Introduction
Insecurity and permeability of pharmaceutical supply chains constitute a major global public health threat. Over 10% of pharmaceutical medicines consumed in low/middle-income countries (LMICs) are thought to be sub-standard or falsified (SF) (WHO 2017b; Almuzaini et al. 2013; Kaur & Singh 2016; Newton et al. 2017; Nayyar et al. 2012), containing little or no active ingredient (WHO 2017a; b), with rates of over 30% reported in parts of Sub-Saharan Africa. The term ’SF’ covers different kinds of threats to medicine quality: deliberate falsification (usually profit motivated); sub-standard production with insufficient quality control; and post-manufacture deterioration (Hamilton et al. 2016). The result from the end-user perspective is the same: a poor-quality drug that will have limited therapeutic effect. Antibiotics and antimalarials are thought to be particularly vulnerable, directly causing an estimated 72,000 to 267,000 ’excess’ under-5 deaths annually (Renschler et al. 2015; WHO 2017b,a) and contributing to accelerating antimicrobial resistance through exposure of pathogens to sub-therapeutic dosages of antimicrobial agents (Yeung et al. 2015; Sharma et al. 2017; Newton et al. 2016).
Efforts to tackle the SF medicine threat have addressed both the supply side (tightening regulation, improving detection rates and prosecuting offenders) and the demand side, though education programmes aimed at health professionals and the general public (Cinnamond & Woods 2015; Hamilton et al. 2016; WHO 2017b). However, the problem persists for several reasons. On the supply side, a combination of limited regulatory resources (financial/human), endemic malpractice/corruption, and the promise of potentially huge profits, confound efforts to prevent poor-quality medicines entering supply chains Nayyar et al. (2015); Newton et al. (2014). On the demand side, high disease burdens, poverty and nadequate access to quality-assured essential medicines Vandam (2016); World Bank (2017) increase consumers’ vulnerability, especially when high-quality imitations are extremely difficult to spot, even for professionals.
This paper represents a first attempt to develop an Agent-Based Model (ABM) that represents this empirically-observed situation: a complex, self-organising and often opaque supply chain; with goods of high value but whose quality cannot easily be observed; and where formal regulatory mechanisms are limited and weak. It is relatively unusual among ABM in trying to incorporate empirically-derived observations into the model design, although this is still at a relatively early stage in our work (see later discussion).
Ethnography: The SF Medicine Threat and The Problem of Trust
In 2015-16, our research team conducted a preliminary empirical (ethnographic) study of pharmaceutical supply chain dynamics in Ghana and Tanzania, two African countries with relatively well-functioning regulatory authorities but where the problem of SF medicine penetration persists (with reported SF prevalence rates of 20-40% in Ghana (Fadeyi et al. 2015; Kaur & Singh 2016; Tivura et al. 2016; Wilson et al. 2017) and 2-12% in Tanzania (Höllein et al. 2016; Kaur & Singh 2016; Mziray et al. 2017).
Local teams based respectively at the University of Cape Coast and the Tanzanian National Institute for Medical Research worked alongside four of the authors (KH, HH, SM, GM) to conduct intensive fieldwork at a variety of ’medicine transaction points’ across pharmaceutical supply chains, ranging from retail outlets (formal and informal sectors) to wholesalers and importers. They also interviewed actors operating at different points in supply chains: those directly buying and selling medicines, as well as regulators, enforcers, professional associations, etc. Altogether, they conducted approximately 500 interviews in Ghana and Tanzania, alongside careful observations of medicine purchases in pharmacies, over-the-counter retailers and market places (Hamill et al. 2019).
Two major insights were gained from this work, which underpin our motivation to model these supply chain systems formally. First, it became clear that medicine supply chains in Ghana/Tanzania are extraordinarily complex and opaque, marked by substantial informational and economic asymmetries, with no single actor having full information about the entire system. This finding is corroborated by other empirical work on medicine supply chains in Sub-Saharan Africa, described Mackintosh et al. (2018a) as “spaghetti-like patterns of overlapping and multiplying supply chains” (see also Baxerres & Le Hesran (2011); Baxerres (2011); Mackintosh & Mujinja (2010); Mackintosh et al. (2011, 2018b); Palafox et al. (2014); Patouillard et al. (2010)). Tremblay (2013), among others, has noted the role of this complexity, within a context of weak regulation, in perpetuating SF medicine penetration. From a modelling point of view, these supply chains may thus be regarded as complex, self-organising systems, with no central controlling authority, with the implication that actions/changes at one point might generate non-intuitive effects elsewhere.
A second key finding was that where uncertainty over medicine quality is pervasive, trust underpins (at least in part) transactions and the movement of medicines over geographical and regulatory space. In order to transact or consume a medicine, whose quality cannot be directly observed, actors need to trust (the medicine, the other party) enough to proceed, given their particular exigencies and constraints. However, the economic and informational asymmetries noted above, along with differences in urgency to act, mean that some actors can afford to be more discerning while others must accept much lower thresholds of trust (Hampshire et al. 2017; Hamill et al. 2019).
Supply Chain Modelling: Existing Approaches and Their Applicability to Pharmaceutical Quality in Sub-Saharan Africa
The last couple of decades have seen several attempts to model supply chain dynamics. Most of this work has sought to represent generic supply chain systems (Garcia-Flores et al. 2000; Fox et al. 2001; Strader et al. 1998; Tykhonov et al. 2008; Jetly et al. 2012; AbuKhousa et al. 2014; Amini et al. 2012; Tran & Cohen 2004). and has rarely dealt specifically with issues of product quality. An exception has been some of the work modelling food supply and safety (McPhee-Knowles 2015; Buurma et al. 2017) However, the assumptions underpinning McPhee-Knowles’ model - that food contamination occurs randomly across sites rather than following particular suppliers - undermines her assertion that her work models supply chains and thus renders it incompatible with our approach in which poor-quality medicines penetrate supply chains through specific actors (manufacturers, suppliers and retailers), while Buurma et al’s work deals more with opinion change than actual shifts in food quality.
A second limitation of existing work is that the assumptions underpinning most models are derived from the experience of (relatively) well-regulated systems in the Global North, where accurate information is more widely available and the penalties for legal infringements may be severe. For example, key elements in the model of Jetly et al. (2012) are drug innovation and company mergers - more relevant for US markets than those in Ghana or Tanzania. In the African context (particularly in more remote areas), transport difficulties and costs represent a major impediment to effective healthcare delivery. This applies particularly to patients (or consumers of medicines) but also to wholesalers and retailers. Delivery failures and failures to maintain the storage conditions necessary for preservation of drug efficacy are widely recognised features of African healthcare connected with transportation challenges (Khojah et al. 2013; Umlauf & Park 2018). Models that independently represent physical space and networks are generally rare in ABM.
Thirdly, the vast majority of ABM on supply chains are based on assumptions that the supply chain is either controlled by a single actor or that each element is at best quasi-autonomous, (e.g. Petrovic et al. (1998); Towill et al. (1992); Tzafestas & Kapsiotis (1994); Strader et al. (1998); Swaminathan et al. (1998)). Our observations and the other empirical work discussed above suggests that such assumptions cannot be made about pharmaceutical supply chains in Africa, where each element is not only autonomous but often competitive, and regulation of the system as a whole is rarely sufficient to ensure global control.
By necessity, our work is thus more in the tradition of the "self-organising" complex systems approach (Choi et al. 2001; Geyer & Cairney 2015), for which ABM is particularly well suited. Non-trivial empirical effects emerging from self-organising structure and dynamics have been investigated in other situations. For example, Mitchell & Ackland (2007, 2009) demonstrate “boom and bust” time variation with a consumer- supplier model, but rarely has this been applied to drug supply. The work of Jetly et al. (2012) on the US pharmaceutical market is an important exception but, as noted above, the processes they describe are not directly translatable to the African context; they use three levels of suppliers but no consumers, and deal predominantly with drug innovation and company mergers. Issues of quality, trust and inspection are also absent from these models.
