertaken by the agent \(q\) to cope with the sub-tasks it faces. All the \(Q \times N\) component actions intertwine, and they together make up a collection – or in other words, a patterned sequence – of actions that we call an actual performance of the routine (Feldman & Pentland 2003; Knudsen 2008).
We utilize the NKCS model (Kauffman & Johnsen 1991; Levitan et al. 2002) to formalize the interdependencies between individual actions as that: for each agent \(q\), the performance contribution of action \(a_{q,n}\) (\(1 \leq q \leq Q\); \(1 \leq n \leq N\)) not only depend on this action’s own status, but also is affected by (1) \(K\) other actions of the agent \(q\) itself, and (2) \(C\) actions assigned to \(S\) other agents, as illustrated in Figure 3.
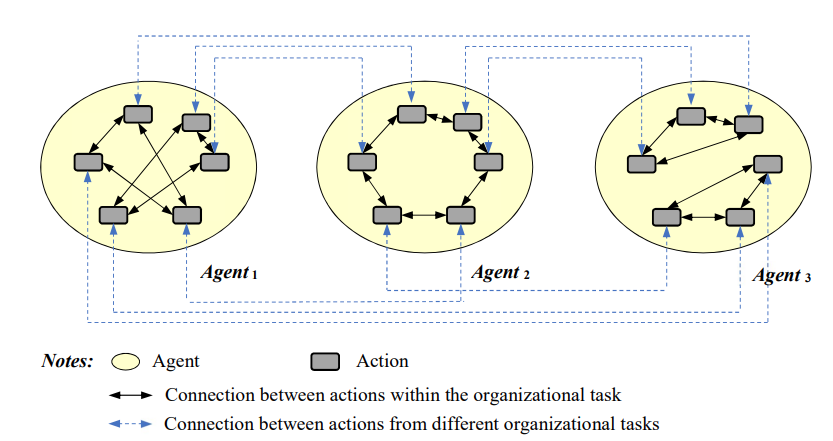
Therefore, for the action list \(action_{q} = (a_{q,1}, \dots, a_{q,n}, \dots, a_{q,N})\) of agent \(q\) (\(1 \leq q \leq Q\)), the performance contribution of each action \(a_{q,n}\) can be defined as that,
\[ f(a_{q,n}) = f(a_{q,n}; a_{q,1}, \dots, a_{q,k}, \dots, a_{q,K}; a_{1,1}, \dots, a_{1,C}; \dots; a_{s,1}, \dots, a_{s,C}; \dots; a_{S,1}, \dots, a_{S,C})\] | \[(2)\] |
Here the variables \(a_{q,1}, \dots, a_{q,k}, \dots, a_{q,K}\) (where \(k \neq n\)) represent the \(K\) other actions carried out by the agent \(q\); and \(a_{1,1}, \dots, a_{1,C}; \dots; a_{s,1}, \dots, a_{s,C}; \dots; \dots, a_{S,1}, \dots, a_{S,C}\) (where \(s \neq q\)) represent the \(C \times S\) actions of the other \(S\) agents. We generate the performance contribution values of those component actions from a uniformly distributed function \(f(a_{q,n}) \sim U[0, 1]\) by following Kauffman (1993)’s (1993) theory. All those uniform random numbers constitute a \(N \times 2^{1 + K+ CS}\) matrix as the entire landscape of the agent \(q\)’s task environment.
However, all agents within a unit (e.g., a community, group, team, etc.) are usually organized with common goals. Hence, they are more or less similar to each other (Civettini 2007), and they operate in a very similar environment. To model the similarity of agents and the closeness between task environments of those similar agents within the community, in this paper, we use the algorithm of \(\rho MNK\)-landscapes (Verel et al. 2013) to generate \(Q\) landscape matrixes – where each landscape matrix for one agent within the community – with pairwise correlations are given as:
\[ \rho = corr(f(a_{q,n}), f(a_{q^{'}, n})) \; (1 \leq q, q^{'} \leq Q \;and \; q \neq q^{'}; 1 \leq n \leq N)\] | \[(3)\] |
Next, at any time period \(t\), we define the performance of agent \(q\) (\(1 \leq q \leq Q\)) equals to the average value of performance contributions of all the individual actions assigned to it.
\[ h_{q}^{(t)} = \frac{1}{N}\sum_{n = 1}^{N}f(a_{q,n}^{(t)})\] | \[(4)\] |
Whilst the community’s overall performance is a mean of all the agents’ performances,
\[ H^{(t)} = \frac{1}{Q}\sum_{q = 1}^{Q}h_{q}^{(t)} = \frac{1}{QN}\sum_{q = 1}^{Q}\sum_{n = 1}^{N}f(a_{q,n}^{(t)})\] | \[(5)\] |
It is obvious that the higher the \(H^{(t)}\) value is, the better of the community’s performance, meaning the more adaptiveness of the community to the living environment it would be.
Agents and activities
This study treats all agents in the community as interdependent and autonomous. They aim to achieve both individual and common shared goals by iteratively accomplishing given organizational tasks. At each simulation tick t, the agent \(q\) (\(1 \leq q \leq Q\)) with bounded rationality carries out a list of actions \(action_{q}^{(t)} = (a_{q,1}^{(t)}, \dots, a_{q,n}^{(t)}, \dots, a_{q,N}^{(t)})\), and pursues to maximize its own rewards – i.e., the performance values \(h_{q}^{(t)}\) as defined in Equation 4 – by successively using the exploration and exploitation strategies as presented in the flowchart in Figure 4. Meanwhile, we illustrate the mechanisms underpinning the agents’ exploration and exploitation regarding artifacts as shown in Figure 5. Every agent can undertake individual actions in different ways which are constrained and afforded by existing artifacts and can be denoted as 0 or 1 in our model. Weichbrodt & Grote (2010) and Gao et al. (2018) highlighted the considerable variation in the degree to which artifacts as artifactual representations prescribe and influence individual action. They distinguished those formal rules and procedures as cognitive representations of artifacts and the materiality into two types. The first one is process-rules or knowledge of standards and regulation that dictate ‘the exact process of how to carry out a task from beginning to end’ (Weichbrodt & Grote 2010 p. 23), which facilitate participants’ exploitation activities. In contrast, the second type refers to goal-rules or knowledge of innovation and change, which function as boundaries shaping individual actions and giving human actors freedom to pursue variation and change, thus promoting participants’ exploration and creativity.
