Introduction
In the fall of 2015, France hosted the 21st Session of the Conference of the Parties to the United Nations Framework Convention on Climate Change. The ambition of the conference has been to achieve a new international agreement on climate change response, aiming to limit global warming to 2°C (COP21 2015). In the past, reaching a global agreement on climate change has proven to be very difficult (Weiler 2012). The difficulty stems from the collective nature of the problem, with common but differentiated ethical responsibilities among the different stakeholders, in combination with the many uncertainties inherent to it (Ostrom 2012). In contrast to the extensive research on the climate system and the impact of anthropogenic activities on it, the different ways in which mankind will possibly respond to climate change has received substantially less attention in modelling and scenario studies that explore the problem of limiting global warming, (Giupponi et al. 2013). Moreover, given the magnitude of the challenge of responding to climate change, there is a clear need for research on this. Society can respond in two ways to climate change. Either it adapts to the consequences of climate change, or it aims to mitigate climate change. In this research, we focus on exploring how climate change mitigation action by society can emerge.
Two issues hamper the modelling of society’s response to climate change. First, the emergence of mitigation action by society is subject to many uncertain factors. Although there is scientific consensus about the fact that human activities influence climate change, there is uncertainty about the exact extent of this influence and about the severity of the future impacts of climate change (Tompkins and Adger 2005; van Vuuren et al. 2011). Uncertainty also exists with respect to the exact mechanisms that underlie society’s response to climate change, such as the time preference citizens apply when valuing future costs and benefits of climate change response (Tol et al. 2013). These uncertainties result in a widespread lack of consensus, both within the scientific community and among policy makers, on the essential facts and mechanisms that determine the overarching dynamics of society’s response to climate change. This effect is amplified by the long time horizon over which the uncertainties manifest (Moss et al. 2010; Balbi and Giupponi 2009; Weaver et al. 2013).
The second issue hampering the model-based investigation of the emergence of climate change mitigation by society difficult, is that the system in which climate change mitigation action by society emerges, is governed and influenced by a network of many interacting actors. Climate mitigation, on the one hand emerges from international negotiations between state governments like the one in Paris in the fall of 2015. On the other hand, various other members of society such as citizens, companies, and subnational governmental bodies, take mitigation actions themselves and pressure their representatives at the international negotiations (Hsu et al. 2015). The various actors involved learn from their behaviour and from the behaviour of others, resulting in behavioural change. Simultaneously, the behaviour of the various actors is subject to bounded rationality. The system behaviour emerging from this self-organising emergent system cannot be predicted by understanding the individual actors and their motivations in isolation (Hughes et al. 2013). Gaining insight in this complex system requires accounting for issues such as the heterogeneity of actors and the bounded rationality in their behaviour; influential factors within the energy transition (Giupponi et al. 2013; Bale et al. 2015). The intrinsic complexity of the system of interaction actors, coupled with the inherent uncertainty, imply that the overarching dynamics of society’s response to climate change are emergent, complex and co-evolving (Rammel et al. 2007; Chappin 2011).
Currently society’s response to climate change has not been incorporated adequately in modelling studies on the energy system, climate change and associated impact as researchers have widely acknowledged (Balbi and Giupponi 2009; Brede and de Vries 2013; Giupponi al. 2013; Hughes et al. 2013; Pfenninger et al. 2014). Moreover, limited attention has been given in the literature to the consequences of uncertainties influencing society’s response to climate (Gers et al. 2013). In this research, we contribute to both challenges simultaneously by developing scenarios for the emergence of climate mitigation. The main rationale for developing scenarios is that the relevant time span – the time over which the consequences of climate change play out – is very long, and the possible consequences of uncertainties are amplified due to the complex and chaotic dynamics of the system in which climate change takes place (van Vuuren et al. 2012). Scenarios provide an intuitive means to characterise and communicate information about uncertain future states of a system (Hughes 2009; Bryant and Lempert 2010; Gerst et al. 2013).
In contrast to conventional scenario development processes by means of an intuitive logics or scenario axes method (van der Heijden 1996; Wright and Cairns 2011), we have used a model-based bottom up scenario development process following Rozenberg et al. (2014). This process starts with the development of an agent-based model of the dynamics of the emergence of society’s response to climate change. Agent-based modelling is a modelling technique that can be used to model the actions of and interactions between agents under influence of their state and the state of the environment. This modelling technique furthermore allows for simulating autonomous and heterogeneous agents with bounded rationality and adaptive agent behaviour over local interactions. In this way, the emergence of macroscopic regularities can be discovered from a bottom-up perspective (Epstein 2007). We argue that the use of agent-based modelling addresses the challenges posed by the complexity of society’s response to climate change. In the development of the model, particular attention has been given to the various unresolvable uncertainties in the inputs to the model, and uncertainties pertaining specific mechanisms within the model. Next, following the model-based bottom up scenario development process, we have explored the consequences of these various unresolved uncertainties using a series of computational experiments (Bankes 1993; Bankes et al. 2013). The results from these computational experiments are analysed using scenario discovery (Bryant and Lempert 2010). We contend that scenario discovery offers a useful additional technique for the analysis of outputs of agent-based models (Lee et al. 2015). Scenario discovery provides insights into the subspaces of the uncertainty space that result in characteristic model outputs (see Figure 1). These subspaces can subsequently be communicated as narratives of the different scenarios.
The structure of the paper follows the scenario development process and starts with a more detailed description of the model and associated uncertain factors, which is given in Section 2. In Section 3, we provide additional background on scenario discovery and the exploratory modelling approach. Section 4 presents the results of applying this bottom up scenario development approach to the agent-based model after which the key conclusions of the results are presented in Section 5. Finally, Section 6 contains a discussion on the model and the applied methodology.
Model description
Climate mitigation action by society is the result of international treaties, and behavioural change by national governments and subnational actors. We conceptualize this in a highly stylised agent-based model, inspired by the work of Putnam (1988) on two-level games, describing the time period of 2000-2100, with a time step of a year. The higher level represents the international climate negations between governments. The lower level represents the dynamics within a country where citizens change their behaviour and pressure their government with respect to their government’s position in the international climate negotiations, while the government actor imposes climate mitigation targets in light of any international climate mitigation agreement.
Governments and citizens
The model makes a distinction between two types of agents: governments and citizens. The model consists of 5 governments and 100 citizens related to each government. Governments are state actors that negotiate internationally and impose agreements locally when established. The citizen agent is a catchall category for all subnational actors, including individual citizens, subnational governmental bodies like municipalities and provinces, and companies. This is a substantial simplification, but it makes the representation of the lower level dynamics more tractable. We return to this simplification in the discussion. In the model, both government agents and citizen agents emit greenhouse gases. They can reduce their emissions through mitigation action. Mitigation action emerges out of the actions and interactions of governments and citizens related to GHG emission reduction. The actions to mitigate are influenced by the agents’ awareness of climate change, by climate disasters and by enforced mitigation action as a result of international negotiations on climate change response.
Climate change awareness
Any decision to unilaterally reduce emissions is taken by both governments and citizens, based on their climate change awareness. This climate change awareness differs between agents and is dependent on the time horizon of the agent. Governments and citizens individually predict future cumulative GHG emissions given their time horizon, and assess the associated climate change impact following the climate change impact curve depicted in Figure 2. Next, the predicted future climate change impacts are translated into climate change awareness level. Based on the level of climate change awareness, each government and each citizen decides on whether and by how much they are going to reduce their individual GHG emissions. Because time horizons vary between governments and citizens, differences in climate change awareness occur, which subsequently results in differences in the emission reduction behaviour of governments and citizens.