Finally, there have been several attempts in ABM to model trust within supply chains and networks (Wang & Vassileva 2003; Sutcliffe & Wang 2012; Chen et al. 2015). Tykhonov et al. (2008) present a model with empirical referents, but they intend their ABM not to represent reality directly but rather the operations of pre-existing gaming simulations like the “Trust and Tracing Game”. This is also the approach taken by Kimbrough et al. (2002) who models the well-known “Beer Game” (Sterman 1989). While this is an innovative way to use ABM and access a different kind of data, the danger is that the game does not itself represent reality and, therefore, an ABM reproducing its behaviour will not do so either. This is a variant on the well-known challenge of ecological validity (Chaytor & Schmitter-Edgecombe 2003) in experiments.
In summary, we have not been able to identify any previous ABM that adequately capture the empirically- observed realities of pharmaceutical supply chains in Africa, where formal regulation is weak, quality uncertain, and where no single actor has full oversight. In contrast to more abstract approaches, our ambition is to develop a model that describes with reasonable accuracy the empirical situation in countries like Ghana or Tanzania, rather than being instrumental (solving a technical problem like supply chain optimisation) or serving as a software tool.
Although we are still at an early stage in terms of calibration and validation, this work represents a first step towards what Gilbert & Troitzsch (2005) refer to as modelling a well-defined “target-system”. It is distinct from more abstract models of phenomena like trust in networks because the different roles of customers, wholesalers and retailers are already explicit in the model. It also represents supply chains and their self-organisation explicitly and, albeit in a stylised way, incorporates features that are relevant to the African context (like travel costs) while excluding others (like innovation and mergers) which are not. While the models described above have represented various permutations of trust, quality, inspection, competing actors and self-organising supply chains, the model presented below is the first ABM to integrate all of these elements because of their relevance to our target system.
Model Design Strategy
This is a first attempt to build a model of a self-organising supply chain involving the need for trust because of the possibility of variable drug quality and allowing for some inspection. It is chiefly designed to be simple and support understanding of what is almost certainly a complex (counter-intuitive) system in its more detailed variants. However, it is also intended to analyse a “self-organising” system for which effective policy intervention is likely to be particularly challenging.
In designing the model, we had to balance several principles. Generally we tried to keep the model as simple as possible, particularly where relevant data were lacking. However, we also ensured that the assumptions in the model broadly followed - or at least did not contradict - our empirical observations or other published work on pharmaceutical supply chains in Africa (see above). Sometimes there were tensions between the two. For example, our ethnographic work suggested that social learning or ’gossip’ may play a role in decision-making, as people share information and experiences of trying different medicines/sellers (Hampshire & Owusu 2013; Hampshire et al. 2017) However, in this case (and some others) we deliberately simplified the model, partly because of a lack of readily quantifiable data but also because we wanted to start by developing an effective understanding of a simpler model. (In fact, no other research we have so far discovered recognises social learning in the operation of trust in supply chains so this is both an obvious empirical and conceptual development for later versions of the model.)
Our model represents three kinds of actors: those who sell only (wholesalers); those who both buy and sell (retailers), and those that buy only (consumers). The challenge faced by the buyers is to determine which of several sellers offers the best value, where some factors (e.g. price) are visible and others (drug quality) must be inferred. However, for a buyer, the number of potential suppliers is larger than can be tested even once. This means that the model cannot use standard regret minimising algorithms for example (Loomes & Sugden 1982; Auer 2002; Bubeck & Cesa-Bianchi 2012; Wang et al. 2009).
An important ingredient of the model is that all the actors are non-corrupt and therefore seek to weed out any low quality drugs. We do not propose this to be true; rather this simplifying assumption is made provisionally to allow us to investigate whether there are system-level issues which can partially explain the empirically-observed persistence of SF drugs.
Characterisation of Actors
The dynamics of the system involve a number of actors in three categories: wholesalers, retailers and consumers. Interaction occurs in discrete rounds in which each consumer makes a purchase. Few of the system processes and parameters are known for certain by the actors. Sellers (wholesalers and retailers) may, or may not, have good quality products and may change their pricing strategy. Good quality drugs will not always produce a satisfactory outcome, while poor-quality ones may still appear to have beneficial effects (through placebo effects or simply getting better anyway). Where such uncertainty exists, we model it stochastically, using random variables \(\eta\) between 0 and 1, such that \(p_j=1+\eta\) means that \(p_j\) is set to a random value between 1 and 2.
Wholesalers (labelled k)
Wholesalers procure large quantities of medicines from domestic and international manufacturers. The drugs they source have a quality \(q_k=\eta\)[1]. Wholesalers also charge a price, given by their own cost (taken as 1) plus a markup \(p_k = 1+\eta\). The details of the production process itself (costs of raw materials, production technologies and so on) are considered outside the model, and the wholesalers neither know nor check the quality of drugs they source. A wholesaler maintains a stock of drugs and cash which is augmented by sales and decremented by costs. This stock acts as a hard constraint on what the wholesaler can sell. We simplify the model by assuming there are no loans, deferred payment arrangements and so on although we know in practice that this is often not the case. The bank account of new wholesalers is “primed” by initial capital that allows the wholesaler to launch their business and determines how long they can continue without selling anything. The stock, bank account and quality of a wholesaler are not visible to other actors.
The wholesalers retain their price and quality after any round in which they make a profit. If their strategy does not lead to a sale, it is assumed that they have no information about why it failed. On this basis they generate a new strategy (\(q_k\) and \(p_k\)) at random. Old stock is retained, and when new stock is bought the quality of the stock becomes a weighted average of old and new batches. Wholesalers can go bankrupt if they run out of cash and stock. It is assumed that if a retailer puts in a request for stock, this can always be met within a simulated time period, provided the wholesaler has sufficient cash.
Retailers (labelled j)
Retailers buy the drugs from wholesalers and then sell them on to consumers. They hold a stock of drugs (\(I_j\)) which is updated each round by purchasing from their preferred wholesaler. This stock has a mean quality \(Ql_j\), which is again a weighted average of the drugs bought and currently unsold. The retailer does not know the value of \(Ql_i\) and therefore cannot use it to decide a pricing strategy. Retailers have a selling price \(p_j\) and will sell to any consumer until their stock is exhausted. They have a physical location which gives them a customer base determined by the maximum distance consumers can travel and the maximum price they can pay. For simplicity, in the current version of the model we do not want to deal with the logic of ``diversification'' so the retailer will buy only from their preferred wholesaler. They can and will sell to anyone who is interested at ``their'' price \(p_j\). They start with an initial stock with quality of \(q_k=\eta\) and a price \(p_k=q_k+\eta\).
Retailers can also inspect and attempt to evaluate the quality of the drugs which enables them to establish trust with the wholesaler \(T^W_{jk}\). Initially the trust is set to 0 between each wholesaler and retailer. On receiving a batch of drugs this trust value is incremented by the result of the inspection (\(q_k+\eta -0.5\)) which depends on actual quality and a random number representing the unreliability of inspection. Thus, on average, wholesalers with quality greater than 0.5 will enjoy enhanced trust and vice versa. At present retailers do not share the outcome of the inspection with any other actor, nor learn about the medical outcomes for their consumers, so trust regarding quality is based only on their own previous experience. Each retailer has a purchasing strategy \(s_j\) which determines the relative importance of the price and perceived quality of a retailer \(j\).