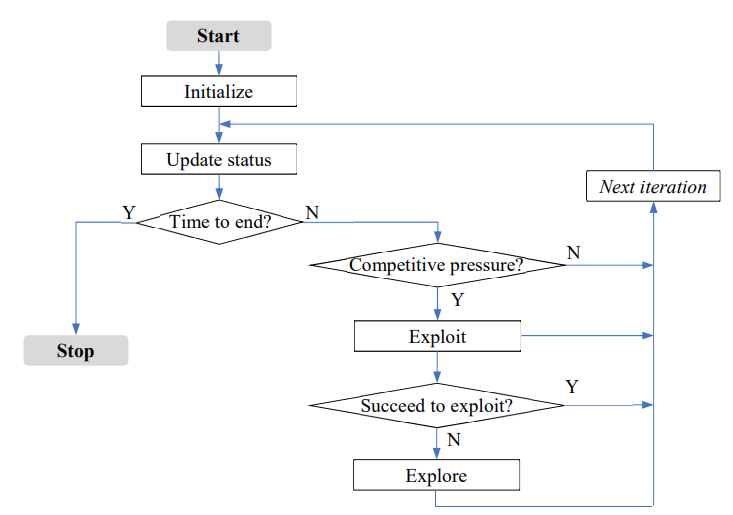
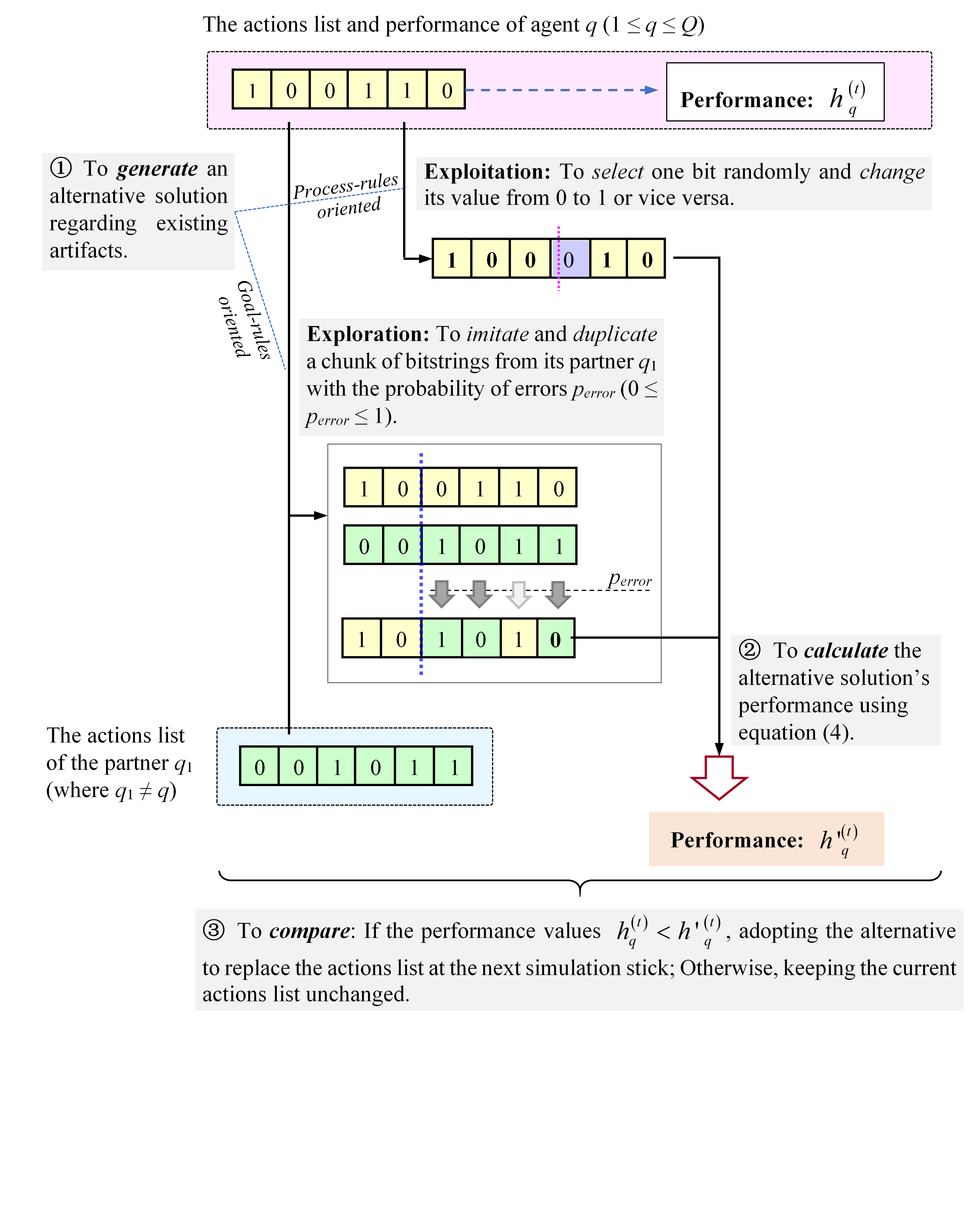
When the agent faces competitive pressure (e.g., having the smallest performance value among its neighbors), it tends to achieve exploitation based on its understanding and interpretation of existing artifacts. Exploitation refers to agents’ utilization of process-rules within artifacts and their locally search for incremental improvements towards the optimal solution step-by-step, despite being limited by their myopia. For example, an agent randomly selects only one bitstring of its actions list at a time, and vary the bitstring’s value from 0 to 1 or vice versa. If the agent finds the alternative solution outperforms its current state (i.e., with a better performance value \(h^{(t)}\)), it then adopts that solution at the next simulation tick.
When the exploitation fails, the agents explore goal-rules of artifacts through a distant or long jump facilitated by organizational learning. This involves communicating with and imitating from partners either consciously with some strong incentives or not (Hodgson 2008; Knudsen 2008). In this paper, we consider the impact of power asymmetry among interaction partners on routine dynamics (Danner-Schröder 2021), and formalize interpersonal interrelationships within the community in accordance with different types of network structures. We primarily use random network as the benchmark and consider two different networks including the Star-shape and Fully-connected network models, representing vertical (supervisor-subordinate) and horizontal (coworker) organizational structures, respectively (see in Figure 6). These two types of organizational structures correspond with the two ends of the spectrum of formal power distribution from high to low levels of power asymmetry.
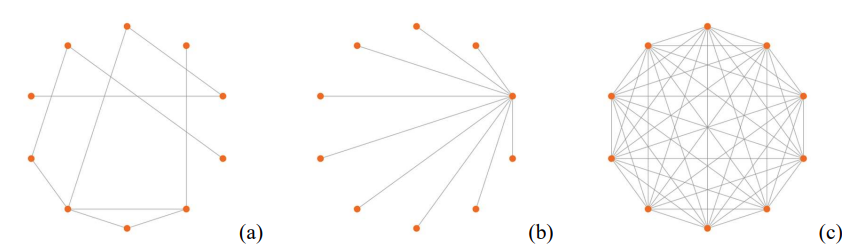
However, it worth noting that copying exactly from partners is often not an ideal approach due to the dissimilarity of the local living environment between agents (Winter & Szulanski 2001). In this case, we design the strategy of exploration inspired by the crossover in genetic algorithms (GA) (Lazer & Friedman 2007; Mitchell 1996) wherein an agent takes a part of its current solution (e.g., a subset of its own actions list) and mixes it with another chunk of bitstrings from one of its partners to generate an alternative solution. However, for duplication of each bitstring, there might be errors (Csaszar & Siggelkow 2010) resulting failures of copying the exact action, and the bitstring keeps unchanged. Let the probability of errors in duplicating each action as \(p_{error}\) (\(0 \leq p_{error} \leq 1\)), if there are \(d_{a}\) (\(1 \leq d_{a} < N\)) different bitstring values from chunks between the replicator and replicatee agents, then we have the possibility of copying exactly as that \(p_{ce} = (1 - p_{error})^{da}\), where \(0 \leq p_{ce} \leq 1\). The agent then compares the alternative solution’s performance with that of its current status, subsequently deciding whether to adopt it at the next simulation tick or not. It has been proven that such a mixing strategy could greatly improve the search process and hence providing ‘a key way of finding short cuts in the problem space’ (Lazer & Friedman 2007 p. 676).
Simulation Experiments, Test, Verification, and Results
Simulation experiments
We construct our model using NetLogo, an agent-based programmable modeling environment developed by Uri Wilensky from Northwestern University, US (The codes are available at CoMSES Net Archive: https://www.comses.net/codebase-release/389e39dd-dff6-460b-93b9-954bd1d7d06e/). Some software engineering methods such as structured code walkthroughs and unit testing (North and Macal, 2007) are employed to ensure that the simulation model is comprehensively verified.
This study is aimed to investigate the role that human actors’ explorative and exploitative activities regarding artifacts would play during the enactment of organizational routines. Additionally, we considered the influence of interpersonal interactions and power asymmetry characterized by different network structures as shown in Figure 6, and the component complexity and near-decomposability of organizational tasks, which are represented by the \(N\) and \(K\), \(C\), \(S\) values as aforementioned.
We primarily used the random network as represented in Figure 6a as the benchmark with default input settings listed in Table 1. Our focus is on the community of agents living in a stable environment, where we assume that the task environments all agents face are relatively similarity with pairwise correlations as a constant \(\rho = 0.90\), and that there are no errors when agents communicate and imitate from partners (i.e., \(p_{error} = 0.00\)). With this simple scenario as testbed, we vary both parametric (e.g., \(Q = 5\), \(10\), \(15\), \(20\); \(N=4\), \(6\), \(8\), \(10\), \(12\)) and non-parametric inputs (e.g., \(isExploit/isExplore = FALSE\), \(TRUE\)) in the following subsection. We conducted a series of sensitivity analysis (Borgonovo et al. 2022) and additional ‘trial-and-error’ experiments to not only explore the parameter space, but also test the robustness and verifying the simulation model across different scales.
Input parameter | Default value(s) | Description |
---|---|---|
\(Q\) | 5, 10, 15, 20 | Number of agents involved in the community, each with one task to cope with iteratively. |
\(N\) | 4, 6, 8, 10, 12 | Number of component actions required to accomplish an organizational task. |
\(K\) | 0, 1, 2, 3 | Internal coupling degree of the organizational task. |
\([C, S]\) | [1], [0, 1] | External coupling degree between organizational tasks. |
\(\rho\) | 0.90 | Similarity of the task environments between agents. |
\([isExplore, isExploit]\) | [FALSE, TRUE], [FALSE, TRUE] | Boolean variables that determine whether exploration and exploitation activities occur (\(TRUE\)) or not (\(FALSE\)), indicating either goal-rules or process-rules, and even the both regarding artifacts and the materiality are considered in the simulation model. |
\(p_{error}\) | 0.00, 0.01 | Probability of errors occurring in imitation between agents. |
\(net\) | [‘Random’, ‘Star-shape’, ‘Fully-connected’] | Network structure that represents the interpersonal interrelationship of agents within the community. |
To investigate the influence of interpersonal interconnection and power asymmetry between agents on the enactment of routines, we expand the benchmark scenario to compare the simulation outcomes between Star-shape and Fully-connected network structures, which we refer to as Scenario I. Furthermore, we introduced in the probability of errors occurring in agents’ imitation activities (i.e., \(p_{error} = 0.01\)) to evaluate the effects of minor disturbances of explorative activities on routines performance while keeping the other input settings the same as those in Scenario I. This new scenario is called Scenario II. However, the environment in which the community of agents live remains unchanged across the simulation progress.
