Communicating Agent-Based Models to Stakeholders: A Scoping Review

, ,
and
aUniversité Toulouse Capitole, France; bUniversity of the Philippines Los Baños, Philippines; cUniversité de Toulouse, CNRS, France; dGEODES, CNRS, Université Toulouse Jean Jaures, France; eInstitute of Renewable Natural Resources, Philippines
Journal of Artificial
Societies and Social Simulation 28 (2) 2
<https://www.jasss.org/28/2/2.html>
DOI: 10.18564/jasss.5623
Received: 02-Feb-2024 Accepted: 13-Feb-2025 Published: 31-Mar-2025
Abstract
Agent-based models (ABMs) are widely used in fields such as ecology, natural resource management, and policy planning to simulate complex systems. However, their adoption by stakeholders remains limited, primarily due to challenges in effectively communicating these models. Communicating ABMs to stakeholders involves the methods and tools used to convey a model’s structure, function, and results to facilitate understanding, trust, and application. This scoping review examines 65 articles from major databases, identifying key barriers and gaps in ABM communication. Three major issues emerge: (1) limited efforts to support the use of existing ABMs; (2) insufficient emphasis on explaining the emergence in ABMs; and (3) the absence of standardized methods to evaluate the effectiveness of communication strategies. By mapping current communication strategies and categorizing them based on levels of stakeholder participation—ranging from nominal to transformative—this review highlights the need for clearer, more transparent methods of conveying ABM complexity and emergent properties. It also emphasizes the importance of systematic evaluations of communication approaches to ensure that stakeholders fully understand and can apply these models. This paper contributes to the field by offering actionable recommendations to improve transparency, stakeholder engagement, and the reuse of ABMs, with the aim of fostering broader adoption and more effective application of these models across various domains.Introduction
The use of Agent-based Models (ABMs) in research has garnered significant attention across disciplines such as social sciences and ecology, particularly for studying complex adaptive systems. Recently, ABMs have also been employed to model social-ecological systems, where incorporating local stakeholder knowledge is vital to accurately represent agents and their interactions. Increasingly, simulation models like ABMs directly or indirectly engage stakeholders, making effective communication of these models a critical factor for the success and impact of such modeling projects (Haveman & Bonnema 2015; Rumeser & Emsley 2016; Waldherr & Wijermans 2013). For ABMs specifically, stakeholder comprehension is crucial, as their perceived utility and success often depend on user confidence and subjective evaluations. Although an exact definition of "communicating simulation models" has not been firmly established, the literature suggests that ABM communication refers to the process of conveying the design, structure, functionality, and outcomes of agent-based models (ABMs) to stakeholders, with the goal of enhancing their understanding, fostering engagement, and facilitating application of the models. This encompasses the use of various tools and approaches—such as visualizations, interactive simulations, and standardized documentation—to ensure clarity and accessibility, address the complexity and emergent properties of ABMs, and tailor communication to the diverse levels of stakeholder involvement and expertise.
One of the major criticisms of the ABM paradigm is its characterization as a "black box" system (Bommel et al. 2011; Taghikhah et al. 2022). The complexity inherent in modeling intricate systems, which involves numerous agents and multiple layers of interactions, poses significant challenges to communicating ABMs effectively to end-users. This issue has led to reluctance among many potential users to adopt this modeling approach (Cartwright et al. 2016; Grimm & Railsback 2012; Sun et al. 2016; Tehrani 2020). Efforts to establish standardized protocols for communicating ABMs have faced difficulties, although some progress has been made (Collins et al. 2015; Daly et al. 2022). Improved communication methods are essential to enable non-specialists and practitioners to understand and trust ABMs, thereby fostering broader adoption and application (Polhill et al. 2008).
Another challenge lies in promoting the reuse of developed models to improve research efficiency (Müller et al. 2014; Polhill & Gotts 2009). Promoting the reuse of developed models requires effective communication strategies to overcome barriers such as lengthy or incomplete documentation, complex interfaces, and abstracted processes. Despite the availability of online repositories that make ABMs accessible (Janssen et al. 2008), many models remain underutilized after their initial publication. Issues such as lack of interoperability, the significant effort required to comprehend and operate them, and incomplete model documentation contribute to this problem (Bersini et al. 2012; Thomas-Vaslin et al. 2013). Facilitating more effective communication is a potential solution to these challenges, as clear and accessible models are more likely to be reproduced and reused (Daly et al. 2022).
The relevance of model communication is closely linked to the specific purpose of the modeling effort. Edmonds et al. (2019) identified seven primary reasons for creating a simulation model: prediction, explanation, description, theoretical exposition, illustration, analogy, and social learning. Depending on these goals, models may be developed as either explicit "how" models, which emphasize replicability and empirical accuracy, or implicit "why" models, which focus on generating inferences and conceptual understanding (Epstein 2008). This study focuses on "how" models, emphasizing that effectively communicating these models to stakeholders is integral to achieving their intended purposes. By investigating the current landscape of ABM communication strategies, we aim to identify gaps and opportunities for enhancing stakeholder engagement and model utility.
The remainder of this paper is structured as follows: (1) Related Work, reviewing relevant state-of-the-art literature on communicating ABMs; (2) Methodology, detailing our approach to collecting and analyzing the relevant corpus; (3) Results, presenting our findings from the scoping review; (4) Discussion, offering insights based on the analysis; and (5) Summary and Conclusion, summarizing the study’s key takeaways and implications for future research.
Related Work
Communicating the model is a crucial component of scientific modeling, as outlined in the modeling cycle proposed by Grimm & Railsback (2005), where communication is identified as a fundamental step. A model holds limited scientific value until it has been clearly and comprehensively communicated to ensure that its design and outcomes are understood by the relevant stakeholders. This section reviews three key studies that have explored the state-of-the-art in communicating agent-based models (ABMs).
The first review, conducted by Müller et al. (2014), assessed the efficiency of ABM descriptions, highlighting challenges in evaluating and replicating these models due to a lack of transparency in model descriptions. Their study identified several categories of ABM descriptions but concluded that no single approach could satisfy all communication needs. This work provided a foundation for understanding the types of stakeholders typically engaged in communicating ABMs.
Cartwright et al. (2016) examined the communication of complex ecological models to non-scientific stakeholders, focusing on the difficulties of ensuring proper interpretation and use of simulation models. They proposed a four-stage process for communicating models, emphasizing the need for clear guidance to help stakeholders accurately interpret model outcomes. This review offered valuable insights into the essential elements required for effective ABM communication.
Daly et al. (2022) provided a more recent perspective on the standardization of ABM methodologies. Although their review primarily addressed model description and documentation rather than communication, it highlighted recent advancements in making ABMs more reproducible. Their discussion on model transparency underscored the need for systematic approaches to improve stakeholder understanding of ABMs.
These studies have laid the groundwork for understanding the challenges in communicating ABMs. However, the older reviews by Müller et al. (2014) and Cartwright et al. (2016) lack systematic analyses and are now somewhat outdated. Building on these works, our study aims to provide an updated review that systematically analyzes approaches for communicating ABMs to stakeholders, with the goal of identifying research gaps and opportunities for enhancing future communication efforts.
Methodology
This study seeks to determine the current scope of contributions in the field of communicating agent-based models (ABMs) to stakeholders. To do this, a systematic literature review was conducted, focusing on peer-reviewed articles retrieved from two major bibliographic databases: Scopus and Web of Science. The corpus collection was finalized in August 2023. The overall process is depicted in Figure 1.
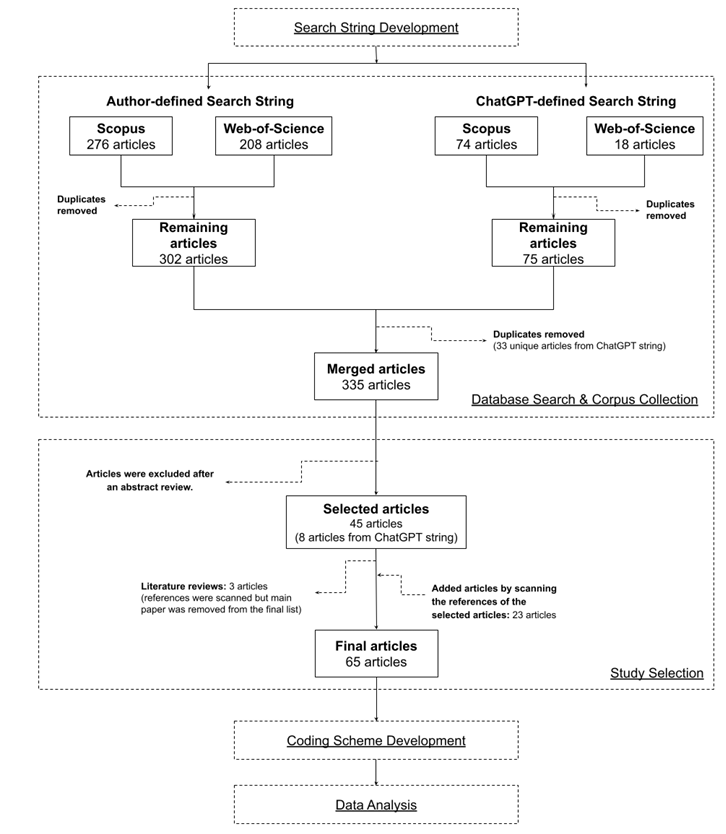
Search string development
To address the question, "How are agent-based models communicated to stakeholders?" we developed a Boolean search query that combined the terms "agent-based," "communication," and "stakeholder." The term "agent-based" was specified to be followed by either "model" or "simulation," while the term "communication" was expanded to include related variations. We also included "user" as a synonym for "stakeholder" after noticing its frequent use in the literature. The resulting search string was:
\[``agent-based\ (model\ OR\ simulation)"\ AND\ (communicat^*\ AND\ (stakeholder\ OR\ user))\] |
Additionally, we employed ChatGPT by OpenAI (2023) to generate an alternative search phrase to ensure comprehensive coverage of relevant publications. The effectiveness of ChatGPT in generating high-precision boolean queries for systematic literature reviews has been explored in Wang et al. (2023), which concluded, after extensive experiments, that ChatGPT is effective for this purpose. However, since we did not plan to rely solely on the results from the ChatGPT-generated query, we kept our interaction with ChatGPT simple. The simplicity in the ChatGPT interaction was intended to ensure the generated search string remained broadly applicable and comparable to the manually developed one. This approach aimed to balance comprehensiveness and relevance without overcomplicating the search. We provided the following prompt: "I want to gather a corpus for my review paper on ‘tools to communicate agent-based models to stakeholders.’ Can you give me a good search string for it?" The search string returned by ChatGPT is provided below.
\[ ``agent-based \ model"\ OR \ ``ABM")\ AND\ (``communication"\ OR\ ``visualization"\ OR\ ``presentation") \\ AND\ (``tools" \ OR\ ``methods" \ OR\ ``techniques") \\ AND\ (``stakeholders" \ OR\ ``decision-makers" \ OR\ ``policy-makers") \] |
Thus, we ended up with two search strings, each of which was input into the databases for collecting related literature.