Consumers (labelled i)
Consumers purchase one dose of the drug per round. The success or failure of the medicine is determined before the next round. Consumers can access drugs from any retailer but they are spatially dispersed and there is a cost associated with travel (\(d_{ij}\)). The consumer \(i\) uses this information to establish a level of trust with the retailer \(j\) (formally written as a kernel \(T^C_{ij}\)), trust increasing if the drug is successful and reducing if it is not (Jonker & Treur 1999). Consumers do not have any direct information about the wholesaler supplying their retailer. For each retailer, the consumer has a level of trust initially set to 0 and updated each time a drug is used (based on the outcome). Consequently, trust in retailers with drug quality above \(0.5\) will rise and it will fall for those with lower quality.
In the simplest version of the model consumers do not communicate with each other: In this simplified model, there is no “gossip” so the possibility of establishing drug quality is based only on the consumer’s direct experience. It turns out in analysing the simulation that this trust kernel is the major determinant of system behaviour and, for this reason, we will examine different updating schemes in the characterisation of the actors.
We assume that over the length of the simulation run the population of consumers (and their locations) are fixed. Consumers have a preference for low prices and can pay an absolute maximum price of 2 units, reflecting the very real resource constraints faced by patients in these settings. The price and location of retailers is known to all consumers but their stock, strategy and wholesaler is unknown.
Individual Dynamics
Geography
We assume there are N\(_k\) wholesalers, N\(_j\) retailers and N\(_i\) consumers. Transport costs are evaluated by assuming that retailers and consumers are equally spaced around a ring (with the cost to travel to a nearest neighbour set at \(d_0\)). Mathematically, this means that with one retailer per \(N_i/N_j\) consumers the separation between retailer \(j\) and consumer \(i\) is
$$d_{ij}= d_0 Min\left (|i-(N_i/N_j)j|, |N_i-i+(N_i/N_j)j|\right )$$ |
Money
Each consumer selects a preferred retailer based on price, distance and trust. Each retailer selects a preferred wholesaler based on price and trust as described below. Money of up to 2 units per transaction enters the system via the consumers. The money then flows through retailers and is lost from the system as wholesaler costs.
Wholesalers
In any given round, the wholesalers can use any cash available from the previous round for further imports based on their current quality/cost. Wholesalers must also pay some overhead each round regardless of whether they trade or not. If the overhead reduces a wholesaler to negative stock, they go bankrupt. Bankrupt wholesalers are replaced in the next round with a new wholesaler, for whom all strategies are reinitialised in the standard way and all trust levels are reset to zero. Consequently, the total number of wholesalers is assumed to be fixed.
Retailers
In each round, retailers sell their product reactively to those consumers who choose them. They receive money according to how much they sell and the price they sell at. They then invest this money in further stock for the next round using their preferred wholesaler which is the one offering best value according to
$$v_{jk} = -p_k + s_jT^W_{jk}$$ |
Although it would be realistic for retailers to hold both cash and stock, this is an unnecessary complication within the basic model that does not affect overall behaviour. Thus, at the end of each round all a retailer’s wealth is held as stock \(I_j\), some of which is then removed as overhead.
If the retailer fails to make a sale in any round, it revises both its strategy \(s_j\) and its price \(p_j\), changing their values by a small random amount between \(\pm\epsilon\). If the overhead causes the stock to become negative, the retailer is deemed bankrupt and replaced with a new retailer. New values of \(p_j\) and \(s_j\) are generated and all trust information, both in wholesalers (\(T^W\)) and consumers (\(T^C\)) is reset to zero.
Consumers
In each round every consumer evaluates every retailer with a value score given by
$$v_{ij} = -p_j - d_{ij} + s_i T^C_{ij}$$ |
The consumer buys drugs from the retailer offering best value, provided they have stock and the price is below 2 units. The drug is then used and its success assigned a value of \(q_j-\eta\) where \(\eta\) is a random number between 0 and 1, and the trust level of the chosen retailer \(T^C_{ij}\) updated accordingly. In the simplest model, consumers all have the same weighting for the importance of the three factors.
The structure of the model is shown schematically in Figure 1 with interactions summarised in Table 1.
The model incorporates the capacity for evolution via price changes and bankruptcies. It also develops a “memory” via the trust kernels \(T^C\) and \(T^W\). The spatial separation of consumers and regeneration of bankrupt suppliers makes it difficult for a supply-side monopoly to form while the existence of numerous consumers precludes a demand-side monopoly.
Although the model is deliberately simplified, its aim is to be at least compatible with empirical knowledge. It is also designed so that certain obvious extensions do not require it to be totally re-engineered. For example, it would be very easy to “turn off” the effectiveness of drugs that spent more than a certain length of time in the supply chain or to add external monitoring and “punishment” (fines or seizure that changed costs or led to supplier closure). Consumers could be given different levels of wealth, perhaps reflected by different weightings for trust and price. The bankruptcy-startup cycle could allow evolving franchises or group level learning, where the new supplier could copy a successful supplier’s strategy and perhaps inherit its trust network. Horizontal communication (“gossip”) could allow one consumer to affect another’s trust network. The list goes on but the purpose of this article is to determine critical drivers in the simplest model as a baseline for future research.
Results and Analysis
Qualitative definition of an optimising state: Nash equilibrium
Complex evolving systems can self-organise into an equilibrium steady state under which no actor can improve their situation by changing supplier (Nash 1951; Taylor & Jonker 1978; Von Neumann & Morgenstern 2007). Such so-called ”Nash Equilibria” (NE) can be determined using Game Theory with an additional assumption that all actors have perfect information. This is not the case in our model, where actors’ information about drug quality comes only through imperfect tests and trust kernels.
In this subsection, we temporarily assume that evolutionary learning works well enough that everyone has perfect information. Under this assumption, it is possible to determine the NE analytically. To do so, we divide the system variables into two groups. The first group (comprising network, prices and quality) defines the supply chain. The second group (comprising strategies and trust kernels) is the information which drives system evolution.
Considering suppliers from a game-theoretic perspective, we identify the payoff functions as “largest profit” for suppliers, and as lowest price plus highest quality for consumers. The absence of any differential transportation cost or price-quality linkage in the wholesaler-retailer game makes competition purely price-driven Bertrand (1883): Any price rise is liable to be undercut by another wholesaler until the point where a further price cut (below costs) leads to bankruptcy, so the wholesaler markup \(p_k-q_k\) is driven to zero[2]. The consumers prefer higher quality, which applies an evolutionary pressure on the retailers and thus indirectly on the wholesalers. Since the sellers’ markup is always driven to zero by the Bertrand competition, there is no profit motive for lowering quality, so the game-theoretic equilibrium quality \(q=1\).
The retailer-consumer game is more complicated. The pressure from consumers for high quality drugs and the indifference (at worst) of the retailer again drive the equilibrium to top quality \(q=1\). The transportation costs ensure many retailers can co-exist. If the retailers could charge different prices to different customers then Bertrand competition would drive the markup towards being equal to the differential transport costs \(p_{ji} =q_j+(d_{ij+1}-d_{ij})\) where \(d_{ij+1}\) is the distance to the second closest retailer. However, we assumed uniform pricing. To analyse this situation in general, we define \(n=N_i/N_j\), the number of consumers per retailer. We consider a situation where all retailers have the same price \(p\) and quality \(q\). Their income is then \(pn\). To attract an additional buyer, a retailer would have to lower the price by \(d_o/2\) to compensate for the extra travel cost[3]. Their new income will be \((p-d_0/2)(n+1)\) and this process will be favourable until
$$pn= (p-d_0/2)(n+1)$$ |
Solving for \(p\) gives the equilibrium price to the consumer of \(p=(n+1)d_0/2\). If this is higher than 2, the price will saturate at 2.
This NE involving high quality drugs and profitable retailers would be achieved in the case of perfect information i.e. if the trust kernel fully represented the quality of the drugs. We will investigate by simulation whether our system of agents reasoning on imperfect information can nonetheless approach this “Optimising” state.
The model was coded in under 100 lines of Fortran, available as open data from the corresponding author.
It is fairly straightforward to characterize possible outcomes from the model which appeared compatible with our ethnographic observations.