Our model utilizes two aggregate measures to evaluate the simulation outputs. The first measure, referred to as the community’s performance (i.e., the parameter value \(H^{(t)}\) as defined in Equation 5). This measure serves as a critical indicator to evaluate the overall outcome of action sequences that carried out by the community of agents iteratively.
The second measure, known as \(l_{s}\), measures the synchrony level, which refers to the similarity of action sequences between different agents. Specifically, for any two agents \(q\) and \(q^{'}\) \((1 \leq q,\, q^{'} \leq Q;\, \text{and} \, q \neq q^{'})\), we define the pairwise cosine similarity of their actions sequences as:
\[ l_{s q, q^{'}}^{(t)} = \frac{a_{q,n} a_{q^{'}, n}}{\|a_{q,n}\| \times \|a_{q^{'},n}\|} = \frac{\sum_{n = 1}^{N}(a_{q,n}^{(t)} \cdot a_{q^{'}, n}^{(t)})}{\sqrt{\sum_{n = 1}^{N}(a_{q,n}^{(t)})^{2} \cdot \sum_{n = 1}^{N}(a_{q^{'}, n}^{(t)})^{2}}}\] | \[(6)\] |
We then define the output \(L_{s}\) as the average of all the pairwise similarity values:
\[ L_{s}^{(t)} = \frac{2}{Q(Q-1)}\sum_{q = 1}^{Q - 1}\sum_{q^{'} = q + 1}^{Q}(l_{s q, q^{'}}^{(t)})\] | \[(7)\] |
We have that \(0 \leq L_{s} \leq 1\). The bigger the value of the variable \(L_{s}\) is, the more synchronous of the action sequences among agents would be, which to some extent implies a much higher routinization level of collective behaviors across the community.
Test and verification of the simulation outputs
In this section, we used the benchmark scenario and perform sensitivity analysis (SA) of both parametric and non-parametric inputs of the model to test the robustness and verify the simulation outputs (Borgonovo et al. 2022). Specifically, we examined explorative and exploitative activities of the agents simultaneously (i.e., \(isExplore = isExploit = TRUE\)), and vary \(Q\), \(N\), \(K\) and \(S\) values to identify their impacts on the model’s outputs.
To address stochasticity inherent in the simulation model and prevent under-/over- powering our simulation results (Secchi & Seri 2017), we set the significance level \(alpha = 0.01\) and the power \(1 - \beta = 0.90\) based on a pilot study and previous testing experiments. We carry out the power analysis using the ANOVA test technique provided by the pwr package of R (see detailed information at https://cran.r-project.org/web/packages/pwr/index.html). For the benchmark scenario, we followed a full factorial design and calculated the ideal number of simulation runs required for it, \(numSimRuns_{ideal} = 47.99\). By following the SESOI approach given by Secchi & Seri (2017), we set the actual number of simulation runs for each parameter configuration \(numSimRuns_{actual} = 50\), resulting in an estimated expected power (EEP) of 0.92. Moreover, we run each simulation 6000 rounds, which is sufficient for results to converge into a stable status – i.e., if such a stable status actually exists.
Input-output relations of the simulation model under the benchmark scenario are shown in Figure 7 and Figure 8, which indicate that the change of \(Q\) and \(N\) values only affects the range of variation but not the median values of the distribution of output series including the synchrony of the action sequences among agents \(L_{s}\) and the community’s performance \(H\) (see in Figure 7, Figure 8a and b). However, there is a negative relationship between \(Q\) values and the simulation output \(L_{s}\) (see in Figure 8a). The community size \(Q\) and the component complexity of organizational tasks \(N\) determine the scale of our simulation model. With the increase of \(Q\) and \(N\) values, it would lead to a larger-scale problem to be solved, requiring much computation resources and cost of time for all the simulation runs.
Furthermore, Figure 8c and d reveal that modifications to both internal and external interdependence of organizational tasks (i.e., here, the corresponding \(K\) and \(S\) values) produce notable fluctuations of the simulation outputs such as \(L_{s}\) and \(H\). As \(K\) and \(S\) values increase, the increment of internal and external interdependences of the organizational tasks that each agent in the community repetitively faces respectively strengthens the connection between individual actions within and between different organizational tasks. This can create resistance and difficulties in modifying individual actions, leading to a reduction in the level of the behavioral synchrony among agents (e.g., relatively small Ls values as shown in Figure 8c and d). In contrast, the mutual impact of \(K\) and \(S\) values on the community’s performance \(H\) exhibits different patterns that requires further exploration in the next step.
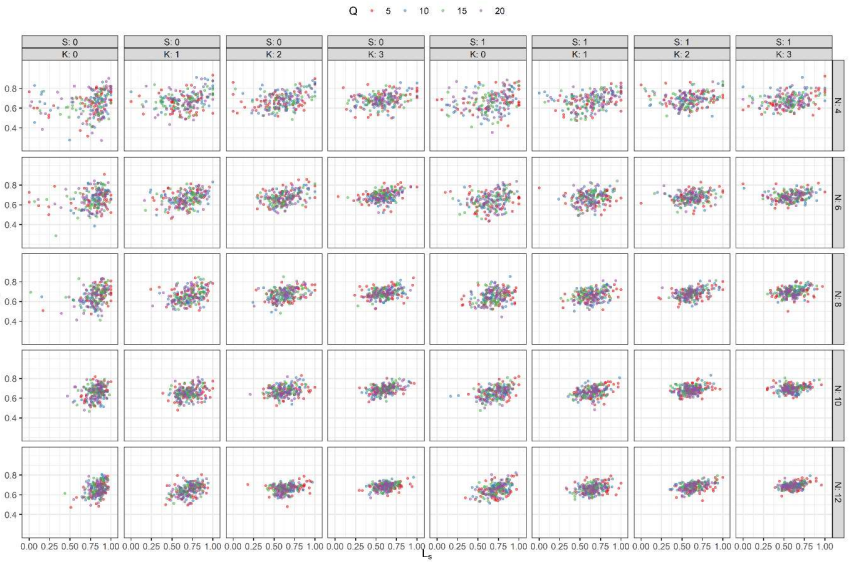
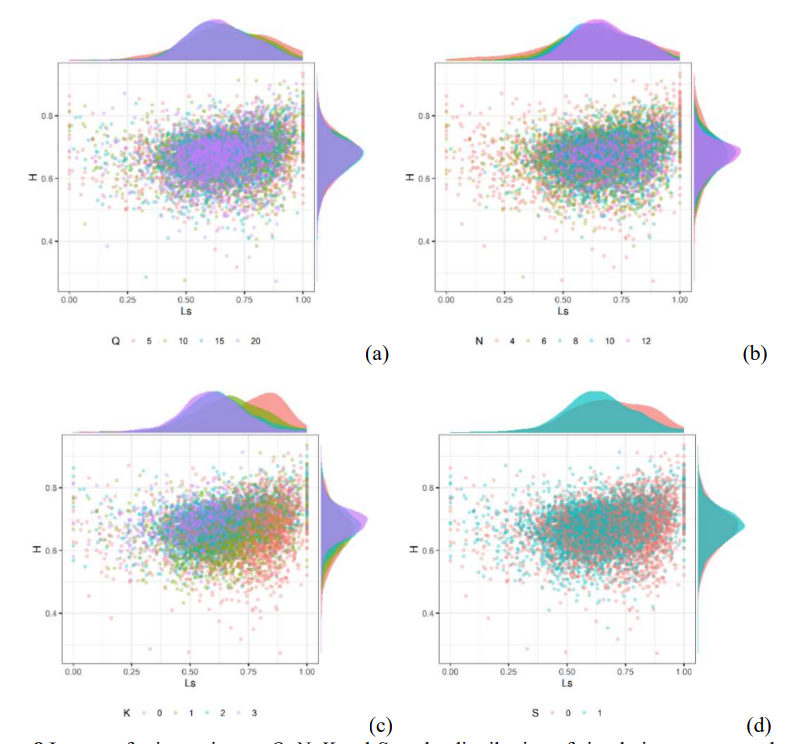
In summary, based on analyzing results under the benchmark scenario as shown above, we confirm that our simulation model behaves robustly to serial variation of primary input parameter values \(Q\), \(N\), \(K\) and \(S\). Gao et al. (2018) argued that ‘the magnitude of participants in routines often varies from case to case’ (p.493). For simplicity and making the trade-off of computation resources, in the following sections, we set the community size \(Q = 10\) and the indicator of component complexity of organizational tasks \(N = 8\), and investigate simulation results of several scenarios with different parameter configurations of \(K\) and \(S\). However, we have that \(0 \leq K \leq N - 1\) drawing on Kauffman & Johnsen (1991)’s(1991) work on the NKCS model. In order to test the impact of extreme \(K\) values, we hereafter change its default setting as that \(K = 0, 1, 3, 7\).
