Introduction
Economic growth is highly correlated with an abundance of small, entrepreneurial firms (Bagley 2017; Glaeser et al. 2010). Increasing importance has been attached to incubators as mechanisms to enhance the economic and technological development of countries by promoting promising entrepreneurial ideas and encouraging the growth of newly established companies (Grimaldi & Grandi 2005). Increasing attention has been paid to incubators because of their important function in cultivating business enterprises (Chen 2007; Tran 2014). Incubators provide crucial elements to entrepreneurial firms, including not only funds, equipment and other external support, but also management, technology and knowledge (Albort-Morant & Oghazi 2016; Lasrado et al. 2016; Pena 2004). Knowledge is one of the most important resources for entrepreneurial enterprises to gain competitive advantage (Rothaermel & Thursby 2005). Knowledge learning of entrepreneurial teams in business incubators (BIETs) also plays an important part in improving their knowledge level (KL). Generally speaking, BIETs acquire knowledge in three main ways: by creating it themselves, by obtaining it from incubator managers and by learning, sharing and diffusing it among entrepreneurial teams. Up until now, the research has mainly focused on how incubators provide knowledge to entrepreneurial teams (e.g., Aerts et al. 2007; Bergek & Norrman 2008) but has focused less on studying knowledge learning among BIETs.
With the rapid development of the global economy, competition between organizations has turned into competition with regard to organizations’ ability to create, acquire and transfer knowledge. For firms, organizational learning enables them to access the managerial capabilities and technological know-how necessary to compete in a global economy. Consequently, they must update their knowledge base to respond to uncertainties and adapt to external changes at a faster rate than rivals to maintain a long-term competitive advantage (Harrison & Leitch 2005; Kowalska-Styczeń et al. 2018; Slater & Narver 1995). Previous research has suggested that organizational learning plays a crucial role in improving the KL of organizations (e.g., Berends & Antonacopoulou 2014; Chae et al. 2015; Saki et al. 2013). Therefore, a large number of organizations hope to improve their KL by organizational learning, which can enhance their competitiveness.
At the same time, to improve its competitive strength, an organization must not only learn but also forget (de Holan & Phillips 2004; Wong & Lam 2012). Forgetting is as important as organizational learning in the development of organizations, so that new knowledge can be explored to adapt to environmental changes. However, prior research has focused less on knowledge forgetting. Given the constant changes in the external environment, a portion of organizations’ original knowledge is unable to adapt (Martínez-Plumed et al. 2015). Forgetting can promote innovation in organizational knowledge and enhance the ability of organizations to adapt to external environment changes, which can improve their KL and competitiveness (Miller & Martignoni 2016). Thus, organizations have to not only learn new knowledge but also forget some old knowledge that cannot help them adapt to environmental changes. Otherwise, the old knowledge will hinder organizations’ competitiveness.
In addition, personnel turnover plays an important role in the innovation of organizational knowledge within the process of organizational learning (Fang et al. 2010; March 1991; Miller et al. 2006). When the external environment changes, maintaining appropriate personnel turnover contributes to the continuous updating of knowledge in organizations, which can improve the ability of organizations to adapt to changes in the external environment, thereby enhancing the competitiveness of organizations.
Similar to organizational learning, knowledge learning is very important for BIETs to improve their KL. However, knowledge learning is different from organizational learning. Organizational learning considers the mutual learning between the code and individuals and between individuals in an organization (Blaschke & Schoeneborn 2006; March 1991; Miller et al. 2006), while BIETs’ knowledge learning refers to the multilevel learning between BIETs and between the leader and members within BIETs. Moreover, forgetting is also considered a part of multilevel learning, given its important impact on KL. In addition, similar to updating knowledge in organizational learning, updating knowledge in incubators is also very important for these incubators’ hatching level, especially in the face of external environmental changes. Personnel turnover is important for the innovation of organizational knowledge in organizational learning; however, BIETs exit from and enter incubators as a whole team. Therefore, we should consider the effects of BIETs’ exit and entry on teams’ KL.
Therefore, this paper constructs a simulation model from the exploration and exploitation perspective to study the effect of entrepreneurial team learning, forgetting and the exit and entry of BIETs on teams’ KL in different environments and provides appropriate references and suggestions for BIETs’ knowledge learning and management. This study has three contributions. First, it discusses multilevel learning among BIETs and between the leader and members of BIETs. Second, it studies the effect of BIETs’ exit and entry on teams’ KL with environmental changes. Third, it adds BIETs’ forgetting to their knowledge learning and then compares their learning process with and without forgetting.
In the next section, we review the related literature. Then, we describe the construction of the simulation model. The simulation results are shown in Section 4. Finally, we discuss our conclusions as well as implications and research directions.
Theoretical Background
Organizational learning
As already mentioned, organizational learning can enable firms to access the managerial capabilities and technological know-how necessary to compete in a global economy (Harrison & Leitch 2005; Slater & Narver 1995). Firms with high levels of learning are associated with greater innovations, and there is a positive and significant relationship between all the elements of organizational learning and organizational innovation (Hurley & Hult 1998; Saki et al. 2013). Effective organizational learning activities can help firms reduce uncertainty and find market opportunities more quickly in order to compete more effectively (Wall 2018; Zhao et al. 2011). Many studies have discussed the relationship between organizational learning and knowledge performance by constructing simulation models. Miller et al. (2006) assume that organizational members are situated in a grid without edges in which each member has four neighbors and members learn through engaging in local and distant search. They found that the intermediate rate of learning from local search produces the highest knowledge, while learning from distant search has little effect on equilibrium knowledge. Fang et al. (2010) examined the impact of three interpersonal networks on organizational-level performance and found that dividing an organization into semi-isolated subgroups increases organizational-level performance.
In addition, studies on organizational learning provide us with empirical evidence. Keh et al. (2007) surveyed 2239 small businesses with fewer than 100 employees, and argue that proactive firms exchange, acquire and utilize knowledge more intensively. Grant & Baden-Fuller (2004) demonstrated that experimental learning (EL) takes precedence over acquisitive learning (AL) because integrating and internalizing knowledge within a firm is easier and more efficient. Zhao et al. (2011) survey 607 firms in China and found that entrepreneurial orientation (EO) is positively related to EL but has an inverse U-shaped relationship with AL. Both EL and AL enhance firm performance (FP), although the effects of AL are weaker and become non-significant when external knowledge is embedded into the firm’s internal private knowledge.
Prior studies on exploration and exploitation in organizational learning generally show that organizations that exhibit better learning are more competitive. However, knowledge in organizations may be out of date, and innovative activities often have a significant impact on the current stock of organizational knowledge (Greve & Taylor 2000). Moreover, organizations’ memory is limited. Hence, competitiveness is not just about learning, it is also about forgetting the right things at the right times (Derbinsky & Laird 2013; de Holan & Phillips 2004). Organizational forgetting and organizational learning are important for organizations and organizational forgetting also plays a critical role in business (Aydin & Gormus 2015; Bettis & Prahalad 1995; Martínez-Plumed et al. 2015). Skeptics argue that organizational learning should be facilitated by unlearning – a process of discarding obsolete beliefs and routines. By employing SEM, Wong & Lam (2012) investigate the effect of organizational learning and unlearning on the performance of construction organizations. Their results imply that if construction organizations unlearn, they may be better able to enhance performance. De Holan & Philipps (2004) indicated that unneeded stocks of knowledge require expensive management and can consume critical management attention, leading to a loss of competitiveness. They classify forgetting along two distinct dimensions and argue for four types of organizational forgetting. Moreover, they discussed three strategies of forgetting, including discarding unneeded knowledge, abandoning unwanted innovations and unlearning knowledge, which make organizational change difficult or impossible.
Blaschke & Schoeneborn (2006) add forgetting to March’s exploration-exploitation model and study how different rates of the code and individuals’ forgetting affect organizational knowledge. Their findings show that higher levels of individual forgetting result in increasingly lower equilibrium knowledge whether in exploration or exploitation and that increasing the organizational forgetting rate leads to even higher levels of knowledge. Based on their own simulation model, Miller et al. (2015) showed that knowledge and diversity are positively correlated across organizations. They also argue that, to a point, knowledge can increase with the rate of organizational learning, and it is quite possible that rapid learning produces higher long-run knowledge than slow learning with forgetting. De Holan & Phillips (2004) draw on an exploratory, multiple-case study of learning in international strategic alliances to explore how and why organizations forget and discuss the role of forgetting in the dynamics of organizational knowledge.