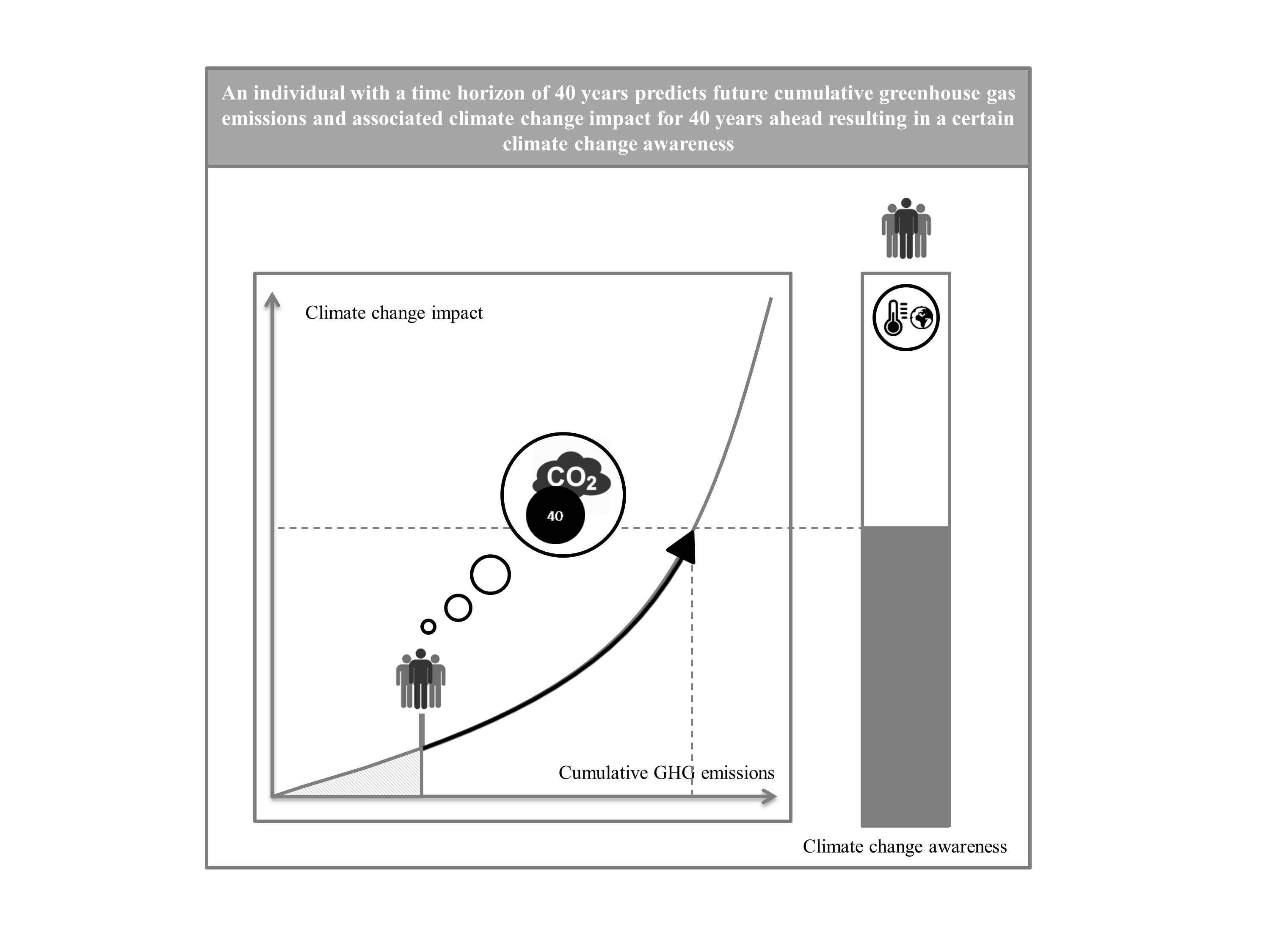
Interaction between agents
At the lower level, citizens pressure their government’s mitigation policy and their government’s position in the international climate negotiations through voting. The extent to which governments act in the interest of the sub-national actors they represent, depends on the democratic property of the government, a heterogeneous characteristic of government agents. The representation of the international negotiations is based on the bargaining game of Smead et al. (2014), together with additional ideas from other climate change negotiation games (Pinto and Harrison 2003; Forgó et al. 2005; Courtois and Tazdaït 2007). Smead et al. (2014) propose a modified version of the N-player Nash bargaining game, where each player represents a negotiating country. The bargaining game incorporates learning dynamics, by representing adaptive players having different emission reduction demands. In this research, governments negotiate on the mitigation goals and whether or not to participate in the final agreement. By applying game theory, the model allows for the formation of coalitions, cooperative solutions, and free-rider behaviour. In the model, we included two alternative game theoretic representations of the negotiations: a cooperative and a competitive representation. In the competitive representation, governments can choose a free-rider strategy in the negotiations.
Depending on the number of governments agreeing (pa) to the emission reduction targets in the first round of the negotiations, and the desired emission reduction targets governments want to reach, a new emission reduction target is set. This new emission reduction target is calculated as the mean of the negotiation positions of the governments, with the not-participating governments having a negotiation position of 0 (T1 = Σpa / n). The reasoning behind this assumption is that the total level of mitigation action that can be proposed in an agreement depends on the number of participating governments. In the second round of negotiations, governments deliberate once more if the emission reduction target is in line with its negotiation position (T1 <= pi). A final emission reduction target is then set according to the agreeing governments (T = Σpa / n). Governments then enforce this emission reduction target T to themselves and their individuals, increasing the voluntary emission reduction level with an enforced emission reduction level.
The model also allows for free-rider behaviour, if a third round of negotiations is executed. In this third round, governments can decide to exit the agreement at the last minute, leaving the emission reduction target for other participating governments the same, without contributing. This decision is made by determining whether the final emission reduction target is larger than its negotiation position (T >= pi).
The emission reduction target of a government is dependent on its citizens. It is assumed that individuals can explicate their preferences on the desired climate change response of their governments through voting. The extent to which governments consider this opinion is determined by the democratic value of governments. This is conceptualised by governments taking the average climate change awareness of the individuals related to that government into account, with respect to their democratic value. This democratic value is another heterogeneous property of governments. Governments can influence mitigation action by individuals in turn, by enforcing mitigation action through top-down legislative, regulatory and economic incentives. It is assumed that governments will enforce extra mitigation action of the individuals related to the government if they participate in an international agreement on climate change response. This top-down enforcement of mitigation action is conceptualised as an increase in mitigation action by governments and individuals to whom the mitigation action is enforced.
Climate disasters
A final element in the model is disasters. With the model, the influence of climate-change related disasters on climate change awareness can be explored. Climate disasters occur locally, randomly affecting only a subset of the countries in the model. Climate disasters temporarily influence either the climate change awareness or the amount of mitigation action of the governments and citizens affected. The influence can either be positive, implying that climate disasters increase demand for mitigation action, or negative, implicitly implying that climate disasters increase demand for adaptation action, thus decreasing the demand for mitigation action.
Synthesis
We argue that the presented stylized agent-based model is adequate for understanding and exploring the dynamics between heterogeneous governments and citizens. The stylized representation also enables us to conduct a range of analyses, within reasonable computational requirements. In the analyses, the most important outcomes of the model are the cumulative GHG reduction, and the annual GHG reduction relative to the initial value in the model. The model is implemented in NetLogo and can found online athttps://www.openabm.org/model/4911/version/1/view. For a more detailed description of the model, see the formal model description following the ODD-protocol (Grimm et al. 2010) in the supplementary material.
Uncertainties influencing the emergence of mitigation action by society in the face of climate change
In order to construct internally consistent and plausible narratives about the pathways of the emergence of mitigation action by society in the face of climate change, uncertainties need to be acknowledged, identified, and their consequences explored. We systematically identify uncertainties using the XLRM framework (Lempert et al. 2006) and a proposed agent-based modelling uncertainty checklist (Greeven 2015). We structure the uncertainties into four categories: uncertainties related to environment settings, agent characteristics, international negotiations on climate change response, and climate disasters. The main uncertainties related to the environment settings are the climate sensitivity and economic sensitivity of climate change. Uncertainties from the agent characteristics category are related to the adaptive traits governments and citizens apply in responding to climate change and factors influencing the interaction between governments and citizens. Uncertainties related to international negotiations on climate change response are factors influencing the internal mechanisms and frequency of these negotiations. Uncertainties related to climate disasters are factors influencing the internal mechanisms and stochastic factors behind the occurrence and effect of climate disasters.
Table 1 specifies the uncertainty space. Some of these uncertainties are represented through a range of values. For example, the climate sensitivity (that is, the sensitivity of the climate to greenhouse gas emission levels) and the economic sensitivity of climate change (that is, the sensitivity of economic impact due to climate change) both have a range of possible values. Other uncertainties are represented as structural uncertainties, allowing switching between different model structures or categorical values of an uncertainty. For example, the model contains both a cooperative game theoretic representation of international negotiations, as well as a competitive game theoretic representation in the form of a repeated prisoner’s dilemma. The left column displays the variable in which uncertainty is incorporated. The middle column describes the assigned uncertainty range for the parametric or structural uncertainties. The column on the right provides an explanation for the assigned uncertainty range.