We then check the consequences of non-parameter inputs including both exploitation and exploration rules of the agents (i.e., \(isExplore = isExploit = FALSE, TRUE\)). We set the actual number of simulation runs for each parameter configuration \(numSimRuns_{actual} = 150\) with the estimated expected power (EEP) bigger than 0.90, and keep each simulation run lasts 6000 rounds. We calculate mean values of each simulation output over all simulation runs (as shown in Figure 9a and 9b), from which we conclude that:
(1) Exploitation generally outperforms exploration, resulting in higher levels of both synchrony of actions among agents \(L_{s}\) and the community performance \(H\). However, when the parameter \(K\) equals to big values such as 3 and 7 (as shown in the first two rows of the graphs), the dominance of exploitation on \(L_{s}\) is weakened. The advantage of exploitation over exploration is due to the fact that although exploration can generate novelty through distant or long jump of strategic search, the bounded capacity of agents to only communicate and imitate from finite number of connected partners limits opportunities for the exploration. Furthermore, the dissimilarity of the locally living environment between agents also impedes human actors from the imitating and exploring progress.In contrast, exploitation optimizes behavioral strategies of agents gradually during the repetitive accomplishment of organizational tasks. This leads to a high synchrony level of individual actions (\(L_{s}\)) especially when component actions within each task are loosely coupled (i.e., the parameter \(K\) equals to small values like 0 and 1 in the graphs, see the red and blue curves in the top row of Figure 9a). However, despite improving the community’s performance \(H\), exploitation spends much more simulation ticks to reach the stable status compared to exploration (see in the top row of Figure 9b). Additionally, our findings indicate that exploration and exploitation, when combined, exhibit some synergistic effect – i.e., resulting in much better performance than either approach alone, as shown in the bottom row of graphs in Figure 9a and 9b.
(2) Increasing the coupling relationship of actions both within and between organizational tasks (i.e., the increase of \(K\) and \(S\) values) leads to resistance and difficulties in changing individual actions carried out by agents in the community. For every assembly of exploitation and exploration rule settings, this stickiness of individual actions remarkably reduces the behavioral synchrony level among agents (i.e., relatively small values of the output parameter \(L_{s}\) as shown in Figure 9a). However, as the value of the parameter \(K\) increases, it has less influence on the routines system (e.g., when \(K = 3\) and 7 as indicated by the green and purple curves in Figure 9a). This effect is especially evident in the situation when the parameter value \(S\) equals to 1 (see in the right column of Figure 9a).The stickiness of individual actions within rather than between organizational tasks (i.e., indicated by the parameter \(K\), instead of \(S\) values) operates more effective in helping the routine system resist random variations, resulting a high performance of the community of agents (i.e., with high H values as shown in Figure 9b). However, there is no significant impact of \(K\) values in the situation when only the exploration rule works (see in the middle row of Figure 9b).
When comparing the impact of \(K\) and \(S\) values on the simulation outputs, we observe the very distinct patterns as demonstrated in Figure 9a and 9b – i.e., increasing the stickiness of individual actions (e.g., the increase of either \(K\) or \(S\) values) to a certain degree decreases the synchrony level of actions \(L_{s}\), but it positively affects the community’s performance \(H\). This implies that organizational designers need to make a trade-off between these two factors.
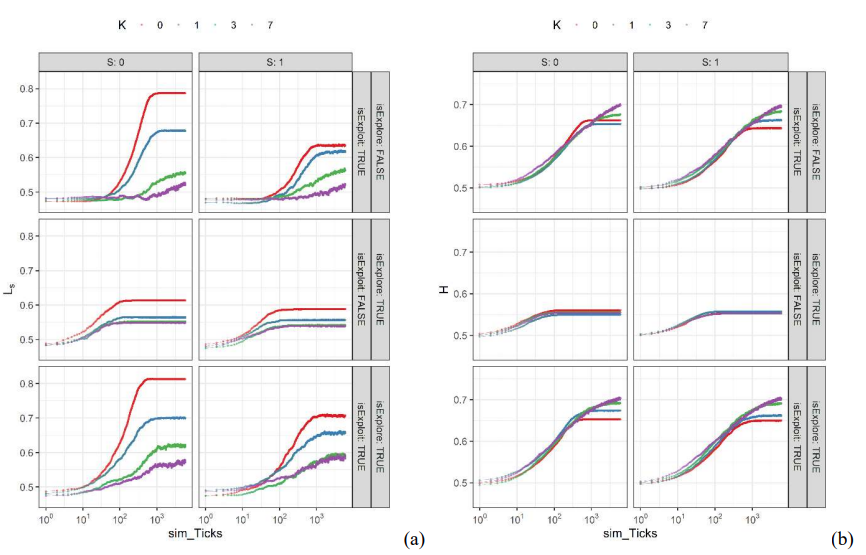
Our simulation model treated the alternative specifications of non-parametric elements, namely exploitation and exploration rules followed by the agents as the levels of categorical variables (Borgonovo et al. 2022). By doing so, we evaluate the model’s performance under the benchmark scenario to determine the contribution of agents’ behavioral rules to the variance of simulation outputs. From the analyzing results as presented above, we can confirm the validity of our simulation model.
Simulation results
In this section, we discuss the impact of power asymmetry among the interpersonal interrelationship of agents within the community by letting the network structure \(net\) = ‘Star-shape’ and ‘Fully-connected’ in accordance with the input settings of Scenario I. Following the design of experiments (DOE) principles (Lorscheid et al. 2012), we use the full factorial experimental design solution and set the actual number of simulation runs for each parameter configuration \(numSimRuns_{actual} = 100\) with the estimated expected power (EEP) greater than 0.90, and keep each simulation run lasts 8000 rounds. We then calculate mean output values for each parameter configuration over all simulation when they reach stable status (if such stable a status exists), as shown in Figure 10.
Regarding the exploitation, we conclude that the different level of power asymmetry over interpersonal interrelationships (i.e., represented by the Star-shape and Fully-connected network structures in our model) determines the way of how competitive pressures diffuse across all the linked neighbors, which arises the possibility for the exploitative activities to occur. Simulation results as shown in the top line of Figure 10a and 10b indicate that the Star-shape network (i.e., a high level of power asymmetry) behaves much better than that of the Fully-connected network structure (i.e., a low level of power asymmetry) resulting in bigger values of the synchrony of individual actions \(L_{s}\) and the community’s performance \(H\). This is because the centralized power in the community, which facilitates the transmission of competitive pressures from person to person, thereby stimulating the agents’ exploitative activities. However, the tight coupling relationship of actions within and between organizational tasks (i.e., the increase of the \(K\) and \(S\) values), to some extent helps the routines system resist variations, resulting in a decrease of the synchrony of individual behaviors \(L_{s}\), but an increase of the organizational performance H. Further, we find that the stickiness of actions across different organizational tasks (e.g., \(S = 1\)) would limit agents’ possibility to exploit and vary their contemporary actions, weaking the effect of different network structures.
In terms of exploration (e.g., as shown in the middle line of Figure 10a and 10b, the Fully-connected network facilitates communication and imitation between agents. This promotes distant or long jump of strategic search of agents. Therefore, the Fully-connected network performs better than that of the Star-shape network with much higher levels of the synchrony of individual actions \(L_{s}\) and organizational performance \(H\). Additionally, although the variation of \(K\) and \(S\) values to a large extent affects the output \(L_{s}\), it has no significant impact on organizational performance \(H\).
Furthermore, we observed both exploration and exploitation as shown in the bottom line of Figure 10a and 10b, and found the combined effect. With the loose coupling relationship of actions within and between organizational tasks (e.g., small \(K\) and \(S\) values), the exploitation dominates the agents’ strategic search and optimizing process. In this case, the Star-shape network works much better in comparison with the Fully-connected network. By increasing the stickiness of actions either within or between organizational tasks (e.g., \(K = 3\) and \(7\); \(S = 1\)), exploration replaces exploitation and becomes a crucial role during the strategic search and optimizing process of agents across the community. Thereby, the Fully-connected network outperforms Star-shape network. By doing this, human actors’ explorative and exploitative activities constantly intertwine with the organizational structure to match the near-decomposability characteristic of organizational tasks, keeping the routines system from becoming either rigidity or collapse.