Learning and acquiring knowledge in business incubators
Business incubators (BIs) have become popular worldwide as a way to foster and accelerate the process of creating successful firms and entrepreneurs. BIs provide an ideal environment for entrepreneurial companies (Adegbite 2001; Perdomo et al. 2014; Porter & Kramer 2011; Tran 2014; Zhang et al. 2016). Current BIs are entrepreneurial centers that offer training, mentoring and technical assistance to startups, and incubators’ services vary (Albort-Morant & Oghazi 2016; Bruneel et al. 2012; Ford 2015; Lasrado et al. 2016; Sherman 1999). Grimaldi & Grandi (2005) map BIs into four categories and argue that the variety of incubating organizations is driven by the evolution of companies’ requirements and needs. Von Zedtwitz & Grimaldi (2006) propose a classification of five different incubators: regional BIs, university incubators, virtual incubators, independent commercial incubators, and company-internal incubators. The National Business Incubation Association (NBIA) (2014) defines a BI as an organization that supports businesses with business planning, counseling, financial assistance and facilities concentrating on startups (Lai & Lin 2015). Incubators are considered to be important policy tools for supporting innovation and entrepreneurial growth (Chen 2009; Mian et al. 2016).
Knowledge is recognized as a critical resource to gain and maintain a competitive advantage in business and it becomes very important in obtaining knowledge from others, especially for entrepreneurs in incubators (Temperli & Siebert 2012). Therefore, learning and acquiring knowledge in incubators has also received a lot of attention. Warren et al. (2009) note that knowledge acquisition can be described as a two-stage process where first, knowledge about business planning is gained from the Director, then firm and industry specific connections provide a unique set of knowledge flows that support the firm through the incubation process toward exit.
Anantatmula & Kanungo (2010) presented approaches to identify important strategies for implementing KM successfully and note that the knowledge factor has become increasingly important in BIs due to the knowledge-intensive nature of new-technology-based firms. Rubin et al. (2015) provide evidence from Australian and Israeli incubators and found that collaborations between incubatees, graduated incubatees and incubator management increase incubatees' knowledge of technology and of the market in both countries. Inkpen & Tsang (2005) examined how social capital dimensions of networks affect the transfer of knowledge between network members. Their results indicate that proximity to other members of an incubator in the network increases the likelihood that tacit knowledge will be transferred. Studdard (2006) explored how the entrepreneurial firm’s acquisition of business processes’ knowledge from interactions with incubator management positively impacts performance and finds that, by interacting with incubator management, entrepreneurial firms can improve their performance. Becker & Gassmann (2006) analyzed the kind of knowledge that facilitates the hatching and leveraging of technologies through the incubation process and distinguish four modes of mainly tacit knowledge according to different incubator types: entrepreneurial knowledge, organizational knowledge, technological knowledge, and complementary market knowledge. They argue that knowledge strategies include both the leveraging of internal knowledge and the in-sourcing of external knowledge for the firm through the corporate incubator. Ioana & Marin (2008) presented knowledge management as a new type of management for incubators, innovation indicators and modern innovative structures. Karapetyan & Otieno (2011) provide a conceptual model to explore, examined and illustrated both knowledge management and project management in the context of incubators.
Environmental changes
Nearly all business environments are in a state of ongoing change and global competition, rapid information transfer, economic challenge and advanced technologies may be advantageous or disadvantageous for organizations (Jovanovic 2015; Namwong et al. 2015; Pansuppawatt & Ussahawanitchakit 2011). Organizational innovations, decision making, characteristics and structures are all affected by the environment (Flynn 2016; Huang et al. 2014). Bielinska-Kwapisz (2014) argued that environmental changes can also influence the probability that the rules will be updated or changed. Current problems cannot be resolved with yesterday's solutions, and environmental conditions are so complicated, dynamic, and uncertain that, without innovation, organizations cannot guarantee longevity (Saki et al. 2013). Environmental changes lead organizations to continuously pursue better solutions and approaches in order to accommodate to the environment and thus, attain a competitive advantage (Ahmadi et al. 2014).
Lessons learned from past experience can often result in learning traps when the environment changes (Lant & Mezias 1990). Organizations should update their knowledge to adapt to the environment; personnel turnover is one way to do this. March (1991) examines both a closed system and an open system in his model. He studied the effects of environmental turbulence with and without personnel turnover on the average code knowledge and finds that turnover is useful in the context of the environment turbulence because it brings new knowledge into an organization. Personnel turnover serves a functional role by preserving knowledge diversity in organizations (Fang et al. 2010; Miller et al. 2006).
To sum up, most attention in the literature on organizational learning investigates exploration and exploitation in organizational knowledge performance. However, the literature focuses less on investigating knowledge learning among BIETs from the perspective of exploration and exploitation. Secondly, forgetting is also discussed widely in the literature on organizational learning. However, few studies discuss the learning process of BIETs, particularly the multilevel learning between BIETs and between leaders and members of a BIET. Third, many studies in organizational learning focus on the effect of organizations’ personnel turnover on their KL in the context of environmental changes. However, there is little work on the effects of the exit and entry of BIETs on teams’ KL, given environmental changes. Therefore, the purpose of this paper is to contribute to these streams of literature.
The Model
In this paper, we have constructed a computer simulation model to study the effect of entrepreneurial team learning, forgetting and the exit and entry of BIETs on teams’ KL in different environments. We aim to discuss the effect of learning between entrepreneurial team leaders, learning between entrepreneurial team leaders and entrepreneurial team members and the periodic exit and entry of BIETs on the KL of organizations with and without forgetting in static and dynamic complex task environments. These problems refer to the relationship between individuals and the relationship between teams, especially BIETs’ entry and exit. Traditional research methods, such as questionnaires, can rarely solve the problem of multilevel dynamic evolution. Therefore, we constructed an agent computer simulation that uses this simulation to carry out a series of experiments. Based on March’s classical model (1991) and on lessons from Miller’s description of forgetting (Miller 2015), we fully considers the BIETs’ network structure and their periodic exit and entry in the following simulation model.
Model specification
(1) External environment. In March’s model (1991), the external environment is modeled as an \(m\)-dimensional vector with each element randomly assigned a value of 1 or -1 with equal probability. Similarly to March, we assumed that the external environment has \(m\) dimensions, each of which is assigned a value of 1 or -1 with equal probability of 0.5. This external environment vector forms the basis for measuring the level of team knowledge.
(2) BIETs. We assumed that there are \(t\) BIETs, each of which consists of a leader and five members. The knowledge vector of the leader and members in each team corresponds to the external environment vector, and it has \(m\) dimensions, each of which has a value of 1, 0 or -1 with equal probability. At the same time, we assume that the leader is connected with each member and each member is connected with other members in each team. In different teams, a team’s leader is connected to other teams’ leaders, but a team’s members are not connected with other teams’ members. Figure 1 shows the simplified network structure of entrepreneurial teams.
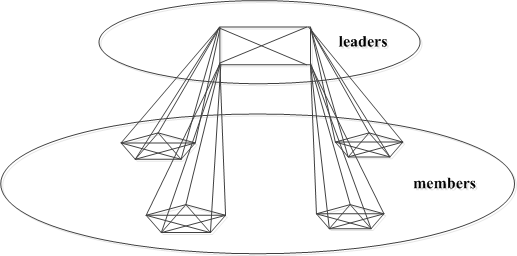
(3) Knowledge learning. March’s model (March 1991) emphasized that all individuals learned knowledge from the organization code but did not allow for direct person-to-person learning. Miller (2006) added person-to-person learning to his model. Miller highlighted knowledge learning among individuals. Considering March’s model and Miller’s model synthetically, we assumed that entrepreneurial team learning includes the learning within a BIET and the learning between different BIETs. Specifically, within a BIET, the team leader and members learn knowledge from each other. That is, the team leader learns from team members and team members learn from the leader. Moreover, team members learn from each other. Across different BIETs, a team leader learns from other team leaders, but team members do not learn from other teams’ members and leaders, so the learning between leaders of different teams represents that between different BIETs.
(4) Forgetting: Miller’s model (2015) assumed that converting a nonzero dimension of the individual knowledge vector (1 or -1) to a zero (0) was forgetting. Therefore, this paper uses Miller’s definition of forgetting as a reference and studies the effect of entrepreneurial team leaders’ forgetting on teams’ KL in the learning between different BIETs. Specifically, we supposed that every team leader converts a non-zero dimension of his knowledge vector (1 or -1) to a zero (0) with the probability \(f\) in every period.