Study selection
The initial search returned 335 unique articles, of which 42 were duplicates. We reviewed the abstracts to focus on articles that specifically presented or discussed efforts to communicate agent-based models (ABMs) to stakeholders. From the original 335 articles, we narrowed the collection to 45, eight of which originated from the database search using the ChatGPT-defined search string. Excluded articles primarily fell into several categories. First, some focused on the use of ABMs to simulate and analyze communication strategies and dynamics. Although these articles discussed both ABM and communication, their focus was predominantly on modeling peer-to-peer and social media communication, crisis communication strategies, word-of-mouth effects, political discourse, and socio-technical collaboration. Second, we excluded articles describing ABMs of network dynamics and communication systems. These articles typically modeled wireless communication networks (e.g., 5G and beyond), social networks, mobile ad-hoc networks, information propagation, and collaborative decision-making in Internet of Things (IoT) environments. Lastly, we excluded articles that presented an ABM or multi-agent system without addressing the communication of the model to stakeholders. While these articles often involved case studies with stakeholders and decision-making methodologies, their communication efforts were limited to the context of the case study and did not extend to engaging stakeholders with the model itself. In total, 290 articles were excluded, including 108 ABM case study articles. The high proportion of excluded articles highlights that while communication is often modeled using agent-based approaches, there is limited literature that explicitly discusses communicating these models to stakeholders. This selection process resulted in a final corpus of 65 articles.
The final 65 articles come from a diverse range of disciplines (Figure 2a), with nearly equal numbers from computer science and environmental science. This distribution likely reflects the fact that, while modeling has its roots in computer science, its applications are increasingly prevalent in environmental research. In this field, researchers have been progressively integrating traditional scientific methods with data science and computer science techniques (Ayllón et al. 2021). In terms of publication year, Figure 2b indicates a steady stream of contributions to the field beginning in 2008, with a notable increase in 2022 that coincides with the surge in COVID-19 modeling papers. Interestingly, research on communicating agent-based models (ABMs) appears to have emerged more than a decade after the ABM paradigm gained prominence in the 1990s.
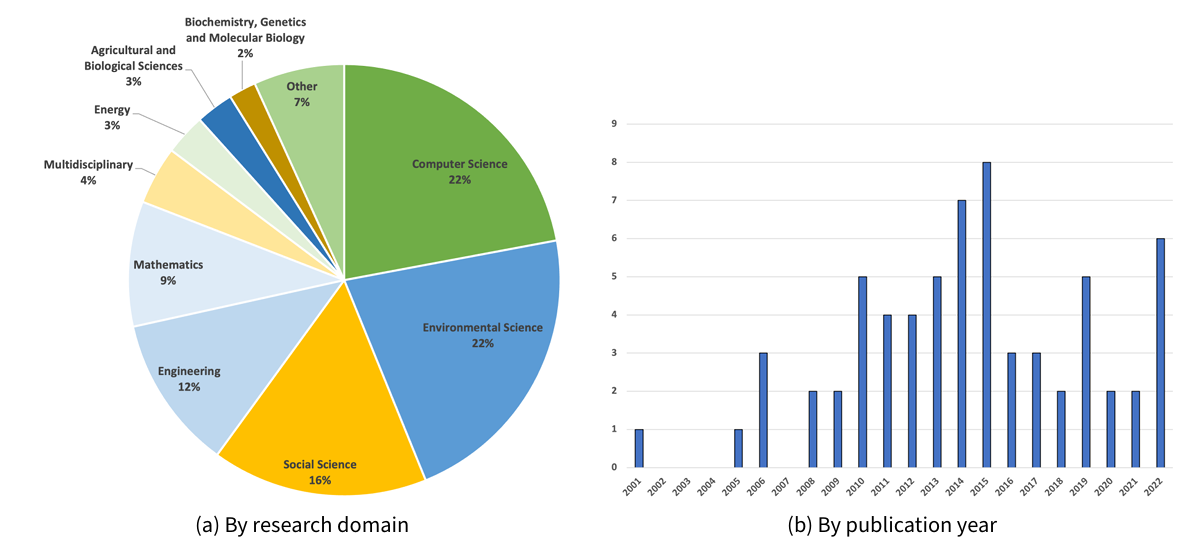
Coding scheme development
The coding scheme used to classify information from the reviewed articles was initially derived from studies discussed in the ‘Related Work’ section. These studies guided the identification of key components, such as stakeholder types typically involved in ABM communication, essential communication elements, and the purposes for engaging stakeholders. Through iterative refinement involving open coding and constant comparison, emergent themes were identified and aligned with existing literature. The coding process continued until theoretical saturation was reached, indicating that no new codes emerged from subsequent analyses.
We now specify the exact components of the final coding scheme: (1) "Participation Forms," which classify stakeholder engagement levels; (2) "Purposes and Focus of Communication Approaches," which outline the goals of ABM communication; (3) "Communication Approaches," which capture the types of ABM communication based on the tools used; (4) "ABM Aspects Conveyed to Stakeholders," which identify the elements communicated to stakeholders; and (5) "Evaluation of the Effectiveness of Communicating ABM to Stakeholders," which assesses whether communication outcomes are considered and measured. Table 1 presents an abridged version of the coding scheme, with complete details provided in Appendix A.
Category | Codes |
---|---|
Participation Forms | Nominal |
Instrumental | |
Representative | |
Transformative | |
Purposes and Focus of Communication Approaches | Specification |
Transparency | |
Validation | |
Presentation | |
Reproducibility | |
Extension | |
Communication Approaches | Document-type |
Visual | |
Interactive | |
ABM Aspects conveyed to stakeholders | Structure |
Complexity | |
Emergence | |
Evaluation of the Effectiveness of Communicating ABM to Stakeholders | With Evaluation |
Planned Evaluation | |
Indirect Evaluation | |
No Evaluation | |
In our use of the term "stakeholder," we refer to the definition provided in Voinov & Bousquet (2010), which describes stakeholders as individuals or groups that bear the consequences of the decisions considered in the simulation model. Stakeholders could represent diverse sectors, such as local and federal governments, private and public organizations, as well as individual citizens and interest groups. Their familiarity with simulation modeling could range from minimal to extensive.
To guide the review, we used the ‘Interests in Participation’ framework, adapted from White (1996) and van Bruggen et al. (2019) (see Table 2). This framework categorizes stakeholder involvement based on their level of participation and control over information flow in modeling projects. The classification includes nominal, instrumental, representative, and transformative participation. This approach enables the analysis of stakeholder motivations and roles in modeling and simulation projects. Participation forms can be examined from three perspectives:
- Top-Down Perspective: In this perspective, stakeholder participation is defined by the project’s objectives, with stakeholders primarily either receiving information derived from the model or contributing data to inform it. In nominal forms, their involvement is limited to legitimizing the project, with minimal or no input on the model’s design. Whereas, in instrumental forms, stakeholders provide essential information to improve project efficiency, though their role remains restricted to supplying data rather than actively shaping the model.
- Bottom-Up Perspective: This perspective views stakeholder participation from the stakeholders’ standpoint, with information flowing from their level to the modelers. In representative forms, stakeholders play an active role in ensuring that the model captures their perspectives and interests. They can review, agree, or challenge aspects of the model, advocating for significant changes, thereby making their input essential to the model’s development and alignment with their needs.
- Function Perspective: This view places stakeholder participation at the core of the modeling project, emphasizing a dynamic exchange of information between stakeholders and modelers. In transformative forms, participation goes beyond mere involvement to become the primary objective of the project. Stakeholders not only contribute to the model but also experience changes in their perspectives through interactions with it. Their engagement influences both the model’s development and their own understanding, creating a mutually transformative process that is vital to the project’s success.
Form | Top-down | Bottom-Up | Function |
---|---|---|---|
Nominal | Legitimation | Inclusion | Display |
Instrumental | Efficiency | Cost | Means |
Representative | Sustainability | Leverage | Voice |
Transformative | Empowerment | Empowerment | Value (means/end) |
During data analysis, we decided to reorder the sequence of participation forms by addressing "instrumental" forms before "nominal" forms. Both nominal and instrumental participation engage stakeholders in a passive manner, meaning they do not actively collaborate with modelers. The only difference lies in the degree of understanding stakeholders have of the developed model. In nominal forms, stakeholders may not contribute to the model’s design but are informed about the final model and its outcomes. In contrast, in instrumental forms, stakeholders provide input on the model’s design but may not receive direct communication during or after model development. Passive involvement, in this case, refers to the extent to which stakeholders can directly impact the model’s design based on their level of understanding. By reordering, we emphasize the progression of stakeholder understanding, from minimal comprehension in instrumental forms to transformative forms, where stakeholders not only understand the model, but also gain a deeper understanding of the target system, thereby becoming empowered to make significant changes to the model’s design. Figure 3a illustrates this order of participation.
We used the four forms of participation to identify and categorize communication instances in the articles. In the instrumental form, we identified communications aimed at gathering design input and parameters from stakeholders. The nominal form involved instances where the primary goal was to inform stakeholders about the model’s structure and its simulation results. In the representative form, we categorized communications intended to secure stakeholders’ approval of the model. Lastly, the transformative form included communications that encouraged stakeholders to take ownership of the model. We also applied the modeling cycle from Grimm & Railsback (2012) to pinpoint specific phases where stakeholder communication occurred, helping to map engagement across different stages of the modeling process. Figure 3b illustrates how each form of participation aligns with the cycle.
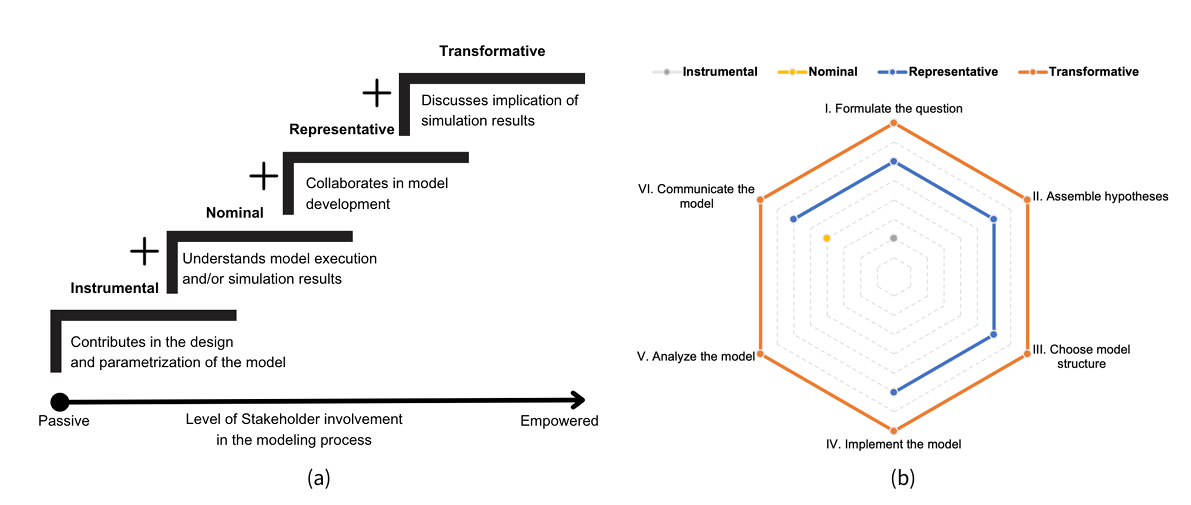
Additionally, we recorded information on the purposes and focus of the communication approaches, along with descriptions of how these approaches were implemented. During the review process, additional categories emerged, including the specific elements of ABMs conveyed through these approaches and whether evaluations of their effectiveness were present or absent.
To identify the specific elements of ABMs conveyed during communication, we referred to the works of Macal & North (2010) and Cenani (2021). According to Macal & North (2010), a typical ABM comprises three core components: agents, the relationships between agents, and the environment in which these agents operate. Collectively, these components define the model’s overall structure. Additionally, Cenani (2021) identified two critical aspects for understanding ABM behavior: complexity and emergence. Complexity pertains to the interactions within the model, while emergence refers to the non-linear and often unpredictable phenomena that arise during simulations. We examined whether communication approaches supporting nominal, representative, and transformative participation effectively conveyed these essential elements – model structure, complexity, and emergent properties – to stakeholders.