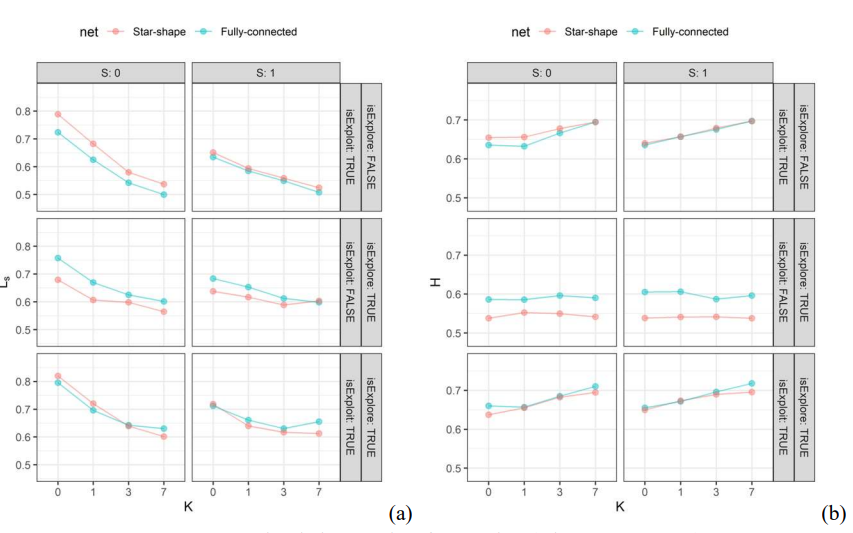
When we consider the possibility of errors occurring in imitation between agents by following the input settings of Scenario II (where, for example, \(p_{error} = 0.01\)), it is obvious that those errors of imitation have no influence on human actors’ exploitative activities, but only affect the fulfillment of explorative activities by yielding a diversity of alternative solutions and expanding the strategic search space of agents across the community. The simulation results shown in the top line of Figure 11a and 11b indicate that such a minor but random disturbance in imitation and exploration do not lead to the fluctuation of the routines system. Instead, it is beneficial to ease and fully accelerate the strategic search and optimizing processes of agents. As a result, we can observe the consequential improvement of simulation outputs for both Star-shape and Fully-connected networks. Furthermore, when \(p_{error} > 0\), the Fully-connected network strengthens the propagation of novelty originated from imitation errors by facilitating communication and imitative activities among agents. This explains why Fully-connected network behaves better than that of the Star-shape network.
In addition, we observed the very similar patterns as those appeared in Scenario I for the situation with both exploration and exploitation as shown in the bottom line of Figure 11a and 11b. However, the trend of these patterns is perceptibly intensified due to the impact of errors in imitation and exploration (e.g., the increase of the perror values). This happens when the loose coupling relationship of actions within and between organizational tasks exists, the exploitation dominates agents’ strategic search and optimizing process, emphasizing the step-by-step strategic search and optimization to prevent the routine system from collapsing. In this context, the Star-shape network outperforms the Fully-connected network at facilitating the diffusion of competitive pressures and providing opportunities for the exploitative activity. In contrary, the tight coupling relationship of actions within and between organizational tasks requires the exploration to take control of the strategic search and optimizing process to prevent routine systems from becoming rigid, calling for the Fully-connected network to seek for an adaptive changing performance in the community.
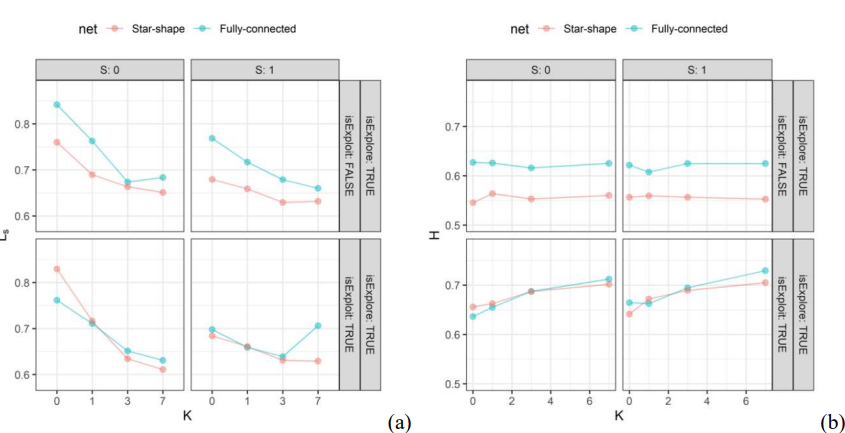
Discussion
This study aims to comprehend human actors’ exploration and exploitation regarding artifacts while repetitively enacting organizational routines. A brief overview of literature outlined the development trajectory of the scholars’ understanding of the role of artifacts and the materiality in routines performances, and addressed those artifacts as the nexus connecting individual situated actions to the collective outcome as patterned routines (Gao et al. 2018; Gao 2019). To investigate the logic between artifacts-based exploration and exploitation on the one hand, and the ‘(re)framing-overflowing’ interaction loop amidst routine performances and artifacts as artifactual representations (D’Adderio 2008, 2011) on the other, we conducted an agent-based modeling study on the formation dynamics of organizational routines over time from the ‘bottom-up’.
The results reveal the complex matching relations among (1) the interdependences between situated-actions within and between organizational tasks, (2) artifacts-based explorative and exploitative activities carried out by human actors, and (3) organizational structures or the power asymmetry that characterizes the interpersonal interrelationship across the routines system, as represented in Figure 12. Such compatible relations of the three key points specify the micro-foundation and mechanisms underpinning routines' formation dynamics over time. This theoretically contributes to the literature on routine dynamics research, and further provides suggestions on designing routine performances (Glaser 2017; Murray et al. 2021; Pentland & Feldman 2008) vis-à the artifacts design for the managerial practice.
Firstly, human actors’ explorative and exploitative activities provide a profitable but new opportunity to consider the role of artifacts and materiality during the enactment of organizational routines. Scholars challenged the traditional view on technical or material determinism (Leonardi & Barley 2008; Orlikowski 2007), through engaging practice theory and addressing routines as effortful and emergent accomplishments (Feldman 2000; Feldman & Pentland 2003; Pentland & Rueter 1994), rather than mindless, rigid, and mundane (Cohen 2007). Artifacts such as written rules, machines and technology provide guidance and control for the enactment of routines by delimiting and closing human actors’ search spaces. However, they are often incomplete and do not perfectly determine actions. Pentland & Feldman (2008) admitted the difference between design and use of artifacts, and argued that ‘designing things while hope for patterns of action is a mistake’ (p.236). One of the reasons is that human actors can always (re)interpret and make changes to the guidance provided by artifacts (D’Adderio 2008; Geiger & Schröder 2014). The personal experience, cognitive capacity, and mindfulness of those human actors in either exploration or exploitation regarding existing artifacts largely explains why the gap between routines performances and the artifacts as artifactual representations is oftentimes addressed in literature (e.g., Desai 2010; Aroles & McLean 2016; Geiger & Schröder 2014; Glaser 2017). It also addresses sources of the novelty and change originate in the course of routines.
Secondly, considering the impact of human actors’ exploration and exploitation on routines performances, it is worth of theorizing the development and use of artifacts simultaneously (Glaser 2017; Pollock & Williams 2016). The material properties of artifacts established during the design maintain constraints and affordances for human actors to act and interact (D’Adderio 2008). However, those symbolic messages embedded in various artifacts and the materiality are incomplete and requires interpretation for routines performances (Cacciatori 2012). Human actors with bounded capacity have their discretion to interpret, and further explore and exploit the symbolic messages embodied and displayed in artifacts (D’Adderio 2008). This leads to the divergence or the drift of routines actual performances from the intended design (D’Adderio 2008, 2011; Pentland et al. 2020; Pentland & Feldman 2008).