(5) The knowledge level (KL). The equilibrium KL of organization can be used to evaluate the effectiveness of organizational learning. When it reaches equilibrium, the level of organizational equilibrium knowledge is the arithmetic average of all individual KLs in the organization. An individual’s KL depends on how many dimensions on which the individual knowledge vector matches the external environment vector. March (1991) calculated the proportion of reality that was correctly represented in individual beliefs to evaluate the average KL of individuals. March’s proposed method hypothesized that each dimension of the individual knowledge vector was independent of each other. However, in fact, teams’ knowledge is complex and not completely independent (Fang et al., 2010). Therefore, this paper adopted a method given by Fang et al. (2010) as follows:
$$\Phi(x) = k \Bigl( \Pi_{j=1}^k \delta_j + \Pi_{j=k+1}^{2k} \delta_j + \dots + \Pi_{j=m-k+1}^m \delta_j \Bigr) $$ |
In this function, \(m\) is the dimension of the external environment and the knowledge vector, \(k\) is the knowledge complexity, \(\Phi(x)\)is individuals’ KL, \(x\) represents individuals (leaders and members), and the value of \(\delta_j\) is 0 or 1. If an individual knowledge vector corresponds with the external environment vector on the corresponding dimension, \(\delta_j\) is 1; otherwise, \(\delta_j\) is 0. For example, we assume that the individual knowledge vector and the external environment vector have 10 dimensions (\(m\)=10), and the external environment vector is 1111111111. There are two members: A and B. The individual knowledge vector of A is 1011111111 and that of B is 1101110111. If \(k\) =2, KL of A is 2\(\cdot\)(1\(\cdot\)0+1\(\cdot\)1+1\(\cdot\)1+1\(\cdot\)1+1\(\cdot\)1)=8, and B is 2\(\cdot\)(1\(\cdot\)1+0\(\cdot\)1+1\(\cdot\)1+0\(\cdot\)1+1\(\cdot\)1)=6; if \(k\)=5, KL of A is 5\(\cdot\)(1\(\cdot\)0\(\cdot\)1\(\cdot\)1\(\cdot\)1+1\(\cdot\)1\(\cdot\)1\(\cdot\)1\(\cdot\)1)=5, and B is 5\(\cdot\)(1\(\cdot\)1\(\cdot\)0\(\cdot\)1\(\cdot\)1+1\(\cdot\)0\(\cdot\)1\(\cdot\)1\(\cdot\)1)=0; if \(k\)=10, KL of A is 10\(\cdot\)(1\(\cdot\)0\(\cdot\)1\(\cdot\)1\(\cdot\)1\(\cdot\)1\(\cdot\)1\(\cdot\)1\(\cdot\)1\(\cdot\)1)=0, and B is 10\(\cdot\)(1\(\cdot\)1\(\cdot\)0\(\cdot\)1\(\cdot\)1\(\cdot\)1\(\cdot\)0\(\cdot\)1\(\cdot\)1\(\cdot\)1)=0. Comparing the results of different values of \(k\), we can see that with different knowledge complexity, individuals’ KLs vary.
By calculating the arithmetic mean value of team leaders’ and members’ KL, we evaluated the KL of a team and then calculated the arithmetic mean value of the KL of all teams (the average KL of all teams) to estimate incubators’ performance.
Simulation process and parameters
(1) Learning within a BIET: The entrepreneurial team’s internal learning includes the team leader and members learning from each other and team members learning from each other. Because each member is connected with other members of a BIET, each member’s KL will be compared with other members’ KL. That is to say, a link between two members indicates that both are sources of learning for each other. Three results were compared: the same, high or low. If members make sure that the KLs of other members are higher, they will update their knowledge to incorporate aspects of this higher knowledge with probability \(p_1\). If there is more than one member whose KL is higher, we adopted a majority decision rule similar to that used in March’s model (1991). The majority decision rule is that members look at all of the other members with whom they connect and identify those that are better performing than themselves. Then, the focal member identifies the dominant knowledge vector on each of \(m\) dimensions of these higher-performing members’ knowledge. After that, the focal member will update his own knowledge with probability \(p_\1\).
While members’ knowledge learning is constant, leaders’ knowledge learning is periodical. We presume that leaders learn knowledge from the members of the same team and the leaders of other teams at every \(\alpha_1\) period. In other words, at every \(\alpha_1\) period, leaders first learn knowledge from the members of the same team. Similar to knowledge learning among members in a team, knowledge learning between a team’s leader and members also has three compared results: the same, high or low. If a leader find that the KL of a member of the same team is higher, he or she will update his or her knowledge to incorporate aspects of the higher-knowledge individual with probability \(p_2\). If there is more than one member whose KL is higher, the majority decision rule will also be used with probability \(p_2\). After leaders learn knowledge, members begin to learn knowledge from their own leaders. Because a leader is connected with all members in a BIET, each member’s KL will be compared to that of the leader. If members find that their team leader’s KL is higher, they will update their knowledge to incorporate aspects of the higher-knowledge leader with probability \(p_3\).
(2) The learning between different BIETs: The learning between different BIETs includes team leaders learning from each other. The process of leaders learning knowledge from other leaders is the same as that of members of a team learning knowledge from other members. Leaders update their knowledge with probability \(p_4\).
(3) Forgetting: In this paper, we mainly studied forgetting in the learning between different BIETs. Therefore, in the previous section, we supposed that every team leader converts a non-zero dimension of his knowledge vector (1 or -1) to a zero (0) with probability \(f\) in every period.
(4) External environment changes. We studied both a static environment and a dynamic environment. In the dynamic environment, we allowed the external environment to change periodically. Specifically, we shifted each of \(m\) dimensions of the external environment vector with probability \(p_5\) at every \(\alpha_2\) period(from 1 to -1, from -1 to 1. For example, we set \(p_5\)=0.1 and \(\alpha_2\)=100, that is, 10% of \(m\) dimensions change their values in every 100 periods.
(5) The exit and entry of BIETs. Entrepreneurial teams exit from incubators in three ways: graduating from incubators successfully; leaving incubators without achieving the standard; and failing in incubators. Here, we studied the first two types. We assumed that the rule of the exit and entry of BIETs was as follows. We calculated the KL of each team in incubators, and then sorted all teams from high to low according to their KL. We allowed the first \(d\) teams and the last \(d\) teams to exit from incubators, and at the same time, we allowed \(2d\) new teams to enter incubators.
All simulation parameters of this paper are shown in Table 1.
Parameter | Meaning | Parameter value |
The dimension of external environment and knowledge vector | 60 | |
The number of entrepreneurial teams and leaders | 20 | |
Knowledge complexity | 3 | |
\(p_1\) | The rate of members learning from each other in a team | 0.1, 0.3, 0.5, 0.7, 0.9 |
\(p_2\) | The rate of the leader learning from members in a team | 0.1, 0.3, 0.5, 0.7, 0.9 |
\(p_3\) | The rate of members learning from the leader in a team | 0.1, 0.3, 0.5, 0.7, 0.9 |
\(p_4\) | The rate of leaders learning from each other | 0.1, 0.3, 0.5, 0.7, 0.9 |
The proportion of external environment change | 0.1 | |
The rate of leaders forgetting | 0, 0.01, 0.05, 0.1 | |
\(\alpha_1\) | The period of leaders’ personal learning | 5 |
\(\alpha_2\) | The period of external environment change | 100 |
The number of BIETs’ entry \(2d\) and exit \(2d\) | 0, 1, 2, 3, 4 |
Analysis of the Simulation and Results
We constructed the simulation model using MATLAB. Details are available in the following sections. In order to ensure the accuracy of the results, all results presented in this paper are averages based on 50 runs of each simulation model.
In a static environment
Without forgetting
Here, we studied the learning process within a BIET and between BIETs in both a static environment and a dynamic environment. First, we studied the knowledge learning process within a BIET and between BIETs in a static environment without forgetting. Simulation results are shown in the figures below.