Uncertainty | Uncertainty range | Explanation and reference |
---|---|---|
Environment settings | ||
Climate sensitivity and economic sensitivity of climate change factor 1 | 1.01 – 1.03 | This factor influences the shape of the climate change impact equation. A low value of this factor represents a high climate sensitivity and economic sensitivity of climate change, and vice versa. The shape of climate change impact equation is based on (Nordhaus 2007;IPCC 2014). |
Climate sensitivity and economic sensitivity of climate change factor 2 | 0.80 – 1.20 | See above explanation |
Emission level change delay years | 1; 3; 5 | Governments and individuals take past years into account when adjusting GHG emission levels. Different delays are explored, ranging from a short delay (1 year) to a longer delay (5 years) |
Agent characteristics | ||
Time horizon of individuals | 0 – 50 | A time horizon of 0 relates to indifference about future climate change impact, whereas a time horizon of 50 could relate to thinking two generations ahead. |
Time horizon of governments | 0 – 50 | See the above explanation |
Standard deviation of time horizon distribution | 0 – 20 | A standard deviation is used to introduce heterogeneity in the individual time horizon of citizens of the same country. A value of 0 relates to a homogeneous distribution, whereas a value of 20 relates to a generation difference in possible distributions. |
Democratic value of governments | 0 – 1 | The democratic value determines the extent to which governments base their mitigation policy on the interest of their citizens. A democratic value of 0 therefore relates to a complete stratocracy, whereas a democratic value of 1 relates to a complete democracy. |
Future cumulative GHG prediction error | 0 – 0.30 | A prediction error is introduced to correct for the fact that governments and citizens do not have the capacity to correctly predict future cumulative GHG emissions. The prediction error ranges from 0 to 0.30, the latter indicating a 30% prediction error. |
International negotiations on climate change response | ||
Years between international negotiations on climate change response | 5; 10; 15 | The frequency of major international negotiations on climate change response has been based on the Kyoto negotiations in 1997 and the Copenhagen negotiations in 2009. This model allows for more frequent or less frequent international negotiations on climate change response. |
Game theoretic representation of international negotiations | Cooperative; Prisoners | Uncertainty exists about the game theoretic representation of the international negotiations on climate change response (Ackerman et al. 2009; Smead et al. 2014). The international negotiations can either be of cooperative nature, in which governments seek a more shared solution for the problem or of a more competitive nature in the form of a repeated prisoner’s dilemma. |
Mitigation enforcement factor | 0.20 – 1 | The strength of the mitigation enforcement that can be a result of international negotiations on climate change response can be altered, ranging from a relatively weak enforcement to a relatively strong enforcement. |
Effect of international policy on citizens | 0.50 – 1 | The mitigation enforcement can have a different effect on citizens and national policy. The strength of this effect can range by adjusting this factor. |
Effect of international policy on national policy | 0.50 – 1 | See above explanation. |
Climate disasters | ||
Base chance of climate disaster | 0.01 – 0.1 | The chance that a climate disaster occurs, independent from the amplifying influence of climate change, can range between climate disasters occurring every year to every 10 years. This range has been based upon major climate disasters that have occurred that past decennia, of which some are accounted to climate change by some people. |
Effect of climate change on climate disasters | 0 – 1 | The relation between climate change and the occurrence of climate disasters is under dispute (Weinkle et al. 2012). This factor determines the strength of the relation between climate change and the occurrence of climate change. The influence of climate change on the occurrence of climate disasters is then subject to the strength of the relation and the amount of cumulative GHG emissions. |
Initial severity of climate disaster | 1; 3; 5 | The influence that a climate disaster has on the climate change awareness of governments and individuals can range from 0 to 0.20 |
Climate disaster memory | 1; 3; 5 | |
Positive effect of climate disasters on climate change awareness | True; False | Scientific evidence on the direction of the effect of climate disasters on mitigation action is limited and in part contradictory. Some literature states that climate disasters have a positive effect on mitigation action (Zahran et al. 2006; Spence et al. 2011;Rudman et al. 2013). Other literature states that climate disasters do not affect mitigation action (Whitmarsh 2008; Dessai and Sims 2010). Finally, there is also literature stating that climate disasters have a negative effect on mitigation action, because those struck by a climate disaster demand adaptation action, pushing mitigation action plans to a further future (Lemonick 2012; Marshall 2012). This ambiguity is captured in a structural uncertainty that allows the model to switch between a positive and negative effect of climate disasters on mitigation action |
Scenario discovery
To address the challenge of constructing narratives on the emergence of mitigation by society in the presence of various uncertainties, we applied an exploratory modelling approach to scenario development using agent-based modelling. The application of agent-based modelling enables us to capture the complexity and emergence of mitigation action by society in the face of climate change (Gerst et al. 2013). To explore the consequences of the various uncertain factors, we use the agent-based model in an exploratory fashion (Bankes 1993; Bankes et al. 2013). We generate series of computational experiments and assess the behaviour of the model across these experiments. To summarise the computational experiments in a small but representative set of scenarios, we applied scenario discovery (Bryant and Lempert 2010; Kwakkel et al. 2013; Rozenberg et al. 2014; Kwakkel and Jaxa-Rozen 2016).
Scenarios provide a commonly used means to communicate and characterize uncertainty by bounding the ways in which the system under study could possibly evolve (van Vuuren et al. 2011). A conventional way of developing scenarios is by means of the intuitive logics or scenario axes method, in which highly uncertain, high impact driving forces form the scenario axes. The scenario logic approach can fail to summarise the multiplicity of plausible futures into a small set of scenarios that is representative of all plausible futures in case of many interdependent uncertain factors (Bryant and Lempert 2010). Moreover, the scenario logic approach relies on the mental models of analysts or stakeholders. It has been found that the mental models which humans (consciously or unconsciously) use to deal with complex systems are typically event based, have an open loop view of causality, ignore feedback, fail to account for time delays, and are insensitive to non-linearity (Sterman 1994). Hence, essential elements of dynamics in complex systems, namely feedback, time delays and non-linearity, cannot be appropriately dealt with. Consequently, mental simulations of complex systems are proven to be highly defective (Sterman 1989; Brehmer 1992; Kleinmuntz 1992; Diehl and Sterman 1995; Dörner 1996; Sastry and Boyd 1998; Atkins et al. 2002). Together, this implies that when one is developing scenarios of the future evolution of complex systems, these scenarios should be derived from an ensemble of runs of one or more bottom up simulation models, rather than feeding scenarios to such models (Allen 1990; Gerst et al. 2013).
Our approach takes up this challenge by generating an ensemble of simulation runs through computational experimentation, which systematically explore the consequences of alternative realisations of the various uncertainties (Kwakkel and Pruyt 2013). This exploratory modelling approach can be used to analyse the consequences of uncertainty on many levels, ranging from parametric uncertainties to structural uncertainties (e.g. different structures or even whole models). A given computational experiment describes one possible resolution to the various uncertain factors. By conducting many of these experiments, insight is generated into the behaviour of the model across the entire space of uncertain factors ((Bankes 1993; Bankes et al. 2013). This space is defined by the particular uncertain factors, and the variable ranges over which each of the factors is considered viable. To support this exploratory modelling, we have used the Exploratory Modelling Workbench developed by Kwakkel (2012). This workbench includes the ability to control NetLogo from Python, which utilizes the java classes that are also used by the RNetLogo controller (Thiele et al. 2012).
The scenario discovery approach has been put forward as a technique that can be used for developing scenarios for problems that involve many uncertainties (Kwakkel et al. 2013). The goal of scenario discovery is to identify the combinations of a small number of input parameters to a simulation model that are most strongly predictive of specific (classes of) model outcomes (Lempert et al. 2006; Groves and Lempert 2007;Bryant and Lempert 2010). In this research, scenario discovery is applied to construct narratives based on internally consistent and plausible assumptions on the emergence of climate change mitigation action by society. The scenario discovery approach that has been applied in this research consists of the following steps:
- Define the key indicator(s) for the subject of the narratives; in the case of this research, the focus is on the emergence of climate change mitigation action by society. An agent-based model is constructed capturing the emergence of climate change mitigation action by society.
- During the conceptual description of the research problem, the system conceptualisation and the model specification, uncertainties influencing the emergence of climate change mitigation action by society are explicitly acknowledged, identified and incorporated in the agent-based model.
- Explore the consequences of the specified uncertainties; a large ensemble of plausible models is generated which covers the space spanned by the uncertainties. By conducting explorative and targeted analyses over this ensemble of plausible models, the consequences of the various uncertainties influencing the emergence of climate change mitigation action by society are explored. As a result, subspaces of the uncertainty space can be identified that are strongly predictive for the emergence or failure of emergence of climate change mitigation action by society.
- Translate the identified subspaces of the uncertainty space into narratives. The number of relevant subspaces and narratives that are communicated are application specific.
In order to identify the subspaces, scenario discovery relies on rule induction algorithms. The most frequently employed algorithm for scenario discovery is the Patient Rule Induction Method (Friedman and Fisher 1999). PRIM aims at finding combinations of values for the uncertain input variables that result in similar characteristic values for an outcome variable. Specifically, PRIM seeks a set of subspaces of the uncertainty space within which the values of a single output variable is considerably different from its average value over the entire space spanned by all the uncertain input variables. PRIM describes these subspaces in the form of hyper rectangular boxes of the uncertainty space. An illustration of what PRIM is trying to achieve is shown in (Figure 3. To complement the insights from scenario discovery, we use partial factorial experimental designs to explore the influence of specific individual uncertainties on the full behavioural landscape. In this partial factorial design, a given uncertain factor is set to a fixed number of possible values, and an ensemble of experiments is generated using Latin Hypercube sampling for the remainder of the uncertain factors. We next explore the dynamics of the model for each of the fixed values for the one uncertain factor across this ensemble. In this way, the influence of the single uncertain factor can be assessed rigorously (see Figure 4).