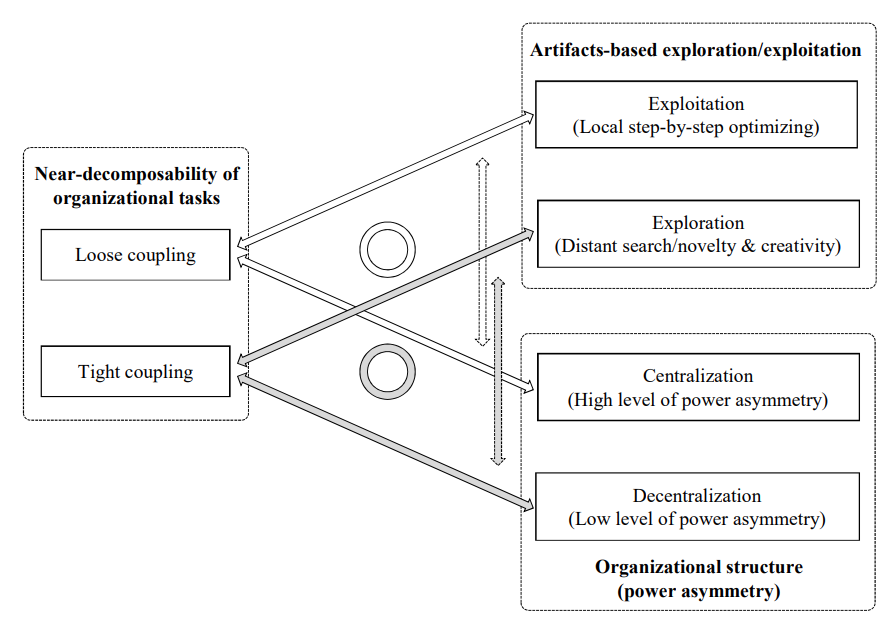
Intentional deviations from the formal rules and procedures inherent in material artifacts could lead to negative consequences such as problematic performance shortfalls, but potentially benefit the organization in the long term (Appelbaum et al. 2007; Desai 2010). Based on the compatible relations as shown in Figure 12, we have proposed a guideline for artifacts and performances design (Glaser 2017; Pentland & Feldman 2008), taking into account of internal and external interdependences characteristic of organizational tasks. That is, when dealing with loose coupling organizational tasks, centralized organizational structure (i.e., represented by the high-power asymmetry and the Star-shape network) facilitates the diffusion of competitive pressures across the community. This promotes exploitative activities and enhances organizational effectiveness, simultaneously keeps the routines system from breakdown. To achieve this, those formal rules and procedures inherent in the material artifacts need to be processual and descriptive enough to enforce the consistency of individual actions (Gao et al. 2018), encouraging exploitative activities for gradually step-by-step strategic search and optimization.
When dealing with tight coupling organizational tasks, decentralized organizational structure (i.e., represented by the low power asymmetry and the Fully-connected network) accelerates the communication and imitation between human actors, encouraging exploration across the community to improve fitness with the environment and avoid the rigidity and inertia in routines performances. In such cases, it is better to have those formal rules and procedures of material artifacts which are open and goal-oriented to encourage innovation and creativity (Weichbrodt & Grote 2010) through undertaking explorative activities.
Nonetheless, it worth noting that many contemporary organizations are multinational corporations located in various countries and regions (Puranam et al. 2014; Zimmermann 2011). These organizations often use advanced information and communication technologies (ICTs) much more than face-to-face meetings to communicate and coordinate between employees. Deep and interactive communication can increase the perceived proximity of virtual members, moderate the negative effect of virtuality (Wilson et al. 2008; Zimmermann 2011), and facilitate explorative activities. Decentralization of organizational structure and effective communication becomes even more crucial when organizational tasks get complex (Blanco-Fernández et al. 2022). Therefore, we assert that a careful balance between the task complexity, organizational structures and artifacts design is always desirable.
Finally, the disturbance, even the minor ones during human actors’ imitation and exploration vis-à existing artifacts are worth of paying attention to. This is because imperfect imitation and exploration including the intended improvisation or accidental errors in strategic copying could lead to essential novelties. This increases search opportunities (Lazer & Friedman 2007), and expand the potential space examined by multiple human actors, resulting in a high level of organizational performance. When it comes to interdependent organizational tasks and/or routines, both intended and unintended variations are of strategic importance for organizations to promote innovation and creativity (Deken et al. 2016; Sonenshein 2016). However, Zbaracki & Bergen (2010) found that introducing large variations in those interdependent actions would significantly breakdown routines. Routine dynamics research has shown that routines are effortful and emergent accomplishments (Feldman et al. 2016). Therefore, we should perpetually tolerate the minor disturbance during the repetitive enactment of routines. This leads to an open question of how such disturbances/variations (e.g., either intended or unintended) affect routine formation dynamics over time, which is worth of exploring in the future work.
Conclusion
Artifacts and the materiality are important in shaping the course of organizational routines (Cacciatori 2012; D’Adderio 2008, 2011). Those material artifacts provide constraints and affordances for multiple human actors, also they act as intermediaries regulate the interactions between the ostensive and performative aspects of patterned routines. This paper focuses on investigating the ways human actors engage in artifacts-based explorative and exploitative activities. We treat those material artifacts as the nexus connecting individual situated actions with the collective phenomena such as organizational routines (Gao et al. 2018; Gao 2019). The essential logic beneath such a micro-macro link created by artifacts and the materiality allows us to conduct an agent-based modeling study on the formation dynamics of organizational routines from the ‘bottom-up’.
The contributions of our research work are twofold. Firstly, we contribute to the literature of routine dynamics by uncovering multiple human actors’ artifacts-based explorative and exploitative activities. The finding provides insight of how social and material settings would affect routines’ formation over time. Secondly, our simulation results display the compatible relations of the three key points including interdependences between situated-actions within and between organizational tasks, artifacts-based explorative and exploitative activities carried out by multiple human actors, and organizational structures or the power asymmetry characterizing the interpersonal interrelationship across the routines system. These complex matching relations further provide managers in practice with appropriate guidelines for designing routine performances vis-à the artifacts design.
We acknowledge that our study has limitations. Firstly, we employed a typified agent-based model to test the theoretical ideas emerged from literature, which can be perceived to lack of empirical evidence. Hence, the findings should be further verified by empirical data in the future work. The limitation of being abstract and lack of empirical evidence is often associated with studies using agent-based model (Boero & Squazzoni 2005). However, engaging practice theory in routine dynamics research during the past two decades has been emphasizing the actual performances of routines (Feldman & Orlikowski 2011) and calls for using the complicated empirical simulation models to capture the natural world of organizational routines (Gao 2021; Gao & Akbaritabar 2022). To address this limitation, future studies could use well-grounded empirical data to validate and calibrate input settings of the simulation model. We believe integrating simulation models with fieldwork will increase the descriptive capacity and ensure validity of those simulation models, hence building confidence and trust in the simulation findings (Edmonds & Moss 2004; Gao & Akbaritabar 2022).
Secondly, we drew our discussion from the concept community (Cohendet & Llerena 2003) and followed March (1991)’s (1991) framework of exploration and exploitation regarding material artifacts. Though the clear focus on these two concepts allowed us to analyze the intricate relationship between the various relevant factors in depth, we limited our discussion at the community level. Our robustness testing shows that the variation in community size (e.g., ranging from 5 to 20) has no significant impacts on simulation outputs including the synchrony of action sequences and the performance of the community. We primarily used random network as the benchmark, and considered the classic Star-shape and Fully-connected network models to represent vertical and horizontal organizational structures as the two ends of the spectrum of power asymmetry. However, Lazer & Friedman (2007) and Fang et al. (2010) developed their simulation models at the organization scale with the population of 100 and 280 individual agents, respectively. Their models highlighted the importance of much more complicated organizational structures such as semi-isolated subgroups in achieving the balance of exploration and exploitation. We do not believe our analyzing results can be directly extrapolated to the organization level. Instead, some modifications are necessary when discussing routines formation at the organizational level.
Furthermore, it is important to note that our model assumes artifacts and the materiality involved in the routines system keep unchanged during the simulation runs, meaning that learning activities just occur among individual human actors. The model does not consider organizational learning that encodes knowledge from higher-performing individuals into artifacts or even creates new artifacts. In this case, coordination (i.e., the synchrony of individual actions) seems more like an emerging result, rather than as an essential mechanism underpinning routines’ formation or even organizational adaptation dynamics (Koçak et al. 2022). Further study of routine dynamics could address this limitation by considering both individual and organizational learning simultaneously in the pursuit of the balance of exploration and exploitation with regards to artifacts. This can be achieved through extending this model in the future.
Acknowledgements
The research has been supported by Shandong Provincial Natural Science Foundation (under Grant No. ZR2020MG023) and College Youths’ Innovative Science & Technology Program of Shandong Province (No. 2020RWG007). The authors also would like to thank the two anonymous reviewers for their constructive comments, and thank the experts of the ESSA@work session at the Social Simulation Conference 2022 (September 12-16, Milan Italy) for their help on an early version of the work.
Conflict of Interest
The authors declare that they have no conflict of interest.