Some are trivial. For example, if distances \(d_{ij}\) are too large, each consumer will access only their nearest retailer: a situation that pertains in many parts of rural Africa where healthcare facilities are sparse and public-sector stock-outs common such that, in practice, a single local retailer is the only available source of medicines (Hampshire et al. 2011, 2016; Mackintosh et al. 2018b) Similarly, behaviour in the initial rounds of the system is very sensitive to the exact initial conditions: since these are chosen randomly, nothing specific can be learned before the system has time to evolve. In general, the long-term limit of an evolving system, based on the assumptions we made, involves three distinct types of end state:
- “Dynamic”: New wholesalers and retailers are continuously introduced. Buyers keep swapping suppliers. There are large fluctuations and no long-term trend in average quality and price.
- “Frozen”: The supply chain is highly dependent on the initial conditions. Retailers and wholesalers who gain market share early retain a dominant position.
- ”Optimising”: The supply chain reaches a stable state which is invadable by new wholesalers or retailers with “better” strategies and proceeds towards an Evolutionarily Stable State independent of initial conditions.
Consumer | Retailer | Wholesaler | |
Price | buys < 2 | sells @ \(p_j\) | sells @ \(p_k\) |
Location | i | \(10_j\) | - |
Trust in | Retailer \(T^C_{ij}\) | Wholesaler \(T^W_{jk}\) | |
Trust based on | recovery | (imperfect) testing | - |
Importance of trust | \(s_i\) | \(s_j\) | - |
Connect to | Retailer | Consumer, Wholesaler | Retailer |
Buy information | Trust, price, location | Trust, price | - |
Strategy change | - | bankruptcy | bankruptcy |
Inventory drug quality | \(q_j\) | \(q_k\) |
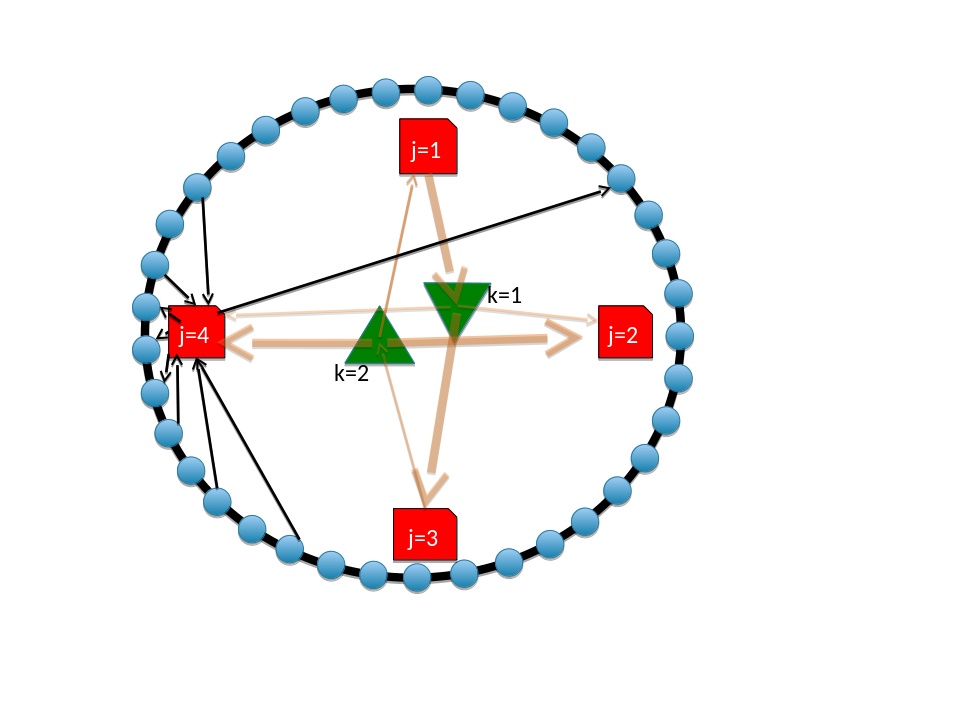
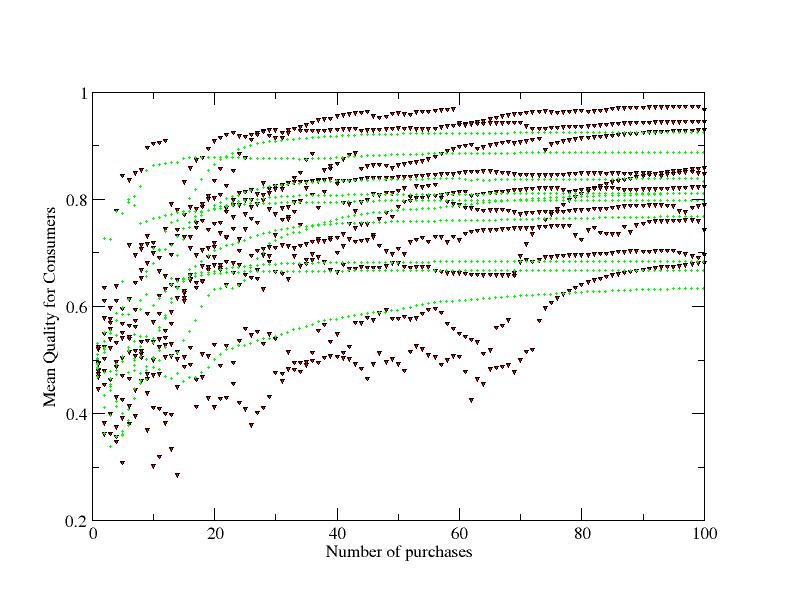
The possibility of an “Optimising” outcome arises from the existence of an Evolutionarily Stable State which the system will tend toward. This is the theoretical Nash Equilibrium.
We begin by comparing two different models for the evolution of the trust kernel based on actors’ experiences (Jonker & Treur 1999) to explore the robustness of the wider results.
In the first, shown with green circles in Figure 2, trust at round n is incremented cumulatively according to
$$T_n=T_{n-1}+q+\eta-\frac{1}{2}$$ |
This model generates the “frozen” state with suppliers charging low prices and \(q>0.5\) becoming dominant. Thus although the supply chain is self-organised to provide drugs of above average quality, once frozen it is impossible for a supplier of better quality drugs to break in. After some examination of parameter sensitivity in the model, we identified the unbounded trust function as the cause. Initially, when no trust has been established, price and distance are the main determinants of system behaviour. High priced retailers fail to make a sale and the high price strategy is eliminated. If a buyer chooses a retailer with quality below \(q = 0.5\), after several visits their trust will drop and the buyer will switch supplier. Thus, low quality drugs are eliminated. However, any drug offered which is better than \(q = 0.5\) tends to increase trust in that retailer. Since the retailer and associated wholesaler are both making sales, they each retain their strategies. Since the consumer never goes elsewhere, trust in other retailers remains at 0.5 even if they in fact have better quality. So a positive feedback process locks the consumer into a suboptimal arrangement. The suppliers with the highest quality drugs may never get to make the initial sale which enables them to establish trust.
In the second model, shown with red circles in Figure 2, the trust kernel is an estimate of how good the drug is. Mathematically it is a running average of how well the drug performs:
$$T_n= \frac{mT_{n-i} + q+\eta-\frac{1}{2}}{m+1}$$ |
Figure 2 displays a representative sample of ten runs with each trust model, showing how the mean quality of the drugs reaching consumers is increased as the trust kernels are established. There is considerable scatter in the outcomes but several common features can be discerned. In the first 20 iterations, there is a strong increase in mean quality. The green “cumulative” method gives a slightly faster quality improvement on average. At longer times, the quality using the “cumulative” method becomes frozen in[4]. By contrast, with the (red) bounded trust model it is possible for higher quality drugs to break into the market even very late in a run, especially when the overall quality is low. The graph shows a “punctuated equilibrium” dynamics where the system appears stable for a time but after a bankruptcy it is then invaded by a new drug. The bankruptcy eliminates a failing strategy, and if the new entrant also has a bad strategy they will also be immediately eliminated. Figure 2 shows that new strategies which become established are usually of higher quality, though sometimes a low price can compensate.