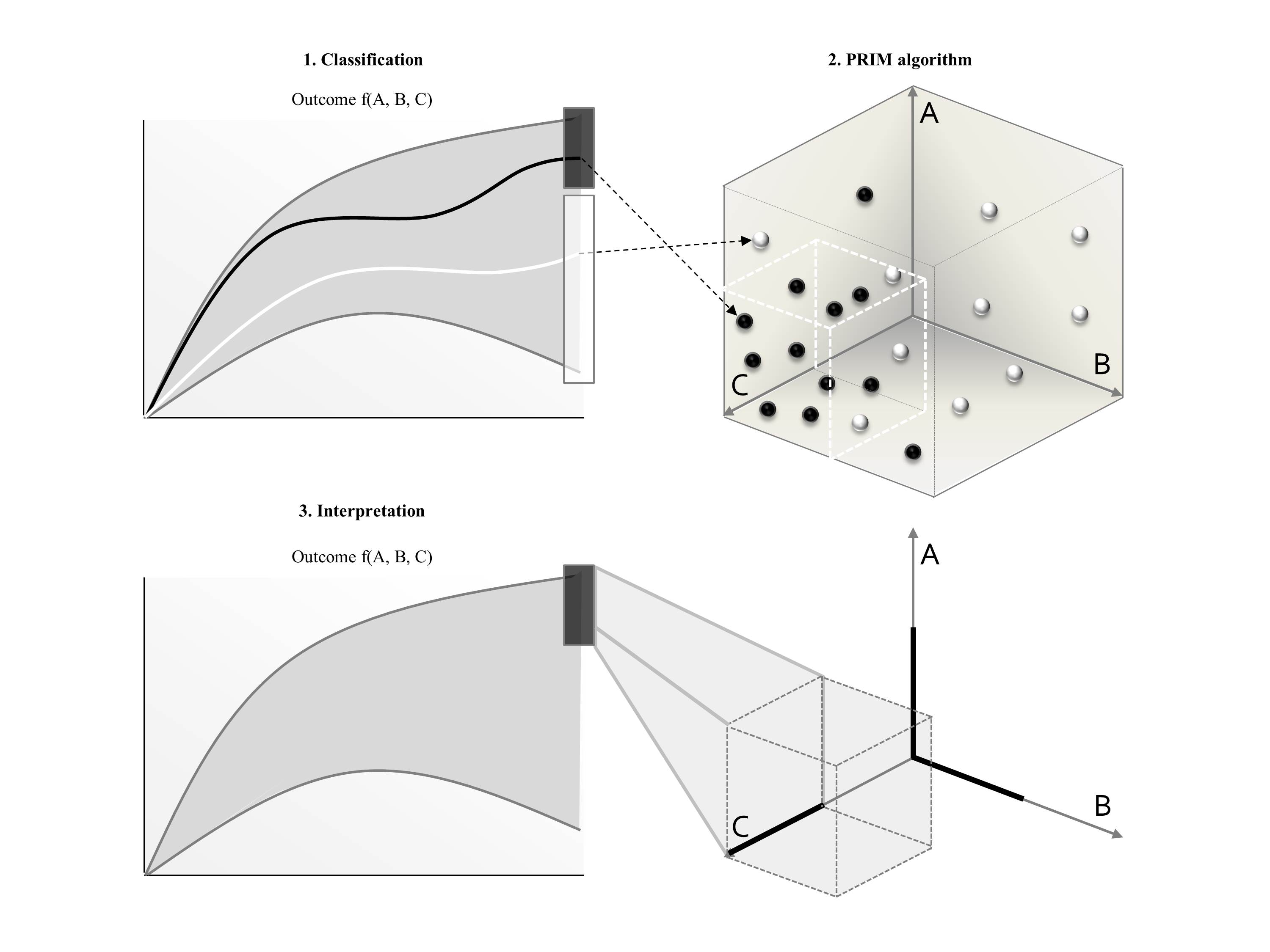
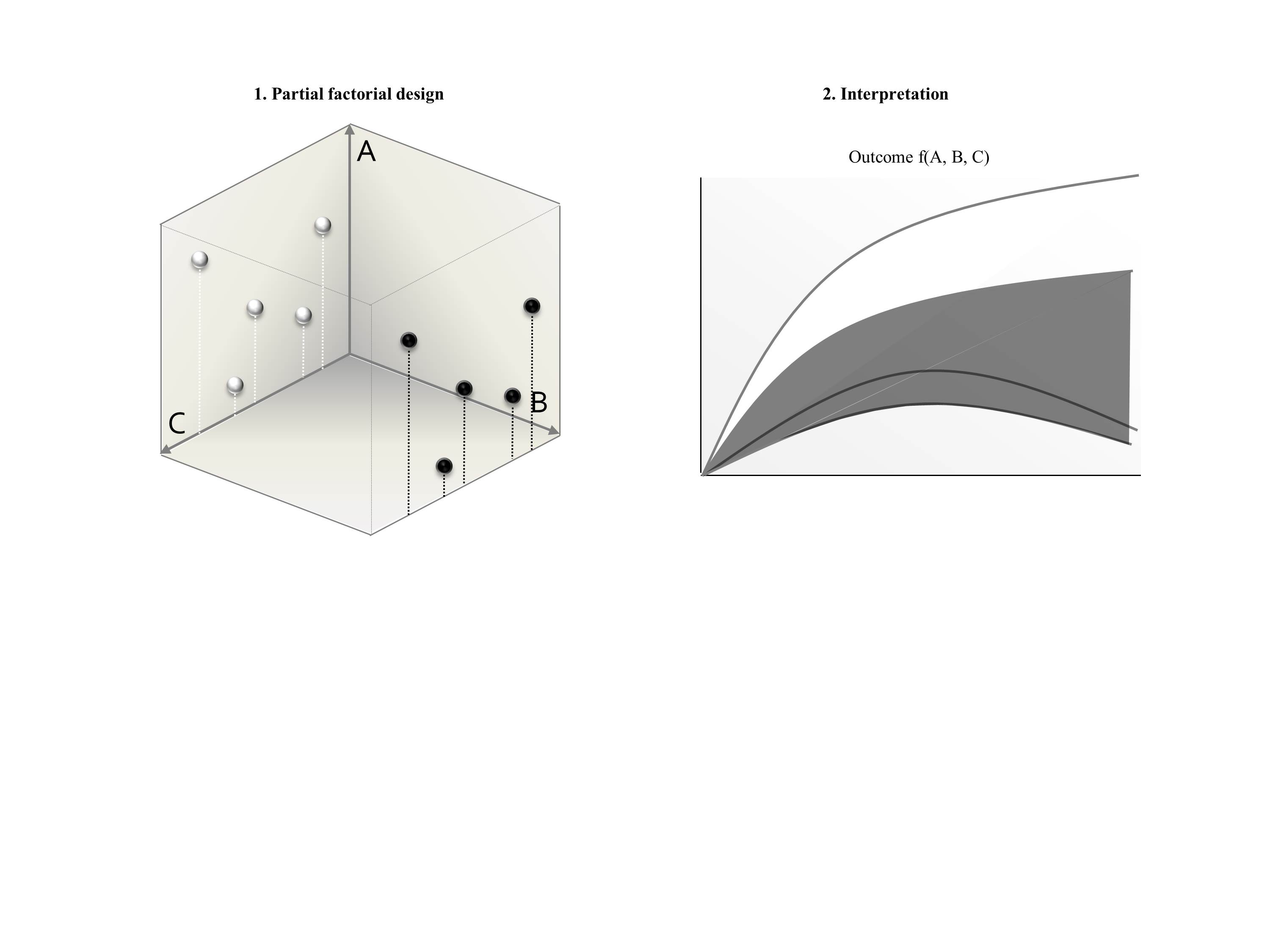
Model outcomes
In this section, we apply scenario discovery and the partial factorial analysis of individual uncertain factors to the agent based model of the emergence of climate mitigation in society. By conducting a large number of computational experiments, this explicit representation of the set of plausible model outcomes is generated. The EMA workbench, developed by Kwakkel (2012), supports generation of this large number of computational experiments and the analysis of the results. For the initial analysis, we generated 1000 computational experiments using Latin Hypercube Sampling. Each of the 1000 experiments is replicated 100 times, in order to account for the stochastic uncertainty present in the model. We arrived at 100 replications through experimentation. The outcome of a given experiment is then the mean over these 100 replications. This implies that in order to map the full uncertainty space, 100.000 model runs are executed. Conducting 100.000 model runs of the model used in this research on a 12-core Xeon workstation took about 3.5 hours to complete. The choice for 1000 experiments is motivated by the fact that we are trying to find a balance between required computation time and having sufficient amount of data to conduct for further analyses. We also observed that increasing the number of experiments did not reveal any strikingly different model behaviour.