References
ALMAHENDRA, R., & Ambos, B. (2015). Exploration and exploitation: A 20-year review of evolution and reconceptualisation. International Journal of Innovation Management, 19(01), 1550008. [doi:10.1142/s1363919615500085]
APPELBAUM, S. H., Iaconi, G. D., & Matousek, A. (2007). Positive and negative deviant workplace behaviors: Causes, impacts, and solutions. Corporate Governance: The International Journal of Business in Society, 7(5), 586–598. [doi:10.1108/14720700710827176]
AROLES, J., & McLean, C. (2016). Rethinking stability and change in the study of organizational routines: Difference and repetition in a newspaper-printing factory. Organization Science, 27(3), 535–550. [doi:10.1287/orsc.2015.1035]
BAPUJI, H., Hora, M., & Saeed, A. M. (2012). Intentions, intermediaries, and interaction: Examining the emergence of routines. Journal of Management Studies, 49(8), 1586–1607. [doi:10.1111/j.1467-6486.2012.01063.x]
BAUM, J. A. C., Li, S. X., & Usher, J. M. (2000). Making the next move: How experiential and vicarious learning shape the locations of chains’ acquisitions. Administrative Science Quarterly, 45(4), 766–801. [doi:10.2307/2667019]
BECKER, M. C. (2004). Organizational routines: A review of the literature. Industrial and Corporate Change, 13(4), 643–678. [doi:10.1093/icc/dth026]
BECKER, M. C. (2008). The past, present and future of organizational routines: Introduction to the handbook of organizational routines. In M. C. Becker (Ed.), The handbook of organizational routines (pp. 3–14). Edward Elgar. [doi:10.4337/9781848442702.00006]
BLANCO-FERNÁNDEZ, D., Leitner, S., & Rausch, A. (2022). The benefits of coordination in (over)adaptive virtual teams. The Social Simulation Conference 2022, September 12-16, Milan Italy
BOERO, R., & Squazzoni, F. (2005). Does empirical embeddedness matter?Methodological issues on agent-based models for analytical social science. Journal of Artificial Societies and Social Simulation, 8(4), 6.
BORGONOVO, E., Pangallo, M., Rivkin, J., Rizzo, L., & Siggelkow, N. (2022). Sensitivity analysis of agent-based models: A new protocol. Computational and Mathematical Organization Theory, 28, 52–94. [doi:10.1007/s10588-021-09358-5]
CACCIATORI, E. (2012). Resolving conflict in problem-solving: Systems of artefacts in the development of new routines. Journal of Management Studies, 49(8), 1559–1585. [doi:10.1111/j.1467-6486.2012.01065.x]
CIVETTINI, N. (2007). Similarity and group performance. Social Psychology Quarterly, 70(3), 262–271.
COHEN, M. D. (2007). Reading Dewey: Reflections on the study of routine. Organization Studies, 28(5), 773–786. [doi:10.1177/0170840606077620]
COHENDET, P., & Llerena, P. (2003). Routines and incentives: The role of communities in the firm. Industrial and Corporate Change, 12(2), 271–297. [doi:10.1093/icc/12.2.271]
CSASZAR, F. A., & Siggelkow, N. (2010). How much to copy? Determinants of effective imitation breadth. Organization Science, 21(3), 661–676. [doi:10.1287/orsc.1090.0477]
D’ADDERIO, L. (2008). The performativity of routines: Theorising the influence of artefacts and distributed agencies on routines dynamics. Research Policy, 37(5), 769–789. [doi:10.1016/j.respol.2007.12.012]
D’ADDERIO, L. (2011). Artifacts at the centre of routines: Performing the material turn in routines theory. Journal of Institutional Economics, 7(2), 197–230.
DANNER-SCHRÖDER, A. (2021). Without actors, there is no action: How interpersonal interactions help to explain routine dynamics. Review of Managerial Science, 15(7), 1913–1936.
DEKEN, F., Carlile, P. R., Berends, H., & Lauche, K. (2016). Generating novelty through interdependent routines: A process model of routine work. Organization Science, 27(3), 659–677. [doi:10.1287/orsc.2016.1051]
DEN Hamer, P., & Frenken, K. (2021). A network-based model of exploration and exploitation. Journal of Business Research, 129, 589–599. [doi:10.1016/j.jbusres.2019.12.040]
DESAI, V. M. (2010). Rule violations and organizational search: A review and extension. International Journal of Management Reviews, 12(2), 184–200. [doi:10.1111/j.1468-2370.2008.00245.x]
DIONYSIOU, D. D., & Tsoukas, H. (2013). Understanding the (re)creation of routines from within: A symbolic interactionist perspective. Academy of Management Review, 38(2), 181–205. [doi:10.5465/amr.2011.0215]
EDMONDS, B., & Moss, S. (2004). From KISS to KIDS - An “anti-simplistic” modelling approach. In P. Davidsson, B. Logan, & K. Takadama (Eds.), Multi-Agent and Multi-Agent-Based simulation (pp. 130–144). Heidelberg, Berlin: Springer. [doi:10.1007/978-3-540-32243-6_11]
FANG, C., Lee, J., & Schilling, M. A. (2010). Balancing exploration and exploitation through structural design: The isolation of subgroups and organizational learning. Organization Science, 21(3), 625–642. [doi:10.1287/orsc.1090.0468]
FELDMAN, M. S. (2000). Organizational routines as a source of continuous change. Organization Science, 11(6), 611–629. [doi:10.1287/orsc.11.6.611.12529]
FELDMAN, M. S. (2021). Practice theory and routine dynamics. In M. S. FELDMAN, B. T. Pentland, L. D’Adderio, K. Dittric, C. Rerup, & D. Seidl (Eds.), Cambridge handbook of routine dynamics (pp. 21–36). Cambridge: Cambridge University Press. [doi:10.1017/9781108993340.002]
FELDMAN, M. S., & Orlikowski, W. J. (2011). Theorizing practice and practicing theory. Organization Science, 22(5), 1240–1253. [doi:10.1287/orsc.1100.0612]
FELDMAN, M. S., & Pentland, B. T. (2003). Reconceptualizing organizational routines as a source of flexibility and change. Administrative Science Quarterly, 48(1), 94–118. [doi:10.2307/3556620]
FELDMAN, M. S., Pentland, B. T., D’Adderio, L., & Lazaric, N. (2016). Beyond routines as things: Introduction to the special issue on routine dynamics. Organization Science, 27(3), 505–513. [doi:10.1287/orsc.2016.1070]
FELDMAN, M. S., & Rafaeli, A. (2002). Organizational routines as sources of connections and understandings. Journal of Management Studies, 39(3), 309–331. [doi:10.1111/1467-6486.00294]
FELIN, T., & Foss, N. J. (2009). Organizational routines and capabilities: Historical drift and a course-correction toward microfoundations. Scandinavian Journal of Management, 25(2), 157–167. [doi:10.1016/j.scaman.2009.02.003]
GAO, D. (2019). Simulating the role of leadership in artifacts-enabled formation of organizational routines. The 35th EGOS Colloquium, Sub-theme 06: Routine Dynamics: New Ways of Organizing, July 4-6, Edinburgh, UK
GAO, D. (2021). Agent-based modeling in routine dynamics. In M. S. Feldman, B. T. Pentland, L. D’Adderio, K. Dittric, C. Rerup, & D. Seidl (Eds.), Cambridge handbook of routine dynamics (pp. 159–171). Cambridge: Cambridge University Press. [doi:10.1017/9781108993340.002]
GAO, D., & Akbaritabar, A. (2022). Using agent-based modeling in routine dynamics research: A quantitative and content analysis of literature. Review of Managerial Science, 16(2), 521–550. [doi:10.1007/s11846-021-00446-z]
GAO, D., Squazzoni, F., & Deng, X. (2018). The role of cognitive artifacts in organizational routine dynamics: An agent-based model. Computational and Mathematical Organization Theory, 24(4), 473–499. [doi:10.1007/s10588-018-9263-y]
GEIGER, D., & Schröder, A. (2014). Ever-changing routines? Toward a revised understanding of organizational routines between rule-following and rule-breaking. Schmalenbach Business Review, 66(2), 170–190. [doi:10.1007/bf03396904]
GLASER, V. L. (2017). Design performances: How organizations inscribe artifacts to change routines. Academy of Management Journal, 60(6), 2126–2154. [doi:10.5465/amj.2014.0842]
GÓMEZ-CRUZ, N. A., Loaiza Saa, I., & Ortega Hurtado, F. F. (2017). Agent-based simulation in management and organizational studies: A survey. European Journal of Management and Business Economics, 26(3), 313–328.
GUPTA, A. K., Smith, K. G., & Shalley, C. E. (2006). The interplay between exploration and exploitation. Academy of Management Journal, 49(4), 693–706. [doi:10.5465/amj.2006.22083026]
HODGSON, G. M. (2008). The concept of a routine. In M. C. Becker (Ed.), The handbook of organizational routines (pp. 15–28). Cheltenham, UK: Edward Elgar.
HOWARD-GRENVILLE, J. A. (2005). The persistence of flexible organizational routines: The role of agency and organizational context. Organization Science, 16(6), 618–636. [doi:10.1287/orsc.1050.0150]
KAHL, C. H., & Meyer, M. (2016). Constructing agent-based models of organizational routines. In D. Secchi & M. Neumann (Eds.), Agent-based simulation of organizational behavior: New frontiers of social science research (pp. 85–107). Cham: Springer. [doi:10.1007/978-3-319-18153-0_5]
KAUFFMAN, S. A. (1993). The origins of order: Self-Organization and selection in evolution. Oxford: Oxford University Press.