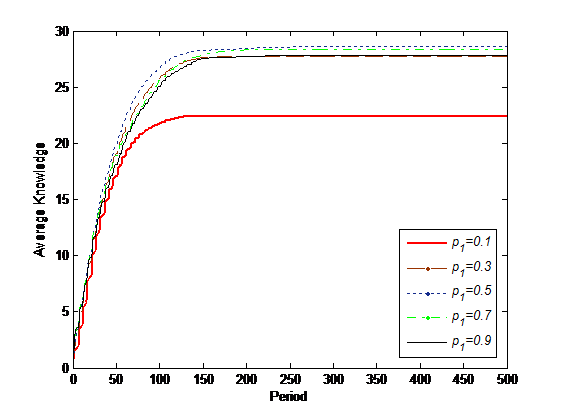
Figure 2 shows the effect of team members learning from each other on knowledge. In Figure 2, different values of \(p_1\) produce different equilibrium KLs among teams. As shown in Figure 2, when the rate of team members learning from each other increases from 0.1 to 0.5, the equilibrium KL of teams also increases gradually. However, the equilibrium KL of teams decreases gradually when the rate increases from 0.5 to 0.9. In other words, an increase in the rate of team members learning from each other does not increase the equilibrium KL.
This result shows that although strengthening learning among team members can improve a team’s KL, as the rate of team members learning from each other increases, this improvement does not necessarily increase. Moderate rates of team members learning from each other (\(0.3\leq p_1 \leq 0.7\)) can produce a superior equilibrium KL among teams, while the rates that are too high or too low have a negative effect on the equilibrium KL of teams. In other words, if the rate of team members learning from each other is too low, the knowledge mastered by team members cannot be shared and used in time, which is not conducive to improving teams’ KL. If the rate of team members learning from each other is too high, the knowledge mastered by team members spreads and converges quickly, decreasing knowledge diversity. This effect is not conducive to the innovation of knowledge and has a negative effect on teams’ KL.
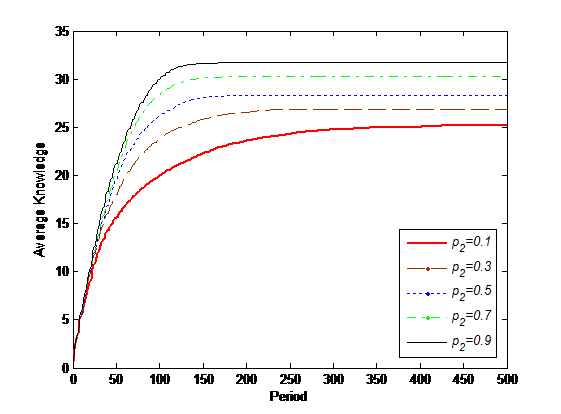
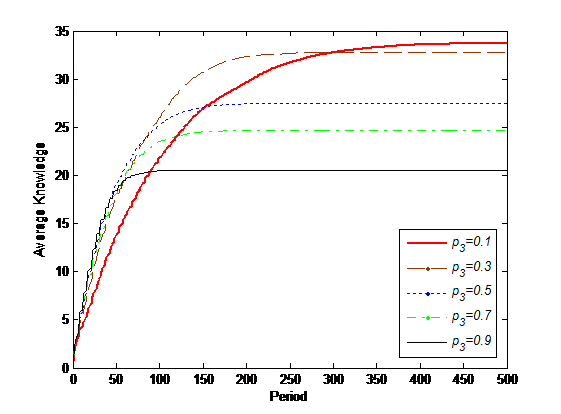
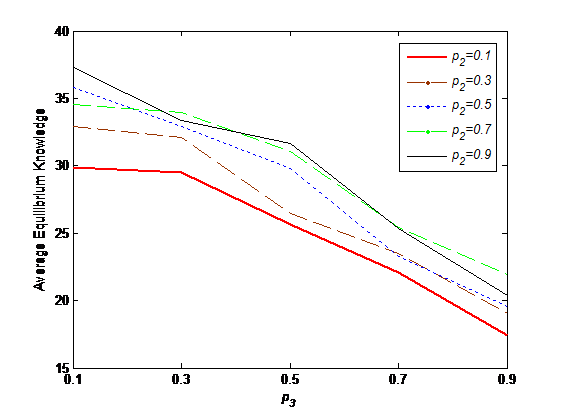
Figure 3 and Figure 4 show the results of learning between the team leader and team members. Figure 3 shows that as the rate of the team leader learning from members increases, the equilibrium KL of teams also increases gradually. Nevertheless, Figure 4 indicates that as the rate of team members’ learning from the team leader increases, the equilibrium KL of teams decreases gradually. In addition, in Figure 3 and Figure 4, rapid learning rates achieve the equilibrium quickly in the short run, while slow learning rates do so in the long run. Figure 5 shows the joint rate of the team leader learning from members and the rate of team members learning from the team leader. As shown in Figure 5, the combination of a high rate of the team leader learning from members and a low rate of team members learning from the team leader can produce a superior equilibrium KL for teams.
These findings correspond with those of March (1991) and Miller et al. (2006). On one hand, rapid learning may offset the heterogeneity of members’ knowledge quickly, so equilibrium will be achieved in the short run, but equilibrium KL is low, which is what March called exploitation. Slow learning, called exploration, can promote the diversity of knowledge, which leads to equilibrium KL in the long run. On the other hand, a team leader is the synthesizer of knowledge and needs to deliver advanced knowledge to team members. Hence, the team leader should learn rapidly from the team members who own advanced knowledge and share knowledge with team members in a timely fashion to improve KL of the team.
This learning process occurs within a BIET. In the following section, we will discuss the learning process between BIETs, that is, the learning process between team leaders.
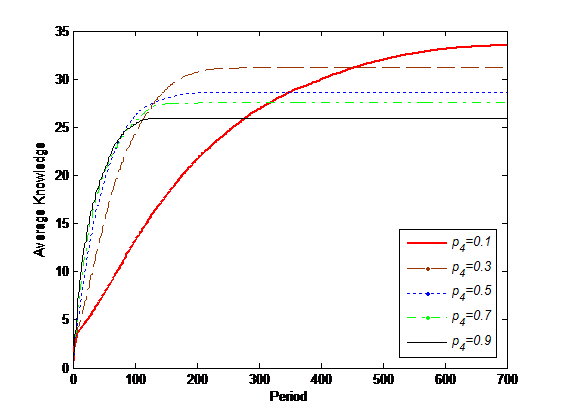
In the previous section, we assumed that BIETs learn from each other through the learning between team leaders. Figure 6 shows the effect of team leaders learning from each other on knowledge. From Figure 6, we find that with the increase in the rate of team leaders learning from each other, the equilibrium KL of teams decreases gradually. While rapid learning rates achieve the equilibrium quickly in the short run, slow learning rates do so in the long run.
Although rapid learning between BIETs improves teams’ KL in a short time (exploitation), it will make the team absorb the knowledge of other teams passively, which is not conducive to the exploration and discovery of new knowledge within a team and results in a low equilibrium KL for teams. When BIETs learn from each other slowly (exploration), teams explore new knowledge and keep knowledge diversity within teams, which leads to slow increase in teams’ KL and a high KL in the long term.
With forgetting
In the previous section, we mainly studied the knowledge learning process within a BIET and between BIETs without forgetting. In the following section, we will add forgetting to the learning process within a BIET and between BIETs. This section defines forgetting as every team leader converting a nonzero dimension of his knowledge vector (1 or -1) to a zero (0) with the probability \(f\) in every period. The simulation results are as follows.
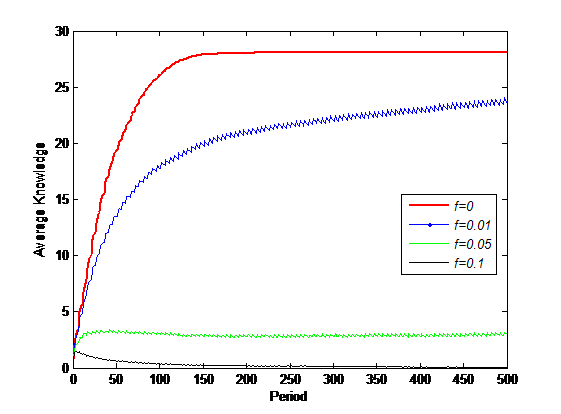
Figure 7 demonstrates the effect of different rates of BIETs forgetting on knowledge. Figure 7 shows that as the forgetting rate increases, the equilibrium KL of teams decreases gradually. This finding indicates that forgetting has a strictly negative effect on teams’ KL.