In Figure 5, the bandwidth of outcomes across the 1000 computational experiments is shown for the cumulative GHG reduction and the annual GHG reduction. The cumulative GHG reduction is the most important indicator for the dynamics of the emergence of climate change mitigation action by society. The cumulative GHG reduction is determined by dividing the cumulative GHG emission by the cumulative GHG emission if no mitigation action would have been taken, that is, the business as usual GHG emission scenario. The annual GHG emission reduction is calculated in the same way. The annual GHG emission reduction is more easily interpreted, as it can be compared to existing emission reduction goals. However, because the annual GHG emission reduction can be temporarily affected at any point in time, it serves less useful for analysing the emergence of mitigation action over time. The blue shaded areas represent the minimum and maximum values of the model outcomes at any point in time. The large uncertainty space influencing the emergence of climate change mitigation action by society results in large differences in possible outcomes.
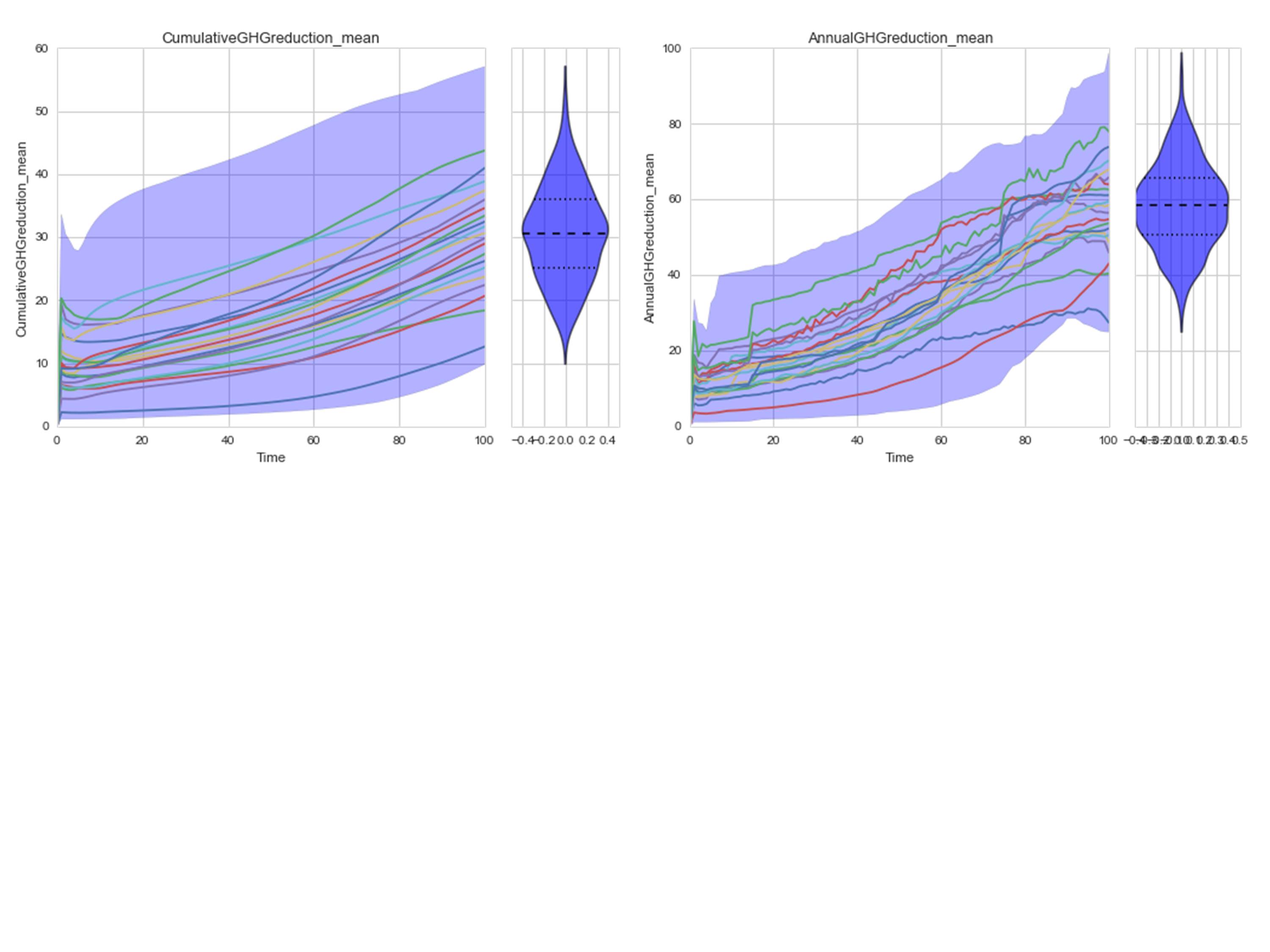
By conducting explorative and targeted analyses on the uncertainty space such as PRIM and the analysis of individual uncertain factors using partial factorial experimental designs, the behavioural landscape of the agent based model of the emergence of climate change mitigation action by society has been explored. We analysed in depth the influence of uncertainties such as the time horizon of governments and citizens, the game theoretic representation of international negotiations, and the effect climate disasters on the demand for mitigation action using the partial factorial experimental design approach. Two examples of these analyses are described below, providing a representation of the various types of analyses used and demonstrating the insights that can be drawn from such analyses. For a complete overview of all analyses conducted on the exploration of the consequences of uncertainties influencing the emergence of climate change mitigation action by society, we refer to the detailed analyses in Greeven (2015).
Figure 6 shows the influence of governments and citizens having a short versus a long time horizon. The blue shaded area represents the envelope of outcomes of 1000 computational experiments spanning the full uncertainty space in which governments and citizens have an average time horizon of 40 years. The green shaded area represents the envelope of outcomes of 1000 experiments spanning the full uncertainty space in which governments and citizens have an average time horizon of 10 years. The large difference in cumulative GHG reduction as a result of the uncertainty related to the time horizon governments and citizens use to assess the future impact of climate change highlights the importance of this single source of uncertainty on the emergence of climate mitigation.
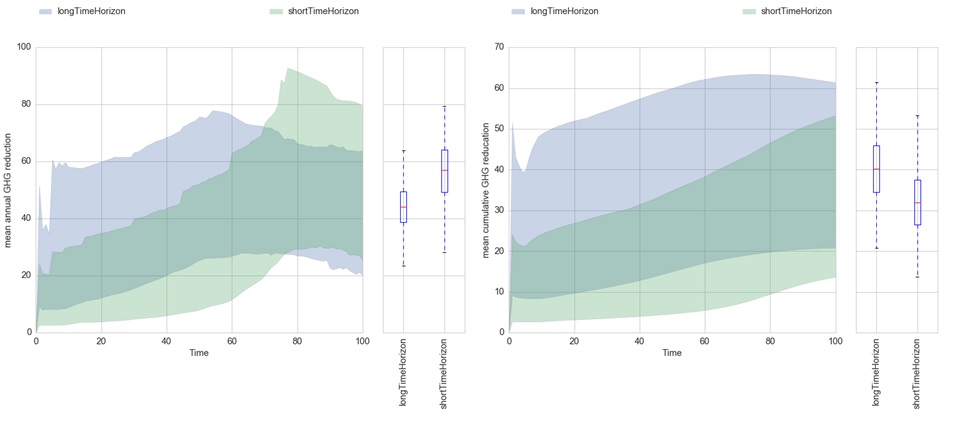
Figure 7 summarizes the results from the PRIM analysis focussed on identifying the combination of uncertain factors where GHG reduction is more than 35% in the year 2100. A high cumulative GHG reduction in 2100 occurs if there is a high climate sensitivity and high economic sensitivity of climate change; in which climate disasters make governments and citizens increase their emission reduction behaviour; and in which the international climate change mitigation negotiation are represented using cooperative game theory.