The trust kernel operates in two places, between consumers and retailers and between retailers and wholesalers. We investigated the relative importance of these. Figure 4 shows that trust in the retailer- wholesaler interaction is much more effective in eliminating SF than in the retailer-consumer one. The upper four curves in the figure also show that price to the consumer drops over time as a result of the price competition built into the model. Competitive price reductions are slower when trust in quality is given more weight in decision making. More interestingly, if retailers ignore quality they are more effective in driving down prices than consumers can be.
Figure 2 shows considerable variation between runs which implies the uncertainty involved in any empirical observation. A more robust description of the model is given by statistical averages over many such runs (Figure 3). Here we see the unrelenting effect of including flexible learning and trust in driving the system to supply higher quality drugs (coloured curves) compared with purely price driven or overly trusting strategies (black solid and dotted lines). This slow increase of average quality arises from rare but rapid changes in individual runs.
A fit to the data suggests that, in the very long term, the system with the flexible learning kernel tends toward the Nash Equilibrium (\(p=q=1\)). Although consumers do not have anything like the complete information assumed for the analytic derivation of the Nash Equilibrium, the system overall (including its capacity to self-organise) has “learnt” enough information to achieve it.
Three final states
We characterise the outcome of the model in terms of three final states. Which state is obtained depends strongly on how “value” and “trust” are determined, and weakly on the system size and initial conditions. Nevertheless, we can summarise the general rules.
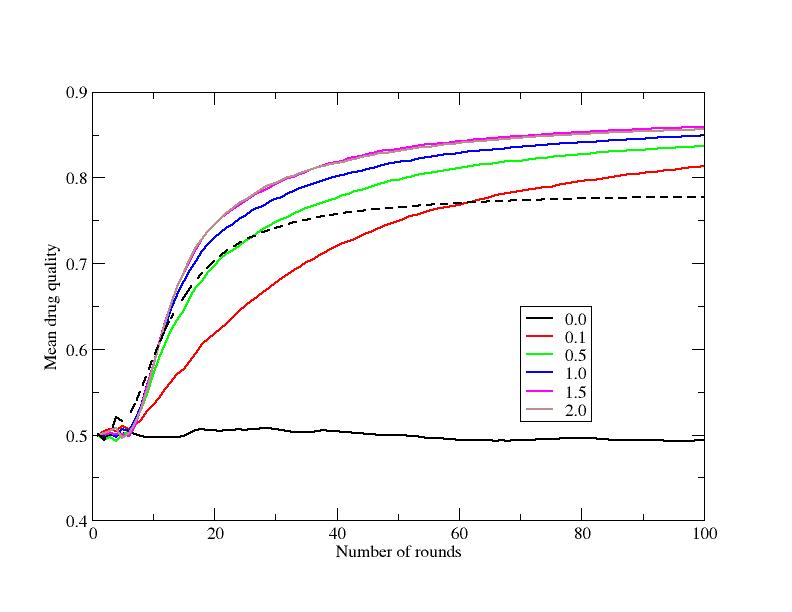
Frozen state
A ’frozen’ state occurs when trust is the dominant factor in value judgement, and is achieved too quickly by any above-average products. The “cumulative” trust kernel tends to lead to this state. The trust kernels continue to change, but the buying decisions do not. New retailers appear in the system, but are unable to attract customers and quickly become bankrupt. The system’s failure to “learn” arises from the lack of information, due to consumers not investigating enough different retailers. In this state, the system provides drugs of mediocre quality at low prices and does not improve over time. We observed this situation during our fieldwork in more remote rural areas, where a small number of suppliers operate and survive in business for long periods (Hamill et al. 2019).
Dynamic state
The system always passes through a dynamic state, in which new suppliers are investigated and the trust kernels are established. The dynamic state is permanent when the weight of the trust kernel is zero or testing is unreliable. In the dynamic state, a number of processes are occuring: buyers change suppliers, new retailers appear and may become established. Quality tends to improve over time, but progressively more slowly. Typically, there are two timescales: the time between wholesalers introducing a new, better drug and the time it takes for retailers of that drug to gain increased trust and custom. At long times, improvements in the quality of the “best drug on the system” are small, and the system’s failure to reach an optimal state arises from the consumers not acting strongly enough on the information available.
In our fieldwork, this state was most closely represented by the mass of informal traders operating in Accra’s (in)famous ’Drug Lane’ (Okaishie), where there is a rapid turnover of traders and products. In model terms the equalization of transportation costs of co-located retailers increases competition based on price and quality.
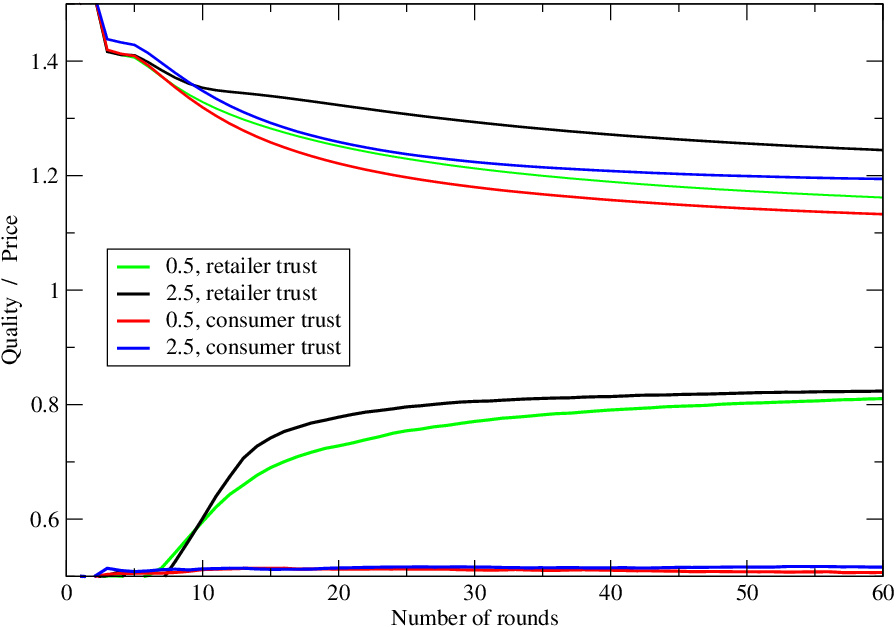
Optimising state
This is defined as the Evolutionary Stable State in the simulation which is equivalent to the Nash Equilibrium of analytic theory. The optimised state delivers the lowest possible prices and highest possible quality. This idealised state was never observed in a simulation, but for intermediate levels of trust, the dynamic state evolves towards it. However, the closer it gets, the slower the rate of improvement. This state most closely resembles western pharmacies, where diligent regulation leads to strong and accurate levels of trust.
Discussion and Conclusions
We designed a simple model for a self-organising supply chain for pharmaceutical drugs which attempted to balance simplicity, existing modelling ideas and compatibility with ethnographic fieldwork in Ghana and Tanzania. The system is characterised by incomplete information at all levels. It offers the possibility for buyers to discover the quality of the drugs supplied by sellers based on inspections or medical outcomes, both of which are subject to stochastic error.
We identified three possible states of the system, “dynamic”, “frozen” and “optimising”. With no learning/trust possible, the “dynamic” state occurred with retailers and consumers continually changing suppliers. The variant involving the “cumulative” trust model had a dynamic phase during which quality improved but eventually the supply chain became frozen. The bounded trust model remained in a dynamic state, evolving slowly towards the Nash Equilibrium, both in terms of high quality and low price.