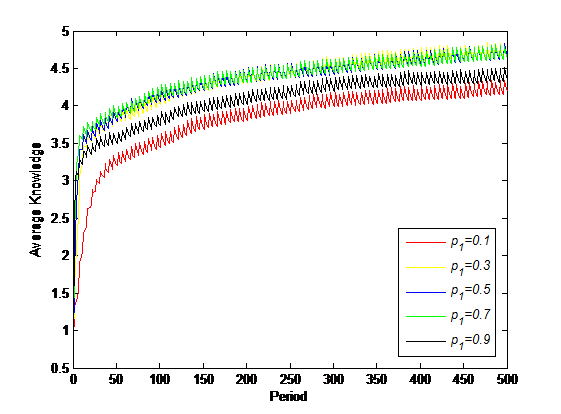
Figure 8 shows the effect of different rates of team members learning from each other on knowledge with forgetting (\(f=0.1\)). As shown in Figure 8, when \(t\) is 500, rates of team members learning from each other that are too low (\(p_1\)=0.1) and too high (\(p_1\)=0.9) produce a lower average team KL, while moderate rates (\(0.3 \leq p_1 \leq 0.7\)) produce a superior average team KL. Comparing Figure 8 and Figure 2 shows that when the period is the same, the average KL of teams with forgetting is lower than that without forgetting on the whole. However, moderate rates (\(0.3 \leq p_1 \leq 0.7\)) of team members learning from each other can produce a superior KL of teams regardless of whether there is forgetting. These results suggest that the effect of forgetting on the relationship between the rate of team members’ learning from each other and teams’ KL is not obvious.
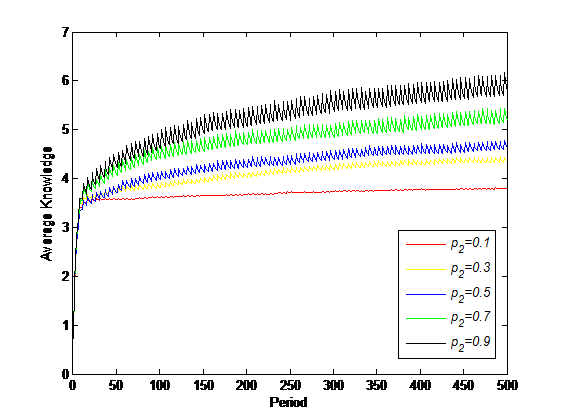
Figure 9 shows the effect of different rates of the team leader learning from members on knowledge with forgetting (\(f\)=0.1). Figure 9 shows that, with the increase of the rate (p_2) of the team leader learning from members, the average KL of teams increases gradually when t is 500. Comparing Figure 9 and Figure 3 shows that when the period is the same, the average KL of teams with forgetting is lower than that without forgetting on the whole. Nevertheless, the rate of the team leader’s learning from members and the average KL of teams is positively correlated regardless of whether there is any forgetting. The results above indicate that forgetting does not affect the relationship between the rate of the team leader learning from members and teams’ KL.
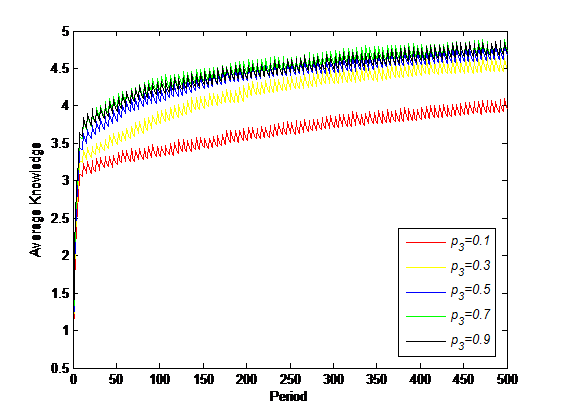
Figure 10 shows the effect of different rates of team members learning from the team leader on knowledge with forgetting (f=0.1). As seen in Figure 10, with the increase in the rate (\(p_3\)) of team members learning from the team leader, the average KL of teams increases at the beginning and then decreases when \(t\) is 500. Comparing Figure 10 and Figure 4 shows that when the period is the same, the average KL of teams with forgetting is lower than that for those without forgetting on the whole. At the same time, the relationship between the rate of team members learning from the team leader and the average KL of teams has changed. When the period is the same, the average KL of teams decreases gradually with the increase in the rate of team members learning from the team leader without forgetting, while it increases at the beginning and then decreases with forgetting.
Here, we considered the mutual learning between the team leader and members within a BIET synthetically. The results are shown in Figure 11.
Figure 11. The joint effect of the team leader and members learning from each other on knowledge with forgetting. Note: Other parameter settings: \(p_1\)=0.5, \(p_2\)=0.5, \(p_4\)=0.5, \(m\)=60, \(t\)=20, \(r\)=5, \(k\)=3, \(f\)=0.1.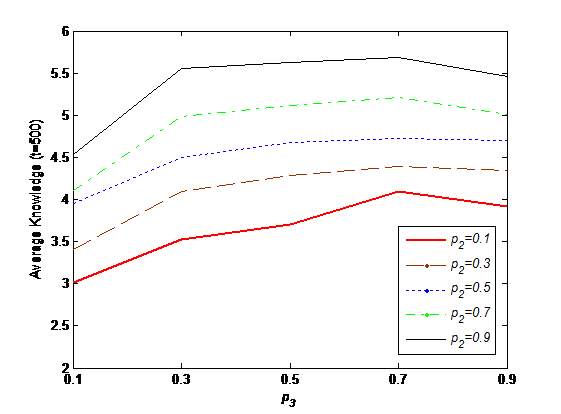
Figure 11 shows the effect of the mutual learning between the team leader and members on knowledge with forgetting (\(f\)=0.1). Comparing ">Figure 11 and ">Figure 5 shows that the relationship between the rate of team leaders learning from members and teams’ KL does not change. However, as the rate (\(p_3\)) of team members learning from the team leader increases, the average KL of teams changes from declining gradually to rising at the beginning and then decreasing. These results suggest that forgetting changes the relationship between the rate of team members learning from the team leader and teams’ KL.
Entrepreneurial team leaders are synthesizers of team knowledge: through learning from members with high KL within teams and other team leaders, they can master advanced knowledge. If the rate of team members learning from the leader is too low (such as \(p_3\)=0.1), team members cannot learn and utilize the advanced knowledge mastered by the leader in a timely fashion, which lower the average KL of teams. If the rate is too high (such as \(p_3\)=0.9), forgetting leads team members to turn their own advanced knowledge into no knowledge, which also lowers the average KL of teams. Moderate rates (\(0.3 \leq p_3 \leq 0.7\)) not only ensure that team members learn advanced knowledge from the leader in time but also reduce the possibility of turning these members’ own advanced knowledge into no knowledge, producing a superior average KL of teams.
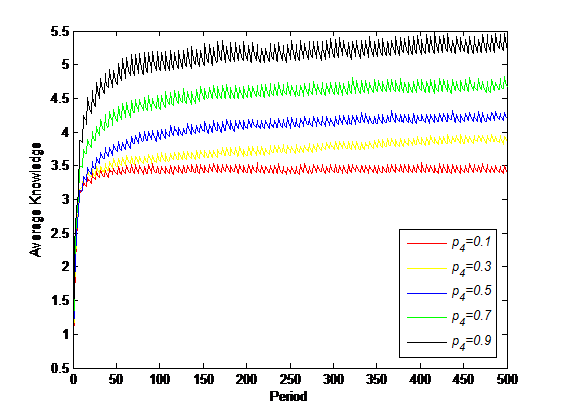
Figure 12 shows the effect of team leaders learning from each other on knowledge with forgetting. When the rate of forgetting is 0.1 (\(f\)=0.1), as the rate of BIETs learning from each other increases, the equilibrium KL of teams also increases gradually. Forgetting can result in a positive correlation between the rate of BIETs learning from each other and the equilibrium KL. Comparing Figure 6 and Figure 12, we find that the rate of BIETs learning from each other and the equilibrium KL are negatively correlated. A moderate rate of BIETs forgetting will increase teams’ KL as the rate of BIETs learning from each other increases.