For evaluating the presence or absence of communication assessments, we adopted criteria from Cartwright et al. (2016), which outlined criteria for assessing the effectiveness of communicating complex models and their outputs. These criteria were: (1) "Does the stakeholder subsequently have an improved knowledge of the model and its relevance?"; (2) "Does the model influence subsequent management decisions?"; and (3) "Does it improve the behavior of the modeled system?" We reviewed the selected articles for any assessment attempts that met these criteria. Articles involving only instrumental participation were excluded from this evaluation, as they did not align with any of the proposed criteria.
Data analysis
To conduct the data analysis, we followed the methodology outlined in Figure 4. The subsequent phases involved coding and synthesizing the findings to identify patterns and draw conclusions about the current landscape of communicating agent-based models (ABMs) to stakeholders. Our goal was to construct a comprehensive overview of ABM communication practices and highlight potential areas for improvement.
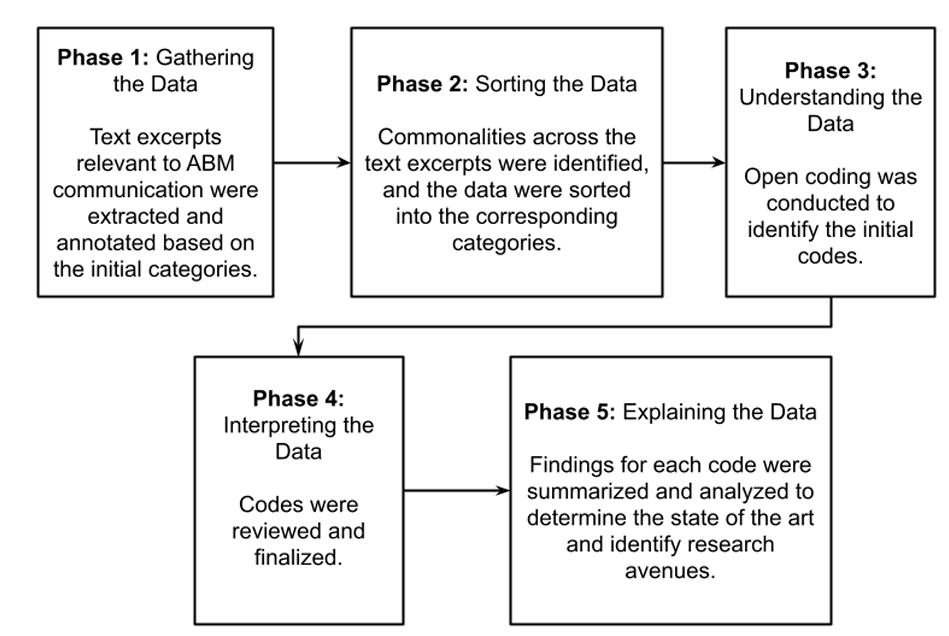
In Phase 1, articles were screened, and text excerpts relevant to ABM communication were extracted and annotated using the initial categories: ‘types of stakeholders typically engaged when communicating ABMs,’ ‘essential elements for effective ABM communication,’ and ‘purposes for communicating ABMs to stakeholders.’ During the article review, additional categories emerged, additional categories emerged, including ‘constraints in stakeholder participation,’ ‘description of communication approaches,’ ‘tools used for stakeholder communication,’ and ‘aspects of the agent-based model (ABM) communicated.’
In Phase 2, we identified commonalities within the annotated information and grouped it based on emerging patterns. Subsequently, in Phase 3, we applied open coding to uncover specific codes for further classification. This phase was guided by key questions informed by insights from previous phases: Are there commonalities across communication approaches? Which aspects of the ABM were communicated using particular tools? What aspects of the modeling process were addressed through these tools? and Was the effectiveness of communicating the ABM to stakeholders assessed? If so, how?
Phase 4 involved reviewing the codes for consistency (ensuring each code corresponds to a single definition) and accuracy (verifying that the codes align with the definitions in the articles from which they were extracted). After this review, the codes were finalized. Appendix A presents the descriptions of the final codes identified during data analysis.
In the final phase, Phase 5, we synthesized the findings within each category to construct an overview of the current state of the art in communicating ABMs to stakeholders. We also highlighted the primary focus areas of the existing literature and identified potential avenues for future research.
Results
This section presents the findings from our analysis, organized into six main categories: (1) Forms of stakeholder participation, (2) Constraints in stakeholder participation, (3) Approaches to communicating ABMs to stakeholders, (4) Aspects of ABMs conveyed to stakeholders, (5) Purposes and focus of the communication approaches, and (6) Evaluation of the effectiveness of communicating ABMs to stakeholders.
Forms of stakeholder participation
Figure 5 illustrates the distribution of articles according to the forms of stakeholder participation. Our analysis revealed that multiple forms of participation often coexist within a single ABM project. We identified 48 articles featuring one form of participation, 9 articles with two forms, and 8 articles with three forms, resulting in a total of 90 instances of stakeholder communication in ABM projects.
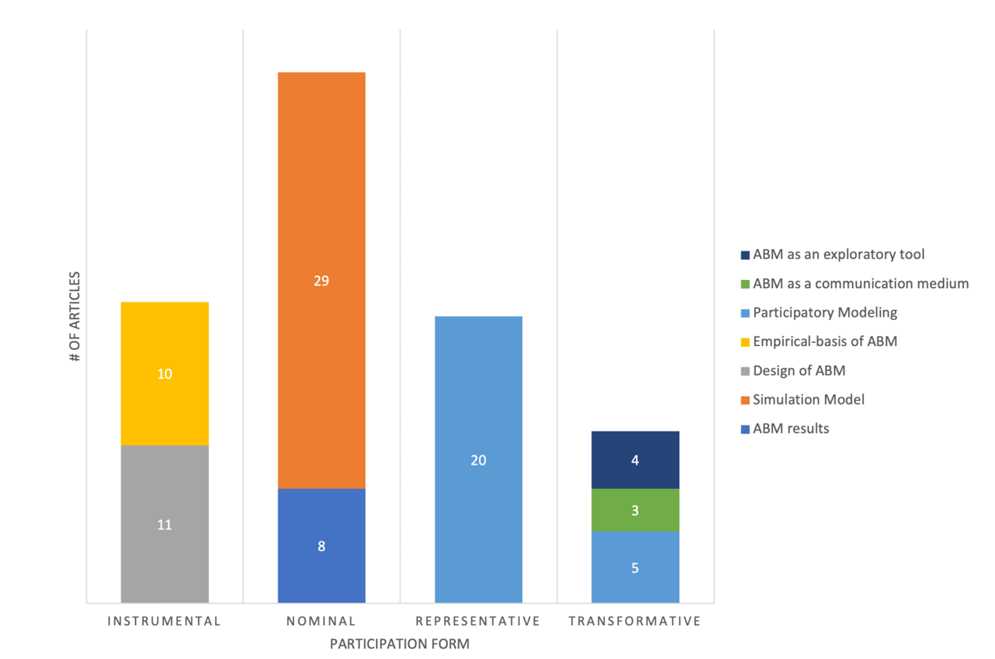
Over 40% of the reviewed articles highlighted efforts to communicate the model’s structure and results to stakeholders, a practice commonly associated with nominal participation. In comparison, approximately 35% of the communication initiatives are focused on enhancing stakeholder representation and enabling transformative applications, while the remainder fall under instrumental participation. Although the limited number of reviewed articles makes it difficult to draw definitive conclusions about the overall state of stakeholder engagement in agent-based modeling (ABM) projects, the findings reveal an increasing emphasis. While much ABM communication still centers on legitimizing models and sharing results, there is a noticeable shift toward actively involving stakeholders in the modeling process.
Constraints in stakeholder participation
The following constraints or challenges were identified in each form of stakeholder participation during ABM projects:
- Instrumental
- Securing long-term stakeholder commitment proved challenging, often leading to generalized model representations due to reliance on limited stakeholder input (Dumrongrojwatthana et al. 2011; Forrester et al. 2014).
- Ethical considerations and the need for prolonged engagement delayed project timelines (Bharwani et al. 2005; Roxburgh et al. 2021).
- Effective communication required a common ontology to bridge knowledge gaps across different disciplines (Cisse et al. 2015).
- Nominal
- Models often had a steep learning curve, leading to low adoption rates (Bersini et al. 2012; Radinsky et al. 2017).
- Stakeholder absenteeism or disinterest during presentations reduced engagement levels (Appel et al. 2010; Comai et al. 2022; Gourmelon et al. 2013).
- The absence of a shared language posed a barrier to understanding complex models (Onggo 2013).
- Representative
- Resource-intensive meetings and skilled facilitation were essential for meaningful engagement (Dumrongrojwatthana et al. 2011).
- Conflicting stakeholder interests created barriers to collaboration (Gurung et al. 2022; Naivinit et al. 2010).
- Collaboration tools often required training and a unified communication strategy (Bommel et al. 2011; Sibertin-Blanc et al. 2019).
- Transformative
- Time and budget constraints limited opportunities for in-depth stakeholder interaction (Simon & Etienne 2010).
- Diverse stakeholder groups required tailored communication methods to ensure effective participation (Naivinit et al. 2010; Shelton et al. 2018).
These constraints highlight the need for improved communication tools that can facilitate stakeholder engagement while minimizing learning barriers.
Approaches to communicate ABMs to stakeholders
Our analysis identified two primary categories of communication approaches: those supporting instrumental participation and those facilitating nominal, representative, and transformative participation. The first category is typically used during fieldwork, through individual (one-on-one) or collective (group-based) stakeholder engagement. Approaches include surveys, interviews, participatory mapping, role-playing games, and focus group discussions. The second category is further divided into three subcategories based on the type of primary tool developed for each: document-based, visual, and interactive. Figure 6 presents the different tools used in the second category, organized according to the type of approach.
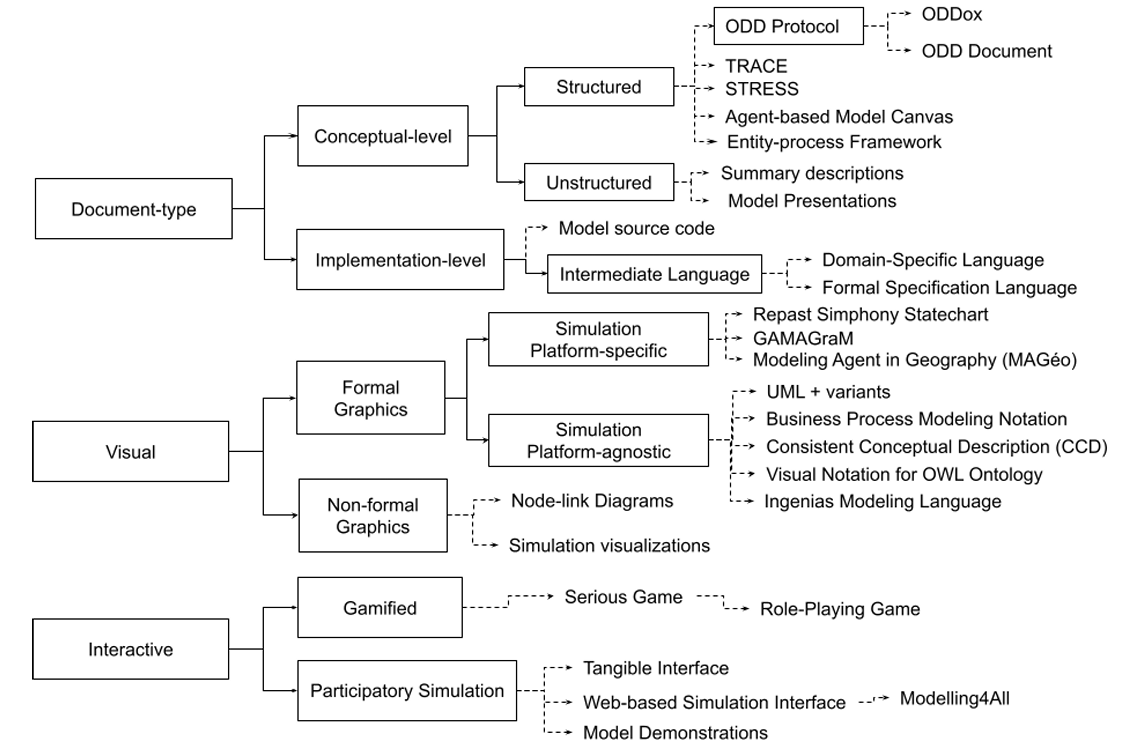
Document-based approaches included structured tools like the ODD (Overview, Design concepts, and Details) protocol (Grimm et al. 2006), TRACE (Ayllón et al. 2021), and STRESS (Monks et al. 2019), as well as unstructured descriptions of the model’s implementation. These tools were mainly used at the end of model development to communicate the finalized model.