We have found that introducing the ability to learn from experience and establish a trust network does serve to eliminate low quality drugs from the supply chain. Unfortunately, too strong a dependence on trust also entrenches the supply of mediocre drugs making it impossible for better quality products to become established. Different assumptions for changing the supply chain, pricing, seller strategies and so on were of secondary importance to the trust model. The model assumed that consumers were price sensitive but not resource limited so increasing personal wealth is not in a position to unfreeze the system.
Counter-intuitively, to get a better outcome, some way is needed to make consumers “take a chance” on drugs of which they have no personal experience, eschewing those that have already earned their trust. Intriguingly, parallel analysis of our ethnographic research points to a similar conclusion. In a context of high uncertainty and high "stakes" (i.e. potentially severe negative consequences of making a wrong decision), many of the "consumers" we interviewed sought to develop long-term relationships of trust with particular medicine retailers (retailers did the same with their suppliers). Our empirical work suggests that, once such a relationship had been established, there were very strong social incentives not to challenge it, even to the extent of deliberately overlooking possible indicators that cast doubt on the trustworthiness of the other party. These socially-embedded practices can underpin community cohesion, but may have the unfortunate consequence of entrenching possibly untrustworthy suppliers and medicines within supply chains (Hamill et al. 2019).
Within the model, trust established by retailer testing was more effective than that established by consumer experience. This is so despite the quantitative effectiveness of the evaluation being the same. We can understand this because the model assumes that SF medicines enter at the wholesaler level: the retailer inspection impacts directly on choice of wholesaler while consumer pressure acts only indirectly by eliminating retailers who made a poor wholesaler choice. (A caveat: if the SF drug is introduced lower in the supply chain then supplier testing cannot prevent it.) Price competition at any level of the chain has the effect of reducing prices.
Model outcomes and implications
Having built the minimal model, we are now in a much better position to understand the potential impact of some of the simplifying assumptions we made. For example, in neglecting direct peer-to-peer communication (“gossip”), partly for reasons of simplicity and partly through a lack of readily-quantifiable data, we have set aside exactly the sort of mechanism that might allow consumers to access data on retailers they have not tried without having to give up what they already trust. A model where the “trust kernel” involved public rather than private information might enable buyers to obtain information about other suppliers from their neighbours. Such developments to the model should proceed in tandem with focused fieldwork to ensure that the inputs are empirically grounded.
Another possibility for making the model more realistic is that suppliers could choose to make information public e.g. by advertising. This does happen to a large extent across Sub-Saharan Africa (Hampshire et al. 2017). For example, branded drugs are a way for retailers to reveal their wholesaler’s quality. Commercial campaigns allow suppliers to signal their quality. However, this opens up another trust network beyond what we consider here, since the consumer has to believe not only that the advertisement is honest, but also the supply chain has integrity. In addition, for falsified drugs at least, deliberate attempts are also made to fake packaging, tamper with expiry dates and so on, thus partially undermining the trust that may be available from strategies such as branding (Hampshire et al. 2017; Hamill et al. 2019).
A third (empirically observed) way of potentially establishing trust is via signalling of success. In this case the profitable retailer demonstrates their success with lavish premises, expensive vehicles, etc., while unprofitable retailers are unable to do so. This allows indirect communication involving peers since their purchasing choices are effectively reflected in the retailer’s premises. However, as with any ’Signalling Game’, effective interpretation of the signal depends on knowledge of the specific socio-cultural context. Ostentatious displays of wealth or success in different contexts may signal rather different things: competence, perhaps, but also selfishness or even corruption (Gambetta & Hamill 2005; Hamill 2011; Hampshire et al. 2017).
A fourth possibility is rigorous inspection with publicly available results, both regarding the veracity of advertising claims and the fidelity of the supply chain. If consumers replace trust based on narrow personal experience with such wider information sources, then the “frozen” state monopoly of mediocre drugs could be broken. However, we know empirically that resources constraints (human, financial and technical) make rigorous inspection very difficult to achieve in many LMIC contexts, despite significant investment by WHO and others (WHO 2017b, a). Moreover, this strategy is fraught with the risk of reaching the “random” final state rather than the “optimal” one if the supply chain is not, in fact, reliable. (This is a good illustration of the problem with policy interventions in complex systems.) Even the most accurate supplier information is irrelevant if the supply chain lacks integrity. This could be modelled relatively easily by introducing a stochastic degradation of drug quality at each step of the chain, thus unifying the treatment of substandard and falsified drugs as is necessary in reality.
Any or all of these factors could be included in an enhanced version of the model and preliminary testing suggests that they could lead to an optimising system. But each additional element introduces a swathe of currently unknown parameters. Rather than layering guesswork upon guesswork, for the present, we have chosen to leave the model available for future use once those parameters are better understood.
Perhaps the most important result of the model is the demonstration of how the role of trust, at the individual level, has massive effects at the system level. This is a connection which has not been strongly made in previous work, and illustrates the need for more detailed ethnographic study of how trust in suppliers is built, and how it affects decision-making.
In summary, we introduced a minimalist but ethnographically inspired model for a self-organising pharmaceutical supply chain with trust, variable quality, inspection and geographical aspects. We determined three types of possible end state. The worst is the “random” outcome where supplies and reliability are always changing. Next is a “frozen” outcome where suboptimal products achieve an unbreakable hold. Finally, there is a more desirable “dynamic optimising” outcome which is stable but does allow successive invasions of higher quality drugs. We identified that trust, built on imperfect information, is the critical feature driving system-level behaviour to one of these three outcomes which vary significantly in social value.
Notes
- The eventual success of the drug is on a scale from 0-2, being the sum of \(q_k\) and another random number \(\eta\) accounting for uncertainties including placebo effects and the fact that no drug works for everyone.
- This second feature is built into the strategy rather than found by evolution.
- In this model, the distances are \(d_0/4\), \(3d_0/4\), \(5d_0/4\) ... \((2n+1)d_0/4\)
- With the exception of the lowest curve which was dominated at the early stages by cheap drugs of below average quality. Over time, however, trust in these was slowly lost and consumers switched to better quality drugs.
References
ABUKHOUSA, E., Al-Jaroodi, J., Lazarova-Molnar, S. & Mohamed, N. (2014). Simulation and modeling efforts to support decision making in healthcare supply chain management. The Scientific World Journal, 2014, 354246. [doi:10.1155/2014/354246]
ALMUZAINI, T., Choonara, I. & Sammons, H. (2013). Substandard and counterfeit medicines: a systematic review of the literature. BMJ OPEN, 3(8), e002923.
AMINI, M., Wakolbinger, T., Racer, M. & Nejad, M. G. (2012). Alternative supply chain production– sales policies for new product diffusion: An agent-based modeling and simulation approach. European Journal of Operational Research, 216(2), 301–311. [doi:10.1016/j.ejor.2011.07.040]
AUER, P. (2002). Using confidence bounds for exploitation-exploration trade-offs. Journal of Machine Learning Research, 3(Nov), 397–422.
BAXERRES, C. (2011). Local/global articulations and the high use of pharmaceuticals in Cotonou, Benin. Medische Antropologie, 23(2), 287–297.
BAXERRES, C. & Le Hesran, J.-Y. (2011). Where do pharmaceuticals on the market originate? An analysis of the informal drug supply in Cotonou, Benin. Social Science & Medicine, 73(8), 1249–1256
BERTRAND, J. (1883). Théorie mathématique de la richesse sociale. Journal des Savants, 67(1883), 499-508.
BUBECK, S., & Cesa-Bianchi, N. (2012). Regret analysis of stochastic and nonstochastic multi-armed bandit problems. Foundations and Trends® in Machine Learning, 5(1), 1-122.