According to Miller and Martignoni (2016), these results imply that when BIETs learn from each other without forgetting, constant learning tends to make teams’ knowledge homogeneous. As the rate of BIETs learning from each other increases, the knowledge of teams tends to become homogeneous rapidly, and the knowledge diversity of teams decreases. When BIETs only forget, teams’ knowledge diversity may decrease for a distinct reason. Forgetting turns nonzero dimensions into zeros, which tends to drive teams to no knowledge and results in the decrease of teams’ knowledge diversity. These two are extreme cases. In reality, when BIETs learn from each other with forgetting, the extremes can be avoided and teams’ knowledge diversity preserved, which may create a positive correlation between the rate of BIETs learning from each other and the average equilibrium KL of teams under a moderate rate of forgetting.
In a dynamic environment
Forgetting
In this subsection, we look at environmental changes, forgetting and the exit and entry of BIETs in a dynamic environment. We assumed that the external environment vector was fixed in a static environment. However, in a dynamic environment, we let each \(m\) dimension of the external environment vector shift with probability \(p_5\) at every \(\alpha_2\)period, which represents environmental changes. First, we studied the relationship between forgetting and teams’ KL in the context of environmental changes. Here, we assumed that the entrepreneurial team leaders’ forgetting is on behalf of the of BIETs’ forgetting and looked into the effects of entrepreneurial team leaders’ forgetting on teams’ KL. The simulation results were as follows.
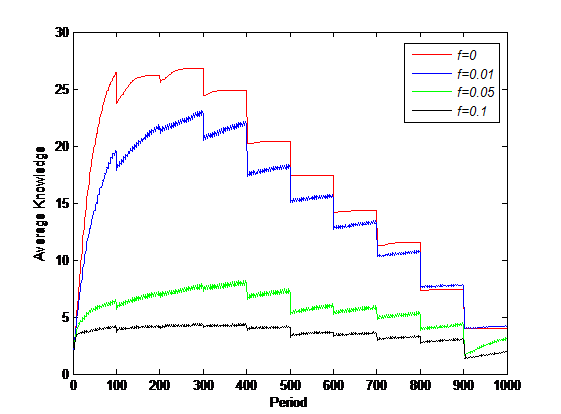
Figure 13 shows the effect of different rates of forgetting on knowledge with environmental changes. From Figure 13, we can see that the average KL of teams decreased gradually when the external environment changed constantly. Simultaneously, different rates of forgetting have different effects on the average KL of teams. As the rate of forgetting increases, the average KL of teams decreases. However, when the rate of forgetting was 0.01, the average KL of teams rebounded in the long run. These results indicated that environmental changes have a negative effect on teams’ KL and that a moderate rate of forgetting can make teams’ KL rebound in the long run, although this rebound was not obvious.
When the external environment changes, BIETs’ original knowledge is not able to adapt to the new environment and BIETs should explore new knowledge to solve these new problems. BIETs’ ability to store knowledge is limited. Forgetting old knowledge that cannot adapt to a new environment can provide conditions for creating new knowledge. However, it requires a large investment and exploration over a long period of BIETs to create new knowledge, which has a certain effect on the long-term KL of teams, but this effect is not obvious.
BIETs’ exit and entry
In this section, we studied the effect of the exit and entry of BIETs on teams’ KL with external environment changes. First, we let each \(m\) dimension of the external environment vector shift with probability \(p_5\) at every \(\alpha_2\) period to represent environmental changes. Second, we allowed exit and entry of BIETs to take place, after sorting all teams from high to low according to the KL, we let the first \(d\) teams and the last \(d\) teams exit from incubators. At the same time, we made \(2d\) new teams enter incubators. When an entrepreneurial team leaves, it was replaced by a new entrepreneurial team, which begins with a randomly assigned knowledge vector. The simulation results are shown in Figure 14.
Figure 14. The effect of environmental changes and the exit and entry of entrepreneurial teams on knowledge. Note: Other parameter settings: \(p_1\)=0.5, \(p_2\)=0.5, \(p_3\)=0.5, \(p_4\)=0.5, \(p_5\)=0.1, \(m\)=60, \(t\)=20, \(r\)=5, \(k\)=3, \(f\)=0, \(\alpha_2\)=100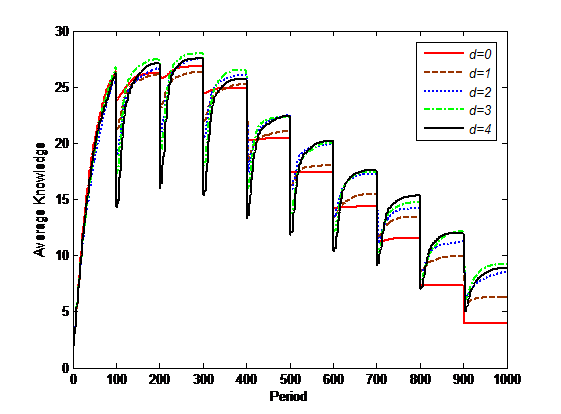
Figure 14 shows the effect of the exit and entry of BIETs on teams’ KL with environmental changes. As shown in Figure 14, we can see that after an environmental shift, teams’ KL suffered an immediate drop. However, with BIETs’ exit and entry, teams’ KL were able to recover and improve from the environmental shift. Without BIETs’ exit and entry, over time, entrepreneurial teams lost their ability to adapt to environmental changes. Meanwhile, different proportions of BIETs’ exit and entry had different effects on teams’ average KL. When \(d\) increased from 1 to 3, the average KL of teams increased gradually, but when \(d\) increased from 3 to 4, it declined. These results suggest that when the external environment changes, maintaining the exit and entry of BIETs can improve teams’ KL. However, greater amounts of exit and entry by BIETs do not necessarily imply better outcomes; a moderate proportion of exit and entry by BIETs can increase teams’ KL.
As argued in the previous section, when the external environment changes, the original knowledge of BIETs is not able to adapt to the new environment and BIETs should explore new knowledge to solve new problems. With BIETs’ exit and entry, new entrepreneurial teams can bring new knowledge to the original entrepreneurial teams. New knowledge replaces the old knowledge that cannot adapt to the environmental changes through the mutual learning between entrepreneurial teams, and this can produce new ways to solve problems caused by environmental changes, which can help BIETs adapt to a new environment and improve the knowledge level of teams in the long run. In addition, the proportion of BIETs’ exit and entry has an effect on the rate of knowledge update in incubators. If the proportion of the exit and entry of BIETs is high, although it can bring new knowledge into incubators quickly, the BIETs’ ability to absorb and utilize new knowledge is different, which may imply that BIETs cannot absorb and utilize new knowledge fully and affect the promotion of teams’ KL. On the contrary, if the proportion of the exit and entry of BIETs is low, new knowledge cannot flow into incubators in time when the external environment changes, which may affect the knowledge update of BIETs and, thus, the promotion of teams’ KL. Therefore, a moderate proportion of the exit and entry of BIETs can produce a higher team KL.
Discussion
This paper studied the effect of entrepreneurial team learning, forgetting and the exit and entry of BIETs on teams’ KL in different environments by using the computer simulation method. It contributes to the literature on the knowledge learning of BIETs in three different ways. First, by describing a typical network structure between BIETs and between the leader and members of BIETs, it discusses the multilevel learning between BIETs and between the leader and members of BIETs. Second, it studies the effect of BIETs’ exit and entry on teams’ KL with environmental changes. Third, it adds BIETs’ forgetting to their knowledge learning and then compares their learning process with forgetting and that without forgetting.
The first contribution of this study is that it discusses multilevel learning between BIETs and between the leader and members within BIETs by describing a typical network structure between BIETs and between the leader and members within BIETs. March (1991) emphasized that all individuals learned knowledge from the organization code, but he did not allow for direct person-to-person learning. Miller et al. (2006) added person-to-person learning in his research. Considering the contact between the leader and members within BIETs and the contact between BIETs, this paper describes a relevant network structure and studies the multilevel learning between BIETs and between the leader and members of BIETs entirely, which includes the learning between members, the learning between the leader and members (the leader learning from members and members learning from the leader) within BIETs and the learning between BIETs.
The second contribution of this study is that it studied the effect of BIETs’ exit and entry on teams’ KL with environmental changes. As shown in prior research, many studies have investigated the effect of personnel turnover in organizations on organizations’ KL from the perspective of individual in organizations (Fang et al. 2010; March 1991; Miller et al. 2006). However, personnel turnover in BIETs is different. BIETs exit from and enter incubators as a whole team. Therefore, we studied the effect of the exit and entry of BIETs on teams’ KL with environmental changes.