Visual approaches comprised graphical representations like UML diagrams (e.g., Bommel et al. 2011) and platform-specific visualizations (e.g., Taillandier 2014), which engaged stakeholders during the model design phase. They helped clarify the model’s structure and complexity but were less effective in illustrating emergent behaviors.
Interactive approaches involved gamified tools, participatory simulations, and tangible interfaces (e.g., Grignard et al. 2018) that enabled stakeholders to engage with the model dynamically. These methods were used across all stages of development, enhancing both stakeholder understanding and model validation.
Aspects of ABMs conveyed to stakeholders
We assessed the effectiveness of the communication approaches in conveying three critical aspects of ABMs: structure, complexity, and emergence. Most tools supported communication of the model’s structure and complexity, allowing stakeholders to grasp how the model functions (Naivinit et al. 2010). However, only a few approaches adequately addressed the concept of emergence, a key feature distinguishing ABMs from other modeling paradigms (Adam et al. 2018; Bonabeau 2002; Hall & Virrantaus 2016). Table 3 provides a summary of the ABM aspects conveyed to stakeholders through the tools used in communication.
Tool type | Tool | Structure | Complexity | Emergence |
---|---|---|---|---|
Document-type | ABMC | x | x | x |
TRACE Standard | x | x | x | |
ODD Protocol | x | x | x | |
ODdox | x | x | x | |
STRESS-ABS Guideline | x | x | ||
Entity-Process Framework | x | x | ||
Model Source Code | x | x | ||
Summary Descriptions | x | x | ||
Model Presentations | x | x | ||
Domain-specific Language | x | x | ||
Formal-specification Language | x | x | ||
Visual | BPMN | x | x | |
CCD | x | x | ||
Ingenias Modeling Language | x | x | ||
UML | x | x | ||
Visual Notation for OWL Ontology | x | x | ||
GAMAGraM | x | x | ||
MAGéo | x | x | ||
Repast Simphony Statechart | x | x | ||
Node-link Diagrams | x | x | x | |
Simulation Visualization | x | x | x | |
Interactive | Role-Playing Game | x | x | x |
Model Demonstrations | x | x | x | |
Tangible Interface | x | x | ||
Web-based Simulation Interface | x | x | x | |
When it comes to communicating emergence, document-type approaches—such as protocols and guidelines proposed for ABM documentation—dedicate specific sections to describe the emergence observed or expected from the simulation. For example, the TRACE standard addresses emergence in the "Model analysis and application" section, the ABMC includes it in the "Target output" section, and the ODD protocol covers it in the "Design concepts" section. Furthermore, Grimm & Railsback (2012) recommended integrating pattern-oriented modeling (POM) with ODD to improve the design and communication of emergent behaviors in ABMs. However, the STRESS-ABS guideline does not explicitly indicate where emergence should be discussed in model reports.
In terms of visual approaches, non-formal graphics, such as node-link diagrams (e.g., Taghikhah et al. 2022) and simulation visualizations (Barry et al. 2008), have been used to communicate emergence in ABMs. In contrast, formal graphics like UML do not yet support the visualization of emergent properties (Forrester et al. 2014). While node-link diagrams have been used to illustrate emergent properties, they were less effective than interactive simulations.
Interactive approaches have also been utilized to observe emergent behaviors, especially in ABMs designed to study emergence. For instance, in Yang et al. (2022), the ABM was presented to stakeholders through demonstrations that successfully sparked group discussions on how to trigger system emergence. In another study, Gurung et al. (2022) engaged stakeholders in a role-playing game designed to promote the emergence of new perspectives on the modeled system. Similarly, Carmenate et al. (2016) employed a web-based simulation interface that allowed stakeholders to interact with the model and observe system emergence, thereby enabling discussions on the influencing factors and potential strategies that could trigger the emergent behaviors. Of the three communication approaches, we found that interactive approaches are a common choice for demonstrating emergent behaviors, as they allow stakeholders to observe and discuss these patterns through role-playing games or web-based interfaces.
Purpose and focus of the communication approaches
Thematic analysis of the corpus revealed six key objectives for model communication, based on the use of the identified communication approaches. In the following, we present the list of these objectives and provide a description of each, as described in the articles:
- Model Specification. Model specification reflects the researcher’s understanding of the details of the target system (Dumrongrojwatthana et al. 2011) and should correspond to the agreed descriptions that are well understood by the stakeholders (Forrester et al. 2014; Sibertin-Blanc et al. 2019). Based on the literature, model specification is particularly important when developers lack the domain expertise that informs the model, or when the model design needs to be updated due to an evolving understanding of the system (e.g. Moeiniyan Bagheri et al. 2015). Tools developed for communicating model specifications include visual languages (Dumrongrojwatthana et al. 2011; Onggo & Karpat 2011; Sansores et al. 2006), metamodels (Hassan et al. 2009; OMG 2006; Sibertin-Blanc et al. 2019), and formal notation (Moeiniyan Bagheri et al. 2015). Moreover, there has been a growing interest toward co-designing ABMs with stakeholders (Bommel et al. 2011; Hall & Virrantaus 2016; Naivinit et al. 2010). Several visual tools have been specifically developed to support this co-design process, including the Agent-Based Model Canvas (ABMC) (Garibay et al. 2019), Consistent Conceptual Description (CCD) (Scherer et al. 2013), extensible UML activity diagrams (Bommel et al. 2011), semi-formal node-link diagrams (Hall & Virrantaus 2016; Smetschka & Gaube 2020), and statecharts (Ozik et al. 2015). Additionally, platform-specific tools like MAGéo (Langlois et al. 2015) and GAMAGraM (Taillandier 2014) offer graphical interfaces that visually abstract model code, helping stakeholders to view the model on the level of its implementation and other modelers to better interpret the model code.
- Model Validation. Agent-based models (ABMs) are created to replicate target systems using empirical data or stakeholder insights (Forrester et al. 2014; Hassan et al. 2009). To ensure accuracy, model validation is conducted by comparing simulation results to observable reality. According to (Yang et al. 2022), model validation can be conducted in three ways: output validation, structural validation, or a combination of both (i.e. Yu et al. 2016). A key component of this process is stakeholder involvement, known as "social validation" (Bommel et al. 2011), "stakeholder validation" (Roxburgh et al. 2021), or "truth grounding" (Forrester et al. 2014). Structural validation often involves stakeholder interviews, model presentations, and semi-structured questionnaires (Barbrook-Johnson et al. 2017). Role-playing games (RPGs) can demonstrate the ABM to stakeholders, with validation occurring in subsequent discussions (Shelton et al. 2018), while interactive visualizations can be used to gather live user feedback (Simon & Etienne 2010; Yu et al. 2016). Additionally, tools like UML, BPMN, CCD, metamodels, or web-based virtual labs are used to conduct collaborative evaluation (Bommel et al. 2011; Forrester et al. 2014; Hassan et al. 2009; Onggo & Karpat 2011; Pooyandeh & Marceau 2013). For output validation, machine learning (ML) techniques were found to be used to validate outputs of empirical models (Taghikhah et al. 2022). However, for models based on hypothetical scenarios it is difficult to do direct output validation (Pooyandeh & Marceau 2013). In this case, several researchers have suggested validating and calibrating the model with stakeholders (Garibay et al. 2019; Joffre et al. 2015; Lotzmann et al. 2015; Smetschka & Gaube 2020). An example can be found in the study by (Joffre et al. 2015), where a role-playing game (RPG) was developed to enable stakeholders to adjust the model’s rules and parameters, thereby confirming in the process that the model accurately reflects the target system.
- Model Presentation. Agent-based models (ABMs) are often presented to disseminate the model, raise awareness of the system being modeled, and promote the application of ABMs in new domains (Adam et al. 2018; Dumrongrojwatthana et al. 2011; Grignard et al. 2018; Ruankaew et al. 2010). Stakeholders play a central role in these presentations (Shindler et al. 2017), and interactive tools are widely used to present models to both stakeholders and non-experts (Adam & Andonoff 2019; Grignard et al. 2018; Gurung et al. 2022). ABMs are frequently presented in the form of games, either as game-based versions of the target system or as gamified simulations (Adam & Andonoff 2019; Ruankaew et al. 2010). When direct stakeholder participation is not feasible, standardized descriptions like the ODD protocol (Grimm et al. 2006) or STRESS are often employed (Monks et al. 2019). Additionally, visual tools such as UML and node-link diagrams are used to complement these textual descriptions (Hall & Virrantaus 2016). Notably, in complex and urgent scenarios, such as COVID-19 modeling, the focus shifts to presenting model results through clear visualizations and web-based interfaces (Barry et al. 2008; Comai et al. 2022; Lichter et al. 2015), often without showing the underlying simulation model itself.
- Model Transparency. Model transparency is crucial for fully understanding how a simulation model functions and how it aligns with the target system (Polhill 2015; Polhill & Gotts 2009). This is particularly important when communicating agent-based models to stakeholders, as these models are often perceived as "black-box" systems (Bommel et al. 2011; Taghikhah et al. 2022). To address this issue, documentation frameworks such as the Entity-Process Framework (Sibertin-Blanc et al. 2019) and the TRACE standard (Ayllón et al. 2021) have been developed to improve the clarity and completeness of model documentation. Additionally, reverse engineering techniques have been employed to extract the ontology of agent-based models (Polhill & Gotts 2009) and to automatically obtain conceptual design of simulation models (Taghikhah et al. 2022).
- Model Reproducibility. Model reproducibility refers to the ability to recreate a model (Sansores et al. 2006) and reproduce its simulation results on different platforms or by other researchers (Grimm & Railsback 2012; Hassan et al. 2009; Monks et al. 2019). Achieving this requires open access to the model’s source code along with clear documentation that adheres to specific protocols (Monks et al. 2019; Roxburgh et al. 2021). To support the replicability of agent-based models, the Open ABM Consortium developed an online archive for individual-based and agent-based models (Janssen et al. 2008), featuring a ranking system that assesses models based on documentation completeness and reproducibility support. The consortium also proposed a specialized electronic journal to recognize and incentivize modelers who successfully test and reproduce models from the archive.