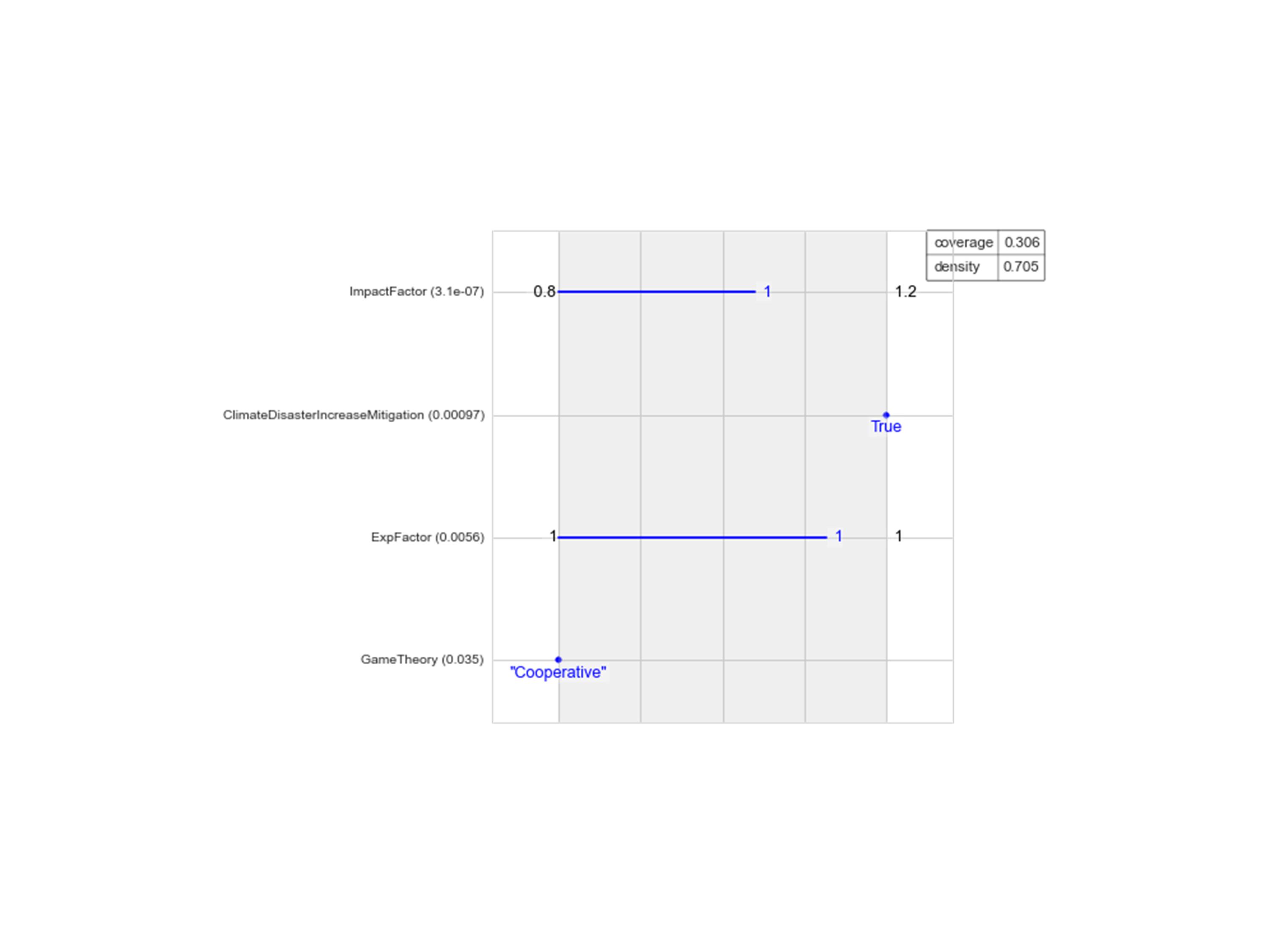
By exploring the consequences of the various uncertain factors influencing the emergence of climate change mitigation action by society using both explorative and targeted analyses, subspaces of the input space have been identified that are strongly predictive for the emergence of climate change mitigation action by society. These subspaces can be translated easily into communicable, internally consistent, and plausible narratives. The scenario discovery method has enabled us therefore to create narratives that naturally follow from the simulation model and its associated uncertain factors. In this research, we focus only on the emergence of climate change mitigation action by society. Therefore, two narratives have been identified, describing how certain sub-spaces of the uncertainty space lead to the co-evolutionary emergence of either strong or weak mitigation action. More narratives can be created if there are more outcomes of interest, or if there are multiple alternative explanations for the same class of outcomes (Guivarch et al. 2016). An example would be to construct four narratives: the emergence of strong mitigation action under a high and a low climate sensitivity and economic sensitivity of climate change, and the emergence of weak mitigation action under a high and a low climate sensitivity and economic sensitivity of climate change. However, the two narratives identified in this research prove to be a succinct representation of the possible outcomes of the emergence of climate change mitigation action by society because of the large uncertainty space. The two narratives are presented below.
Narrative 1: The emergence of strong climate change mitigation action by society
A strong emergence of mitigation action, measures by the amount of GHG emission reduction over the course of the 21st century, is mainly determined by the climate sensitivity and the economic sensitivity of climate change, and the time horizon members of society apply in assessing their belief about required response. In the case of a strong relation between cumulative GHG emissions and climate change, a strong relation between climate change and the economic impact of climate change, and governments and individuals assessing the long-term future impact of climate change, society’s response to climate change will be characterised by high annual GHG emission reductions in the start of the 21st century. Society is concerned about the future impact of climate change that results in high climate change awareness. Stimulated by their citizens, governments are more willing to cooperate in tackling the problem of climate change in the international arena. Furthermore, governments are more cooperative in the negotiations with the aim of taking collective responsibility for this global problem. International negotiations on climate change response will take place frequently and the mitigation agreements resulting from these negotiations consist of stronger mitigation goals. Finally, the potential impact of climate change is becoming visible through the occurrence of climate disasters. This results in an increase in climate change awareness highlighting the importance of taking mitigation action. International negotiations on climate change response that happen to be right after the occurrence of a climate disaster are more successful and result in stronger mitigation goals. In conclusion, this narrative describes a scenario in which climate change is serious, but a conscious society is aware of the future impact climate change can have and is prepared to respond with strong mitigation action.
Narrative 2: The emergence of weak climate change mitigation action by society
Weak mitigation action by society emerges under the circumstances of a low climate sensitivity and economic sensitivity of climate change in combination with a short time horizon among members of society in assessing the future impact of climate change. Because climate change only increases gradually and members of society do not want to adapt their behaviour to the possible consequences climate change may have in the future, little mitigation action is undertaken. International negotiations on climate change response are unsuccessful. The failure of the negotiations is worsened by the fact that some governments are incentivised to free-ride in these negotiations as they want other governments to respond to climate change without taking responsibility themselves. Finally, climate disasters have an adverse effect on the demand of mitigation action. Mitigation action plans are pushed to the future, because those struck by the climate disaster demand to spend their resources on adaptation action. International negotiations on climate change response that are held right after the occurrence of a climate disaster are therefore bound to be unsuccessful to result in ambitious mitigation goals. In conclusion, little mitigation action by society emerges when the impact of climate change is low, in combination with a society unwilling to act in advance to mitigate the future impact of climate change.
Discussion
The agent-based model constructed in this research has been an abstract and primarily theoretical representation of the emergence of climate change mitigation action by society. Many uncertainties and simplification have been made in the system conceptualisation. However, this level of abstraction has been justified by the purpose of the modelling study and the way in which the model has been used: the limitations of the data have been acknowledged, and the consequences of uncertainties within the model have been explored. There has not been a claim for a correct representation of reality, but by applying EMA there has been an exploration over the internally consistent and plausible assumptions that lead to plausible states of the system. In this way, it has been possible to construct internally consistent and plausible narratives about the pathways of society’s response to climate change. Within this research, the benefits of the application of EMA to ABM have been shown. Conversely, it can be concluded that ABM lends itself well to exploratory purposes.
While the narratives might not be particularly surprising, one should note that the methodology applied in this research covers a multiplicity of possible futures into two narratives. Moreover, these narratives illustrate the internal consistency and plausibility of these futures, since they represent subspaces of co-evolutionary emerged futures: they form a generative proof for a relatively simple result. A significant portion of the many and vast uncertainties related to climate response can be captured by these two intuitive narratives.
Translating the results of this research into to comprehensive policy advice would require additional research but we want to highlight that, although the results might be found unsatisfying in the sense that they do not provide clear policy suggestions, the methodology which we used to arrive at our results can give policy makers additional food for thought. While explicitly accounting for the large range of uncertain factors, this research has aimed at describing the possible outcomes of the emergence of climate change mitigation by society. This might help in conveying the consequences of the various uncertainties influencing the emergence of climate change mitigation action by society to policy makers. A policy suggestion naturally following from the presented narratives is to educate citizens on assessing the long-term future impact of climate change, since the narratives have shown this to be an important factor in determining the future state of the system. Another policy suggestion offered by the narrative is to make use of the window of opportunity for climate change policy as a result of a temporary raise in climate change awareness, for example after a climate disaster. The same applies to the period following successful climate change negotiations. That is, policy makers should exploit the momentum created by the recent Conference of Parties in Paris to steer towards collaborative climate change mitigation action.