BUURMA, J., Hennen, W. & Verwaart, T. (2017). How social unrest started innovations in a food supply chain. Journal of Artificial Societies and Social Simulation, 20(1), 8: https://www.jasss.org/20/1/8.html. [doi:10.18564/jasss.3350]
CHAYTOR, N. & Schmitter-Edgecombe, M. (2003). The ecological validity of neuropsychological tests: A review of the literature on everyday cognitive skills. Neuropsychology Review, 13(4), 181–197.
CHEN, S. H., Chie, B. T. & Zhang, T. (2015). Network-based trust games: An agent-based model. Journal of Artificial Societies and Social Simulation, 18(3), 5: https://www.jasss.org/18/3/5.html. [doi:10.18564/jasss.2767]
CHOI, T. Y., Dooley, K. J. & Rungtusanatham, M. (2001). Supply networks and complex adaptive systems: control versus emergence. Journal of Operations Management, 19(3), 351–366
CINNAMOND, M. & Woods, T. (2015). The joint interagency task force and the global steering committee for the quality assurance of health products: Two new and proactive approaches promoting access to safe and effective medicines. The American Journal of Tropical Medicine and Hygiene, 92(6_Suppl), 133–136. [doi:10.4269/ajtmh.15-0208]
FADEYI, I., Lalani, M., Mailk, N., Van Wyk, A., & Kaur, H. (2015). Quality of the antibiotics—amoxicillin and co-trimoxazole from Ghana, Nigeria and the United Kingdom. The American Journal of Tropical Medicine and Hygiene, 92(6_Suppl), 87-94.
FOX, M. S., Barbuceanu, M. & Teigen, R. (2001). 'Agent-oriented supply-chain management.' In M. J. Shaw (Ed.), Information-Based Manufacturing, Berlin: Springer, pp. 81–104. [doi:10.1007/978-1-4615-1599-9_5]
GAMBETTA, D. & Hamill, H. (2005). Streetwise: How Taxi Drivers Establish Customer’s Trustworthiness. New York, NY: Russell Sage Foundation.
GARCIA-FLORES, R., Wang, X. & Goltz, G. (2000). Agent-based information flow for process industries’ supply chain modelling. Computers & Chemical Engineering, 24(2-7), 1135–1141. [doi:10.1016/S0098-1354(00)00494-4]
GEYER, R. & Cairney, P. (2015). Handbook on Complexity and Public Policy. Cheltenham: Edward Elgar Publishing.
GILBERT, N. & Troitzsch, K. (2005). Simulation for the Social Scientist (Second edition). Buckingham: Open University Press.
HAMILL, H. (2011). The Hoods. Princeton, NJ: Princeton University Press.
HAMILL, H., Hampshire, K., Mariwah, S. & Mshana, G. (2019). Managing uncertainty in medicine quality in Ghana: The cognitive and affective basis of trust in a high-risk, low-regulation context. Manuscript under review.
HAMILTON, W. L., Doyle, C., Halliwell-Ewen, M., & Lambert, G. (2016). Public health interventions to protect against falsified medicines: A systematic review of international, national and local policies. Health Policy and Planning, 31(10), 1448-1466.
HAMPSHIRE, K., Hamill, H., Mariwah, S., Mwanga, J., & Amoako-Sakyi, D. (2017). The application of Signalling Theory to health-related trust problems: The example of herbal clinics in Ghana and Tanzania. Social Science & Medicine, 188, 109-118. [doi:10.1016/j.socscimed.2017.07.009]
HAMPSHIRE, K., Porter, G., Mariwah, S., Munthali, A., Robson, E., Owusu, S. A., Abane, A. & Milner, J. (2016). Who bears the cost of ‘informal mhealth’? Health-workers’ mobile phone practices and associated political-moral economies of care in Ghana and Malawi. Health Policy and Planning, 32(1), 34-42.
HAMPSHIRE, K., Porter, G., Owusu, S. A., Tanle, A. & Abane, A. (2011). Out of the reach of children? Young people’s health-seeking practices and agency in Africa’s newly-emerging therapeutic landscapes. Social Science & Medicine, 73(5), 702–710. [doi:10.1016/j.socscimed.2011.06.035]
HAMPSHIRE, K. R. & Owusu, S. A. (2013). Grandfathers, Google, and dreams: Medical pluralism, globalization, and new healing encounters in Ghana. Medical Anthropology, 32(3), 247–265
HÖLLEIN, L., Kaale, E., Mwalwisi, Y. H., Schulze, M. H. & Holzgrabe, U. (2016). Routine quality control of medicines in developing countries: Analytical challenges, regulatory infrastructures and the prevalence of counterfeit medicines in Tanzania. TrAC Trends in Analytical Chemistry, 76, 60–70 [doi:10.1016/j.trac.2015.11.009]
JETLY, G., Rossetti, C. L. & Handfield, R. (2012). A multi-agent simulation of the pharmaceutical supply chain. Journal of Simulation, 6(4), 215–226.
JONKER, C. & Treur, J. (1999). Formal analysis of models for the dynamics of trust based on experiences. In European Workshop on Modelling Autonomous Agents in a Multi-Agent World, Berlin, Heidelberg: Springer, pp. 221-231. [doi:10.1007/3-540-48437-X_18]
KAUR, H. & Singh, S. P. (2016). Sustainable procurement and logistics for disaster resilient supply chain. Annals of Operations Research, 1–46.
KHOJAH, H. M., Pallos, H., Tsuboi, H., Yoshida, N., Abou-Auda, H. S., & Kimura, K. (2013). Adherence of community pharmacies in Riyadh, Saudi Arabia, to optimal conditions for keeping and selling good-quality medicines. Pharmacology & Pharmacy, 4(05), 431. [doi:10.4236/pp.2013.45061]
KIMBROUGH, S. O., Wu, D.-J. & Zhong, F. (2002). Computers play the beer game: can artificial agents manage supply chains?. Decision Support Systems, 33(3), 323–333.
LOOMES, G. & Sugden, R. (1982). Regret theory: An alternative theory of rational choice under uncertainty. The Economic Journal, 92(368), 805–824. [doi:10.2307/2232669]
MACKINTOSH, M., Chaudhuri, S. & Mujinja, P. G. (2011). Can NGOs regulate medicines markets? social enterprise in wholesaling, and access to essential medicines. Globalization and Health, 7(1), 4.
MACKINTOSH, M., Mugwagwa, J., Banda, G., Tibandebage, P., Tunguhole, J., Wangwe, S. & Karimi Njeru, M. (2018a). Health-industry linkages for local health: reframing policies for African health system strengthening. Health Policy and Planning, 33(4), 602–610. [doi:10.1093/heapol/czy022]
MACKINTOSH, M. & Mujinja, P. G. (2010). Markets and policy challenges in access to essential medicines for endemic disease. Journal of African Economies, 19(suppl_3), iii166–iii200.
MACKINTOSH, M., Tibandebage, P., Njeru, M. K., Kungu, J. K., Israel, C. & Mujinja, P. G. (2018b). Rethinking health sector procurement as developmental linkages in East Africa. Social Science & Medicine, 200, 182–189. [doi:10.1016/j.socscimed.2018.01.008]
MCPHEE-KNOWLES, S. (2015). Growing food safety from the bottom up: An agent-based model of food safety inspections. Journal of Artificial Societies and Social Simulation, 18(2), 9: https://www.jasss.org/18/2/9.html.
MITCHELL, L. & Ackland, G. J. (2007). Strategy bifurcation and spatial inhomogeneity in a simple model of competing sellers. EPL Europhysics Letters, 79(4), 48003. [doi:10.1209/0295-5075/79/48003]
MITCHELL, L. & Ackland, G. J. (2009). Boom and bust in continuous time evolving economic model. The European Physical Journal B, 70(4), 567–573.