- Model Extension. Model extension refers to the ability to update or repurpose agent-based models over time. Significant effort is needed to facilitate the extension of existing models, with effective communication to future users being a crucial component. Providing comprehensive model documentation and source code, including detailed links to specific aspects of model design, is essential for helping researchers understand and build upon the model (i.e. Topping et al. 2013). Like in model reproducibility, the only existing effort to ensure models can be extended or built upon is the ABM archive initiated by the Open ABM Consortium (Janssen et al. 2008). This repository, managed by the Network for Computational Modeling in the Social and Ecological Sciences (CoMSES Net) community, currently houses over 7,500 agent-based and individual-based models, providing a solid foundation for those looking to extend and explore these models.
Figure 7 presents the distribution of communication approaches based on the modeling objectives they help achieve. To summarize our findings, we identified the following trends:
- Model Specification: Visual tools were most frequently used to engage stakeholders in defining and refining the model’s components, particularly when co-designing ABMs.
- Model Validation: Interactive methods were preferred due to their ability to involve stakeholders directly in testing and refining the model’s accuracy.
- Model Presentation: A balanced use of document-based, visual, and interactive approaches was observed, indicating a need for multiple strategies to reach a diverse audience.
- Model Transparency and Reproducibility: Document-based tools were predominant, as they provided detailed technical descriptions essential for scientific rigor.
- Model Extension: Open-source repositories and comprehensive documentation were key to facilitating the future adaptation and expansion of ABMs.
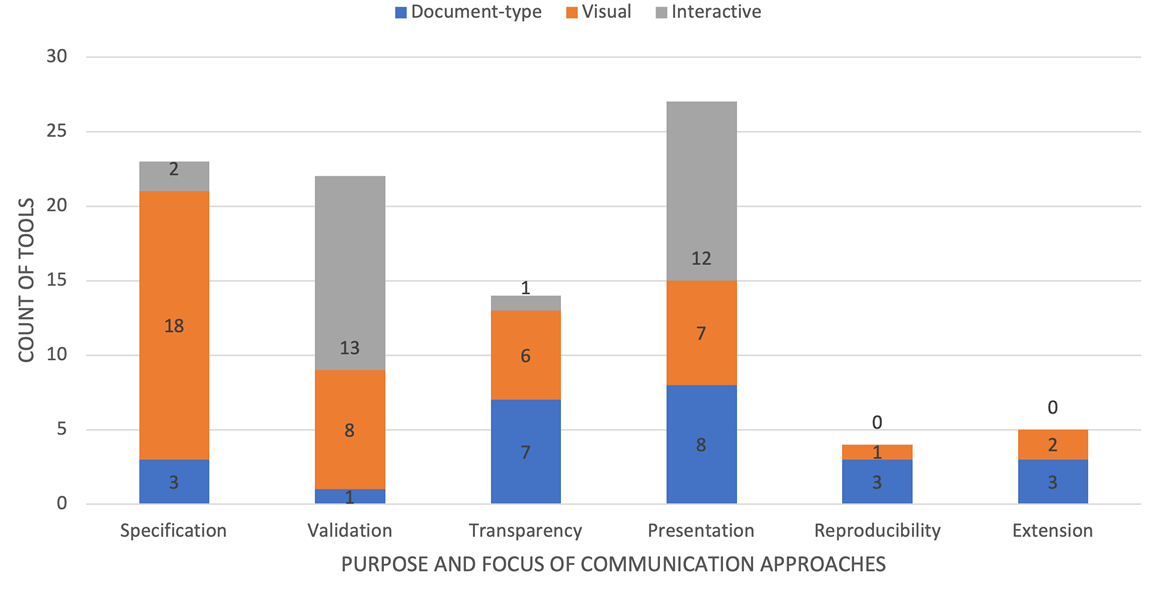
Evaluation of the effectiveness of communicating ABM to stakeholders
Among the 57 articles that involved stakeholder participation beyond the instrumental level, only three conducted a direct evaluation of the communication tools’ effectiveness. The assessments indicated that approaches like CCD tools (Scherer et al. 2013), role-playing games (Joffre et al. 2015), and node-link diagrams (Hall & Virrantaus 2016) effectively enhanced stakeholder understanding.
Six additional articles outlined plans for future evaluations (Ayllón et al. 2021; Grimm & Railsback 2012), while 16 articles used indirect methods such as post-game debriefings (e.g., Appel et al. 2010; Adam et al. 2018; Gurung et al. 2022) or open discussions to gauge stakeholder engagement (e.g., Ruankaew et al. 2010; Gourmelon et al. 2013). However, the majority of the articles (56%) did not include any form of evaluation, highlighting a significant gap in the assessment of communication strategies. Figure 8 illustrates the distribution of articles according to the presence or absence of communication effectiveness evaluation.
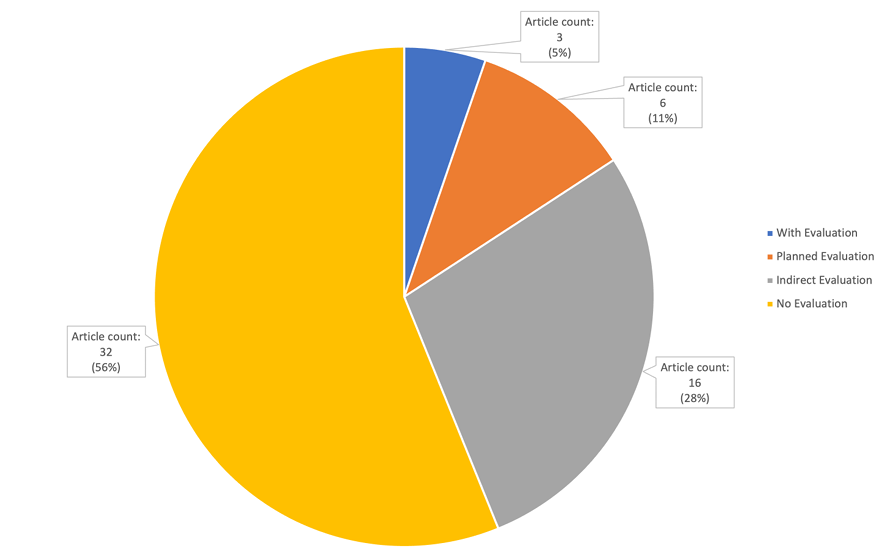
Discussion
Our analysis revealed three key gaps in the current approaches to communicating agent-based models (ABMs) to stakeholders. These gaps highlight areas where further development and research are necessary to enhance the effectiveness and impact of ABM communication.
Gap 1: Focus on Communication During Development with Limited Attention to Post-Development Communication
The corpus review revealed two perspectives on the focus and purpose of model communication. On the one hand, agent-based modeling is seen as an activity where model communication plays a central role, making it the primary product of the modeling project. In this perspective, the resulting ABM is often seen as disposable due to its highly specific design and limited application. This perspective is prevalent in participatory and co-modeling projects. An example is the ABM developed by Gurung et al. (2022) to mitigate conflicts over the use of a renewable natural resource. Since the model design was specific to the stakeholders involved, the resulting model was treated as disposable and communicating with stakeholders around the development and use of the model was the core of the project. On the other hand, agent-based modelling is perceived to be a scientific tool, where models are the ‘materials and methods’ used by modelers to achieve scientific results (Monks et al. 2019). According to Grimm et al. (2006), model reproducibility is a conditio sine qua non for establishing a simulation model as a rigorous scientific instrument. While post-presentation communication may not be essential for disposable models, ABMs must be transparent enough to enable model and result reproduction for scientific legitimacy (Grimm & Railsback 2012). As such, it is essential to communicate these models with future users and stakeholders in mind and ensure that it is transparent enough for other researchers/users to get the same results using the same "materials and methods".
The majority of communication tools identified in the review focus primarily on facilitating stakeholder interaction during the model’s development phase. In Figure 7, we see 72 communication support tools designed for model specification, validation, and presentation, versus only 23 that aim to improve model transparency, reproducibility, and extension. There is a noticeable lack of tools designed to support communication after the model has been developed and validated. This tendency reflects a perspective that prioritizes immediate stakeholder engagement over long-term usability and adaptability of ABMs.
Recommendation
To address this gap, we recommend developing communication tools that extend beyond the model development phase. Based on the literature review, UML diagrams and the ODD protocol have commonly been used to present the details of developed simulation models. However, these tools often prioritize immediate design clarity over long-term usability and are typically employed only for model presentation, rather than for purposes such as engaging stakeholders, replication, or extension. To fill these gaps, we propose the development of new tools that integrate interactive visualizations and gamified simulations, which can promote ongoing engagement with stakeholders even after the model has been developed. These tools should focus on summarizing complex model details into more accessible formats, combining visual diagrams with interactive elements to reduce the learning curve for stakeholders. Additionally, public repositories should be used to store these simplified resources, thereby enhancing transparency and encouraging broader reuse of ABMs. Gamified ABMs could also play a key role in reaching a wider audience by making complex models more engaging and easier to understand.
Gap 2: Insufficient Communication of Emergent Behaviors in ABMs
Communicating the emergent aspects of an agent-based model is a challenging task, as it requires a thorough investigation of each part of the model’s structure that could potentially lead to the emergence of these behaviors. Before these behaviors can be communicated to stakeholders, the first step is to verify whether they are genuine attributes of the system or merely artifacts of a coding error. Unlike the model’s complexity and structure which can be verified from the model’s conceptual documentation, emergent behaviors are results of interactions that are not deliberately included by design. As such, there is a high risk that observed emergent behaviors can be just a result of an implementation error (López-Paredes et al. 2005). This makes communicating the emergent aspect of an ABM even more important, as communicating this suggests that the model has been thoroughly observed and verified that it can claim presence/absence of emergent behaviors.
Emergent behavior is a key characteristic of ABMs, though not all models exhibit emergent properties. For those where emergence is relevant, effectively communicating this aspect remains a significant challenge. Our review revealed that many studies failed to address this in their communication strategies; notably, 45 out of 65 articles (69%) in the corpus did not mention emergent behavior at all. A possible explanation is that negative feedback loops, which are common in environmental models (the majority of the reviewed articles), tend to suppress significant emergent behavior, leading researchers to deem it less important to report. However, even a brief acknowledgment of whether emergence is a characteristic of the modeled system could greatly enhance stakeholders’ understanding and trust in the model’s outcomes.
Recommendation
We recommend integrating emergence-focused descriptions directly into model documentation. This should include detailed explanations of how model simulations were conducted and verified for accuracy to distinguish genuine emergent behavior from potential coding artifacts. Additionally, interactive demonstrations and simulations that allow stakeholders to observe and manipulate the system in real-time can be highly effective in illustrating these behaviors, fostering deeper insights into the dynamics of the modeled system.
Gap 3: Lack of Systematic Evaluation of Communication Effectiveness
A significant proportion (56%) of the reviewed articles did not include an evaluation of how effective their communication efforts were in improving stakeholder understanding (see Figure 8). This observation aligns with Cartwright et al. (2016), who noted that "evaluating communication effectiveness has received little formal attention, yet the degree to which communication is effective ultimately governs the relevance and societal impact of the model". Without systematic evaluation, it is difficult to determine which communication approaches are most successful in enhancing stakeholder engagement and model adoption. Moreover, evaluations can also reveal whether there is strong likelihood that the model and its results will be adopted and used by stakeholders.
Recommendation
To fill this gap, we advocate for the development of standardized guidelines to evaluate the effectiveness of ABM communication strategies. This includes integrating evaluation frameworks into the project design from the outset to measure stakeholder understanding and engagement throughout the modeling process. Where possible, evaluations should involve both primary stakeholders and broader audiences to ensure comprehensive feedback (e.g., Hall & Virrantaus 2016). As there are currently no established guidelines to evaluate ABM communication with stakeholders (Cartwright et al. 2016), tracking model usage and adoption post-development can also provide valuable insights into the long-term impact of communication efforts. Additionally, integrating post-evaluations of model usage and stakeholder understanding into projects from the outset can also facilitate model monitoring and improvement.