There are plenty of opportunities for further improvement of the model and its analysis. A major area for further research relates to the incorporation of climate adaptation as an additional possible response to the model. This requires an extensive exploration regarding the interplay between climate change mitigation and adaptation response. In the current model, the assumption has been made that governments and individuals choose for mitigation once the effects of climate change become more visible. However, over time, the increasingly visible consequences of climate change might affect a change from (a demand for) more proactive behaviour into (a demand for) more reactive behaviour. In such a situation, people are not any more willing to spend their money on mitigation measures, but rather spend the same money on adaptation measures.
Second, heterogeneity in the model could be further extended. Currently, governments and citizens use a general climate change impact equation. This implies that the consequences of climate change are equal for everyone, a premise that can be easily challenged. This heterogeneity can be extended to the way in which governments and citizens would determine their response, being a mix of mitigation and adaptation measures. It might be the case that countries that are more prone to climate change induced damages have a larger preference for adaptation measures and countries that will face climate change induced damages further in the future have a larger preference for mitigation measures.
A third area for improvement of the model is related to amount of agents used in the model. In our model, society has been represented as a two-level game. However, the amount of layers that represents society could be extended by including inter-governmental organisations, multinationals, regional governments, non-governmental organisations, et cetera. Furthermore, it is essential to explore the influence of the number of agents, an issue that requires a modification to the model. Exploring the influence of the number of agents can yield interesting insights into the course of climate change negotiations and into the dynamics of top-down and bottom-up interaction.
A final area for improvement relates to the cognitive agent behaviour. Climate change response is influenced by many factors, and further research could be conducted at extending the amount of factors that are incorporated in the model. This could include low-level interaction, such as the influence of PV visibility in neighbourhoods, image, word of mouth, et cetera.
Conclusions
In this research we have conducted a scenario discovery study that enabled the identification of internally consistent and plausible narratives on the pathways of the emergence of climate change mitigation by society. A modelling approach combining agent-based modelling and exploratory modelling and analysis supported the scenario discovery approach. The various uncertainties influencing the emergence of climate change mitigation action by society have been acknowledged, identified, and their consequences have been explored. As a result, two narratives have been constructed, describing how certain sub-spaces of the uncertainty space lead to the co-evolutionary emergence of strong and weak mitigation action. That is, out of the 15 uncertain factors, only the climate sensitivity, the economic sensitivity, the time horizon of individuals and governments, and the game theoretic representation of the international negotiations, are critical in explaining the success or failure of the emergence of strong climate change mitigation.
The narratives constructed in this research are based on a model-based exploration of the consequences of uncertainties influencing the emergence of climate change mitigation action. They prove to be a succinct representation of the possible outcomes of the emergence of climate change mitigation action by society as a consequence of the large uncertainty space. The large difference between the two narratives shows the consequences of the large uncertainty space influencing the emergence of climate change mitigation action by society. The research therefore highlights the importance of uncertainties and ambiguities such as the ones related to the time horizon members of society apply in assessing the future impact of climate change, the internal mechanisms behind international negotiations on climate change and the influences of climate disasters on climate change response. Constructing narratives in such an open, objective and exploratory way can help in conveying the consequences of uncertainties influencing society’s response to climate change. This research can therefore serve as a though-provoking stimulus for analysts or decision makers in the run up to international climate change negotiations such as the recent Conference of Parties in Paris and the further policy process afterwards, by highlighting the many possible pathways of society’s response to climate change and the consequences of uncertainties.
Appendix
Here is a repository that include full data and code of the model.References
ACKERMAN, F., S. J. DeCanio, R. B. Howarth and K. Sheeran (2009) Limitations of integrated assessment models of climate change. Climatic change, 95(3-4): 297-315. [doi:10.1007/s10584-009-9570-x]
ALLEN, P. M. (1990) Why the future is not what it was: New models of evolution. Futures, 22(6): 555-570.
ATKINS, P. W., R. E. Wood and P. J. Rutgers (2002) The effects of feedback format on dynamic decision making. Organizational Behavior and Human Decision Processes, 88(2): 587-604. [doi:10.1016/S0749-5978(02)00002-X]
BALBI, S. and C. GIUPPONI (2009) Reviewing agent-based modelling of socio-ecosystems: a methodology for the analysis of climate change adaptation and sustainability. Department of Economics, University of Venice "Ca' Foscari".
BALE, C. S., L. Varga and T. J. Foxon (2015) Energy and complexity: New ways forward. Applied Energy, 138: 150-159. [doi:10.1016/j.apenergy.2014.10.057]
BANKES, S. C. (1993) Exploratory Modeling for Policy Analysis. Operations Research, 4(3): 435-449.
BANKES, S. C., W. E. Walker and J. H. Kwakkel (2013) Exploratory Modeling and Analysis. Encyclopedia of Operations Research and Management Science. S. Gass and M. C. Fu. Berlin, Germany, Springer.
BREDE, M. and B. J. M. de Vries (2013) The energy transition in a climate constrained world: regional vs. global optimization. Environmental Modelling & Software, 44: 44-61.
BREHMER, B. (1992) Dynamic decision making: Human control of complex systems. Acta psychologica, 81(3): 211-241. [doi:10.1016/0001-6918(92)90019-A]
BRYANT, B. P. and R. J. Lempert (2010) Thinking Inside the Box: a participatory computer-assisted approach to scenario discovery. Technological Forecasting and Social Change, 77: 34-49.
CHAPPIN, E. J. (2011) Simulating energy transitions. Delft University of Technology.
COP21. (2015). The 21st Session of the Conference of the Parties to the United Nations Framework Convention on Climate Change. Accessible at: http://www.cop21.gouv.fr/en.
COURTOIS, P. and T. Tazdaït (2007) Games of influence in climate change negotiations: Modelling interactions. Ecological modelling, 204(3): 301-314. [doi:10.1016/j.ecolmodel.2007.01.015]
DESSAI, S. and C. Sms (2010) Public perception of drought and climate change in southeast England. Environmental Hazards, 9(4): 340-357.
DIEHL, E. and J. D. Sterman (1995) Effects of feedback complexity on dynamic decision making. Organizational Behavior and Human Decision Processes, 62(2): 198-215. [doi:10.1006/obhd.1995.1043]
DÖRNER, D. (1996) The logic of failure: Recognizing and avoiding error in complex situations, Basic Books.
EPSTEIN, J. M. (2007). Generative Social Science: Studies in Agent-Based Computational Modeling. Princeton, NJ: Princeton University Press.
FORGÓ, F., J. Fülöp and M. Prill (2005) Game theoretic models for climate change negotiations. European Journal of Operational Research , 160(1): 252-267.
FRIEDMAN, J. H. and N. I. Fisher (1999) Bump hunting in high-dimensional data. Statistics and Computing, 9(2): 123-143. [doi:10.1023/A:1008894516817]
GERST, M. D., P. Wang and M. E. Borsuk (2013) Discovering plausible energy and economic futures under global change using multidimensional scenario discovery. Environmental Modelling & Software, 44: 76-86.
GERST, M. D., P. Wang, A. Roventini, G. Fagiolo, G. Dosi, R. B. Howarth and M. E. Borsuk (2013) Agent-based modeling of climate policy: An introduction to the ENGAGE multi-level model framework. Environmental Modelling & Software, 44(0): 62-75. [doi:10.1016/j.envsoft.2012.09.002]
GIUPPONI, C., M. E. Borsuk, B. J. M. de Vries and K. HaAnalytic Method for sselmann (2013) Innovative approaches to integrated global change modelling. Environmental Modelling & Software , 44(0): 1-9.
GREEVEN, S. (2015) Society's response to climate change - a scenario discovery study using agent-based modelling. MSc, Delft University of Technology.
GRIMM, V., U. Berger, D. L. DeAngelis, J. G. Polhill, J. Giske and S. F. Railsback (2010) The ODD protocol: A review and first update. Ecological Modeling, 221(23): 2760-2768.
GROVES, D. G. and R. J. Lempert (2007) A New Finding Policy-Relevant Scenarios. Global Environmental Change, 17(1 ): 73-85. [doi:10.1016/j.gloenvcha.2006.11.006]
GUIVARCH, C., J. Rozenberg and V. Schweizer (2016) The diversity of socio-economic pathways and CO2 emissions scenarios: insights from the investigation of a scenarios database. Environmental Modelling & Software , 80: 336-353.