MZIRAY, S., Mwamwitwa, K., Kisoma, S., Augustine, S., Fimbo, A., Hipolite, D., ... & Kaale, E. (2017). Post marketing surveillance of anti-malarial medicines in Tanzania. Pharmaceutical Regulatory Affairs, 6(191), 2. [doi:10.4172/2167-7689.1000191]
NASH, J. (1951). Non-cooperative games. Annals of Mathematics, 54(2), 286-295.
NAYYAR, G. M., Attaran, A., Clark, J. P., Culzoni, M. J., Fernandez, F. M., Herrington, J. E., Kendall, M., Newton, P. N. & Breman, J. G. (2015). Responding to the pandemic of falsified medicines. The American Journal of Tropical Medicine and Hygiene, 92(6_Suppl), 113–118. [doi:10.4269/ajtmh.14-0393]
NAYYAR, G. M., Breman, J. G., Newton, P. N. & Herrington, J. (2012). Poor-quality antimalarial drugs in southeast Asia and sub-saharan Africa. The Lancet Infectious Diseases, 12(6), 488–496.
NEWTON, P. N., Caillet, C. & Guerin, P. J. (2016). A link between poor quality antimalarials and malaria drug resistance? Expert Review of Anti-infective Therapy, 14(6), 531-533. [doi:10.1080/14787210.2016.1187560]
NEWTON, P. N., Hanson, K. & Goodman, C. (2017). Do anti-malarials in Africa meet quality standards? the market penetration of non quality-assured artemisinin combination therapy in eight African countries. Malaria Journal, 16(1), 204.
NEWTON, P. N., Tabernero, P., Dwivedi, P., Culzoni, M. J., Monge, M. E., Swamidoss, I., Mildenhall, D., Green, M. D., Jähnke, R., de Oliveira, M. d. S. et al. (2014). Falsified medicines in Africa: all talk, no action. The Lancet Global Health, 2(9), e509–e510. [doi:10.1016/S2214-109X(14)70279-7]
PALAFOX, B., Patouillard, E., Tougher, S., Goodman, C., Hanson, K., Kleinschmidt, I., Rueda, S. T., Kiefer, S., O’Connell, K. A., Zinsou, C. et al. (2014). Understanding private sector antimalarial distribution chains: a cross-sectional mixed methods study in six malaria-endemic countries. PloS ONE, 9(4), e93763.
PATOUILLARD, E., Hanson, K. G. & Goodman, C. A. (2010). Retail sector distribution chains for malaria treatment in the developing world: a review of the literature. Malaria Journal, 9(1), 50. [doi:10.1186/1475-2875-9-50]
PETROVIC, D., Roy, R. & Petrovic, R. (1998). Modelling and simulation of a supply chain in an uncertain environment. European Journal of Operational Research, 109(2), 299–309.
RENSCHLER, J. P., Walters, K. M., Newton, P. N. & Laxminarayan, R. (2015). Estimated under-five deaths associated with poor-quality antimalarials in sub-saharan Africa. The American Journal of Tropical Medicine and Hygiene, 92(6_Suppl), 119–126. [doi:10.4269/ajtmh.14-0725]
STERMAN, J. D. (1989). Modeling managerial behavior: Misperceptions of feedback in a dynamic decision making experiment. Management Science, 35(3), 321–339. [doi:10.1287/mnsc.35.3.321]
STRADER, T. J., Lin, F.-R., Shaw, M. J. et al. (1998). Simulation of order fulfillment in divergent assembly supply chains. Journal of Artificial Societies and Social Simulation, 1(2), 5: https://www.jasss.org/1/2/5.html.
SUTCLIFFE, A. & Wang, D. (2012). Computational modelling of trust and social relationships. Journal of Artificial Societies and Social Simulation, 15(1), 3: https://www.jasss.org/15/1/3.html. [doi:10.18564/jasss.1912]
SWAMINATHAN, J. M., Smith, S. F. & Sadeh, N. M. (1998). Modeling supply chain dynamics: A multiagent approach. Decision Science, 29(3), 607–632.
TAYLOR, P. D. & Jonker, L. B. (1978). Evolutionary stable strategies and game dynamics. Mathematical Biosciences, 40(1-2), 145–156. [doi:10.1016/0025-5564(78)90077-9]
TIVURA, M., Asante, I., van Wyk, A., Gyaase, S., Malik, N., Mahama, E., ... & Owusu-Agyei, S. (2016). Quality of artemisinin-based combination therapy for malaria found in Ghanaian markets and public health implications of their use. BMC Pharmacology and Toxicology, 17(1), 48.
TOWILL, D. R., Naim, M. M. & Wikner, J. (1992). Industrial dynamics simulation models in the design of supply chains. International Journal of Physical Distribution & Logistics Management, 22(5), 3–13. [doi:10.1108/09600039210016995]
TRAN, T. & Cohen, R. (2004). 'Improving user satisfaction in agent-based electronic marketplaces by reputation modelling and adjustable product quality.' In Proceedings of the Third International Joint Conference on Autonomous Agents and Multiagent Systems- Volume 2, New York, NY, pp. 828–835.
TREMBLAY, M. (2013). Medicines counterfeiting is a complex problem: a review of key challenges across the supply chain. Current Drug Safety, 8(1), 43–55 [doi:10.2174/1574886311308010007]
TYKHONOV, D., Jonker, C., Meijer, S. & Verwaart, T. (2008). Agent-based simulation of the trust and tracing game for supply chains and networks. Journal of Artificial Societies and Social Simulation, 11(3), 1: https://www.jasss.org/11/3/1.html.
TZAFESTAS, S. & Kapsiotis, G. (1994). Coordinated control of manufacturing/supply chains using multi- level techniques. Computer integrated manufacturing systems, 7(3), 206–212. [doi:10.1016/0951-5240(94)90039-6]
UMLAUF, R. & Park, S.-J. (2018). Stock-outs! improvisations and processes of infrastructuring in uganda’s hiv/aids and malaria programmes. Global Public Health, 13(3), 325–338.
VANDAM, S. (2016). Access to essential medicines - WHO update. Accessible at: https://ec.europa.eu/research/health/pdf/hpforum/access_to_essential_medicines-a_who_update.pd.
VON NEUMANN, J., & Morgenstern, O. (2007). Theory of Games and Economic Behavior (commemorative edition). Princeton, NJ: Princeton University Press.
WANG, Y., Audibert, J.-Y. & Munos, R. (2009). 'Algorithms for infinitely many-armed bandits.' In Advances in Neural Information Processing Systems pp. 1729–1736.
WANG, Y., & Vassileva, J. (2003). 'Bayesian network-based trust model.' In Proceedings IEEE/WIC International Conference on Web Intelligence (WI 2003), Halifax, NS, Canada, pp. 372-378.
WHO (2017a). A study on the public health and socioeconomic impact of substandard and falsified medical products. Geneva: World Health Organisation.
WHO (2017B). WHO Global Surveillance and Monitoring System for substandard and falsified medical products. Geneva: World Health Organisation.
WILSON, B. K., Kaur, H., Allan, E. L., Lozama, A. & Bell, D. (2017). A new handheld device for the detection of falsified medicines: demonstration on falsified artemisinin-based therapies from the field. The American Journal of Tropical Medicine and Hygiene, 96(5), 1117–1123. [doi:10.4269/ajtmh.16-0904]
WORLD BANK (2017). Tracking universal health coverage: 2017 Global Monitoring Report. Accessible at: http://www.worldbank.org/en/topic/universalhealthcoverage/publication/tracking-universal-healt.
YEUNG, S., Lawford, H. L., Tabernero, P., Nguon, C., van Wyk, A., Malik, N., DeSousa, M., Rada, O., Boravann, M., Dwivedi, P. et al. (2015). Quality of antimalarials at the epicenter of antimalarial drug resistance: results from an overt and mystery client survey in Cambodia. The American Journal of Tropical Medicine and hygiene, 92(6_Suppl), 39–50.