Understanding the Drivers of the Research Gap
Several factors contribute to these gaps, including time constraints, quality of stakeholder interaction, and power dynamics within the modeling process. Time plays a crucial role in enabling stakeholders to gain a thorough understanding of the model, which ultimately enhances their confidence in its results. However, limited time often restricts post-development communication efforts, which directly impacts the quality of interactions with stakeholders, particularly when it comes to explaining and validating the model’s emergent behaviors. Ideally, engaging stakeholders throughout the development process would build their familiarity with the model’s inner workings and support the verification of its emergent properties (similar to the Companion Modeling approach in Étienne 2014). However, such consistent engagement is not always feasible, especially when the goal is to promote the model’s reuse for replication or further development. In these situations, transparency is crucial, enabling future users to independently interpret the model’s structure and outcomes.
Power dynamics, as expounded in Arnstein (1969), also significantly influence how modeling decisions are made, the transparency of those decisions, and the evaluation process. For instance, publications on agent-based models rarely discuss the rationale behind the selection of issues to be modeled, whether those choices arise from stakeholder demand or predefined options. This lack of transparency restricts stakeholder involvement right from the beginning. Moreover, power imbalances affect both the decision to conduct communication evaluations and the selection of participants involved in these assessments. To address these gaps, a more transparent approach is needed throughout the entire modeling process, ensuring that both current and future stakeholders have the clarity required for effective model utilization and adoption.
It can be argued that the factors contributing to this gap are more anthropogenic (or, originating from human factors) than intrinsic (or, stemming from the modeling approach). Although our discussion has primarily focused on agent-based models (ABMs), similar challenges are evident in other modeling domains, particularly in system dynamics modeling. For instance, the application of system dynamics (SD) models in project management has often been found unsuccessful. Größler (2007), drawing from two case studies of unsuccessful SD projects, concluded that even a well-designed SD model may yield minimal or no impact without adequate engagement from key project stakeholders. In the study by (Rumeser & Emsley 2016), it was determined that the root causes of the lack of participation of stakeholders were identified as lack of understanding and trust in the model. Among the contributing factors are (1) a short-term cost-saving mentality, which leads to using the model only once, and (2) a lack of confidence in the system dynamics model. Interestingly, the study also revealed that the lack of confidence in SD models is often due to questions on model transparency, doubts arising from stakeholders’ prior beliefs, particularly when the model yields counterintuitive solutions, and political conflicts arising from conflicting interests.
Summary and Conclusion
Significant advancements have been made in the field of agent-based modeling (ABM), particularly in the development of approaches and tools to enhance communication with stakeholders. These efforts have improved stakeholder understanding and promoted the adoption of ABMs in various fields. However, despite these achievements, substantial gaps persist that hinder the effective communication and broader utilization of ABMs.
One key finding of this review is that most communication efforts are concentrated on engaging stakeholders during the development phase of ABMs, with considerably less emphasis on post-development dissemination and long-term usability. This limited focus on immediate stakeholders often constrains the broader adoption of ABMs and reduces their potential for reuse or adaptation in future research. Additionally, while emergent behavior is a defining feature of ABMs, it is rarely communicated effectively. This omission diminishes stakeholders’ ability to fully understand the model’s outcomes and their implications.
Another critical gap identified is the lack of systematic evaluation of communication strategies in terms of their effectiveness in improving stakeholder understanding and engagement. Without robust assessment protocols, it remains challenging to identify which methods foster trust and encourage the widespread use of ABMs among diverse audiences.
Furthermore, the complexity of ABMs presents significant challenges for stakeholders, who often have limited time to develop a comprehensive understanding of the model’s functionality. The specialized language and technical nature of ABMs can create barriers for stakeholders, making it difficult to bridge the gap between the model’s complexity and the stakeholders’ level of expertise. To address this, it is essential to provide a diverse range of communication resources and tools – a "toolbox" – that present the model from multiple perspectives. Such tools would equip stakeholders with the necessary information to better understand, trust, and effectively utilize the model’s outcomes.
Key recommendations
Based on these findings, we propose the following recommendations to address the identified research gaps:
- Expand Post-Development Communication Efforts: Develop and deploy communication tools that extend beyond the initial modeling phase to include more accessible visualizations, interactive demonstrations, and simplified summaries. These tools should be designed to engage a wide range of stakeholders, including those who may encounter the model after its initial release.
- Highlight Emergent Behaviors in ABMs: Incorporate detailed descriptions of emergent properties in model documentation and use interactive simulations to illustrate these behaviors in real-time. This will help stakeholders better understand the dynamic interactions within the model and trust its predictive capabilities.
- Implement Standardized Evaluation Protocols: Develop and standardize guidelines for evaluating the effectiveness of communication strategies in ABM projects. Integrating these evaluation frameworks into the modeling process will ensure that stakeholder feedback is systematically collected and used to improve future communication efforts.
Conclusion
This scoping review has provided a comprehensive analysis of the current state of ABM communication strategies, highlighting the areas where improvements are needed to enhance stakeholder engagement and model adoption. We identified three main research gaps: a focus on development-phase communication with limited post-development tools, insufficient emphasis on emergence in ABMs, and a lack of systematic evaluation methods. Addressing these gaps is essential to making ABMs more accessible, transparent, and impactful in both scientific research and practical applications. By implementing the recommended strategies, researchers can improve the communication of ABMs, leading to greater stakeholder confidence, broader model adoption, and ultimately, more effective decision-making based on these powerful simulation tools. The advancements in ABM communication outlined in this review have the potential to significantly enhance the utility and acceptance of these models across various domains. Future research should continue to focus on developing more intuitive communication tools and robust evaluation methods, ensuring that ABMs can be widely understood and effectively applied to address complex, real-world problems.
Acknowledgements
We gratefully acknowledge the constructive insights provided by the anonymous reviewers, which significantly improved the quality of this manuscript. We also thank the Department of Science and Technology – Science Education Institute (DOST-SEI), Philippines, and the French Embassy to the Philippines and Micronesia for their financial support through the PhilFrance-DOST Fellowship of Z. Arnejo.Appendix A: Coding Schemes
The coding framework used to analyze the data is detailed in the following tables, each focusing on specific aspects of the study. Table 4 presents the coding scheme for classifying the various forms of stakeholder participation observed in the reviewed articles, highlighting the levels and types of engagement. Table 5 explains the purposes for which models were communicated, offering definitions that contextualize each purpose within the study. Table 6 catalogs the tools used to communicate models with stakeholders, showcasing methods and approaches designed to enhance understanding and collaboration. Finally, Table 7 outlines additional categories related to ABM aspects conveyed to stakeholders, as well as the evaluation of communication effectiveness. Together, these tables provide a comprehensive overview of the systematic methodology used to categorize and interpret the data.
Classification | Definition | Theoretical basis | Example snippet |
---|---|---|---|
Nominal | Model communication is limited only to dissemination of research outcomes. Key point: “Communicate the ABM results”, " Present the simulation model" | Definition of “Form of Interest in Participation” adapted from van Bruggen et al. (2019) | “Finally, we discuss visualization techniques for these types of systems that enhance the ability of subject matter experts and decision-makers to understand the results.” (Barry et al. 2008) |
Instrumental | Model communication occurs only during consultations. Key point: “Gather empirical basis for ABM”, “Communicate to design the ABM” | “We gathered data from in-depth interviews with homeowners and installers and built individual journey maps. Then we identified interactions between the two, and merged their two maps to identify points on the journey that create difficulty or frustration. These maps provide comprehensive insight analysis for later surveys and agent-based model creation.” (Sinitskaya et al. 2020) | |
Representative | Model communication takes place during stakeholder dialogues and collaborative model development. In these interactions, stakeholders provide input and insights but do not have direct control over how the model is ultimately used. Key point: “Co-construction”, “Shared representation” | “The approach was based on the co-construction and use of an agent-based model providing a shared representation of the current management of farms and providing multiple viewpoints on alternative forest management scenarios.” (Simon & Etienne 2010) | |
Transformative | Model communication occurs through stakeholder dialogues and collaborative model-building. The primary aim of this participation is to empower stakeholders and foster a sense of ownership over the model. As a result, stakeholders are granted significant control over how the model is utilized. Key point: “ABM as a communication or exploratory tool” | “Aiming to facilitate stakeholder communication and collaboration for cellulosic bioeconomy growth, an agent-based model is developed as a community communication tool in this study… The communication tool is applied to bringing stakeholders and researchers together to develop shared visions, assess barriers, and explore solution portfolios to initialize and speed up the regional cellulosic bioeconomy” (Yang et al. 2022) | |
Classification | Definition | Theoretical basis | Example snippet |
---|---|---|---|
Specification | Specification refers to a detailed description of how something should be done. In model communication, this involves collaborating with stakeholders to define the necessary components for model development or assessment. Key point: Design, creation, formulation, conceptualization, define, specification, development | Open coding. codes identified during the course of literature review, guided by the Cambridge Dictionary (2024) definition of the main keyword. | “The process of participatory modelling involves farmers and agricultural experts in designing the agent-based model and in creating scenarios.” (Smetschka & Gaube 2020) |
Transparency | Transparency is the quality of being easily understood or visible. In model communication, it aims to make the model’s underlying logic and structure accessible to stakeholders. Key point: Accessibility, stakeholder ownership, documentation | “Even if UML diagrams are used to design an ABM, they are also useful when attempting to explain a model… The stakeholders need to understand them in order to assume ownership of the model and to criticize it.” (Bommel et al. 2011) | |
Validation | Validation is the process of ensuring something is officially acceptable or approved. In model communication, it involves engaging stakeholders to perform calibration and validation activities. Key point: Stakeholder validation, calibration | “Designing role-play games alongside a model has great potential as a validation strategy through which to continue a PM process with stakeholders while maintaining equal cultural access to the model among diverse stakeholders. During the prolonged phase in which modelers are assimilating data into the model and stakeholder interactions are limited, games for information validation may be designed and used to re-engage stakeholders even though the model is not yet finished.” (Shelton et al. 2018) | |
Presentation | Presentation refers to the act of showing something to an audience. In model communication, the goal is to display the model to stakeholders in a clear and engaging way. Key point: Result presentation, description | “A number of different visualization tools have been developed to aid in the understanding of these systems and for presentation of results to decision-makers.” (Barry et al. 2008) | |
Reproducibility | Reproducibility means that an experiment can be repeated with the same results. To communicate for reproducibility, the model must be shared with other modelers, enabling them to replicate the model and verify its results. Key point: Reproducible, replicable | “A common language to describe agent-based models, independent of the implementation platform, could be a better means of communication among users or tools than using source code, since it demands a high effort to understand and interpret the models, sometimes leading to misunderstandings. Given that this kind of modeling language is independent of a specific simulation toolkit or library, it should also facilitate model replication.” (Sansores et al. 2006) | |
Extension | Extension refers to expanding the scope or adding new elements to something. In the context of model communication, it involves presenting the model in a way that allows other modelers to update, adapt, or integrate it into new or existing models. Key point: Improvement, evolution, maintenance, archive | “The adoption of diagrammatic visual computer language should however greatly help the immunologists to better communicate, to more easily identify the model’s similarities and facilitate the reuse and extension of existing software models.” (Thomas-Vaslin et al. 2013) | |
Classification | Definition | Theoretical basis | Example snippet |
---|---|---|---|
Document-type | Description of an ABM in narrative or written form. Key point: “Description of the conceptual design”, “Details of the implementation” | Open coding. codes were identified during the course of literature review. | “TRACE documents are meant to provide comprehensive documentation of models that can be submitted as supplementary material with scientific publications, reports, or dossiers where models are presented to support decision making.” (Ayllón et al. 2021) |
Visual | Representation of an ABM in a graphical form. Key point: Visualization, Diagrams | “The diagrams build on the recognition that the workings of an ABM can only be understood through the behavior of the agents, and that the behavior of the agents can only be understood through their percepts, objectives, and actions… Their strength lies in the fact that they visualize the relevant elements and clarify how these are interrelated by explicitly stating their relationships.” (Hall & Virrantaus 2016) | |
Interactive | Representation of an ABM that allow stakeholder to interact with the model. Key point: Participatory, Gamified | “We also aim to stimulate the participants’ thinking and co-learning through the collective exploration of scenarios of varying water and labor availability, with the ultimate goal of further strengthening their adaptive management ability throughout this very interactive participatory modeling and simulation process.” (Naivinit et al. 2010) | |
Category | Classification | Definition | Theoretical basis |
---|---|---|---|
ABM aspects conveyed to stakeholders | Structure | The agents, their interrelationships, and the environment in which they operate. | Macal & North (2010); Cenani (2021) |
Complexity | Interactions within the model. | ||
Emergence | A phenomena characterized by non-linear and often unpredictable behaviors arising during simulations. | ||
Evaluation of the Effectiveness of Communicating ABM to Stakeholders | With Evaluation | The evaluation of the model’s communication was described in the paper. | Cartwright et al. (2016) |
Planned Evaluation | The paper mentioned a plan for future evaluations. | ||
Indirect Evaluation | The evaluation results were derived from the outcomes of another modeling activity (such as open discussions and post-game debriefings). | ||
No Evaluation | There was no mention of the evaluation of the model’s communication. | ||
Appendix B: Additional Files
Additional File 1: Complete corpus details (XLSX 45 kb) available at: https://www.jasss.org/28/2/2/Complete_Corpus_Details.xlsx
References
ADAM, C., & Andonoff, E. (2019). VigiFlood: A serious game for understanding the challenges of risk communication. 16th Conference on Information Systems for Crisis Response and Management (ISCRAM 2019).