KAUFFMAN, S. A., & Johnsen, S. (1991). Coevolution to the edge of chaos: Coupled fitness landscapes, poised states, and coevolutionary avalanches. Journal of Theoretical Biology, 149(4), 467–505. [doi:10.1016/s0022-5193(05)80094-3]
KNUDSEN, T. (2008). Organizational routines in evolutionary theory. In M. C. Becker (Ed.), The handbook of organizational routines (pp. 125–151). Cheltenham, UK: Edward Elgar. [doi:10.4337/9781848442702.00014]
KOÇAK, Ö., Levinthal, D. A., & Puranam, P. (2022). The dual challenge of search and coordination for organizational adaptation: How structures of influence matter. Organization Science, 34(2).
LAVIE, D., Kang, J., & Rosenkopf, L. (2011). Balance within and across domains: The performance implications of exploration and exploitation in alliances. Organization Science, 22(6), 1517–1538. [doi:10.1287/orsc.1100.0596]
LAZER, D., & Friedman, A. (2007). The network structure of exploration and exploitation. Administrative Science Quarterly, 52(4), 667–694. [doi:10.2189/asqu.52.4.667]
LEONARDI, P. M., & Barley, S. R. (2008). Materiality and change: Challenges to building better theory about technology and organizing. Information and Organization, 18(3), 159–176. [doi:10.1016/j.infoandorg.2008.03.001]
LEVITAN, B., Lobo, J., Schuler, R., & Kauffman, S. (2002). Evolution of organizational performance and stability in a stochastic environment. Computational & Mathematical Organization Theory, 8(4), 281–313. [doi:10.1023/a:1025416617948]
LIN, H., Chen, M., & Su, J. (2017). How management innovations are successfully implemented? An organizational routines’ perspective. Journal of Organizational Change Management, 30(4), 456–486. [doi:10.1108/jocm-07-2016-0124]
LIN, H., Qu, T., & Hu, Y. (2021). How do organizational routines paradoxically affect organizational innovation? European Journal of Innovation Management, 24(4), 1400–1429. [doi:10.1108/ejim-03-2020-0093]
LORSCHEID, I., Heine, B.-O., & Meyer, M. (2012). Opening the “black box” of simulations: Increased transparency and effective communication through the systematic design of experiments. Computational and Mathematical Organization Theory, 18(1), 22–62. [doi:10.1007/s10588-011-9097-3]
MARCH, J. G. (1991). Exploration and exploitation in organizational learning. Organization Science, 2(1), 71–87. [doi:10.1287/orsc.2.1.71]
MITCHELL, M. (1996). An introduction to genetic algorithms. Cambridge, MA: MIT Press.
MURRAY, A., Rhymer, J., & Sirmon, D. G. (2021). Humans and technology: Forms of conjoined agency in organizations. Academy of Management Review, 46(3), 552–571. [doi:10.5465/amr.2019.0186]
NELSON, R. R., & Winter, S. (1982). An evolutionary theory of economic change. Cambridge, MA: Harvard University Press.
NIGAM, A., Huising, R., & Golden, B. (2016). Explaining the selection of routines for change during organizational search. Administrative Science Quarterly, 61(4), 551–583. [doi:10.1177/0001839216653712]
ORLIKOWSKI, W. J. (2007). Sociomaterial practices: Exploring technology at work. Organization Studies, 28(9), 1435–1448. [doi:10.1177/0170840607081138]
PARMIGIANI, A., & Howard-Grenville, J. (2011). Routines revisited: Exploring the capabilities and practice perspectives. Academy of Management Annals, 5(1), 413–453. [doi:10.5465/19416520.2011.589143]
PENTLAND, B. T., & Feldman, M. S. (2005). Organizational routines as a unit of analysis. Industrial and Corporate Change, 14(5), 793–815. [doi:10.1093/icc/dth070]
PENTLAND, B. T., & Feldman, M. S. (2008). Designing routines: On the folly of designing artifacts, while hoping for patterns of action. Information and Organization, 18(4), 235–250. [doi:10.1016/j.infoandorg.2008.08.001]
PENTLAND, B. T., & Hærem, T. (2015). Organizational routines as patterns of action: Implications for organizational behavior. Annual Review of Organizational Psychology and Organizational Behavior, 2(1), 465–487. [doi:10.1146/annurev-orgpsych-032414-111412]
PENTLAND, B. T., Liu, P., Kremser, W., & Hærem, T. (2020). The dynamics of drift in digitized processes. MIS Quarterly, 44(1), 19–47. [doi:10.25300/misq/2020/14458]
PENTLAND, B. T., PENTLAND, A. P., & Calantone, R. J. (2017). Bracketing off the actors: Towards an action-centric research agenda. Information and Organization, 27(3), 137–143. [doi:10.1016/j.infoandorg.2017.06.001]
PENTLAND, B. T., & Rueter, H. H. (1994). Organizational routines as grammars of action. Administrative Science Quarterly, 39(3), 484–510. [doi:10.2307/2393300]
POLLOCK, N., & Williams, R. (2016). How industry analysts shape the digital future. Oxford: Oxford University Press. [doi:10.1093/acprof:oso/9780198704928.001.0001]
PURANAM, P., Alexy, O., & Reitzig, M. (2014). What’s “new” about new forms of organizing? Academy of Management Review, 39(2), 162–180. [doi:10.5465/amr.2011.0436]
REYNAUD, B. (2005). The void at the heart of rules: Routines in the context of rule-following. The case of the Paris Metro Workshop. Industrial and Corporate Change, 14(5), 847–871. [doi:10.1093/icc/dth073]
SARGIS-ROUSSEL, C., Belmondo, C., & Deltour, F. (2017). Bringing people back in: How group internal social capital influences routines’ emergence. European Management Review, 14(1), 101–112. [doi:10.1111/emre.12100]
SECCHI, D., & Seri, R. (2017). Controlling for false negatives in agent-based models: A review of power analysis in organizational research. Computational and Mathematical Organization Theory, 23(1), 94–121. [doi:10.1007/s10588-016-9218-0]
SIMON, H. A. (1997). Models of bounded rationality: Empirically grounded economic reason. Cambridge, MA: MIT press. [doi:10.7551/mitpress/4711.001.0001]
SONENSHEIN, S. (2016). Routines and creativity: From dualism to duality. Organization Science, 27(3), 739–758. [doi:10.1287/orsc.2016.1044]
STADLER, C., Rajwani, T., & Karaba, F. (2014). Solutions to the exploration/exploitation dilemma: Networks as a new level of analysis. International Journal of Management Reviews, 16(2), 172–193. [doi:10.1111/ijmr.12015]
TURNER, S. F., & Rindova, V. (2012). A balancing act: How organizations pursue consistency in routine functioning in the face of ongoing change. Organization Science, 23(1), 24–46. [doi:10.1287/orsc.1110.0653]
VEREL, S., Liefooghe, A., Jourdan, L., & Dhaenens, C. (2013). On the structure of multiobjective combinatorial search space: MNK-landscapes with correlated objectives. European Journal of Operational Research, 227(2), 331–342. [doi:10.1016/j.ejor.2012.12.019]
WEICHBRODT, J., & Grote, G. (2010). Rules and routines in organizations: A review and extension. The Fourth International Conference on Organizational Routines. June 11-12, Nice, France
WILDEN, R., Hohberger, J., Devinney, T. M., & Lavie, D. (2018). Revisiting James March (1991): Whither exploration and exploitation? Strategic Organization, 16(3), 352–369. [doi:10.1177/1476127018765031]
WILSON, J. M., Boyer O’Leary, M., Metiu, A., & Jett, Q. R. (2008). Perceived proximity in virtual work: Explaining the paradox of far-but-close. Organization Studies, 29(7), 979–1002. [doi:10.1177/0170840607083105]
WINTER, S. G., & Szulanski, G. (2001). Replication as strategy. Organization Science, 12(6), 730–743. [doi:10.1287/orsc.12.6.730.10084]
YANG, Y., Gong, Y., Land, L. P. W., & Chesney, T. (2020). Understanding the effects of physical experience and information integration on consumer use of online to offline commerce. International Journal of Information Management, 51(1), 102046. [doi:10.1016/j.ijinfomgt.2019.102046]
ZBARACKI, M. J., & Bergen, M. (2010). When truces collapse: A longitudinal study of price-adjustment routines. Organization Science, 21(5), 955–972. [doi:10.1287/orsc.1090.0513]
ZIMMERMANN, A. (2011). Interpersonal relationships in transnational, virtual teams: Towards a configurational perspective. International Journal of Management Reviews, 13(1), 59–78. [doi:10.1111/j.1468-2370.2010.00284.x]
ZOLLO, M., & Winter, S. G. (2002). Deliberate learning and the evolution of dynamic capabilities. Organization Science, 13(3), 339–351. [doi:10.1287/orsc.13.3.339.2780]