The third contribution of this study is that it adds BIETs’ forgetting to their knowledge learning and then compares BIETs’ learning process with forgetting and that without forgetting. As argued previously, for a long time, enterprises only paid attention to organizational learning and ignored or even tried to avoid forgetting. In fact, organizational learning and forgetting are mutually complementary (Blaschke & Schoeneborn 2006; Holan and Phillips 2004; Miller & Martignoni 2016). Similar to organizational learning, forgetting also plays a vital role in improving organizations’ KL. Hence, we considered BIETs’ learning and forgetting equally to study the effect of BIETs’ forgetting on teams’ KL and compared the learning process of BIETs with forgetting and that without forgetting.
The results of this paper show that first, in a static environment, the rate of BIETs learning from each other and the average equilibrium KL of teams are negatively correlated, but a moderate rate of BIETs’ forgetting can create a positive correlation. Second, within a BIET, high rates of leader learning from members and low rates of members’ learning from the leader can improve teams’ KL, while a high rate of leader learning from members and a moderate rate of members learning from the leader can improve teams’ KL with forgetting. Third, in a dynamic environment, external environment changes affect the process of BIETs’ knowledge learning. When the external environment changes, BIETs’ original knowledge is unable to help BIETs to adapt to the new environment, which results in the gradual decline of teams’ KL. With the exit and entry of BIETs and forgetting, however, teams’ KL can recover and improve to create new knowledge. Therefore, maintaining a moderate amount of BIETs’ exit and entry and forgetting can help to improve teams’ KL in the long run; BIETs’ exit and entry improves teams’ KL more significantly.
Theoretical implications
Our study contributes to research on knowledge learning of BIETs and has several theoretical implications. In the field of organizational learning, researchers have discussed the importance of exploration and exploitation for organizational learning (Blaschke & Schoeneborn 2006; Fang et al. 2010; March 1991; Miller et al. 2006). However, there is less research discussing BIETs’ learning . Therefore, this paper adopts an exploration and exploitation perspective to study the effect of the multilevel learning between BIETs and between the leader and members within BIETs (including knowledge learning and forgetting) on teams’ KL. Simultaneously, this paper illustrates the importance of maintaining a moderate amount of BIETs’ exit and entry by discussing its effect on teams’ KL in a dynamic environment. This study supplements the theory of BIETs’ learning to some extent and provides theoretical references for future research.
Managerial implications
The conclusions of this paper also have several managerial implications for incubator managers and entrepreneurial team managers. For entrepreneurial teams, first, within an entrepreneurial team, team leaders should strengthen their contacts with team members. By learning from team members, leaders can gather advanced knowledge and then deliver advanced knowledge within the entrepreneurial team in a timely manner.
Team members should also strengthen their contacts with other team members. However, more contact between team members does not necessarily produce better outcomes. BIETs should aim for a moderate rate of learning, which allows team members to explore and discover new knowledge independently. For example, team leaders should encourage team members to combine learning lessons from experience with thinking independently to solve problems. Second, different entrepreneurial teams should learn from each other in incubators.
Learning advanced knowledge from other entrepreneurial teams can improve teams’ KL. Nevertheless, entrepreneurial teams should not depend on other entrepreneurial teams excessively to learn knowledge. Strengthening the exploration and innovation of knowledge within the entrepreneurial team, as well as learning from other entrepreneurial teams, plays a critical role in the long-term development of the team. At the same time, team leaders’ ability to store knowledge is limited, so, they should focus not only on knowledge learning but also on knowledge forgetting. Entrepreneurial teams should discard old, useless knowledge when they learn new knowledge in order to absorb and create new knowledge better. The joint action of knowledge learning and knowledge forgetting contributes to maintaining teams’ knowledge diversity, which can help entrepreneurial teams adapt to environmental changes.
For incubator managers, it is very important to not only ensure the transfer and utilization of knowledge among entrepreneurial teams but also update knowledge in incubators constantly according to environmental changes. Managers of incubators should maintain a moderate amount of exit and entry among entrepreneurial teams on the basis of their performance. Therefore, the entry of new entrepreneurial teams allows new knowledge to enter incubators. Hence, original entrepreneurial teams in incubators can learn from new entrepreneurial teams to update their own knowledge, which is helpful for entrepreneurial teams in the face of the environmental changes and enhances the ability to adapt to environmental changes.
Limitations and research directions
In this paper, using the computer simulation method, we studied the effect of entrepreneurial team learning, forgetting and the exit and entry of BIETs on teams’ KL in incubators in different environments. However, our study does have some limitations. First, the network structure of BIETs and that between the leader and members within BIETs described in this paper is typical, but it is not the only network structure. Future research could be based on this premise and discuss the effect of different network structures between BIETs and between the leader and members within BIETs on teams’ KL.
Another limitation is that it studies mainly BIETs’ knowledge learning process by using computer simulation methods. Because of the limitations of this research method, we only simulated BIETs’ knowledge learning. However, BIETs’ learning includes not only knowledge but also other resources. Therefore, future research could simulate the learning of BIETs’ other resources to discuss BIETs’ learning process entirely.
Finally, the simulation model constructed in this paper adopted mainly the theoretical perspective to study the relationship between entrepreneurial team knowledge learning, forgetting and teams’ KL. Theoretical research should in practice be verified. Therefore, future research can conduct a more comprehensive and deeper discussion and verification by combining theoretical and empirical research.
Acknowledgements
This work is supported by the National Social Science Foundation of China (Grant No. 17BGL025). The authors are grateful to an anonymous referee for helpful comments that improved this paperReferences
ADEGBITE, O. (2001). Business incubators and small enterprise development: the Nigerian experience. Small Business Economics, 17(3), 157-166.
AERTS, K., Matthyssens, P., Vandenbempt, K., 2007. Critical role and screening practices of European business incubators. Technovation, 27(5), 254-267.
AHMADI, A., Daryani, S.M. & Bevrani, H. (2014). Evaluation of organizational learning process based on Marquardt model. Case study: Municipality of Noor City, Iran. International Journal of Management and Innovation, 6(1), 56-66.
ALBORT-MORANT, G., Oghazi, P., 2016. How useful are incubators for new entrepreneurs? Journal of Business Research, <69i>(6), 2125-2129.
ANANTATMULA, V.S. & Kanungo, S. (2010). Modeling enablers for successful KM implementation. Journal of Knowledge Management , 14(1), 100-113.
AYDIN, E., Gormus, A.S., 2015. Does organizational forgetting matter? Organizational survival for life coaching companies. Learning Organization 22, 150-162.
BAGLEY, M.J.O., 2017. A simulation of entrepreneurial spawning. Journal of Artificial Societies and Social Simulation, <20i>(3), 9.
BECKER, B. & Gassmann, O. (2006). Gaining leverage effects from knowledge modes within corporate incubators. R and D Management, 36(1), 1-16.
BERENDS, H., Antonacopoulou, E. (2014). Time and organizational learning: a review and agenda for future research. International Journal of Management Reviews, 16(4), 437-453.
BERGEK, A. & Norrman, C., (2008). Incubator best practice: A framework. Technovation, 28(1-2), 20-28.
BETTIS, R.A., Prahalad, C.K. (1995). The dominant logic: retrospective and extension. Strategic Management Journal, 16, 5-14.
BIELINSKA-KWAPISZ, A. (2014). Triggers of organizational change: duration, previous changes, and environment. Journal of Change Management, 14(3), 405-424.
BLASCHKE, S. & Schoeneborn, D. (2006). The forgotten function of forgetting: revisiting exploration and exploitation in organizational learning. Soziale Systeme, 12(1), 99-119.
BRUNEEL, J., Ratinho, T., Clarysse, B. & Groen, A. (2012). The evolution of business incubators: comparing demand and supply of business incubation services across different incubator generations. Technovation, 32(2), 110-121.
CHAE, S.W., Seo, Y.W., & Lee, K.C. (2015). Task difficulty and team diversity on team creativity: multi-agent simulation approach. Computers in Human Behavior, 42, 83-92.
CHEN, C.-J. (2009). Technology commercialization, incubator and venture capital, and new venture performance. Journal of Business Research, 62(1), 93-103.
CHEN, M.H., 2007. Entrepreneurial leadership and new ventures: creativity in entrepreneurial teams. Creativity and Innovation Management, <16i>(3), 239–249.
DE HOLAN, P. M. & Phillips, N. (2004). Remembrance of things past? The dynamics of organizational forgetting. Management Science, 50(11), 1603–13.