ADAM, C., Bailly, C., & Dugdale, J. (2018). Communication during bushfires, towards a serious game for a serious matter. International Journal of Information Systems for Crisis Response and Management, 10(2), 79–105. [doi:10.4018/ijiscram.2018040104]
APPEL, F., Ostermeyer, A., Balmann, A., & Larsen, K. (2010). Improving an agent-Based model by using interdisciplinary approaches for analyzing structural change in agriculture. Lecture Notes in Computer Science. [doi:10.1007/978-3-642-12079-4_45]
ARNSTEIN, S. R. (1969). A ladder of citizen participation. Journal of the American Institute of Planners, 35(4), 216–224. [doi:10.1080/01944366908977225]
AYLLÓN, D., Railsback, S. F., Gallagher, C., Augusiak, J., Baveco, H., Berger, U., Charles, S., Martin, R., Focks, A., Galic, N., Liu, C., Van Loon, E. E., Nabe-Nielsen, J., Piou, C., Polhill, J. G., Preuss, T. G., Radchuk, V., Schmolke, A., Stadnicka-Michalak, J., … Grimm, V. (2021). Keeping modelling notebooks with TRACE: Good for you and good for environmental research and management support. Environmental Modelling & Software, 136, 104932.
BARBROOK-JOHNSON, P., Badham, J., & Gilbert, N. (2017). Uses of agent-Based modeling for health communication: The TELL ME Case Study. Health Communication, 32(8), 939–944. [doi:10.1080/10410236.2016.1196414]
BARRY, P., Koehler, M., Bergin-Hill, T., McMahon, M., Tierney, M., & Jacyna, G. (2008). Optimization of heterogeneous simulations of complex enterprise systems. 2008 2nd Annual IEEE Systems Conference. [doi:10.1109/systems.2008.4518996]
BERSINI, H., Klatzmann, D., Six, A., & Thomas-Vaslin, V. (2012). State-Transition diagrams for biologists. PLoS One, 7(7), e41165. [doi:10.1371/journal.pone.0041165]
BHARWANI, S., Bithell, M., Downing, T. E., New, M., Washington, R., & Ziervogel, G. (2005). Multi-Agent modelling of climate outlooks and food security on a community garden scheme in Limpopo, South Africa. Philosophical Transactions: Biological Sciences, 360(1463), 2183–2194. [doi:10.1098/rstb.2005.1742]
BINGHAM, A. J. (2023). From data management to actionable findings: A five-Phase process of qualitative data analysis. International Journal of Qualitative Methods, 22, 16094069231183620. [doi:10.1177/16094069231183620]
BOMMEL, P., Dieguez, F., Morales, H., Bartaburu, D., Montes, E., Pereira, M., & Corral, J. (2011). One more step towards participatory modeling. Involving local stakeholders in designing scientific models for participative foresight studies. ESSA’11, 7th Conference of the European Social Simulation Association, Montpellier, France. [doi:10.18564/jasss.2381]
BONABEAU, E. (2002). Agent-Based modeling: Methods and techniques for simulating human systems. Proceedings of the National Academy of Sciences, 99(10), 7280–7287. [doi:10.1073/pnas.082080899]
CAMBRIDGE Dictionary. (2024). English meaning - Cambridge Dictionary. Cambridge: Cambridge University Press.
CARMENATE, T., Inyim, P., Pachekar, N., Chauhan, G., Bobadilla, L., Batouli, M., & Mostafavi, A. (2016). Modeling occupant-Building-Appliance interaction for energy waste analysis. Procedia Engineering, 145, 42–49. [doi:10.1016/j.proeng.2016.04.012]
CARTWRIGHT, S., Bowgen, K., Collop, C., Hyder, K., Nabe-Nielsen, J., Stafford, R., Stillman, R., Thorpe, R., & Sibly, R. (2016). Communicating complex ecological models to non-scientist end users. Ecological Modelling, 338, 51–59. [doi:10.1016/j.ecolmodel.2016.07.012]
CENANI, S. (2021). Emergence and complexity in agent-based modeling: Review of state-of-the-art research. Journal of Computational Design, 2(2), 1–24.
CISSE, A., CISSE, A., Bah, A., Ndjone, J., & Kébé, C. (2015). A methodology for designing agent-based models: Agent from « UP » for complex systems. Lecture Notes in Electrical Engineering, 312, 493–503. [doi:10.1007/978-3-319-06764-3_63]
COLLINS, A., Petty, M., Vernon-Bido, D., & Sherfey, S. (2015). A call to arms: Standards for agent-Based modeling and simulation. Journal of Artificial Societies and Social Simulation, 18(3), 12. [doi:10.18564/jasss.2838]
COMAI, S., Simeone, D., Ventura, S. M., & Ciribini, A. L. C. (2022). Simulation modelling in a BIM environment: The case of school re-opening during Covid-19 pandemic. Proceedings of the Institution of Civil Engineers: Smart Infrastructure and Construction, 176(1), 12–23. [doi:10.1680/jsmic.21.00026]
DALY, A. J., De Visscher, L., Baetens, J. M., & De Baets, B. (2022). Quo vadis, agent-based modelling tools? Environmental Modelling & Software, 157, 105514. [doi:10.1016/j.envsoft.2022.105514]
DUMRONGROJWATTHANA, P., Le Page, C., Gajaseni, N., & Trébuil, G. (2011). Co-constructing an agent-based model to mediate land use conflict between herders and foresters in northern Thailand. Journal of Land Use Science, 6(2–3), 101–120. [doi:10.1080/1747423x.2011.558596]
EDMONDS, B., Le Page, C., Bithell, M., Chattoe-Brown, E., Grimm, V., Meyer, R., Montañola-Sales, C., Ormerod, P., Root, H., & Squazzoni, F. (2019). Different modelling purposes. Journal of Artificial Societies and Social Simulation, 22(3), 6. [doi:10.18564/jasss.3993]
EPSTEIN, J. M. (2008). Why model? Journal of Artificial Societies and Social Simulation, 11(4), 12.
ÉTIENNE, M. (2014). Companion Modelling. Dordrecht: Springer Dordrecht.
FORRESTER, J., Greaves, R., Noble, H., & Taylor, R. (2014). Modeling social-ecological problems in coastal ecosystems: A case study. Complexity, 19(6), 73–82. [doi:10.1002/cplx.21524]
GARIBAY, I., Gunaratne, C., Yousefi, N., & Scheinert, S. (2019). The agent-Based model canvas: A modeling lingua franca for computational social science. In P. K. Davis, A. O’Mahony, & J. Pfautz (Eds.), Social‐Behavioral Modeling for Complex Systems (pp. 521–544). Hoboken, NJ: John Wiley & Sons. [doi:10.1002/9781119485001.ch22]
GOURMELON, F., Chlous-Ducharme, F., Kerbiriou, C., Rouan, M., & Bioret, F. (2013). Role-playing game developed from a modelling process: A relevant participatory tool for sustainable development? A co-construction experiment in an insular biosphere reserve. Land Use Policy, 32, 96–107. [doi:10.1016/j.landusepol.2012.10.015]
GRIGNARD, A., Macià, N., Alonso Pastor, L., Noyman, A., Zhang, Y., & Larson, K. (2018). CityScope Andorra: A multi-Level interactive and tangible agent-Based visualization. Proceedings of the 17th International Conference on Autonomous Agents and MultiAgent Systems, Richland, SC.
GRIMM, V., Berger, U., Bastiansen, F., Eliassen, S., Ginot, V., Giske, J., Goss-Custard, J., Grand, T., Heinz, S. K., Huse, G., Huth, A., Jepsen, J. U., JNørgensen, C., Mooij, W. M., Müller, B., Pe’er, G., Piou, C., Railsback, S. F., Robbins, A. M., … DeAngelis, D. L. (2006). A standard protocol for describing individual-based and agent-based models. Ecological Modelling, 198(1), 115–126. [doi:10.1016/j.ecolmodel.2006.04.023]
GRIMM, V., & Railsback, S. F. (2005). Individual-Based modeling and ecology. Princeton, NJ: University Press.
GRIMM, V., & Railsback, S. F. (2012). Designing, formulating, and communicating agent-Based models. In A. J. Heppenstall, A. T. Crooks, L. M. See, & M. Batty (Eds.), Agent-Based Models of Geographical Systems (pp. 361–377). Dordrecht: Springer Netherlands. [doi:10.1007/978-90-481-8927-4_17]
GRÖSSLER, A. (2007). System dynamics projects that failed to make an impact. System Dynamics Review, 23, 437–452.
GURUNG, T. R., Le Page, C., & Trébuil, G. (2022). Collaborative modeling and simulation to mitigate high-Elevation rangeland degradation in Eastern Bhutan. Mountain Research and Development, 42(4), D14–D24. [doi:10.1659/mrd-journal-d-21-00067]
HALL, A., & Virrantaus, K. (2016). Visualizing the workings of agent-based models: Diagrams as a tool for communication and knowledge acquisition. Computers, Environment and Urban Systems, 58, 1–11. [doi:10.1016/j.compenvurbsys.2016.03.002]
HASSAN, S., Fuentes-Fernández, R., Galán, J. M., López, A., & Pavón, J. (2009). Reducing the modeling gap: On the use of metamodels in agent-based simulation. 6th Conference of the European Social Simulation Association, ESSA 2009.