HSU, A., A. S. Moffat, A. J. Weinfurter and J. D. Schwartz (2015) Towards a new climate diplomacy. Nature Clim. Change , 5(6): 501-503. [doi:10.1038/nclimate2594]
HUGHES, N. (2009) Using scenarios to bring about low carbon energy transitions : Lessons from transitions theory and the scenario building tradition Transition Pathways To A Low Carbon Economy, ON UK / EPSRC
HUGHES, N., N. Strachan and R. Gross (2013) The structure of uncertainty in future low carbon pathways. Energy Policy , 52: 45-54. [doi:10.1016/j.enpol.2012.04.028]
IPCC (2014) Summary for Policymakers.Climate Change 2014: Impacts, Adaptation, and Vulnerability. Part A: Global and Sectoral Aspects. Contribution of Working Group II to the Fifth Assessment Report of the Intergovernmental Panel on Climate Change. C. B. Field, V. R. Barros, D. J. Dokken et al. Cambridge, United Kingdom, and New York, NY, USA, Cambridge University Press: 1-32.
KLEINMUNTZ, B. (1992) Computers as clinicians: An update. Computers in biology and medicine, 22(4): 227-237. [doi:10.1016/0010-4825(92)90062-R]
KWAKKEL, J. H. (2012, 2014) Exploratory Modelling and Analysis Workbench. Accessible at: http://simulation.tbm.tudelft.nl/ema-workbench/contents.html.
KWAKKEL, J. H., W. L. Auping and E. Pruyt (2013) Dynamic scenario discovery under deep uncertainty: The future of copper. Technological Forecasting and Social Change, 80(4): 789-800. [doi:10.1016/j.techfore.2012.09.012]
KWAKKEL, J. H. and M. Jaxa-Rozen (2016) Improving scenario discovery for handling heterogeneous uncertainties and multinomial classified outcomes. Environmental Modelling & Software.
KWAKKEL, J. H. and E. Pruyt (2013) Exploratory Modeling and Analysis, an approach for model-based foresight under deep uncertainty. Technological Forecasting and Social Change, 80(3): 419-431. [doi:10.1016/j.techfore.2012.10.005]
LEE, J. S., T. Filatova, A. Ligmann-Zielinska, B. Hassani-Mahmooei, F. Stonedahl, I. Lorscheid, A. Voinov, G. Polhill, Z. Sun and D. C. Parker (2015) The complexities of Agent-Based Modeling output analysis. Journal of Artificial Societies and Social Simulation, 18(4) 4: https://www.jasss.org/18/4/4.html.
LEMONICK, M. D. (2012) Sandy & Other Disasters Could Hurt Climate Change Cause. Climate Central: http://www.climatecentral.org/blogs/hurricane-sandy-could-actually-hurt-the-climate-change-cause-15213/2015.
LEMPERT, R. J., D. G. Groves, S. W. Popper and S. C. Bankes (2006) A general, analytic method for generating robust strategies and narrative scenarios. Management science, 52(4): 514-528.
MARSHALL, G. (2012) Reasons Why Climate Disasters Might Not Increase Cocern About Climate Change. Climate Change Denial: http://climatedenial.org/2012/11/06/reasons-why-climate-disasters-might-not-increase-concern-about-climate-change/ 2015.
MOSS, R. H., J. A. Edmonds, K. A. Hibbard, M. R. Manning, S. K. Rose, D. P. Van Vuuren, T. R. Carter, S. Emori, M. Kainuma and T. Kram (2010) The next generation of scenarios for climate change research and assessment. Nature , 463(7282): 747-756.
NORDHAUS, W. D. (2007) A Review of the "Stern Review on the Economics of Climate Change". Journal of economic literature, XLV: 686-702. [doi:10.1257/jel.45.3.686]
OSTROM, E. (2012) "Nested externalities and polycentric institutions: must we wait for global solutions to climate change before taking actions at other scales?", Economic Theory 49(2): 353-369.
PFENNINGER, S., A. Hawkes and J. Keirstad (2014) Energy systems modeling for twenty-first century energy challenges. Renewable and Sustainable Energy Reviews, 33: 74-86. [doi:10.1016/j.rser.2014.02.003]
PINTO, L. G. M. and G. W. Harrison (2003) Multilateral negotiations over climate change policy. Journal of Policy Modeling, 25(9): 911-930.
PUTNAM, R. D. (1988). Diplomacy and domestic politics: the logic of two-level games. International organization, 42(03): 427-460. [doi:10.1017/S0020818300027697]
RAMMEL, C., S. Stagl and H. Wilfing (2007) Managing complex adaptive systems—a co-evolutionary perspective on natural resource management. Ecological economics, 63(1): 9-21.
ROZENBERG, J., C. Guivarch, R. Lempert and S. Hallegatte (2014) Building SSPs for climate policy analysis: a scenario elicitation methodology to map the space of possible future challenges to mitigation and adaptation. Climatic change , 122(3): 509-522. [doi:10.1007/s10584-013-0904-3]
RUDMAN, L. A., M. C. McLean and M. Bunzl (2013) When Truth Is Personally Inconvenient, Attitudes Change: The Impact of Extreme Weather on Implicit Support for Green Politicians and Explicit Climate-Change Beliefs. Psychological Science, 24(11): 2290-2296.
SASTRY, L. and D. R. Boyd (1998) Virtual environments for engineering applications. Virtual Reality, 3(4): 235-244. [doi:10.1007/BF01408704]
SMEAD, R., R. L. Sandler, P. Forber and J. Basl (2014) A bargaining game analysis of international climate negotiations. Nature Climate Change , 4(6): 442-445.
SPENCE, A., W. Poortinga, C. Butler and N. F. Pidgeon (2011) Perceptions of climate change and willingness to save energy related to flood experience. Nature Climate Change, 1(1): 46-49. [doi:10.1038/nclimate1059]
STERMAN, J. D. (1989) Modeling managerial behavior: Misperceptions of feedback in a dynamic decision making experiment. Management science, 35(3): 321-339.
STERMAN, J. D. (1994) Learning in and about complex systems. System Dynamics Review, 10(2‐3): 291-330. [doi:10.1002/sdr.4260100214]
THIELE, J. C., W. Kurth and V. Grimm (2012) Agent-Based Modelling: Tools for Linking NetLogo and R. Journal of Artificial Societies and Social Simulation, 15(3): 8: https://www.jasss.org/15/3/8.html.
TOL, R. S. J., K. J. Arrow, M. L. Cropper, C. Gollier, B. Groom, G. M. Heal, R. G. Newell, W. D. Nordhaus, R. S. Pindyck, W. A. Pizer and P. R (2013). How Should Benefits and Costs Be Discounted in an Intergenerational Context? RFF Working Paper Series.
TOMPKINS, E. L. and N. W. Adger (2005) Defining response capacity to enhance climate change policy. Environmental Science & Policy , 8(6): 562-571.
VAN DER Heijden, K. (1996). Scenarios: the Art of Strategic Conversation. Chichester, England, John Wiley & Sons.
VAN VUUREN, D. P., M. Isaac, Z. W. Kundzewicz, N. Arnell, T. Barker, P. Criqui, F. Berkhout, H. Hilderink, J. Hinkel, A. Hof, A. Kitous, T. Kram, R. Mechler and S. Scrieciu (2011) The use of scenarios as the basis for combined assessment of climate change mitigation and adaptation. Global Environmental Change, 21(2): 575-591.
VAN VUUREN, D. P., K. Riahi, R. Moss, J. Edmonds, A. Thomson, N. Nakicenovic, T. Kram, F. Berkhout, R. Swart, A. Janetos, S. K. Rose and N. Arnell (2012) A proposal for a new scenario framework to support research and assessment in different climate research communities. Global Environmental Change, 22(1): 21-35. [doi:10.1016/j.gloenvcha.2011.08.002]
WEAVER, C. P., R. J. Lempert, C. Brown, J. A. Hall, D. Revell and D. Sarewitz (2013) Improving the contribution of climate model information to decision making: the value and demands of robust decision frameworks. Wiley Interdisciplinary Reviews: Climate Change, 4(1): 39-60.
WEILER, F. (2012). "Determinants of bargaining success in the climate change negotiations." Climate Policy 12(5): 552-574. [doi:10.1080/14693062.2012.691225]
WEINKLE, J., R. Maue and R. Pielke Jr (2012) Historical global tropical cyclone landfalls. Journal of Climate, 25(13): 4729-4735.
WHITMARSH, L. (2008) Are flood victims more concerned about climate change than other people? The role of direct experience in risk perception and behavioural response. Journal of Risk Research, 11(3): 351-374. [doi:10.1080/13669870701552235]
WRIGHT, G. and G. Cairns (2011) Scenario thinking - practical approaches to the future, Palgrave Macmillan.
ZAHRAN, S., S. D. Brody, H. Grover and A. Vedlitz (2006) Climate change vulnerability and policy support. Society and Natural Resources, 19(9): 771-789. [doi:10.1080/08941920600835528]