DE HOLAN, P. M. & Phillips, N. (2011). 'Organizational forgetting.' Handbook of Organizational Learning and Knowledge Management. John Wiley, Chichester, pp. 433-452.
DERBINSKY, N. & Laird, J.E. (2013). Effective and efficient forgetting of learned knowledge in Soar’s working and procedural memories. Cognitive Systems Research, 24, 104-113.
FANG, C., Lee, J. & Schilling, M.A. (2010). Balancing exploration and exploitation through structural design: the isolation of subgroups and organizational learning. Organization Science, 21(3), 625-642.
FLYNN, S. I. (2016). Organizational environment. Research Starters Sociology, 1-7.
FORD, C.M. (2015). Beyond a social capital agenda: exploring metrics and motives inside business incubators in Arkansas, 5th International Conference on Engaged Management Scholarship, Baltimore, MD.
GLAESER, E.L., Kerr, W.R., Ponzetto, G.A.M., 2010. Clusters of entrepreneurship. Journal of Urban Economics, <67i>(1), 150-168.
GRANT, R.M. & Baden-Fuller, C. (2004). A knowledge accessing theory of strategic alliances. Journal of Management Studies, 41(1), 61-84.
GREVE, H.R. & Taylor, A. (2000). Innovations as catalysts for organizational change: shifts in organizational cognition and search. Administrative Science Quarterly, 45(1), 54-80.
GRIMALDI, R. & Grandi, A. (2005). Business incubators and new venture creation: an assessment of incubating models. Technovation, 25(2), 111-121.
HARRISON, R.T. & Leitch, C.M. (2005). Entrepreneurial learning: researching the interface between learning and the entrepreneurial context. Entrepreneurship Theory and Practice, 29(4), 351-371.
HUANG, S., Ding, D. & Chen, Z. (2014). Entrepreneurial leadership and performance in Chinese new ventures: A moderated mediation model of exploratory innovation, exploitative innovation and environmental dynamism. Creativity and Innovation Management, 23(4), 453-471.
HURLEY, R.F. & Hult, G.T.M. (1998). Innovation, market orientation, and organizational learning: an integration and empirical examination. Journal of Marketing, 62, 42-54.
INKPEN, A.C. & Tsang, E.W.K. (2005). Social capital, networks, and knowledge transfer. Academy of Management Review, 30(1), 146-165.
IOANA, L., Maria, N. (2008). Financing, innovation and knowledge management defining elements for business incubators. Annals of the University of Oradea, Economic Science Series, 17(13), 322-326.
JOVANOVIC, Z. (2015). Management and changes in business environment. Ekonomika, 61(2), 143–151.
KARAPETYAN, A. & Otieno, R. (2011). A study of knowledge management challenges in project management: Case of start-up projects in Swedish incubators. Umea School of Business, Umea University.
KEH, H.T., Nguyen, T.T.M. & Ng, H.P. (2007). The effects of entrepreneurial orientation and marketing information on the performance of SMEs. Journal of Business Venturing, 22(4), 592-611.
KOWALSKA-STYCZEŃ, A., Malarz, K., Paradowski, K. (2018). Model of knowledge transfer within an organisation. Journal of Artificial Societies and Social Simulation, 21(2), 3.
LAI, W.-H. & Lin, C.-C. (2015). Constructing business incubation service capabilities for tenants at post-entrepreneurial phase. Journal of Business Research, 68(11), 2285-2289.
LANT, T.K. & Mezias, S.J. (1990). Managing discontinuous change: A simulation study of organizational learning and entrepreneurship. Strategic Management Journal, 11, 147-179.
LASRADO, V., Sivo, S., Ford, C., O’Neal, T., Garibay, I., 2016. Do graduated university incubator firms benefit from their relationship with university incubators? Journal of Technology Transfer, 41(2), 205-219.
MARCH, J.G. (1991). Exploration and exploitation in organizational learning. Organization Science, 2(1), 71-87.
MARTÍNEZ-PLUMED, F., Ferri, C., Hernández-Orallo, J., & Ramírez-Quintana, M.J. (2015). Knowledge acquisition with forgetting: an incremental and developmental setting. Adaptive Behavior, 23(5), 283-299.
MIAN, S., Lamine, W. & Fayolle, A. (2016). Technology business incubation: an overview of the state of knowledge. Technovation, 50, 1-12.
MILLER, K.D. & Martignoni, D. (2016). Organizational learning with forgetting: Reconsidering the exploration–exploitation tradeoff. Strategic Organization, 14(1), 53–72.
MILLER, K.D., Zhao, M. & Calantone, R.J. (2006). Adding interpersonal learning and tacit knowledge to March's exploration-exploitation model. Academy of Management Journal, 49(4), 709-722.
NBIA (2014). Business incubation FAQ. Available at: https://www.nbia.org/resource_library/faq/.
NAMWONG, K., Jhundra-Indra, P. & Raksong, S. (2015). Dynamic organizational learning strategy and firm survival: A conceptual model. In: Allied Academies International Conference. Academy of Organizational Culture, Communications and Conflict. Proceedings vol. 20, pp. 12.
PANSUPPAWATT, P. & Ussahawanitchakit, P. (2011). Strategic organizational creativity of medical and cosmetic businesses in Thailand: An empirical investigation of the antecedents and consequences. International Journal of Strategic Management, 11(2), 1-25.
PENA, I., 2004. Business incubation centers and new firm growth in the Basque Country. Small Business Economics, 22(3-4), 223-236.
PERDOMO, G., Alvarez, C. & Urbano, D. (2014). Analyzing a successful incubator business model: The case of Barcelona Activa. Strategies in E-Business, (pp. 39-54). Berlin/Heidelberg: Springer.
PORTER, M. & Kramer, M.R. (2011). Creating shared value: How to reinvent capitalism and unleash a wave of innovation and growth. Harvard Business Review, 89, 62-77.
ROTHAERMEL, F.T., Thursby, M., 2005. University–incubator firm knowledge flows: assessing their impact on incubator firm performance. Research Policy, 34(3), 305-320.
RUBIN, T.H., Aas, T.H. & Stead, A., 2015. Knowledge flow in technological business incubators: evidence from Australia and Israel. Technovation 41, 11-24.
SAKI, S., Shakiba, H. & Savari, M. (2013). Study of the relationship between the organizational learning and organizational innovation at University of Tehran. E Journal of Organizational Learning & Leadership, 11(1).
SHERMAN, H.D., 1999. Assessing the intervention effectiveness of business incubation programs on new business start-ups. Journal of Developmental Entrepreneurship, 4(2), 117-133.
SLATER, S.F. & Narver, J.C. (1995). Market orientation and the learning organization. Journal of Marketing, 59(3), 63-74.
STUDDARD, N.L. (2006). The effectiveness of entrepreneurial firm’s knowledge acquisition from a business incubator. International Entrepreneurship and Management Journal, 2(2), 211-225.
TEMPERLI, R. & Siebert, S. (2012). Knowledge Sharing in a Business Incubator. Lunds Universitet.
TRAN, K., Gibson A. & Wong, D., et al. (2014). Designing a low-cost multifunctional infant incubator [J]. Journal of Laboratory Automation, 19(3), 332-337.
VON Zedtwitz, M.V. & Grimaldi, R. (2006). Are service profiles incubator-specific? Results from an empirical investigation in Italy. Journal of Technology Transfer, 31(4), 459-468.
WALL, F. (2018). Emergence of task formation in organizations: balancing units' competence and capacity. Journal of Artificial Societies and Social Simulation, 21(12), 6.
WARREN, L., Patton, D., & Bream, D. (2009). Knowledge acquisition processes during the incubation of new high technology firms. International Entrepreneurship and Management Journal, 5, 481.
WONG, P.S.P., & Lam, K.Y. (2012). Facing turbulence: driving force for construction organizations to regain unlearning and learning traction. Journal of Construction Engineering and Management, 138(10), 1202–1211.
ZHANG, H., Wu, W. & Zhao, L. (2016). A study of knowledge supernetworks and network robustness in different business incubators. Physica A: Statistical Mechanics and Its Applications, 447, 545-560.
ZHAO, Y., Li, Y., Lee, S.H., & Bo Chen, L. (2011). Entrepreneurial orientation, organizational learning, and performance: Evidence from China. Entrepreneurship Theory and Practice, 35(2), 293–317.