HAVEMAN, S. P., & Bonnema, G. M. (2015). Communication of simulation and modelling activities in early systems engineering. Procedia Computer Science, 44, 305–314. [doi:10.1016/j.procs.2015.03.021]
JANSSEN, M. A., Alessa, L. N., Barton, M., Bergin, S., & Lee, A. (2008). Towards a community framework for agent-Based modelling. Journal of Artificial Societies and Social Simulation, 11(2), 6.
JOFFRE, O., Bosma, R., Ligtenberg, A., Tri, V., Ha, T., & Bregt, A. (2015). Combining participatory approaches and an agent-based model for better planning shrimp aquaculture. Agricultural Systems, 141, 149–159. [doi:10.1016/j.agsy.2015.10.006]
LANGLOIS, P., Blanpain, B., & Daudé, E. (2015). Modeling agent in geography (MAGeo), a modeling platform and multi-agent simulation for social sciences. CyberGeo, 2015, 51–88. [doi:10.4000/cybergeo.27236]
LICHTER, M., Grinberger, A. Y., & Felsenstein, D. (2015). Simulating and communicating outcomes in disaster management situations. ISPRS International Journal of Geo-Information, 4(4), 1827–1847. [doi:10.3390/ijgi4041827]
LOTZMANN, U., Neumann, M., & Moehring, M. (2015). From text to agents - Process of developing evidence-Based simulation models. ECMS 2015 Proceedings. [doi:10.7148/2015-0071]
LÓPEZ-PAREDES, A., Saurí, D., & Galán, J. M. (2005). Urban water management with artificial societies of agents: The FIRMABAR simulator. SIMULATION, 81(3), 189–199.
MACAL, C. M., & North, M. J. (2010). Tutorial on agent-based modelling and simulation. Journal of Simulation, 4(3), 151–162. [doi:10.1057/jos.2010.3]
MOEINIYAN Bagheri, S., Smith, G., & Hanan, J. (2015). Using Z in the development and maintenance of computational models of real-World systems. Software Engineering and Formal Methods. [doi:10.1007/978-3-319-15201-1_3]
MONKS, T., Currie, C. S. M., Onggo, B. S., Robinson, S., Kunc, M., & Taylor, S. J. E. (2019). Strengthening the reporting of empirical simulation studies: Introducing the STRESS guidelines. Journal of Simulation, 13(1), 55–67. [doi:10.1080/17477778.2018.1442155]
MÜLLER, B., Balbi, S., Buchmann, C. M., de Sousa, L., Dressler, G., Groeneveld, J., Klassert, C. J., Bao Le, Q., Millington, J. D. A., Nolzen, H., Parker, D. C., Polhill, J. G., Schlüter, M., Schulze, J., Schwarz, N., Sun, Z., Taillandier, P., & Weise, H. (2014). Standardised and transparent model descriptions for agent-based models: Current status and prospects. Environmental Modelling & Software, 55, 156–163.
NAIVINIT, W., Le Page, C., Trébuil, G., & Gajaseni, N. (2010). Participatory agent-based modeling and simulation of rice production and labor migrations in Northeast Thailand. Environmental Modelling & Software, 25(11), 1345–1358. [doi:10.1016/j.envsoft.2010.01.012]
OMG. (2006). Meta object facility (MOF) core specification. https://www.omg.org/spec/MOF/2.0/PDF.
ONGGO, B. (2013). Agent-Based simulation model representation using BPMN. Formal Languages for Computer Simulation: Transdisciplinary Models and Applications. [doi:10.4018/978-1-4666-4369-7.ch012]
ONGGO, B. S. S., & Karpat, O. (2011). Agent-Based conceptual model representation using BPMN. Proceedings of the Winter Simulation Conference, Phoenix, Arizona. [doi:10.1109/wsc.2011.6147795]
OPENAI. (2023). GPT-3 Language Model. Accessed August 2023.
OZIK, J., Collier, N., Combs, T., Macal, C. M., & North, M. (2015). Repast Simphony Statecharts. Journal of Artificial Societies and Social Simulation, 18(3), 11. [doi:10.18564/jasss.2840]
POLHILL, G. (2015). Extracting OWL ontologies from agent-Based models: A Netlogo extension. Journal of Artificial Societies and Social Simulation, 18(2), 15. [doi:10.18564/jasss.2810]
POLHILL, J. G., & Gotts, N. M. (2009). Ontologies for transparent integrated human-natural system modelling. Landscape Ecology, 24(9), 1255–1267. [doi:10.1007/s10980-009-9381-5]
POLHILL, J., Parker, D., Brown, D., & Grimm, V. (2008). Using the ODD protocol for describing three agent-based social simulation models of land-use change. Journal of Artificial Societies and Social Simulation, 11(2), 3. [doi:10.18564/jasss.1563]
POOYANDEH, M., & Marceau, D. J. (2013). A spatial web/agent-based model to support stakeholders’ negotiation regarding land development. Journal of Environmental Management, 129, 309–323. [doi:10.1016/j.jenvman.2013.07.028]
RADINSKY, J., Milz, D., Zellner, M., Pudlock, K., Witek, C., Hoch, C., & Lyons, L. (2017). How planners and stakeholders learn with visualization tools: Using learning sciences methods to examine planning processes. Journal of Environmental Planning and Management, 60(7), 1296–1323. [doi:10.1080/09640568.2016.1221795]
RAILSBACK, S. F., & Grimm, V. (2011). Agent-Based and Individual-Based Modeling: A Practical Introduction Princeton, NJ: Princeton University Press.
ROXBURGH, N., Evans, A., Gc, R., Malleson, N., Heppenstall, A., & Stringer, L. (2021). An empirically informed agent-based model of a Nepalese smallholder village. METHODSX, 8. [doi:10.1016/j.mex.2021.101276]
RUANKAEW, N., Le Page, C., Dumrongrojwattana, P., Barnaud, C., Gajaseni, N., Van Paassen, A., & Trébuil, G. (2010). Companion modelling for integrated renewable resource management: A new collaborative approach to create common values for sustainable development. International Journal of Sustainable Development and World Ecology, 17(1), 15–23. [doi:10.1080/13504500903481474]
RUMESER, D., & Emsley, M. (2016). Key challenges of system dynamics implementation in project management. Procedia - Social and Behavioral Sciences, 230. [doi:10.1016/j.sbspro.2016.09.004]
SANSORES, C., Pavón, J., & Gómez-Sanz, J. (2006). Visual modeling for complex agent-Based simulation systems. In J. S. Sichman & L. Antunes (Eds.), Multi-Agent-Based Simulation VI (pp. 174–189). Berlin Heidelberg: Springer. [doi:10.1007/11734680_13]
SCHERER, S., Wimmer, M. A., & Markisic, S. (2013). Bridging narrative scenario texts and formal policy modeling through conceptual policy modeling. Artificial Intelligence and Law, 21(4), 455–484. [doi:10.1007/s10506-013-9142-2]
SHELTON, R. E., Baeza, A., Janssen, M. A., & Eakin, H. (2018). Managing household socio-hydrological risk in Mexico city: A game to communicate and validate computational modeling with stakeholders. Journal of Environmental Management, 227, 200–208. [doi:10.1016/j.jenvman.2018.08.094]
SHINDLER, B., Spies, T. A., Bolte, J. P., & Kline, J. D. (2017). Integrating ecological and social knowledge: Learning from CHANS research. Ecology and Society, 22(1). [doi:10.5751/es-08776-220126]
SIBERTIN-BLANC, C., Therond, O., Monteil, C., & Mazzega, P. (2019). The entity-Process framework for integrated agent-Based modeling of social-Ecological systems. In R. Boulet, C. Lajaunie, & P. Mazzega (Eds.), Law, Public Policies and Complex Systems: Networks in Action (Vol. 42, pp. 57–86). Cham: Springer International Publishing. [doi:10.1007/978-3-030-11506-7_4]
SIMON, C., & Etienne, M. (2010). A companion modelling approach applied to forest management planning. Environmental Modelling & Software, 25(11), 1371–1384. [doi:10.1016/j.envsoft.2009.09.004]
SINITSKAYA, E., Gomez, K. J., Bao, Q., Yang, M. C., & MacDonald, E. F. (2020). Designing linked journey maps to understand the complexities of the residential solar energy market. Renewable Energy, 145, 1910–1922. [doi:10.1016/j.renene.2019.06.018]
SMETSCHKA, B., & Gaube, V. (2020). Co-creating formalized models: Participatory modelling as method and process in transdisciplinary research and its impact potentials. Environmental Science and Policy, 103, 41–49. [doi:10.1016/j.envsci.2019.10.005]
SUN, Z., Lorscheid, I., Millington, J. D., Lauf, S., Magliocca, N. R., Groeneveld, J., Balbi, S., Nolzen, H., Müller, B., Schulze, J., & Buchmann, C. M. (2016). Simple or complicated agent-based models? A complicated issue. Environmental Modelling & Software, 86, 56–67. [doi:10.1016/j.envsoft.2016.09.006]
TAGHIKHAH, F., Voinov, A., Filatova, T., & Polhill, J. G. (2022). Machine-assisted agent-based modeling: Opening the black box. Journal of Computational Science, 64, 101854. [doi:10.1016/j.jocs.2022.101854]
TAILLANDIER, P. (2014). GAMAGraM: Graphical modeling with the GAMA platform. The 4th International Conference on Complex Systems and Applications.
TEHRANI, M. A. (2020). An agent-based model of stem and cancer cell interaction. PhD Thesis, University of Sheffield.
THOMAS-VASLIN, V., Six, A., Ganascia, J.-G., & Bersini, H. (2013). Dynamical and mechanistic reconstructive approaches of T Lymphocyte dynamics: Using visual modeling languages to bridge the gap between immunologists, theoreticians, and programmers. Frontiers in Immunology, 4. [doi:10.3389/fimmu.2013.00300]
TOPPING, C., Odderskaer, P., & Kahlert, J. (2013). Modelling skylarks (Alauda arvensis) to predict impacts of changes in land management and policy: Development and testing of an agent-Based model. PLoS One, 8(6), e65803. [doi:10.1371/journal.pone.0065803]
VAN Bruggen, A., Nikolic, I., & Kwakkel, J. (2019). Modeling with stakeholders for transformative change. Sustainability, 11(3), 825. [doi:10.3390/su11030825]
VOINOV, A., & Bousquet, F. (2010). Modelling with stakeholders. Environmental Modelling & Software, 25(11), 1268–1281. [doi:10.1016/j.envsoft.2010.03.007]
WALDHERR, A., & Wijermans, N. (2013). Communicating social simulation models to sceptical minds. Journal of Artificial Societies and Social Simulation, 16(4), 13. [doi:10.18564/jasss.2247]
WANG, S., Scells, H., Koopman, B., & Zuccon, G. (2023). Can ChatGPT write a good boolean query for systematic review literature search? Association for Computing Machinery, New York, NY, USA. [doi:10.1145/3539618.3591703]
WHITE, S. C. (1996). Depoliticising development: The uses and abuses of participation. Development in Practice, 6(1), 6–15. [doi:10.1080/0961452961000157564]
YANG, P., Cai, X., Hu, X., Zhao, Q., Lee, Y., Khanna, M., Cortés-Peña, Y. R., Guest, J. S., Kent, J., Hudiburg, T. W., Du, E., John, S., & Iutzi, F. (2022). An agent-based modeling tool supporting bioenergy and bio-product community communication regarding cellulosic bioeconomy development. Renewable and Sustainable Energy Reviews, 167. [doi:10.1016/j.rser.2022.112745]
YU, Z., Rouse, W., Serban, N., & Veral, E. (2016). A data-rich agent-based decision support model for hospital consolidation. Journal of Enterprise Transformation, 6(3–4), 136–161. [doi:10.1080/19488289.2016.1248802]